Criticality supports cross-frequency cortical-thalamic information transfer during conscious states
Figures
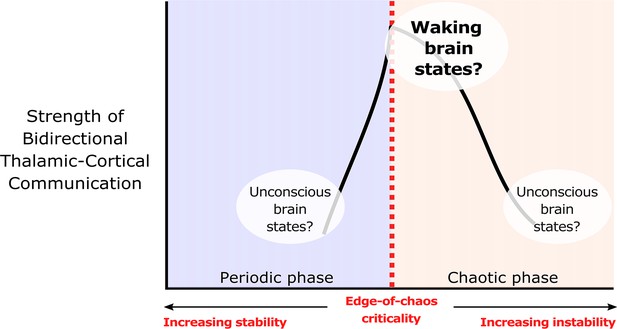
We hypothesize that edge-of-chaos criticality supports thalamic-cortical communication during waking brain states.
We hypothesize that the strength of bidirectional information transfer between the cortex and thalamus should be highest during waking brain states, owing to the proximity of slow neural electrodynamics to edge-of-chaos criticality during these states. We also predict that as slow neural electrodynamics transition away from this critical point during unconscious states, either into the chaotic phase or into the periodic phase, the strength of cortical-thalamic information transfer should be diminished.
© 2022, Toker et al. Figure 1 is adapted from Figure 1 from Toker et al., 2022, Proceedings of the National Academy of Sciences. The image is published under the terms of the Creative Commons Attribution-NonCommercial-NoDerivatives License 4.0 (CC BY-NC-ND) (https://creativecommons.org/licenses/by-nc-nd/4.0/)
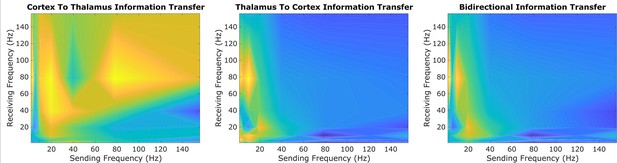
An exploratory sweep suggests that thalamus and cortex transmit information bidirectionally from low-to-high frequencies during conscious states.
In our initial exploratory sweep of spectral patterns of directed cortical-thalamic information transfer during conscious states, based on half of all patients’/animals’ trials, we identified a prominent motif of low-to-high frequency bidirectional communication that was present during waking states in nearly all subjects and species. We first estimated the (z-scored) strengths of information transfer across every possible pair of frequency bands, for every 10 s trial, and for every subject during waking states. We then took the average cross-trial result for every subject. Here, we plotted the mode across subjects’ cross-trial averages in order to reveal the spectral patterns of information transfer that occurred most frequently across subjects during conscious states. For cortico-thalamic information transfer (left), we found that information sent from the cortex across all frequencies is frequently received by the thalamus in the high range. For thalamo-cortical information transfer (middle), we observed a prominent pattern of low-to-high frequency information transfer. When looking at the mode across all cross-trial averages of both cortico-thalamic and thalamo-cortical information transfer during conscious states (right), there seems to be a consistent channel of communication from the low-frequency range (∼1–13Hz) to the high-frequency range (52–104 Hz) in both directions (cortico-thalamic and thalamo-cortical). We therefore chose to study this cross-frequency pattern of information transfer in our subsequent analyses of waking, GABAergic anesthesia, generalized spike-and-wave seizure, and psychedelic states.
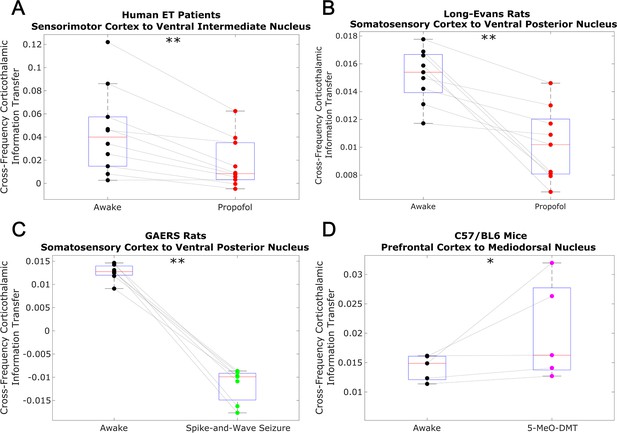
Low-to-high frequency information transfer from cortex to thalamus is diminished during unconsciousness and enhanced during psychedelic states.
Using a spectrally resolved measure of directed information transfer (see Materials and methods), we found that the strength of information transferred from cortical // waves (∼1–13 Hz) to thalamic high waves (52–104 Hz) is significantly reduced during unconsciousness induced by propofol anesthesia (A–B) and generalized spike-and-wave seizures (C). Conversely, the strength of this low-to-high frequency corticothalamic information transfer is significantly increased during psychedelic states induced by 5-MeO-DMT (D). *p<0.05, **p<0.01, significance assessed using a one-tailed Wilcoxon signed-rank test.
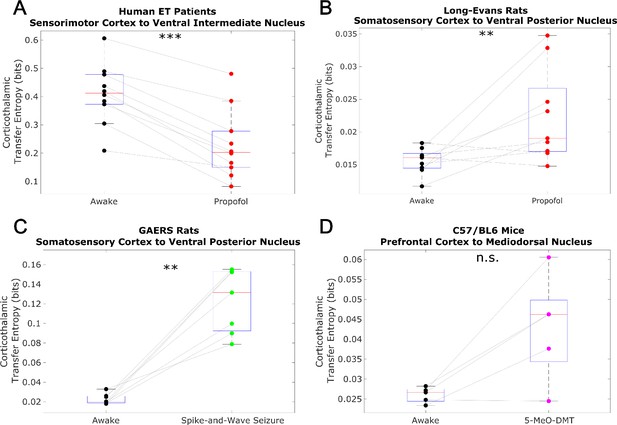
Non-spectrally resolved transfer entropy from cortex to thalamus does not track consciousness.
