Atypical cognitive training-induced learning and brain plasticity and their relation to insistence on sameness in children with autism
Figures
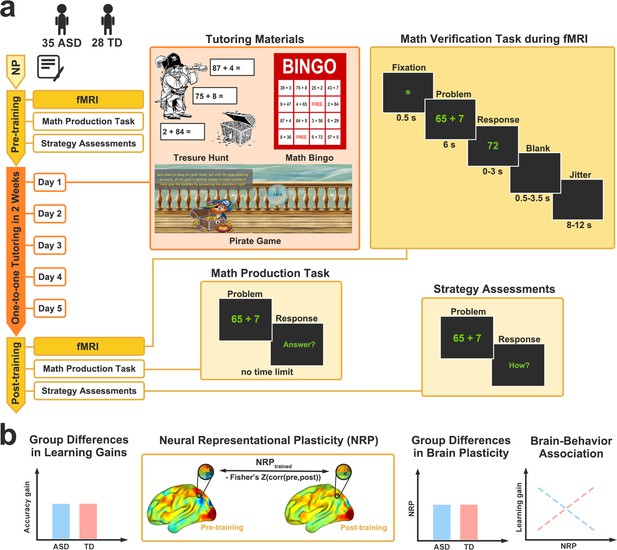
Overview of study design and analysis approach.
(a) Study design. Before training, all eligible children with autism spectrum disorder (ASD) and typically developing (TD) children underwent neuropsychological (NP) assessments, a functional magnetic resonance imaging (fMRI) scan session (math verification task), and tasks outside of the scanner (math production task and strategy assessment). Children completed 5 days of one-to-one math training. On each training day, children completed multiple interactive activities with a tutor, including Treasure Hunt, Math Bingo, and Pirate Game (Materials and methods). After training, children completed a second fMRI scan session and outside-of-scanner tasks. In the math verification task, each trial began with a 500ms fixation cross, followed by a 6 s presentation of a math problem. Participants considered the problem and then indicated if a subsequent solution probe matched their answer. In the math production task, children were required to solve each addition problem by verbalizing their answers. After solving each problem, children’s problem-solving strategies were assessed, in which they were asked to describe how they solved the problem. Each task fMRI run lasted 4 min and 50 s. Children’s performance on trained and untrained (novel, similar-to-trained) problems was assessed by a math verification task during fMRI and a math production task before and after training. Children’s problem-solving strategy use (memory-based or rule-based strategy) for trained and untrained problems was assessed using strategy assessments (Materials and methods). (b) Analysis approach. We first assessed learning, using multiple measures including learning gain, in children with ASD and TD children and then investigated whether cognitive and neural mechanisms of learning are altered in children with ASD, compared to TD children. Training-related brain plasticity was assessed by neural representational plasticity (NRP), multivariate spatial correlation of brain activation patterns between pre- and post-training for trained problems. Brain imaging analyses included comparisons between ASD and TD groups, using both whole-brain and region of interest analysis approaches. Schematic graphs illustrate possible outcomes.
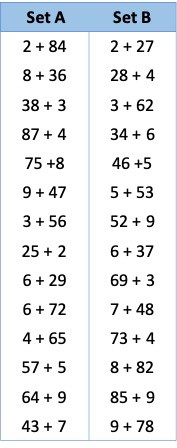
Problem sets used in two tranining problem sets, Set A and Set B.
Problem sets were balanced in structure and task difficulty.
