Neural circuit-wide analysis of changes to gene expression during deafening-induced birdsong destabilization
Figures
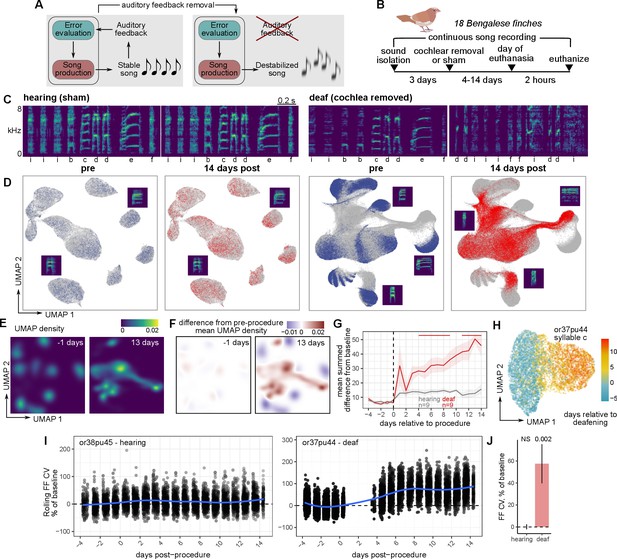
Rapid and global destabilization of the song following deafening.
(A) Song destabilization through the removal of auditory feedback. Adult songbirds use auditory feedback to evaluate their own song production and maintain song quality and consistency. Loss of auditory feedback results in the gradual destabilization of song. (B) Experimental overview. After a baseline period of song recording, Bengalese finches (Lonchura striata domestica) were either deafened through bilateral cochlear removal or underwent a sham surgery. After 4, 9, or 14 days post-surgery, birds were euthanized for gene expression analysis. Bengalese finch graphic obtained from Sainburg, 2020a. (C) Example spectrograms from one hearing (sham) and one deaf (bilateral cochlear removal) bird. Songs are shown from before the procedure and 14 days following the procedure. Labels below each spectrogram correspond to discrete categories of song units (‘syllables’). kHz, kiloHertz. (D) Uniform Manifold Approximation and Projection (UMAP) representation of syllable spectrograms (see Methods) across the entire recording period for each bird (4 days before to 14 days after the procedure). Data are split into ‘pre’-procedure (4–1 day before surgery) and ‘post’-procedure (1–14 days after surgery) subsets. For reference, gray points in each plot correspond to data from the other subset. Example syllable spectrograms are placed adjacent to their position in UMAP space. (E) Density plots of UMAP projections for the syllables from one deafened bird (shown in panel (A)) at two timepoints, one day before and 13 days after deafening. (F) Subtraction of UMAP densities in (E) from the average pre-procedure density. (G) Mean sum of UMAP density differences for syllables from birds that were either deafened (deaf) or underwent a sham surgery (hearing). For each bird and each day, positive UMAP density differences were summed and then averaged across birds. Error bands are standard errors of the mean. Color bars indicate days in which values were significantly different between deaf and hearing birds (Student’s t-test, two-sided, p<0.05). (H) UMAP plot of one syllable from one deafened bird colored by the day following deafening. (I) Comparison of fundamental frequency (FF) variability between hearing and deafened birds. Rolling coefficient of variation (CV, window size 11 syllables) was calculated for the fundamental frequencies of each harmonic stack for each bird. Shown are two example syllables from one hearing and one deafened bird, plotted across the number of days relative to the procedure date (sham or cochlear removal). (J) Mean FF CV in the 7–9 days following sham or cochlear removal normalized to FF CV in the 2 days before the procedure. Linear mixed-effects regression (see Methods) was used to estimate the group post vs. pre-procedure FF CV difference for hearing and deaf birds. P-values are obtained from the regression model using Satterthwaite’s degrees of freedom method.
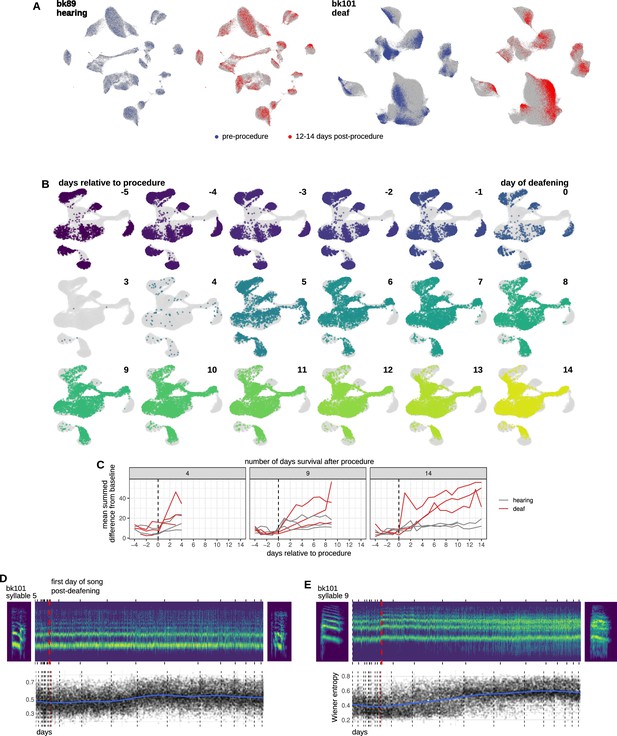
Additional quantification of deafening-induced changes to the song.