We here plot changes to (non-spectrally resolved) transfer entropy from cortex to thalamus across brain states (see Materials and methods). We found no consistent relationship between corticothalamic transfer entropy and consciousness. *p<0.05, **p<0.01, ***p<0.001, significance assessed using a one-tailed Wilcoxon signed-rank test.
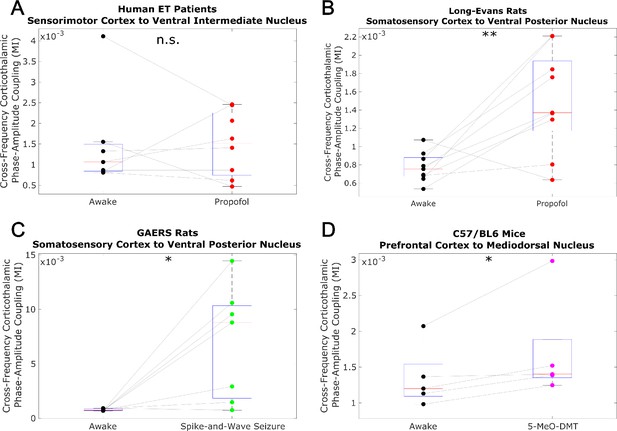
Low-to-high-frequency phase-amplitude coupling from cortex to thalamus does not track consciousness.
We evaluated cross-frequency phase-amplitude coupling from cortexto thalamus using the modulation index (MI). Specifically, we evaluated coupling between the phase of the low-frequency (1-13 Hz) activity and the amplitude of high-frequency (52-104 Hz) activity (matching the frequency ranges analyzed in the main body of our paper). Note that the MI is a bivariate measure, meaning that it is calculated between pairs of univariate channels. As such, for our human essential tremor patient data, which consisted of multiple cortical and thalamic channels, we calculated the MI from all cortical channels to all thalamic channels, and set the corticothalamic MI as the median across all resulting values. As was the case with transfer entropy, we found noconsistent relationship between cross-frequency corticothalamic phase-amplitude coupling (across the frequencies studied in this paper) and consciousness. *p<0.05, **p<0.01, ***p<0.001, significance assessed using a one-tailed Wilcoxon signed-rank test.
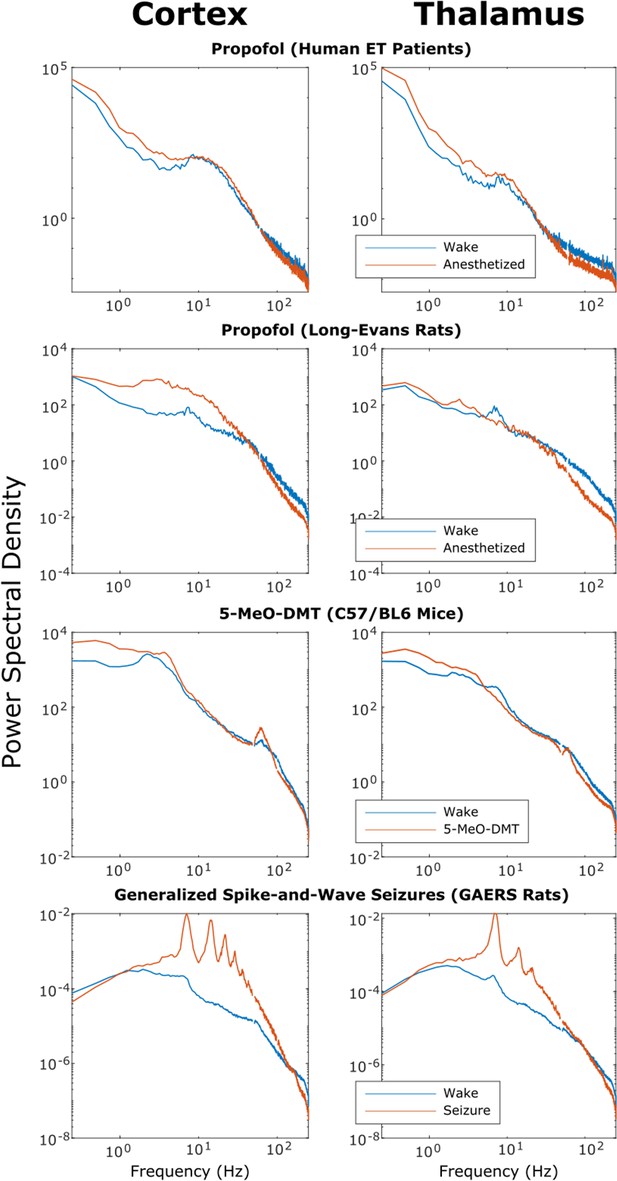
Power spectra of thalamic and cortical electrodynamics during waking, anesthesia, psychedelic, and seizure states.
We here plot the cross-subject median power spectral densities (estimated using Welch’s method) for all brain states. Note that both propofol and 5-MeO-DMT increased spectral power in the slow/delta range (≤4 Hz) and decreased spectral power above 80 Hz in both cortex and thalamus, despite opposing effects on cross-frequency corticothalamic information transfer (Figure 3).