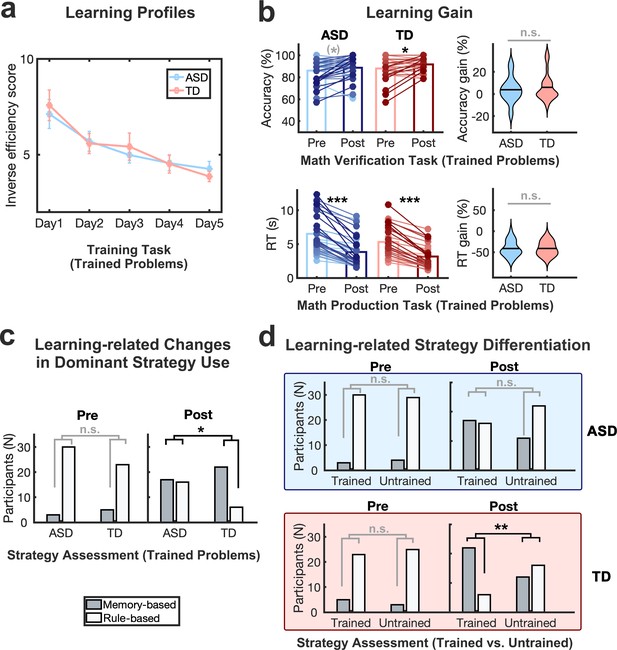
Similar learning outcomes but altered cognitive mechanisms of learning in response to training in children with autism relative to typically developing children.
(a) Learning profiles. Children with autism spectrum disorder (ASD) showed significant improvements in inverse efficiency score (reaction time/accuracy) of the training task from training day 1–5 at a comparable level to typically developing (TD) children. Error bars represent standard errors of the mean. Sample size: n(ASD)=29 and n(TD)=26. (b) Learning gains. Training improved performance on trained problems in children with ASD at comparable levels to TD children, with no significant differences in learning gains between groups in accuracy in math verification task or reaction time (RT) in the math production task. The pair of dots connected by a line represents the performance of the same child at pre- and post-training, with darker blue/red dots/lines indicating greater performance gains. Group means are presented in lighter and darker blue/red bars at pre- and post-training. Sample size for math verification task: n(ASD)=35 and n(TD)=28; Sample size for math production task: n(ASD)=33 and n(TD)=28. (c) Changes in dominant strategy use for trained problems. At pre-training, children with ASD and TD children showed comparable distribution of dominant strategy use (memory-based or rule-based) for trained problems. At post-training, the distribution of dominant strategy use was significantly different between groups for trained problems. While most TD children used the memory-based strategy most frequently following training, nearly half of the children with ASD used rule-based strategies most frequently for trained problems. (d) Differentiation of dominant strategy use between trained and untrained problems. No difference in the distribution of dominant strategy use between types of problems was observed at pre-training. After training, the distribution of strategy use was not significantly distinguishable between trained and untrained problems in children with ASD, whereas TD children reported significantly greater use of memory-retrieval strategy for trained problems compared to untrained problems. Sample size: n(ASD)=33 and n(TD)=28. (*) p<0.1; * p<0.05; ** p<0.01; *** p<0.001; n.s., not significant.
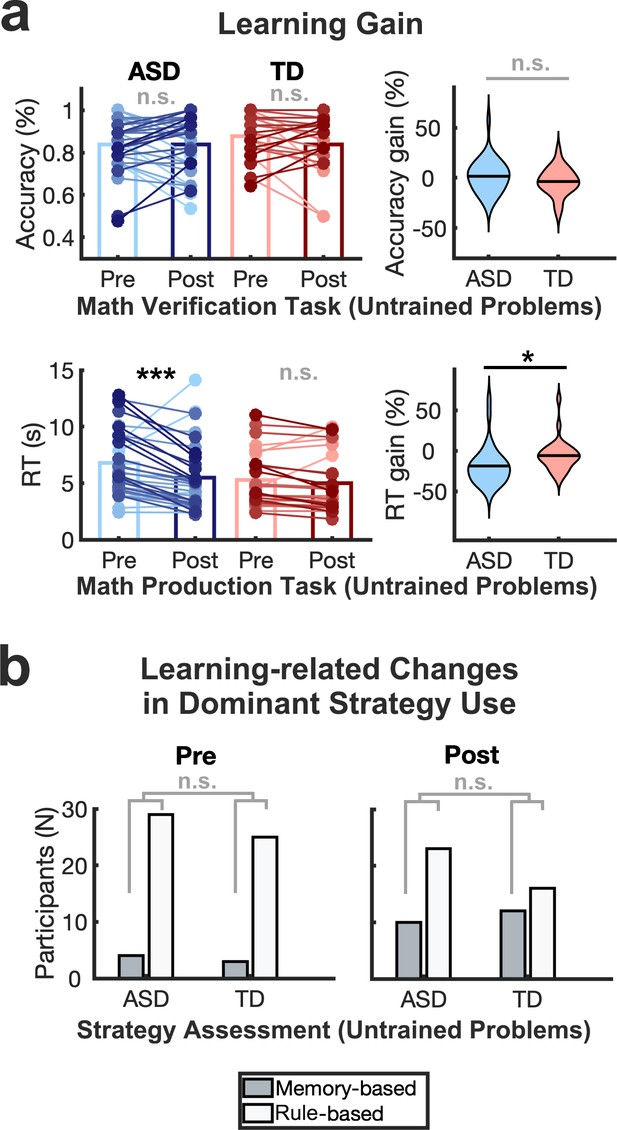
Changes in performance and strategy use for untrained problems in children with autism spectrum disorder (ASD) and typically developing (TD) children in response to training.