(A) Uniform manifold approximate projection (UMAP) representation of syllable spectrograms (see Methods) across the entire recording period (4 days before to 14 days after the procedure) for an example hearing (bk89) and deafened (bk101) bird. Data are split into ‘pre’-procedure (4–1 day before surgery) and ‘post’-procedure (12–14 days after surgery) subsets. For reference, gray points in each plot correspond to data from other days. Example syllable spectrograms are placed adjacent to their position in UMAP space. (B) UMAP representations of syllable spectrograms from a deafened bird split by day relative to cochlear removal. Data used is the same that is presented in Figure 1C and D. (C) Break out the UMAP distance analysis from Figure 1G into individual birds. (D) Increase in syllable noise over time following deafening. Example spectrograms for two syllables from the day before (left) and 14 days following (right) deafening. Spectrograms were averaged along the time axis within red-boxed regions shown in the examples and plotted over time. Vertical dashed red line indicates the day of deafening. Tick marks indicate the beginning of each day. Shown is 20% of the total number of syllables. (E) Spectral flatness (also known as Wiener entropy) over time. Measure was z-scored relative to pre-procedure. Vertical dashed red line indicates the day of deafening. Vertical dashed black lines indicate the beginning of each day.
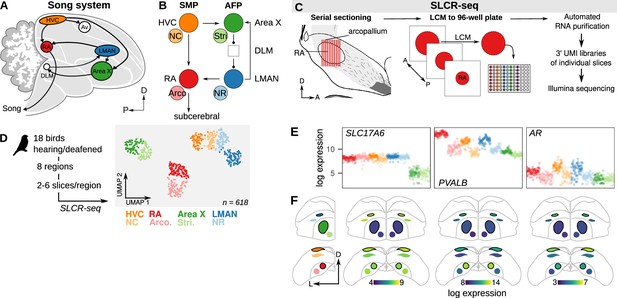
Neural circuit transcriptomics using Serial Laser Capture RNA-sequencing (SLCR-seq).
(A) Schematic overview of the song system. HVC, proper name; RA, robust nucleus of the arcopallium; LMAN, lateral magnocellular nucleus of the nidopallium; Av, avalanche; DLM, medial portion of the dorsolateral thalamic nucleus; D, dorsal; P, posterior. (B) Circuit diagram of the song system. Arrowheads and closed circles indicate excitatory and inhibitory connections, respectively. NC, caudal nidopallium; Arco., arcopallium; NR, rostral nidopallium; Stri., striatum. (C) Schematic of SLCR-seq. Fresh-frozen brains were cryosectioned for Laser Capture Microdissection (LCM). Individual sections of regions of interest were collected into wells of 96-well plates, and then total RNA was purified using an optimized solid phase reversible immobilization (SPRI) protocol. After RNA purification, 3’-end sequencing libraries were prepared containing unique molecular identifiers (UMI) using a custom protocol. (D) Left: Experimental overview of SLCR-seq on hearing and deaf birds. After a baseline period of song recording, birds were either deafened through bilateral cochlear removal or underwent a sham surgery. After 4, 9, or 14 days post-surgery, birds were euthanized and SLCR-seq libraries were prepared from HVC, NC, RA, Arco., LMAN, NR, Area X, and Stri. Right: Uniform Manifold Approximation and Projection (UMAP) plot of SLCR-seq data colored by section position. Each point reflects the gene expression profile of a single SLCR-seq sample. Samples show segregation by broad anatomical area — striatal (Area X), nidopallial (HVC, NC, LMAN, NR), arcopallial (RA, Arco.) — and song system nuclei from surrounding areas. (E) Normalized log gene expression data of three example genes — SLC17A6, PVALB, and AR. Each point is gene expression in a single SLCR-seq sample. SLC17A6 is a marker for glutamatergic cells and is distinctly depleted in the striatal samples; PVALB and AR are two genes known to be enriched in song system nuclei. (F) Coronal anatomical atlas representation of the expression of the three genes shown in panel (F). Each region is colored according to the log gene expression value. D, dorsal; L, lateral.
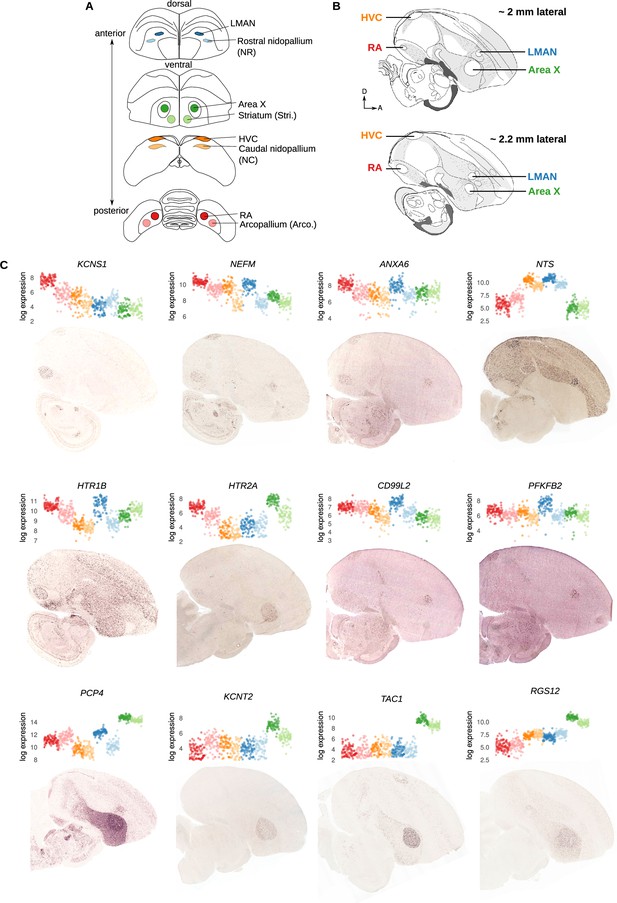
Additional validation of Serial Laser Capture RNA-seq (SLCR-seq) data.