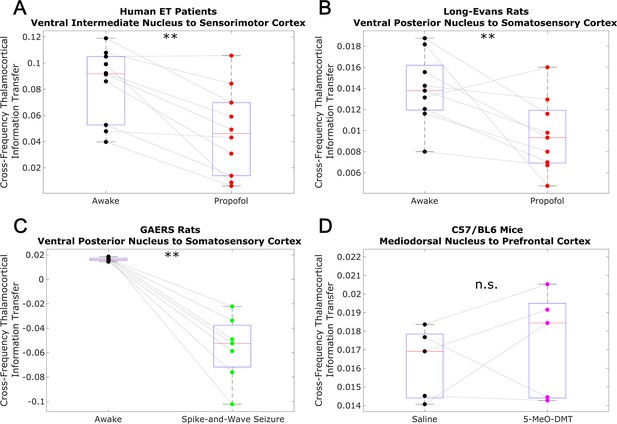
Low-to-high frequency information transfer from thalamus to cortex is diminished during unconsciousness.
Similar to the results we observed for communication from the cortex to the thalamus (Figure 3), we found that strength of information transferred from thalamic // waves (∼1–13 Hz) to cortical high waves (52–104 Hz) is significantly reduced during unconsciousness induced by propofol anesthesia (A–B) and generalized spike-and-wave seizures (C). Unlike corticothalamic information transfer (Figure 3), however, the strength of this low-to-high frequency information transfer from the thalamus to cortex does not change significantly during psychedelic states induced by 5-MeO-DMT (D). *p<0.05, **p<0.01, significance assessed using a one-tailed Wilcoxon signed-rank test.
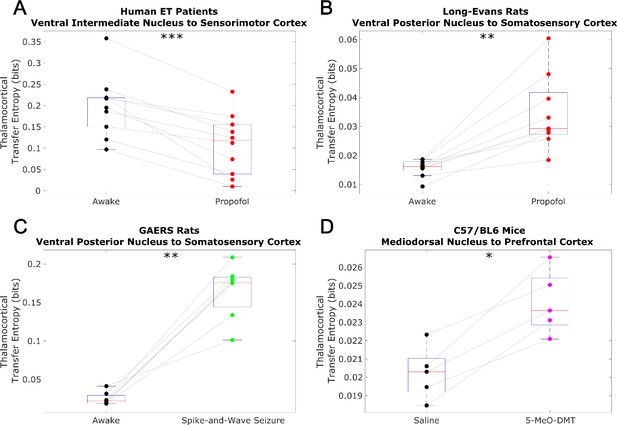
Non-spectrally resolved transfer entropy from thalamus to cortex does not track consciousness.
We here plot changes to (non-spectrally resolved) transfer entropy from thalamus to cortex across brain states. We again found no consistent relationship between thalamocortical transfer entropy and consciousness. *p<0.05, **p<0.01, ***p<0.001, significance assessed using a one-tailed Wilcoxon signed-rank test.
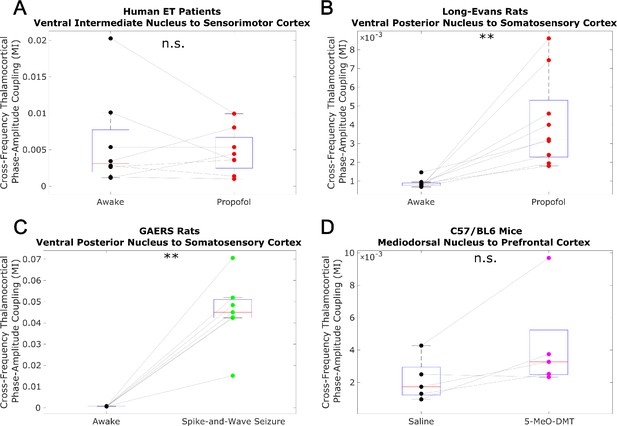
Low-to-high-frequency phase-amplitude coupling from thalamus to cortex does not track consciousness.
We analyzed cross-frequency phase-amplitude coupling from thalamus to cortex using the same methods described in Figure 3—figure supplement 2, and again observed no consistent relationship between cross-frequency thalamocortical phase-amplitude coupling and consciousness. *p<0.05, **p<0.01, ***p<0.001, significance assessed using a one-tailed Wilcoxon signed-rank test.
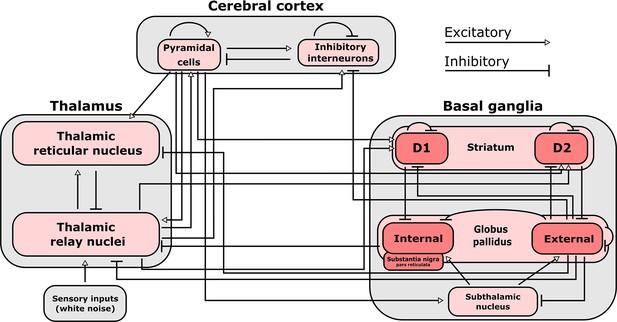
Connections included in our mean-field model of the macro-scale electrodynamics of the basal ganglia-thalamo-cortical system.
We here plot the structural connectivity in out mean-field model. Note that the internal globus pallidus and the substantia nigra pars reticulata, which are both inhibitory output nuclei of the basal ganglia, are treated as a single structure. See Table 2 for the mean firing rates for each neural population in the model, alongside known region-specific firing rates in multiple mammalian species. See Supplementary file 3 for parameters describing the properties of each neural population, as well as parameters describing the propagation of electric fields along each anatomical connection.