(a) Changes in performance. Accuracy for untrained problems of math verification task in the fMRI scanner at pre- and post-training is shown for each group (top). Accuracy gain, measured as percentage of change from pre- to post-training (Accuracygain = (Accuracypost - Accuracypre)/Accuracypre), was not significantly different between groups. Reaction time (RT) for untrained problems of math production task outside the scanner at pre- and post-training is shown for each group (Bottom). RT gain, measured as percentage of change from pre- to post-training (RTgain = (RTpost - RTpre)/RTpre), was significantly different between groups, with children with ASD becoming faster to solve the problems correctly with training while TD children did not significantly change for untrained problems. The pair of dots connected by a line represents the performance of the same child at pre- and post-training, with darker blue/red dots/lines indicating greater gains in performance. The bars indicate the mean value in each group at pre- and post-training (lighter and darker blue/pink). (b) Changes in dominant strategy use for untrained problems. The distribution of participants using memory-based and rule-based strategies as their dominant strategy for untrained problems before and after training is shown for each group. Children with ASD showed similar distribution of dominant strategy use as TD children for untrained problems at pre- and post-training. Sample size: n(ASD)=33 and n(TD)=28. *** p<0.001; ** p<0.01; * p<0.05; n.s., not significant.
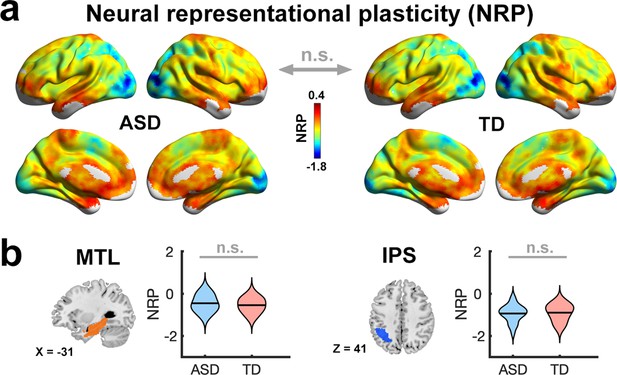
Comparable training-related neural representational plasticity (NRP) in children with autism and typically developing children.
Both (a) whole-brain and (b) region of interest (ROI) multivariate neural representational pattern analysis showed no significant differences in mean NRP across individuals between children with autism spectrum disorder (ASD) and typically developing (TD) children. The results of left hemisphere ROIs are shown here, and the results of right hemisphere ROIs are shown in Figure 3—figure supplement 1. Sample size: n(ASD)=21 and n(TD)=24. MTL, medial temporal lobe; IPS, intraparietal sulcus; n.s., not significant.
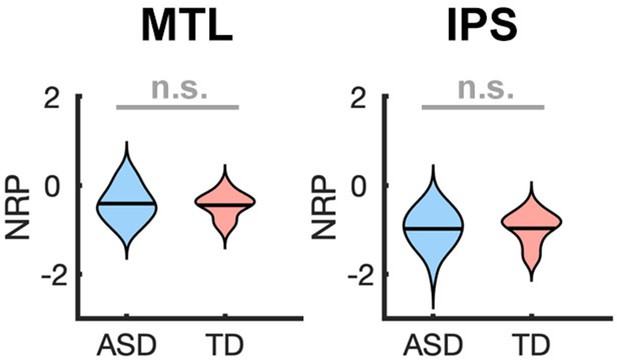
Group differences in neural representation plasticity (NRP) for right hemisphere regions of interest (ROIs).