(A) Coronal sections of the finch brain showing collection locations for SLCR-seq. Each song region was identified by its position relative to anatomical landmarks, size, and characteristic Nissl staining. For each song nucleus, a region of identical size was collected outside of the nucleus in the same anatomical territory. ‘Arco.’ collection region refers to the region lateral to RA encompassing AId (dorsal part of the intermediate arcopallium) and AD (dorsal arcopallium). ‘NC’ (caudal nidopallium) collection region lies ventral to HVC outside of the HVC shelf. ‘NR’ (rostral nidopallium) collection region lies lateral to LMAN within the nidopallium. ‘Stri.’ (striatum) lies medial and ventral to Area X. (B) Sagittal schematics of the zebra finch brain retrieved from the ZEBrA database (http://www.zebrafinchatlas.org)(Lovell et al., 2020). Shown are two sections ~2 and ~2.2 mm lateral from the midline. Positions of RA, HVC, LMAN, and Area X are indicated. In situ hybridization images in panel C correspond to these representative sections. D, dorsal; A, anterior. (C) SLCR-seq and in situ hybridization (ISH) expression for selected marker genes with differential expression in the song system. Each subpanel contains at the top, SLCR-seq log expression values where each point is a single SLCR-seq section. Points are colored and ordered by collection region. On the bottom is a representative ISH of the corresponding gene on a sagittal zebra finch brain section, obtained from the ZEBrA database.
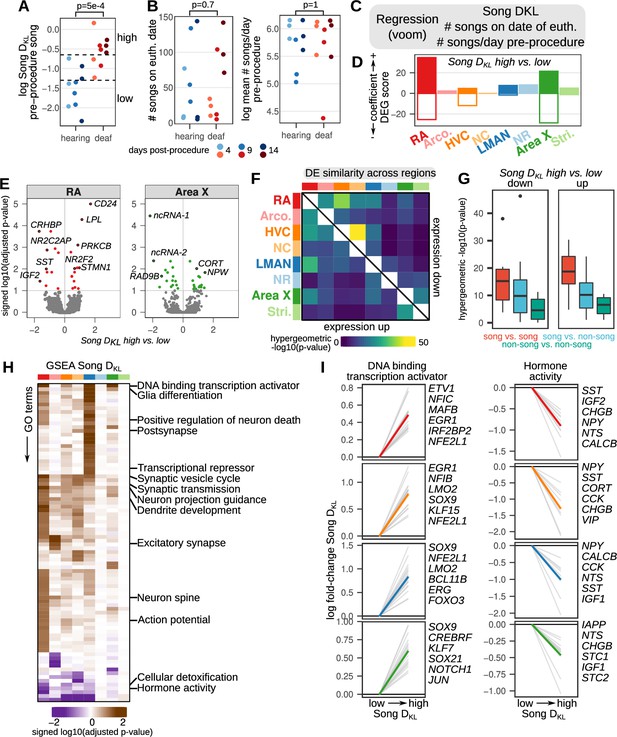
Song destabilization is associated with song system-wide alterations to gene expression.
(A) Relative spectral distance between syllables pre- and post-procedure, represented as the mean Kullback-Leibler (KL) distance between Gaussian mixture models or ‘Song DKL’ (see Methods for calculation). Song DKL trends higher with increasing days from deafening. Significance was calculated using a two-sided Wilcoxon rank-sum test. (B) (left) Number of songs sung on euthanasia date (i.e. within the two hour period between lights-on and euthanasia) and (right) log mean number of songs sung per day pre-procedure for each bird grouped by hearing or deaf. Significance was calculated using a two-sided Wilcoxon rank-sum test. (C) Differential expression analysis of song destabilization. Multiple regression using voom/limma provided estimates of gene expression fold change with variation in song deviation (Song DKL), number of songs on the day of euthanasia, and baseline differences in singing rate. (D) Differential expression gene (DEG) scores from gene expression regressions. Positive values reflect genes with increased expression, while negative values indicate genes with reduced expression. Scores are the sum of the –1 * log10(adjusted p-values) of high vs. low Song DKL regression coefficients. Each score is multiplied by the sign of the coefficient to obtain a signed value. Separate coefficients were estimated for each neural region. (E) Volcano plots of –1 * log10(adjusted p-values) versus the log fold-change of gene expression in RA and Area X in high vs. low Song DKL birds. Signed adjusted p-values above five were assigned values of five to aid visualization. Labeled are the 10 genes with the highest signed adjusted p-value. ‘ncRNA-1’ accession is LOC116184561, ‘ncRNA-2’ accession is LOC116183441. (F) Similarity of song destabilization differential gene expression across the song system and surrounding regions. Heatmaps show the -log10(p-value) from hypergeometric tests comparing the expected versus observed overlap of the top 250 differentially expressed genes for each compared region, divided into genes with increased expression with song destabilization (lower left triangle) and those with decreased expression (upper right triangle). (G) Distribution of values from (F) comparing song versus song regions, song versus non-song regions, and non-song versus non-song regions. Box middle is the median, box upper and lower bounds are the 25th and 75th percentile, and whisker ends lie at 1.5 times the inter-quartile range. (H) Gene set enrichment analysis (GSEA) of song destabilization-associated genes. Shown are the Gene Ontology (GO) terms that are significant in at least one song or non-song region (adjusted p-value <0.1, see Methods). Heatmap represents the signed log10(adjusted p-value) for each GO term and region, with the sign indicating that a given term is associated with increased or decreased expression in Song DKL high versus low birds. Terms are ordered by hierarchical clustering (euclidean distance, Ward squared method). Representative terms are listed for each cluster. (I) Song destabilization gene expression responses of genes in two gene sets — ‘DNA binding transcription activator’ (GO:0001216) and ‘Hormone activity’ (GO:0005179) — that have differential expression across song regions. Shown are the top 20 leading edge genes from GSEA (gray lines) and the top six of these are labeled at right. The mean expression change for these 20 genes is shown as a colored line in each panel.