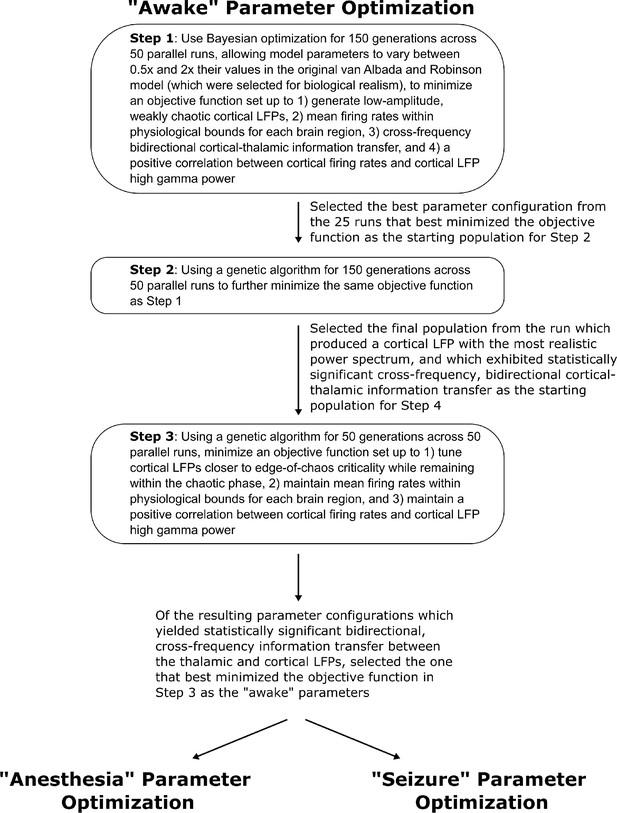
Method for optimizing parameters of the waking simulation.
We here depict the workflow for the use of Bayesian-genetic optimization to derive model parameters for the ‘awake’ state of the mean-field model of the electrodynamics of the basal ganglia-thalamo-cortical system.
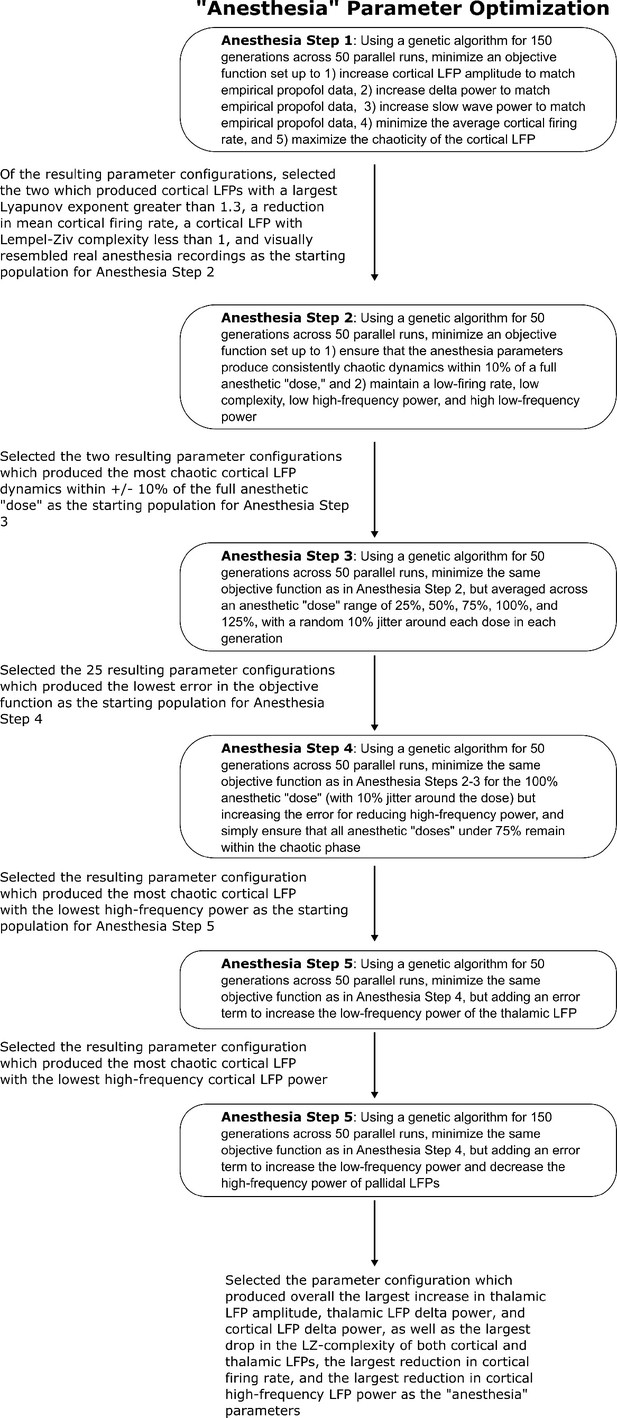
Method for optimizing parameters of the anesthesia simulation.
We here depict the workflow for the use of genetic optimization to derive model parameters for the anesthesia state of the mean-field model, starting from the parameters for the wake state of the mean-field model.
