A reductionist paradigm for high-throughput behavioural fingerprinting in Drosophila melanogaster
Figures
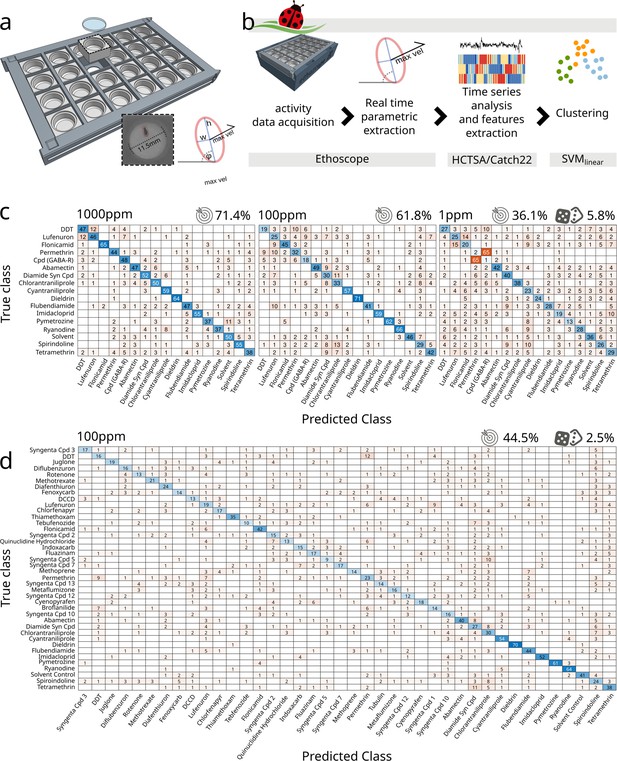
Coccinella successfully classifies pharmacobehaviours.
(a) Bespoke 3D-printed arena can house 24 individual flies in a two-dimensional circular space. Each arena measures 11.5 mm in diameter and allows for back illumination either by visible or infrared light sources embedded in the ethoscope base. The red ellipse shows the data being extracted by the ethoscope in real time. w, h: width and height of the ellipse inscribing the animal. φ: the angle of the ellipse in reference to the region of interest. max. vel.: maximal velocity over the last 10 s. (b) Flowchart describing the analytical pipeline and the tools that compose coccinella. (c) Confusion matrices for treatments with 16 compounds and a solvent control, with drugs used at concentrations of 1000 ppm (left), 100 ppm (centre), and 1 ppm (right). The target icon indicates calculated accuracy while the rolling die indicate the accuracy of a random classifier. (d) Confusion matrix for the largest panel of 40 treatments at 100 ppm.
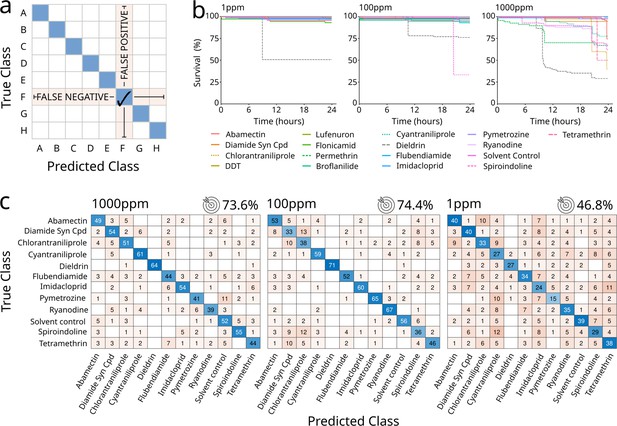
Successful classification of a smaller group of 11 compounds.
(a) Explanatory drawing of how confusion matrices present the data. The blue diagonal boxes indicate the number of flies correctly classified (true positives). The vertical cells indicate the number of flies wrongly classified (false positives). The horizontal cells indicate the number of flies that should have been classified (false negatives). (b) Survival curves upon treatment with a panel of 12 drugs administered at different concentrations, indicated above each figure (1, 100, and 1000 ppm). (c) Confusion matrices for treatments with 11 compounds and a solvent control, with drugs used at concentrations of 1000 ppm (left), 100 ppm (middle), and 1 ppm (right). The target icon indicates calculated accuracy. Accuracy of a random classifier would be 8.3%.
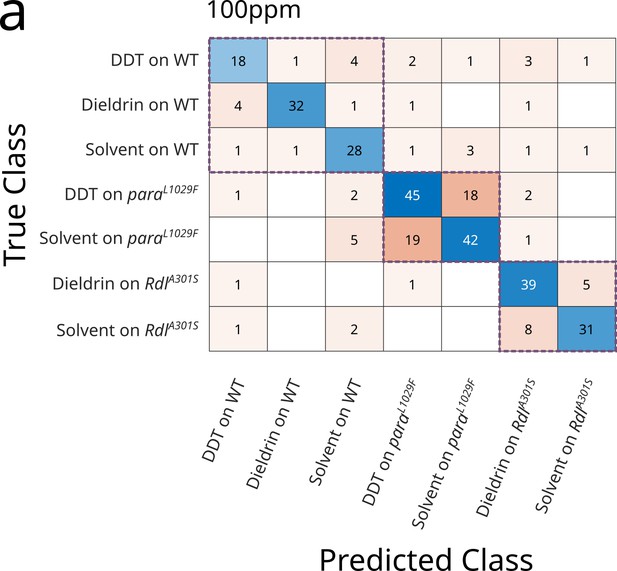
Effect of drug resistance conferring mutations on Coccinella's performance.