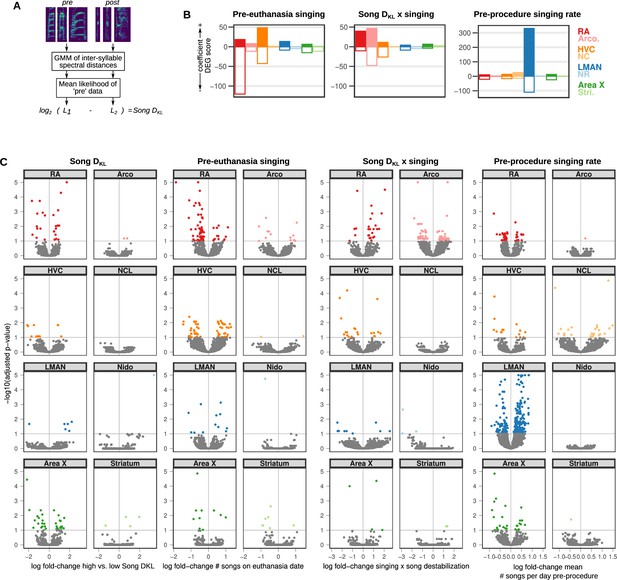
Extended analysis of song destabilization-associated gene expression.
(A) Schematic of Song DKL calculation. GMM, gaussian mixture model. See Methods under “‘Song DKL’ and (Mets and Brainard, 2018) for details. (B) Differential expression gene (DEG) scores from voom regression. Scores are the sum of the –1 * log10(adjusted p-values) of regression coefficients. Each score is multiplied by the sign of the coefficient to obtain a signed value. Coefficients shown are pre-euthanasia singing (# of songs sung on euthanasia date), Song DKL × singing (interaction term), and pre-procedure singing rate (mean number of songs sung per day during pre-procedure days). (C) Volcano plots of –1 * log10(adjusted p-values) versus the log fold-change of gene expression in RA for high vs. low Song DKL (‘Song DKL’), the number of songs sung on the day of euthanasia (‘Pre-euthanasia singing’), the interaction between Song DKL and the number of songs sung on the day of euthanasia (‘Song DKL × singing’), and the mean number of songs sung per day during pre-procedure days (‘Pre-procedure singing rate’). Signed adjusted p-values above five were assigned values of five to aid visualization.
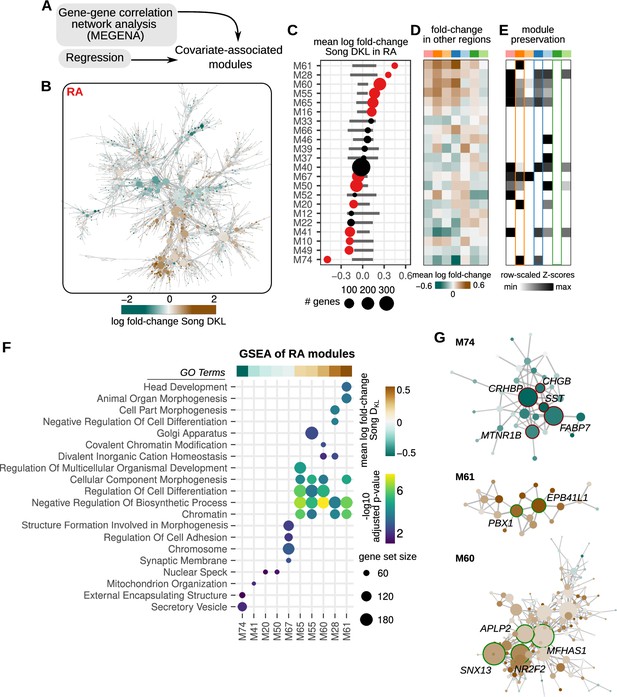
Correlated modules of gene expression associated with song destabilization.
(A) To identify correlated patterns of gene expression, gene-gene correlation networks were constructed for each region using MEGENA (Multiscale Embedded Gene Co-expression Network Analysis). These networks were then used to identify correlated sets of gene modules. Estimated regression coefficients were mapped onto correlation networks to identify covariate-associated expression modules. (B) Gene-gene correlation network for RA. Each node is colored by the log fold-change expression between deaf and hearing birds. (C) Average song destabilization gene expression changes for each RA module. Error bars are null distributions generated by repeatedly sampling the network (100 times) for the number of nodes in a given module and then averaging their high vs. low Song DKL fold-changes. Dots that are colored have mean coefficient values that are lower or higher than 1% or 99% of the sampled distribution, respectively. (D) Average change in expression of RA modules across each song system and non-song system region. (E) Preservation scores for RA modules in the correlation networks of other song and non-song system regions. Only significant values are shown (Bonferroni p-values <0.01), and values are scaled to the maximum and minimum for each module to show relative levels of preservation across regions. (F) Gene set enrichment analysis (GSEA) of RA modules with significant gene expression alteration with song destabilization. Mean fold-change values for each module, as represented in (B), are shown at the top of the GSEA plot. Shown are at most the top five significant Gene Ontology (GO) terms (GSEA adjusted p-values <0.2). (G) Network diagrams for three modules (M61, M60, and M74) that show large deviations with song destabilization. Labeled and highlighted are selected hub genes for each module (see Methods for classification). Node colors indicate log fold-change expression between deaf and hearing birds (scale given in (A)).