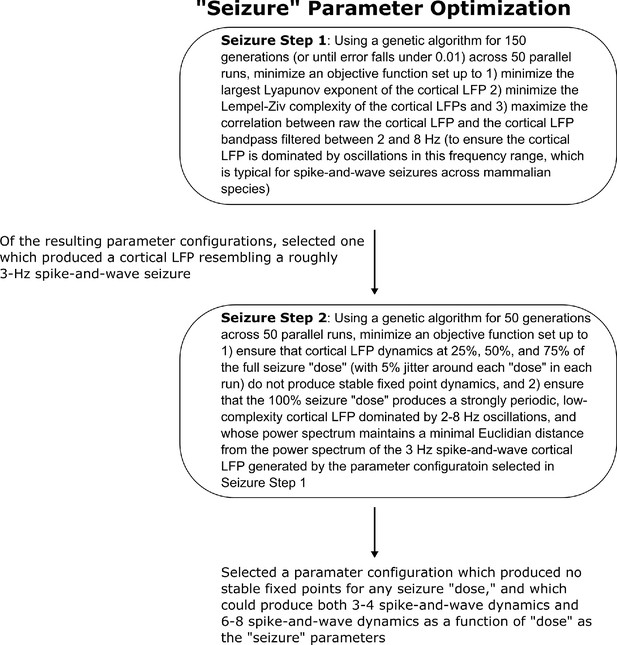
Method for optimizing parameters of the seizure simulation.
We here show the workflow for the use of genetic optimization to derive model parameters for the generalized spike-and-wave seizure state of the mean-field model, starting from the parameters for the wake state of the model.
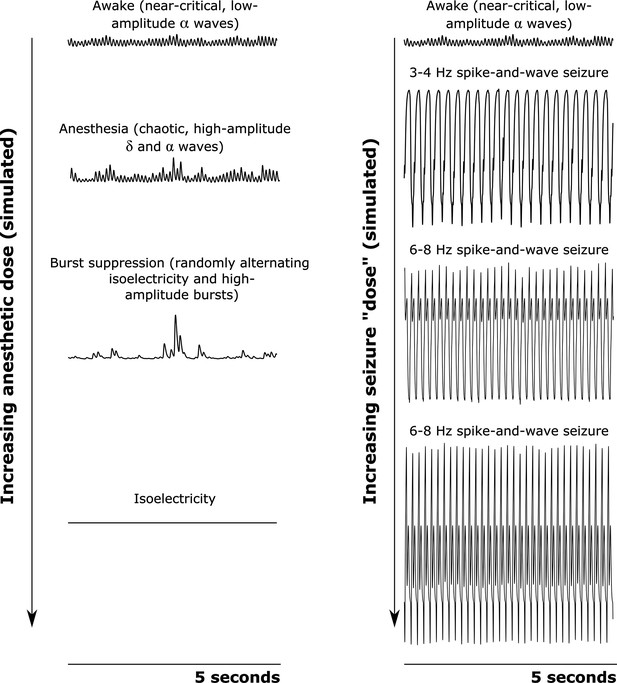
Simulated cortical local field potentials as a function of increasing anesthetic or seizure ’dose‘.
We here plot example time-traces of our simulated cortical local field potentials (LFPs). Note that all data plotted here are on the same scale. For our awake simulation (top), the mean-field model generates near-critical, weakly chaotic, low-amplitude oscillations dominated by waves (8–13 Hz), with significant bidirectional cross-frequency information transfer between the cortex and thalamus (as observed in our empirical data). With increasing anesthetic dose (left), the simulated cortical LFP transitions to chaotic, high-amplitude waves (1–4 Hz) and waves. At a higher dose, the simulated cortical LFP transitions to burst suppression-like dynamics, which are characterized by stochastic switching between isoelectricity and high-amplitude bursts. Finally, at the highest anesthetic doses, the simulated cortical LFP transitions to isoelectricity. This simulated anesthetic dose-response trajectory closely mirrors well-established empirical dose-response trajectories. For our seizure simulation (right), increasing ‘doses’ first push the cortical LFP into a 3–4 Hz spike-and-wave seizure (which is characteristic of human epilepsy patients), followed by a 6–8 Hz spike-and-wave seizure (which is characteristic of rodent models of epilepsy, including the GAERS rats studied here).
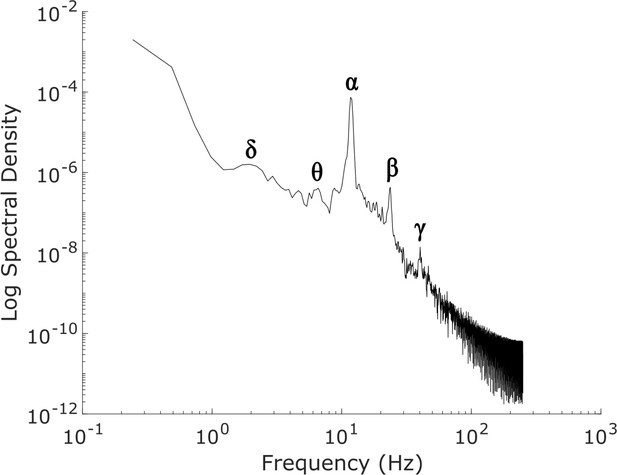
Power spectrum of the simulated, waking-state cortical local field potential.
The power spectrum of our simulated ’awake’ cortical local field potential (LFP), which was generated by optimizing the parameters of a mean-field model of the basal ganglia-thalamo-cortical system using machine learning (see Materials and methods). Our simulated cortical LFP produces spectral peaks at frequencies precisely corresponding to canonical cortical electrodynamic oscillations, including δ waves (1-4 Hz), θ waves (4-8 Hz), α waves (8-13 Hz), β waves (15-30 Hz), and low-γ waves (35-60 Hz).