Mean NRP across individuals in the right medial temporal lobe (MTL) and right intraparietal sulcus (IPS) was comparable between children with ASD and TD children. Sample size: n(ASD)=21 and n(TD)=24. n.s., not significant.
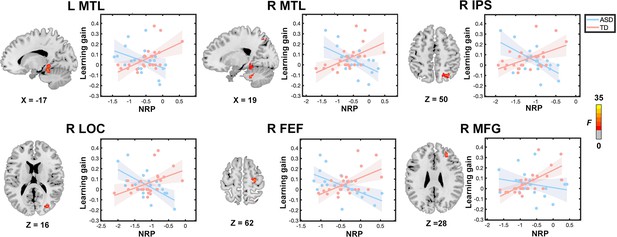
Atypical relation between training-related neural representational plasticity (NRP) and learning gains in children with autism.
Whole-brain multivariate neural representational pattern analysis revealed a significant group by learning gain interaction in the bilateral medial temporal lobe (MTL), right intraparietal sulcus (IPS), right lateral occipital cortex (LOC), right frontal eye field (FEF), and right middle frontal gyrus (MFG). NRP scores were normalized values of correlation between pre- and post-training multiplied by –1. Larger NRP scores indicated a greater degree of training-related brain plasticity, while smaller NRP scores indicated a lower degree of plasticity in neural representation between pre- and post-training, reflecting increased stability of neural representations over time. In typically developing (TD) children, shown in the pink line, greater learning gains were associated with greater NRP. In children with autism spectrum disorder (ASD), shown in the blue line, greater learning gains were associated with lower NRP, indicative of more stable neural representations. NRP in each region identified from the interaction between group and learning gains in whole-brain analysis was extracted from 6 mm spheres centered at peaks for visualization of results. Shaded areas indicate 95% confidence intervals. Sample size: n(ASD)=21 and n(TD)=24.
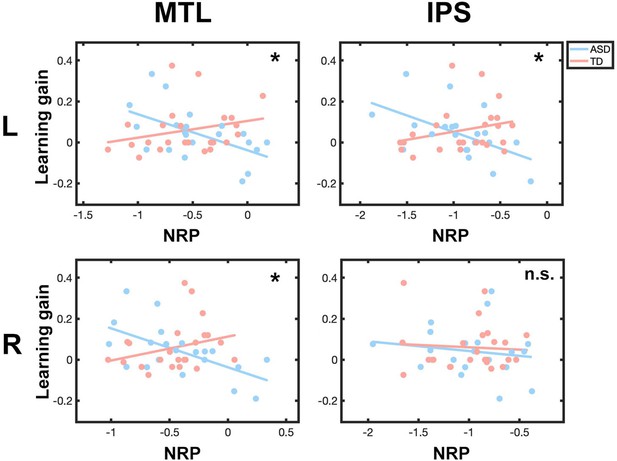
Results of brain-behavior association between region of interest (ROI)-based neural representational plasticity (NRP) and learning gains for trained problems in children with autism spectrum disorder (ASD), compared to typically developing (TD) children.
ROI-based analysis showed significant interaction between group and learning gain on NRP for trained problems in bilateral medial temporal lobe (MTL) and left intraparietal sulcus (IPS). Sample size: n(ASD)=21 and n(TD)=24. L, Left; R, Right; *, p<0.05, FDR-corrected; n.s., not significant.
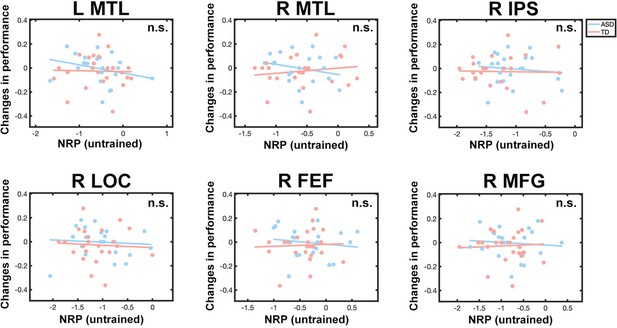
Brain-behavior association between neural representational plasticity (NRP) and changes in performance for untrained problems in children with autism spectrum disorder (ASD) relative to typically developing (TD) children.