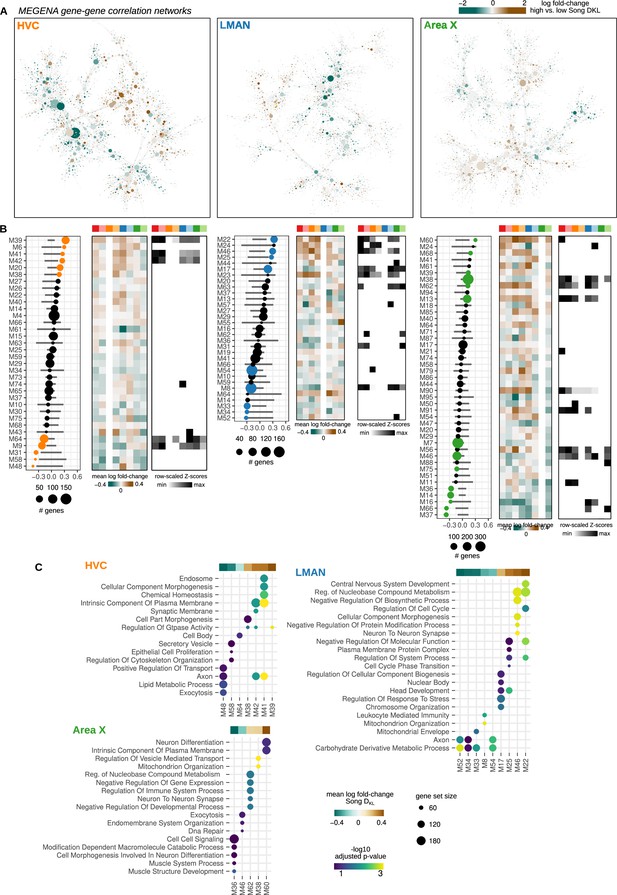
Network analysis of song destabilization-associated gene expression.
(A) Multiscale Embedded Gene Co-expression Network Analysis (MEGENA) gene-gene correlation network for HVC (proper name), LMAN (lateral magnocellular nucleus of the anterior nidopallium), and Area X. Each node is colored by the log fold-change expression between high and low Song DKL groups. (B) Average differential expression (left), patterns of differential expression across regions (middle), and preservation scores (right) for the modules identified in the HVC, LMAN, and Area X networks. Analysis and figure presentation are as described in Figure 4C–E. (C) Gene set enrichment analysis (GSEA) of HVC, LMAN, and Area X modules with significant gene expression alteration with song destabilization. Mean fold-change values for each module, as represented in Figure 4—figure supplement 1B, are shown at the top of the GSEA plot. Shown are at most the top five significant Gene Ontology (GO) terms (GSEA adjusted p-values <0.2).
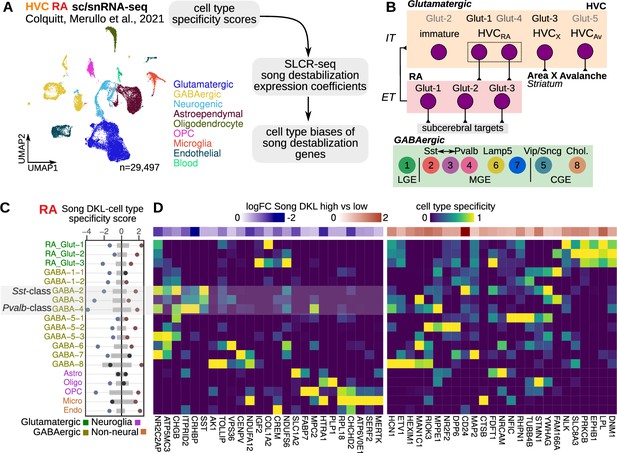
Cell-type specificity of destabilization-modulated genes.
(A) Schematic of the approach to determine the cell type expression biases of genes that are differentially regulated with song destabilization. A previously generated cell-resolved gene expression dataset for RA (robust nucleus of the arcopallium) and HVC (proper name) (Colquitt et al., 2021) was combined with RA and HVC song destabilization regression coefficients from this study to compute a cell-type bias score (see Methods). Shown also is a uniform manifold approximate projection (UMAP) plot of the full dataset with major cell type groups indicated. OPC, oligodendrocyte precursor cell. (B) Schematic of neuronal cell types in the song motor pathway, as previously defined in Colquitt et al., 2021. HVC glutamatergic neurons are broadly similar to intratelencephalic (IT) mammalian neocortical neurons from multiple layers, and RA neurons are similar to extratelencephalic (ET) neurons from layer 5. Eight primary GABAergic clusters are found equally in both HVC and RA and are organized into clusters corresponding to subpallial regions of origin — lateral, medial, and caudal ganglionic eminences (LGE/MGE/CGE). The LGE-class GABA-1 has no known correspondence with mammalian neocortical neurons; GABA-2 is transcriptionally similar to Sst-class neurons, GABA-4 is similar to Pvalb-class neurons, and GABA-3 is transcriptionally intermediate between GABA-2/4. (C) Integration of cell type specificity scores and song destabilization differential expression to identify cell type-associated transcriptional effects of song destabilization. For the top 50 differentially expressed genes, cell type specificity was multiplied by log fold-change between high and low Song DKL birds. These values were then split by sign then summed within each cell type to yield a cell type Song DKL score. Gray bars indicate the distribution (1–99%) of Song DKL-cell type specificity scores for 100 random sets of 50 genes. (D) RA cell type specificity scores for top Song DKL differentially expressed genes, divided into upregulated and downregulated genes. Values are scaled for each gene such that the cell type with the highest specificity score equals 1 and that with the lowest equals 0. At the top of each specificity score heatmap is the log fold-change expression for high vs. low Song DKL.
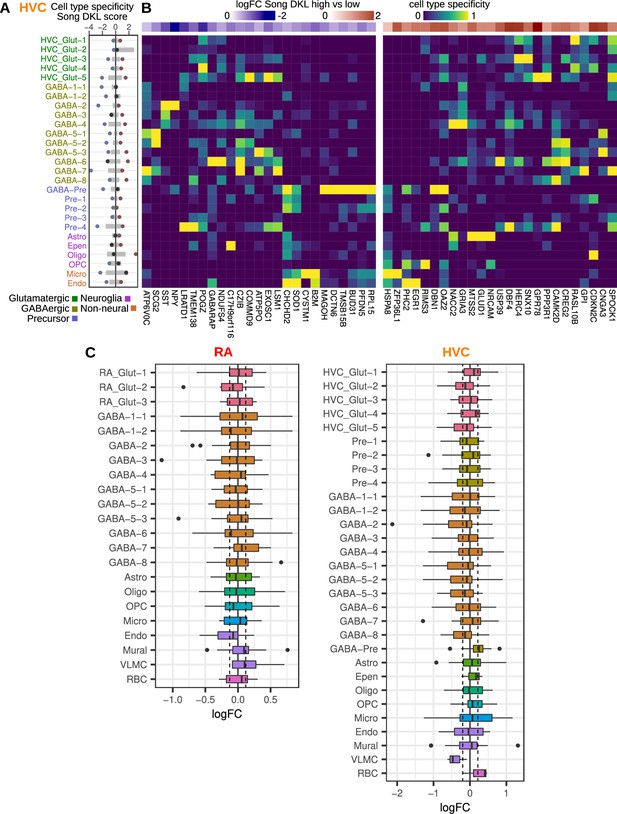
Cell type-associated differential expression.