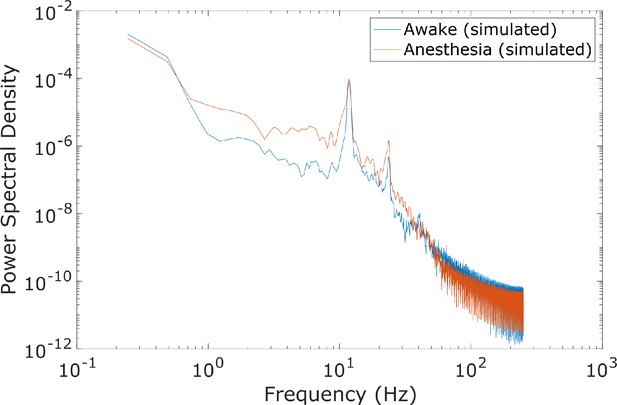
Spectral changes in the anesthesia simulation.
Compared to the power spectrum of our simulated ‘awake’ cortical local field potential (LFP), the power spectrum of our simulated anesthesia LFP exhibited increased low-frequency power and decreased high-frequency power. Here, the anesthesia simulation corresponds to the 100% ’dose‘, which is the set of parameters arrived at through our genetic optimization.
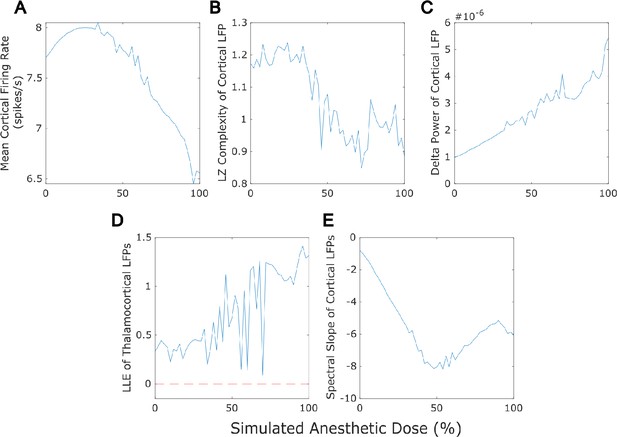
Dose-response effects of simulated anesthesia.
Our mean-field model successfully recapitulated several previously established features of anesthesia, including a reduction in cortical firing rate (A), a loss of the information-richness of cortical local field potential (LFPs) as indexed by Lempel-Ziv complexity (B), a rise in the spectral power of delta (1-4 Hz) oscillations in cortical LFPs (C), strongly chaotic neural electrodynamics (D - note that the dashed red line at largest Lyapunov exponent = 0 corresponds to edge-of-chaos criticality), and a steepening spectral slope of cortical electrodynamics (here measured by fitting a line to the logspectral density of the simulated cortical LFP between 30 and 45 Hz) (E). Note that we here plot only up to 100% anesthesia ’dose‘, which is the set of parameters arrived at through our genetic optimization. At higher ’doses’ (see Materials and methods), dynamics switch to stochastic burst suppression followed by isoelectricity with a complete cessation of firing (see Figure 5 for example LFP tracesfrom these higher-dose states).
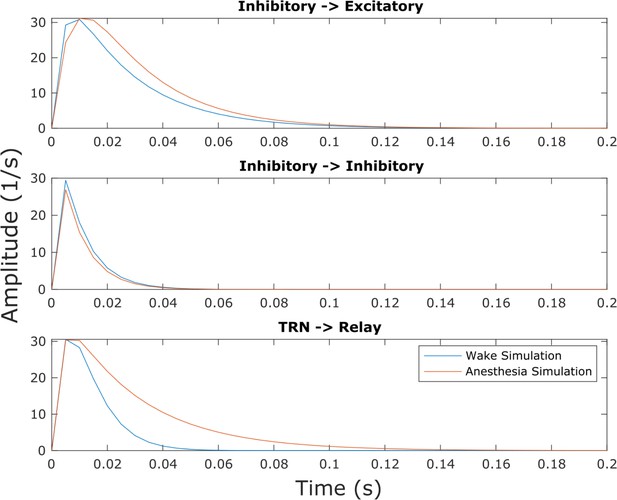
Inhibitory postsynaptic potentials in the anesthesia simulation.
Though this effect was not explicitly selected for in our parameter optimization, we found that our simulated anesthesia state resulted in prolonged inhibitory postsynaptic potentials at excitatory cells in both the cortex and thalamic relay nucleus relative to the waking state of the model, owing to changes in synaptodendritic rise and decay rates (Supplementary file 3).
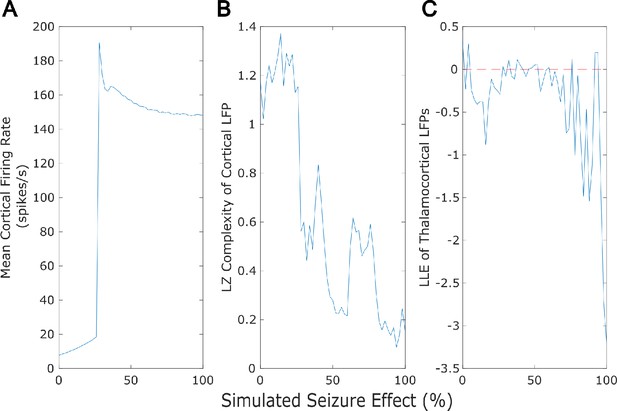
Dose-response effects of simulated seizures.
Our mean-field model successfully recapitulated several previously established features of generalized seizures, including a large rise in cortical firing rate (A), a loss of the information-richness of cortical local field potentials as indexed by Lempel-Ziv complexity (B), and strongly periodic neural electrodynamics (C - note that the dashed red line at largest Lyapunov exponent = 0 corresponds to edge-of-chaos criticality).