Brain regions identified from whole brain analysis for trained problems did not show significant interaction between group and change in performance for untrained problems. Sample size: n(ASD)=21 and n(TD)=24. L, Left; R, Right; MTL, medial temporal lobe; IPS, intraparietal sulcus; LOC, lateral occipital cortex; FEF, frontal eye field; MFG, middle frontal gyrus; NRP, neural representational plasticity; n.s., not significant.
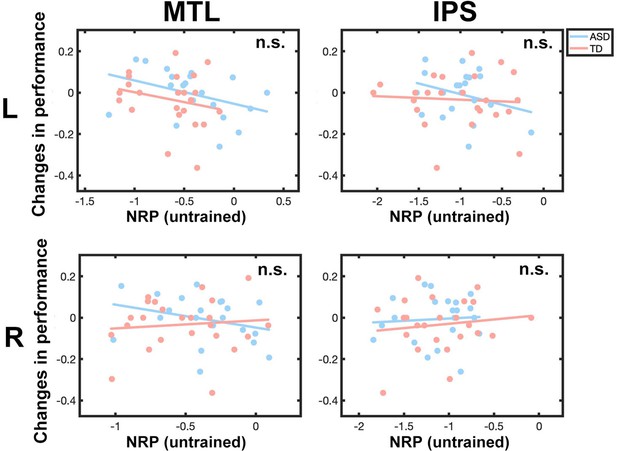
Brain-behavior association between region of interest (ROI)-based neural representational plasticity (NRP) and changes in performance for untrained problems in children with autism spectrum disorder (ASD) relative to typically developing (TD) children.
ROI-based analysis did not show significant interaction between group and changes in performance for untrained problems in regions related to math learning, including medial temporal lobe (MTL) and intraparietal sulcus (IPS). Sample size: n(ASD)=21 and n(TD)=24. L, Left; R, Right; n.s., not significant.
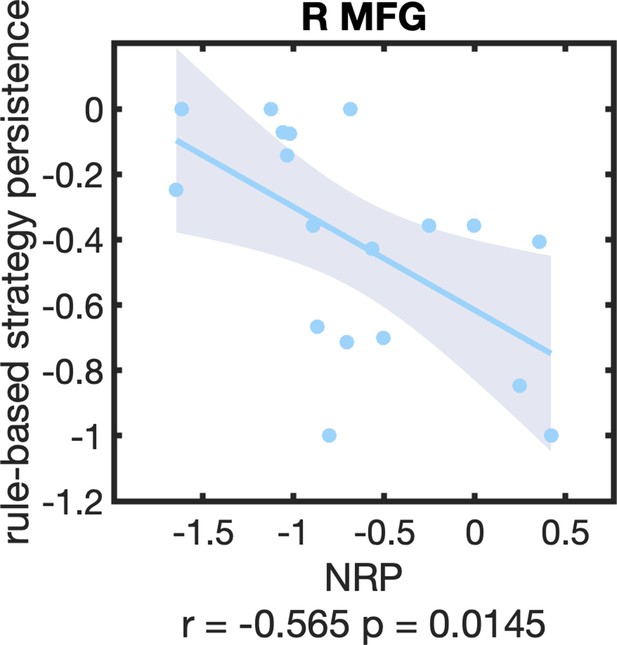
Correlation between neural representational plasticity (NRP) and rule-based strategy persistence in children with autism spectrum disorder (ASD).