(A) Integration of cell type specificity scores and Song DKL coefficients to identify cell type-associated transcriptional effects of song destabilization in HVC (proper name). For the top 50 differentially expressed genes, cell type specificity was multiplied by its log fold-change between high and low Song DKL birds. These values were then split by sign then summed within each cell type to yield a cell type Song DKL score. Gray bars indicate the distribution (1–99%) of Song DKL-cell type specificity scores for 100 random sets of 50 genes. (B) HVC cell type specificity scores for top Song DKL differentially expressed genes, divided into upregulated and downregulated genes. Values are scaled for each gene such that the cell type with the highest specificity score equals 1 and that with the lowest equals 0. At the top of each specificity score heatmap is the log fold-change expression for high vs. low Song DKL. (C) Distributions of Song DKL high vs. low log fold-change for the top 25 marker genes for each cell type in RA (robust nucleus of the arcopallium) and HVC. Dashed lines indicate the 1% and 99% median log fold-change for 100 randomly selected sets of 25 genes.
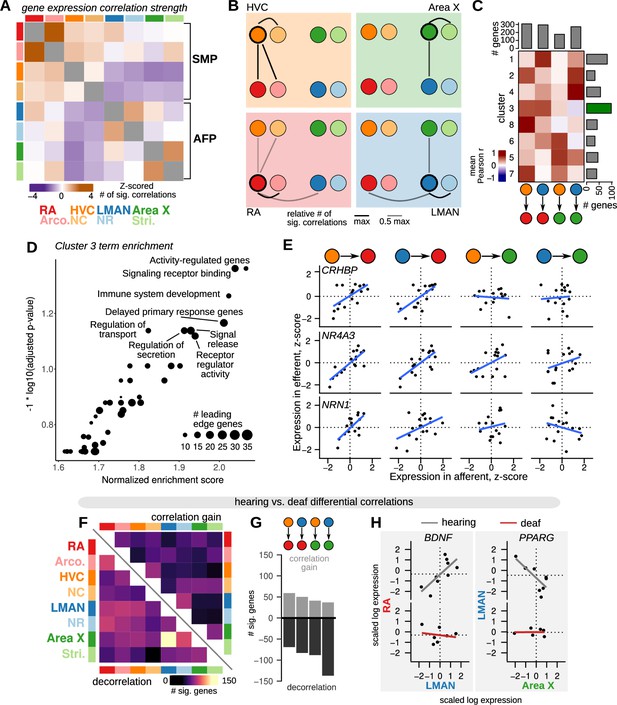
Inter-region gene expression correlation and decorrelation with song destabilization.
(A) Inter-region gene expression correlations across song and non-song regions. For each region, the 500 genes with the highest variability across birds were selected (see Methods), then the expression of each gene was correlated across regions. Significant genes were called as those with an observed correlation less than 1% or greater than 99% of a shuffled correlation distribution (100 shuffles, calculated for each pairwise comparison between regions). The number of significantly correlated genes was Z-scored across the set of pairwise comparisons to highlight the relative strength of inter-region expression correlations. Within-region correlations were excluded from the Z-scoring and are colored gray. (B) Representation of the data in (A) showing the strength of gene expression correlation between each song system nucleus and other assayed regions. (C) Patterns of inter-region gene expression correlations. Genes were clustered into eight clusters by their pairwise correlation values between HVC-RA, LMAN-RA, HVC-Area X, and LMAN-Area X. Heatmap shows mean correlations within each cluster (rows) and region comparison (columns). Barplots represent the number of genes in each row or column. Highlighted is cluster 3, the HVC-RA and LMAN-RA correlation cluster, which has the greatest number of genes. (D) Gene set enrichment analysis (GSEA) indicates that cluster 3 is enriched for genes that are activity-dependent and have a signaling-related function. (E) Expression of three cluster 3 genes across pairs of song system regions with direct projections — HVC to RA, LMAN to RA, HVC to Area X, and LMAN to Area X. Each point is the z-scored expression estimate for each nucleus in one bird. (F) Schematic of inter-region gene expression differential correlation analysis between hearing and deaf birds. For each gene, region pair, and condition, a Pearson correlation was calculated, then a differential correlation was calculated as the difference between unsigned correlations for hearing versus deaf conditions. To determine significance, 100 random permutations of the expression data were made for each gene, region pair, and condition and differential correlation was computed in the same manner as for the observed values. Genes with observed differential correlations in the top or bottom 2.5% of the shuffled distribution were considered significant. (G) Analysis of inter-region gene expression correlations compared between hearing and deaf birds. Heatmap shows the number of genes that became decorrelated (bottom-left) or gained correlation (top-right) in deaf versus hearing birds. (H) Total number of genes that show significant correlation gain or decorrelation in the four pairs of assayed song system regions with direct projections. (I) Examples of genes that show decorrelation in deafened birds relative to hearing birds. BDNF (brain-derived neurotrophic factor) expression is correlated between RA (y-axis) and LMAN (x-axis) in hearing birds but shows no inter-region correlation in deafened birds. Similarly, PPARG expression is correlated between LMAN (y-axis) and Area X (x-axis) in hearing birds but is uncorrelated in deaf birds. Each point is the z-scored expression estimate for one bird.