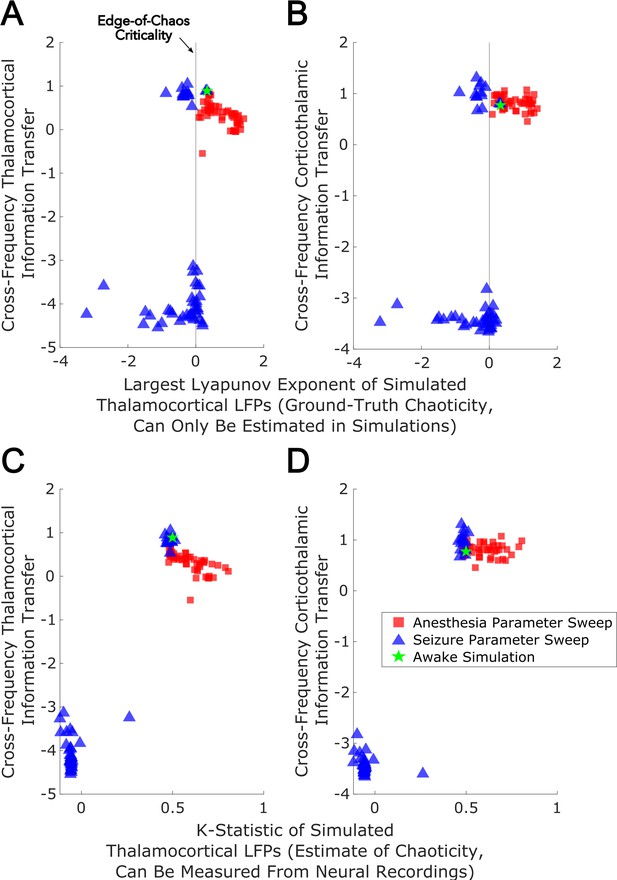
Edge-of-chaos criticality supports cross-frequency thalamic-cortical information transfer in a mean-field model.
We performed parameter sweeps for different ‘doses’ of simulated anesthetic (red square) and seizure (blue triangle) effects. For each ‘dose’, we calculated the median estimated largest Lyapunov exponent (LLE) of simulated thalamocortical LFPs across 10 runs, and plotted the median strength of cross-frequency thalamocortical (A) and corticothalamic (B) information transfer as a function of those median LLEs. We found a clear peak in the strength of bidirectional cross-frequency cortical-thalamic information transfer when our simulated thalamocortical electrodynamics were poised near edge-of-chaos criticality (the vertical lines at LLE = 0). We further found that the strength of this bidirectional, cross-frequency information transfer decayed in both the periodic phase (negative LLEs) with increasing seizure effect and the chaotic phase (positive LLEs) with increasing anesthetic effect. However, because this decay was exponentially faster in the periodic phase, we here plotted the bi-symmetric log-transform (Webber, 2013) of our results for the sake of visualization. Because LLEs can only be estimated with some accuracy in simulations, we also calculated the estimated the median chaoticity of the low-frequency (1–13 Hz) component of our simulated cortical and thalamic LFPs using the K-statistic of the modified 0–1 chaos (which can be measured from real neural recordings). We plotted those results against the (bi-symmetric log-transformed) median strength of cross-frequency thalamocortical (C) and corticothalamic (D) information transfer, and observed the same overall relationship between chaoticity and bidirectional cross-frequency information transfer, suggesting that this relationship can be evaluated in real neural recordings.
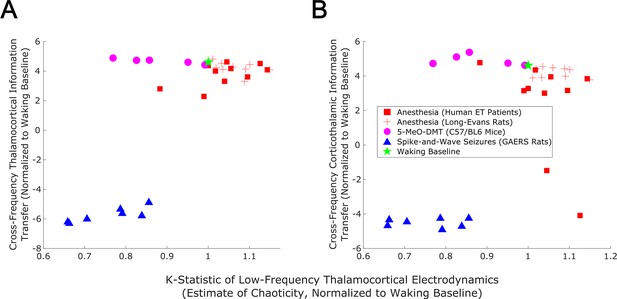
Empirical evidence that edge-of-chaos criticality supports cross-frequency thalamic-cortical information transfer during conscious states.
We here plot the median strength of cross-frequency thalamocortical (A) and corticothalamic (B) information transfer across brain states (normalized to each patient’s or animal’s waking baseline, and bi-symmetrically log-transformed) as a function of the median estimated chaoticity of the low-frequency (1–13 Hz) component of thalamic and cortical electric field recordings (also normalized to waking baselines). We found the same trend as in our mean-field model (Figure 8), with bidirectional cross-frequency information transfer exhibiting the most pronounced decay as thalamocortical electrodynamics hyper-stabilize in the generalized spike-and-wave seizure state. The strength of bidirectional cross-frequency information transfer also decays, though not as quickly, as thalamocortical electrodynamics become increasingly chaotic in the GABAergic anesthesia state. Conversely, the strength of cross-frequency information transfer from the cortex to the thalamus, but not from the thalamus to the cortex, increases as thalamocortical electrodynamics moderately stabilize in the 5-MeO-DMT psychedelic state, presumably reflecting a transition closer to edge-of-chaos criticality relative to normal waking states, which are near-critical but weakly chaotic.
Tables
Statistical analysis confirms that thalamus and cortex transmit information bidirectionally from low-to-high frequencies during waking states.