Pearson’s correlation between rule-based strategy persistence and NRP was assessed for each region of interest in children with ASD. Rule-based strategy persistence was quantified as the extent to which children with ASD continued to rely on rule-based strategy across pre- and post-training sessions, by subtracting the proportion of rule-based strategy use across all trained problems at pre-training from that at post-training. Values ranged from –1 to 0, with –1 indicating a shift from rule-based to memory-based problem-solving strategy for all of the trained problems and 0 indicating no change in strategy use for any of the trained problems following training. Larger values (i.e. those closer to 0) represented persistence using a rule-based strategy across sessions. Regions of interest included brain regions that showed significant group differences in NRP-learning gain relation, which included the bilateral medial temporal lobe, right intraparietal sulcus, right lateral occipital cortex, right frontal eye field, and right middle frontal gyrus (MFG; see also Figure 4 and Supplementary file 6). We found a significant negative correlation between rule-based strategy persistence and NRP in the right MFG, which indicates that children with ASD who persisted more on using rule-based strategy across trained problems were those who showed more reduced NRP across pre- and post-training sessions (see also Discussion). No other ROI showed a significant correlation in children with ASD (ps >0.183). Sample size: n(ASD)=18.
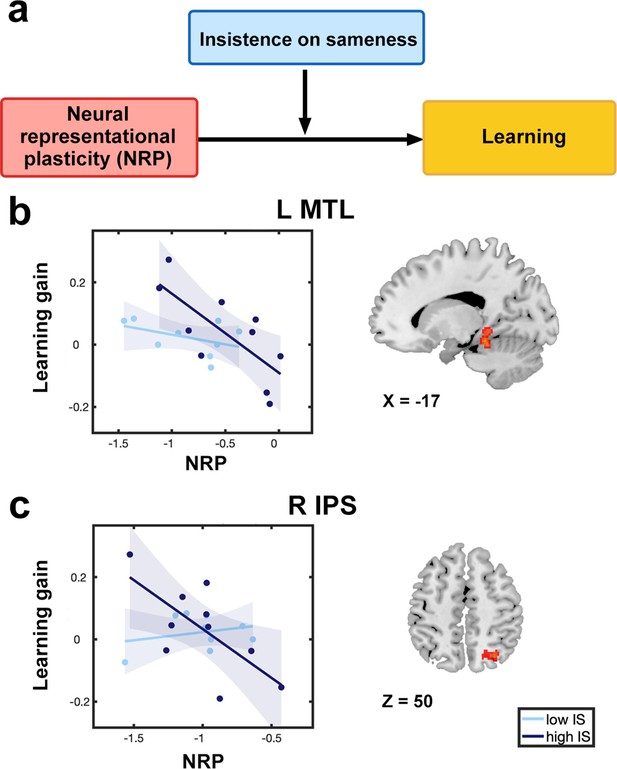
Insistence on sameness moderates the relation between training-induced brain plasticity and learning in children with autism.
(a) A moderation analysis was performed to examine whether insistence on sameness (IS), a cognitive component of clinical symptoms (RRIB) in autism, influences the relation between functional brain plasticity and learning. (b–c). IS moderates the relation between neural representational plasticity (NRP) and learning gains in (b) the left medial temporal lobe (MTL) and (c) right intraparietal sulcus (IPS). Higher levels of IS were linked to a more atypical association between NRP and learning gains in children with autism spectrum disorder (ASD). The sample of ASD participants was median-split into high and low IS groups based on the scores on IS to illustrate atypical brain-behavior association moderated by the severity of IS symptoms. Shaded areas indicate 95% confidence intervals. Sample size: n(ASD)=19.
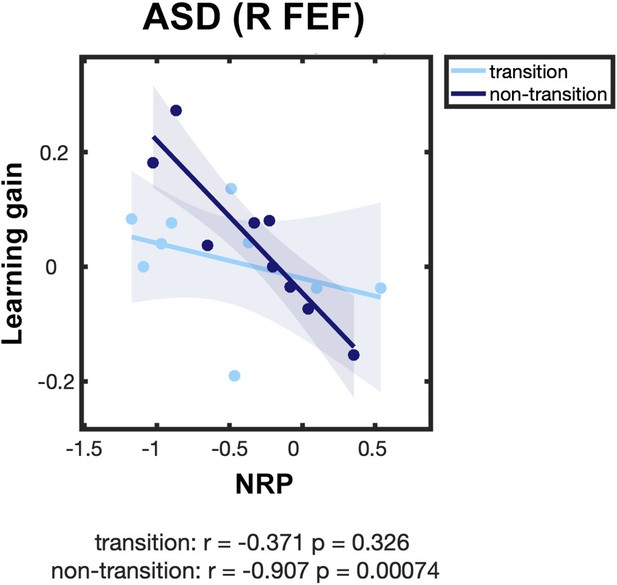
Strategy transition moderates atypical relation between training-induced brain plasticity and learning in children with autism spectrum disorder (ASD).