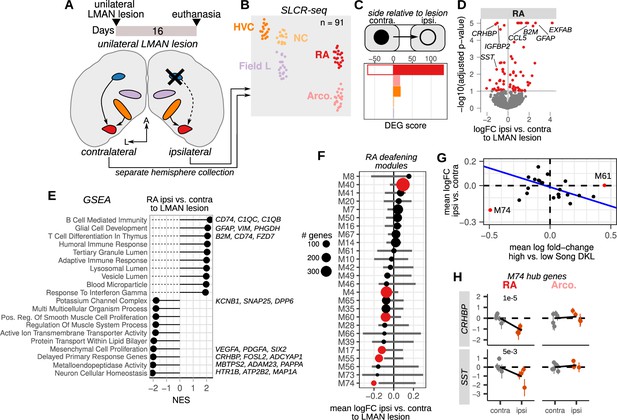
Loss of afferent input to the motor pathway nucleus RA (robust nucleus of the arcopallium) alters destabilization-associated gene expression.
(A) Schematic of unilateral LMAN (lateral magnocellular nucleus of the anterior nidopallium) lesions and sample collection for Serial Laser Capture RNA-seq (SLCR-seq). Five birds received unilateral LMAN lesions (three left and two right hemisphere). After 16 days, birds were euthanized, and HVC (proper name), NC, RA, arcopallium (Arco.), and the primary auditory region Field L were collected for SLCR-seq. Each hemisphere was processed separately to examine the ipsilateral versus the contralateral influence of LMAN lesioning on gene expression in hearing birds. (B) UMAP plot of SLCR-seq samples colored by region. (C) Within-bird differential expression analysis of the influence of LMAN lesions on different regions of the songbird brain. Shown are differential expression gene (DEG) scores for each region assayed. Positive values reflect genes with increased expression, while negative values indicate genes with reduced expression. DEG scores are calculated as the sum of the –1 * log10(adjusted p-values) of regression coefficients for gene expression in brain regions ipsilateral versus contralateral to the LMAN lesion. Each score is multiplied by the sign of the coefficient to obtain a signed value. Separate coefficients were estimated for each neural region. (D) Volcano plot showing the genes that had the most significant difference in expression (red points) between RA on the ipsilateral versus the contralateral side of LMAN lesion, quantified as –1 * log10(adjusted p-values) versus the log fold-change of gene expression for RA ipsilateral versus contralateral to the LMAN lesion. Signed adjusted p-values above five were assigned values of five to aid visualization. (E) Gene set enrichment analysis of differential expression in RA ipsilateral versus contralateral to the LMAN lesion side. Top leading edge genes are listed at right. NES, normalized enrichment score. (F) Average expression change between ipsilateral and contralateral RA for each Song DKL module that was identified in Figure 4. Error bars are null distributions generated by repeatedly sampling the network (100 times) for the number of nodes in a given module and then averaging their differential ipsilateral versus contralateral coefficients. Dots are colored that have mean coefficient values that are lower or higher than 1% or 99% of the sampled distribution, respectively. (G) Comparison of mean fold-change expression differences in RA between high-vs-low Song DKL and contralateral versus ipsilateral to LMAN lesions. Blue line indicates linear regression through the data after excluding outlier modules M74 and M61. (H) Expression of two M74 hub genes, CRHBP and SST, between RA and Arco contralateral or ipsilateral to the LMAN lesion. Each dot is the estimated gene expression within a given bird and region, and error bars are standard errors of this estimate. Adjusted p-values were obtained from the ipsilateral versus contralateral regression analysis.

Validation and quantification of unilateral lateral magnocellular nucleus of the anterior nidopallium (LMAN) lesions.
(A) Nissl stains of coronal sections from two example birds with unilateral LMAN lesions in the left hemisphere (bird identification pk63gr79) and right hemisphere (rd88rd70). Percentages indicate the fraction of LMAN lesioned, relative to the size of LMAN on the unlesioned side (see Methods for calculation). (B) LMAN lesion percentages across birds colored by lesion side. Dashed horizontal line indicates the threshold used to consider LMAN as lesioned. (C) Example spectrograms from two birds before and after unilateral LMAN lesion. Percentages indicate LMAN lesion extent as indicated in panels A and B. Lettering below the spectrograms are manually assigned syllable labels. (D) Song DKL of unlesioned hearing and deaf birds (from main deafening dataset) and hearing birds with unilateral LMAN lesion. p-values are from two-sided Wilcoxon rank-sum tests.
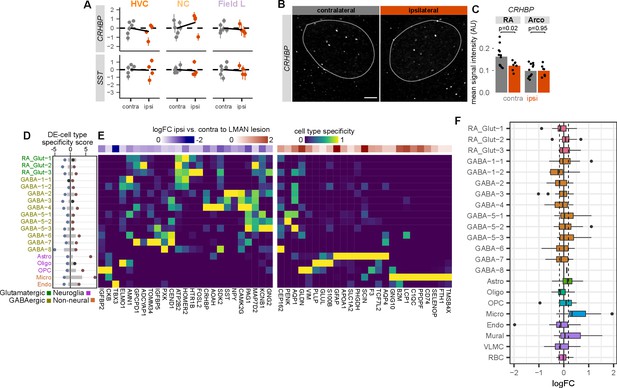
Validation of unilateral lateral magnocellular nucleus of the anterior nidopallium (LMAN) lesion effects on robust nucleus of the arcopallium (RA) gene expression.