Following our initial exploratory sweep of all possible spectral patterns of cortical-thalamic communication (Figure 2), which was based on one half of the 10 s trials for each patient/animal, we used surrogate testing on the remaining half of trials to evaluate whether there was statistically significant information transfer from slow (∼1–13 Hz) to fast (52–104 Hz) electrodynamics between anatomically connected sub-regions of the thalamus and cortex (see Materials and methods). For each 10 s window of activity, surrogate testing produced a single p-value reflecting the significance of cross-frequency information transfer in each direction (cortico-thalamic and thalamo-cortical). Overall statistical significance, across 10 s windows within each subject, was assessed by evaluating the harmonic mean (Wilson, 2019) of all single-trial p-values. The number of 10 s trials used in this analysis for each patient/animal are listed here in the right-hand column. In line with our initial exploratory sweep (Figure 2), we found that there was significant low-to-high frequency bidirectional information transfer between the thalamus and cortex in nearly every species, strain, and subject. Note that we did not correct for multiple comparisons because each subject’s harmonic mean p-value contributes to a single overarching hypothesis about inter-region neural communication, rather than representing separate, independent hypotheses.
Cortex to Thalamus | Thalamus to Cortex | Number of Trials | |
---|---|---|---|
Human ET Patient 1 | 3 | ||
Human ET Patient 2 | 3 | ||
Human ET Patient 3 | 3 | ||
Human ET Patient 4 | 2 | ||
Human ET Patient 5 | 3 | ||
Human ET Patient 6 | 2 | ||
Human ET Patient 7 | 3 | ||
Human ET Patient 8 | 1 | ||
Human ET Patient 9 | 3 | ||
Human ET Patient 10 | 3 | ||
Long-Evans Rat 1 | 6 | ||
Long-Evans Rat 2 | 6 | ||
Long-Evans Rat 3 | 7 | ||
Long-Evans Rat 4 | 7 | ||
Long-Evans Rat 5 | 6 | ||
Long-Evans Rat 6 | 6 | ||
Long-Evans Rat 7 | 5 | ||
Long-Evans Rat 8 | 1 | ||
Long-Evans Rat 9 | 3 | ||
GAERS Rat 1 | 71 | ||
GAERS Rat 2 | 31 | ||
GAERS Rat 3 | 159 | ||
GAERS Rat 4 | 148 | ||
GAERS Rat 5 | 80 | ||
GAERS Rat 6 | 55 | ||
GAERS Rat 7 | 79 | ||
C57BL/6 Mouse 1 | 104 | ||
C57BL/6 Mouse 2 | 78 | ||
C57BL/6 Mouse 3 | 76 | ||
C57BL/6 Mouse 4 | 76 | ||
C57BL/6 Mouse 5 | 81 |
Mean firing rates of each brain region in the waking state of the mean-field model (in spikes/s), compared to empirical ranges of firing rates from multiple mammalian species.
Note that the GPi and SNr were treated as a single population, and thus have the same firing rate. Mean firing rates for each simulated brain region were within or near known physiological ranges, and were tuned to be as such using Bayesian-genetic optimization. GPi = internal globus pallidus, SNr = substantia nigra pars reticulata, GPe = external globus pallidus, STN = subthalamic nucleus, TRN = thalamic reticular nucleus.
Model | Monkeys | Rats | Mice | Humans | Cats | |
---|---|---|---|---|---|---|
Cortex | 7.7 | 5-20* | 2-5† | 2-11‡ | 1-10§ | |
Striatum | 5.19 | 4-7¶ | 1-7** | |||
GPi | 75.72 | 60-90†† | 15-20‡ ‡ | |||
SNr | 75.72 | 50-70§ § | ||||
GPe | 30.85 | 16-70¶ ¶ | 16-115*** | 1-64††† | ||
STN | 13.62 | 20-30‡ ‡ ‡ | 8-11§ § § | |||
Relay nuclei | 16.39 | 5-25¶ ¶ ¶ | 10-20**** | |||
TRN | 15.41 | 4-64†††† | 20-30‡ ‡ ‡ ‡ |
-
*
-
†
-
‡
-
§
-
¶
-
**
-
††
-
‡ ‡
-
§ §
-
¶ ¶
-
***
-
†††
-
‡ ‡ ‡
-
§ § §
-
¶ ¶ ¶
-
****
-
††††
-
‡ ‡ ‡ ‡
Additional files
-
Supplementary file 1
Extended analysis of cross-frequency thalamic-cortical information transfer during sample waking-state trials, using a larger number (250) of surrogates.
- https://cdn.elifesciences.org/articles/86547/elife-86547-supp1-v2.pdf
-
Supplementary file 2
ANCOVA results showing that brain state, but not spectral power, significantly explains the observed variances in cross-frequency thalamic-cortical information transfer.
- https://cdn.elifesciences.org/articles/86547/elife-86547-supp2-v2.pdf
-
Supplementary file 3
Parameters for the three states (waking, anesthesia, and spike-and-wave seizure) of the mean-field model.
- https://cdn.elifesciences.org/articles/86547/elife-86547-supp3-v2.pdf
-
Supplementary file 4
ANCOVA results showing that brain state, but not spectral power, significantly explains the observed variances in the chaotictiy of low-frequency thalamocortical electrodynamics.
- https://cdn.elifesciences.org/articles/86547/elife-86547-supp4-v2.pdf
-
Supplementary file 5
Analysis of the stochasticity of both low-frequency and broadband thalamocortical electrodynamics, suggesting predominantly deterministic behavior.
- https://cdn.elifesciences.org/articles/86547/elife-86547-supp5-v2.pdf
-
MDAR checklist
- https://cdn.elifesciences.org/articles/86547/elife-86547-mdarchecklist1-v2.pdf