A moderation analysis was performed for each region of interest to examine whether the transition from a rule-based to a memory-based strategy influences the relationship between functional brain plasticity and learning. Each child was assigned a status of either ‘transition’ or ‘non-transition’ based on whether or not their dominant strategy changed from rule-based to memory-based strategy between pre- and post-training sessions. Regions of interest included brain regions that showed significant group differences in NRP-learning gain relation, which included the bilateral medial temporal lobe, right intraparietal sulcus, right lateral occipital cortex, right frontal eye field (FEF), and right middle frontal gyrus (see also Figure 4 and Supplementary file 6). We found that strategy transition moderates the relation between neural representational plasticity (NRP) and learning gains in the right FEF in children with ASD (b=–0.48, se = 0.19, t=–2.58, p=0.022, model p=0.005), which indicates that atypical association between lower NRP and greater learning gain was driven by non-transition individuals with ASD, who showed a strong persistence of the rule-based strategy (see also Discussion). No other ROI showed a significant moderation effect in children with ASD (ps >0.084). Sample size: n(ASD)=18.
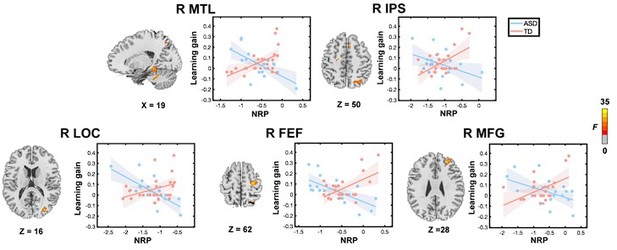
The Results of the brain-behavior relation after mean frame-wise displacement regression.
Whole-brain multivariate neural representational pattern analysis revealed a significant group by learning gain interaction in the right medial temporal lobe (MTL), right intraparietal sulcus (IPS), right lateral occipital cortex (LOC), right frontal eye field (FEF), and right middle frontal gyrus (MFG). NRP in each region identified from the interaction between group and learning gains in whole-brain analysis was extracted from 6-mm spheres centered at peaks for visualization of results. Shaded areas indicate 95% confidence intervals.
Additional files
-
Supplementary file 1
Demographic and clinical measures.
- https://cdn.elifesciences.org/articles/86035/elife-86035-supp1-v2.docx
-
Supplementary file 2
Number of participants included in each analysis.
- https://cdn.elifesciences.org/articles/86035/elife-86035-supp2-v2.docx
-
Supplementary file 3
Results of repeated measures ANOVA for behavioral performance.
- https://cdn.elifesciences.org/articles/86035/elife-86035-supp3-v2.docx
-
Supplementary file 4
Results of t-tests for behavioral performance.
- https://cdn.elifesciences.org/articles/86035/elife-86035-supp4-v2.docx
-
Supplementary file 5
Results of chi-squared tests for dominant strategy use.
- https://cdn.elifesciences.org/articles/86035/elife-86035-supp5-v2.docx
-
Supplementary file 6
Brain regions showing significant group by behavior interaction on neural representational plasticity between pre- and post-training for trained problems.
- https://cdn.elifesciences.org/articles/86035/elife-86035-supp6-v2.docx
-
Supplementary file 7
Results of brain-behavior association between region of interest (ROI)-based neural representational plasticity and learning gains.
- https://cdn.elifesciences.org/articles/86035/elife-86035-supp7-v2.docx
-
Supplementary file 8
Moderation results for RRIB sub-scores on the association between brain and behavioral measures.
- https://cdn.elifesciences.org/articles/86035/elife-86035-supp8-v2.docx
-
MDAR checklist
- https://cdn.elifesciences.org/articles/86035/elife-86035-mdarchecklist1-v2.pdf