(A) Expression of two M74 hub genes, corticotropin-releasing hormone binding protein (CRHBP) and somatostatin (SST), between HVC (proper name), NC, and Field L contralateral or ipsilateral to the LMAN lesion. Each dot is the estimated gene expression within a given bird and region, and error bars are standard errors of this estimate. (B) In situ hybridizations showing expression of CRHBP in RA either ipsilateral or unilateral to LMAN lesion. Scale bar is 100 µm. (C) Quantification of CRHBP signal intensities. Each point is the average ISH intensity in each RA or surrounding arcopallium (lesioned or unlesioned hemisphere) for a given bird. p-values are from a two-sided Student’s t-test. (D) Integration of cell type specificity scores and ipsilateral versus contralateral coefficients to identify cell type-associated transcriptional effects of LMAN lesions. For the top 50 differentially expressed genes, cell type specificity was multiplied by log fold-change between ipsilateral and contralateral RA. These values were then split by sign then summed within each cell type to yield a differential expression (DE)-cell type score. Gray bars indicate the distribution (1–99%) of DE-cell type specificity scores for 100 random sets of 50 genes. (E) RA cell type specificity scores for top differentially expressed genes, divided into upregulated and downregulated genes. Values are scaled for each gene such that the cell type with the highest specificity score equals 1 and that with the lowest equals 0. At the top of each specificity score heatmap is the log fold-change expression for ipsilateral versus contralateral RA. (F) Distributions of Song DKL high vs. low log fold-change for the top 25 marker genes for each cell type. Dashed lines indicate the 1% and 99% median log fold-change for 100 randomly selected sets of 25 genes.
Tables
Reagent type (species) or resource | Designation | Source or reference | Identifiers | Additional information | |
---|---|---|---|---|---|
Biological sample (Lonchura striata domestica) | Brain tissue | Lab animal colony | |||
Sequence-based reagent | RT_primer_v1 | IDT | SLCR-seq primer | AAGCAGTGGTATCAACGCAGAGTA CNNNNNNNNNNNNNNNNNNNNNNN NNXXXXXXTTTTTTTTTTTTTTTTTTT TTTTTTTTTVN | |
Sequence-based reagent | RT_primer_v2 | IDT | SLCR-seq primer | AAGCAGTGGTATCAACGCAGAGTA CNNNNNNNNNNNNNNATCTAGCCGG CCTTTTTTTTTTTTTTTTTTTTTTTTTTTTVN | |
Sequence-based reagent | TSO_LNA | Exiqon | SLCR-seq primer | AAGCAGTGGTATCAACGCAG AGTGAATrGrG +G | |
Sequence-based reagent | TSO_PCR | IDT | SLCR-seq primer | AAGCAGTGGTATCAACGCAGAGT | |
Sequence-based reagent | P5-TSO_Hybrid | IDT | SLCR-seq primer | AATGATACGGCGACCACCGAGAT CTACACGCCTGTCCGCGGAAGCA GTGGTATCAACGCAGAGT*A*C | |
Sequence-based reagent | Read1CustomSeqB | IDT | SLCR-seq primer | GCCTGTCCGCGGAAGCAGTG GTATCAACGCAGAGTAC | |
Sequence-based reagent | PCR2 | IDT | SLCR-seq primer | CAAGCAGAAGACGGCATACGAGA TYYYYYYYYGTCTCGTGGGCTCGG | |
Commercial assay or kit | KAPA HiFi Hotstart | Roche | 7958927001 | ||
Commercial assay or kit | Qubit dsDNA HS | ThermoFisher | Q32851 | ||
Commercial assay or kit | Nextera XT | Illumina | FC-131–1024 | ||
Commercial assay or kit | KAPA Library Quantification Kit | Roche | 07960140001 | ||
Commercial assay or kit | 2% BluePippin Gels | Sage | BEF2010 | ||
Commercial assay or kit | ISH-HCR | Molecular instruments | ISH probes and reagents | ||
Commercial assay or kit | AMPure XP | Beckman Coulter | A63881 | ||
Other | molecule sieve beads | Sigma | 208582 | Used to make anhydrous ethanol solution used in 'SLCR-seq — rapid Nissl stain' | |
Other | cresyl violet powder | Sigma | 255246 | Stain used in 'SLCR-seq — rapid Nissl stain' | |
Other | Guanidine thiocyanate | Sigma | G9277 | Component of lysis solution used for RNA purification in 'SLCR-seq — SPRI RNA purification' | |
Other | Sera-Mag SpeedBeads Carboxyl Magnetic Beads, hydrophobic | FisherScientific | 09-981-123 | Used to create homemade SPRI RNA purification solution, as in 'SLCR-seq — SPRI RNA purification' | |
Other | EvaGreen | Biotium | 13000 | Dye added to library amplification to determine needed number of amplification cycles, as in 'SLCR-seq — library preparation' |
Additional files
-
Supplementary file 1
SLCR-seq barcode and index sequences.
Full sequences for RT_primer_v1, RT_primer_v2, and PCR2.
- https://cdn.elifesciences.org/articles/85970/elife-85970-supp1-v1.xls
-
Supplementary file 2
Deafening voom statistics Fold-change estimates and p-values for voom regression analysis of the deafening SLCR-seq dataset.
- https://cdn.elifesciences.org/articles/85970/elife-85970-supp2-v1.csv
-
Supplementary file 3
GSEA statistics Gene set enrichment analysis of song destabilization-associated genes.
- https://cdn.elifesciences.org/articles/85970/elife-85970-supp3-v1.xlsx
-
Supplementary file 4
Network module memberships Gene memberships in MEGENA modules for the RA, HVC, LMAN, and Area X networks.
- https://cdn.elifesciences.org/articles/85970/elife-85970-supp4-v1.xlsx
-
Supplementary file 5
Differential correlation analysis Inter-region gene correlations results for the combined dataset and split between hearing and deaf birds.
- https://cdn.elifesciences.org/articles/85970/elife-85970-supp5-v1.xlsx
-
Supplementary file 6
Unilateral LMAN lesion voom statistics Fold-change estimates and p-values for voom regression analysis of the unilateral LMAN lesion SLCR-seq dataset.
- https://cdn.elifesciences.org/articles/85970/elife-85970-supp6-v1.txt
-
MDAR checklist
- https://cdn.elifesciences.org/articles/85970/elife-85970-mdarchecklist1-v1.docx