(a) Confusion matrix classifying the action of two selected drugs (Dichlorodiphenyltrichloroethane (DDT) 100 ppm and dieldrin 100 ppm) or solvent on wild-type flies (WT) or mutants with known resistance to those drugs. The LD100 of dieldrin is 0.1–1 ppm and the LD100 of DDT is 10–100 ppm. The three purple dotted boxes highlight the three experimental clusters (wild-type flies, para mutants, Rdl mutants).
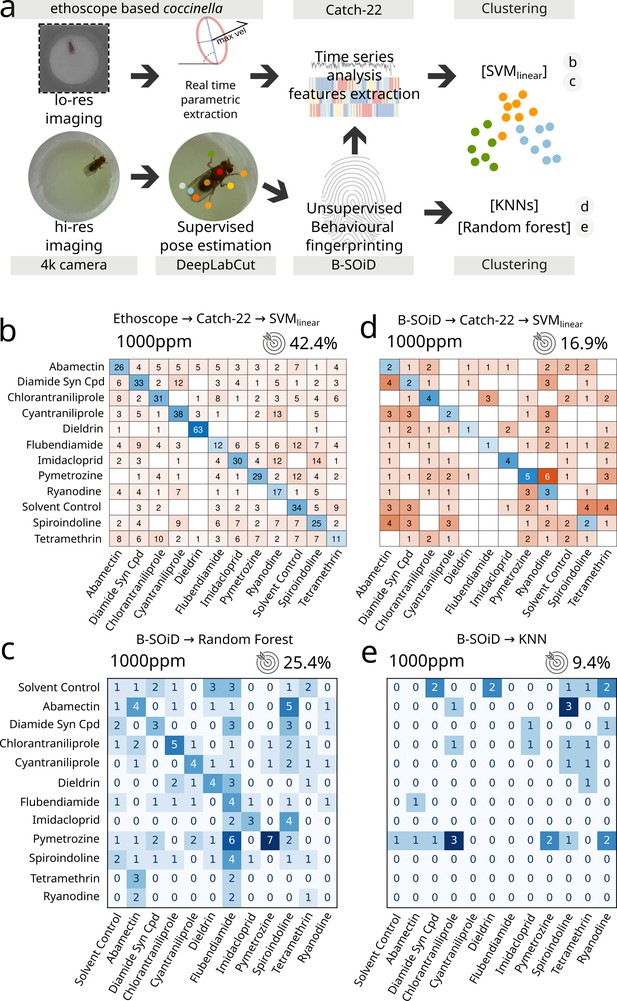
Comparison between coccinella and the state-of-the-art.
(a) Experimental pipeline illustrating the four experimental analyses. (b) Confusion matrix for 12 treatments (11 drugs at 1000 ppm and 1 solvent control) analysed using coccinella. (c) Same experimental treatments as in b, analysed using the DeepLabCut → B-SoID → random forest pipeline starting from high-resolution images. The random forest classifier was trained on a 4:1 training:testing ratio. (d) Same as c but with Catch22 identification and support vector machine (SVM) clustering after B-SoID grammar dissection. This is a hybrid treatment combining highly comparative time-series analysis (HCTSA) feature extraction to the high-resolution pipeline. (e) Same as c but using K-nearest neighbours (KNN) as cluster algorithm. KNN required a much higher training:testing ratio of 9:1, dramatically reducing the size of the testing dataset. The accuracy of a random classifier for all matrices on this figure would be 8.3% (not shown on figures for lack of space).
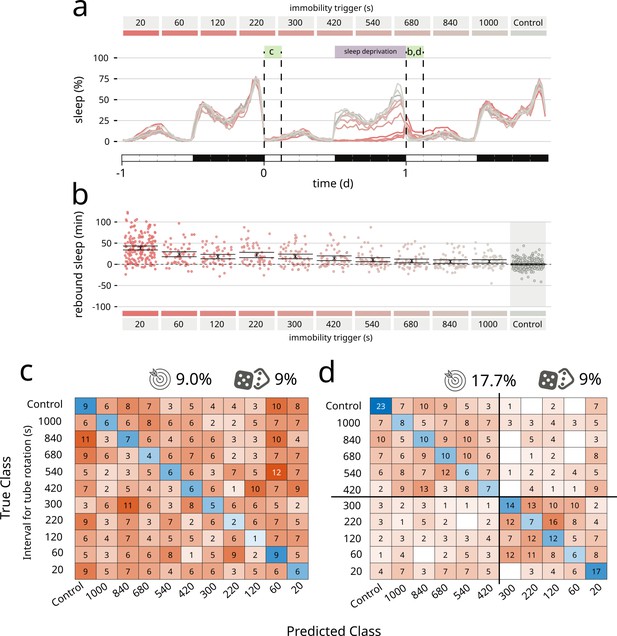
Coccinella finds differences in type of sleep rebound after sleep deprivation.
(a) Sleep profile of flies over the period of 3 days. A 12-hr sleep deprivation regime starts at the beginning of the dark phase of day 0 (purple bar). The 3-hr windows labelled with green boxes were analysed by coccinella in search of meaningful differences. The letters above refer to the panels using data in those time windows. (b) Extent of rebound as observed following sleep deprivation as performed in a. Panels a and b reproduce data from Geissmann et al., 2019a. (c) Confusion matrix showing the classification using coccinella of the baseline time series. No accuracy gain compared to the random classifier. (d) Confusion matrix of the rebound data. The classification finds two clusters, separated by the 300 s threshold (thick black lines).
Additional files
-
Supplementary file 1
Table listing of all the compounds used in this study, each with its relative bibliographic reference.
- https://cdn.elifesciences.org/articles/86695/elife-86695-supp1-v1.zip
-
Supplementary file 2
Two Jupyter notebooks guiding the user through the integration of ethoscope data with highly comparative time-series analysis (HCTSA; notebook 1) and Catch22 (notebook 2).
- https://cdn.elifesciences.org/articles/86695/elife-86695-supp2-v1.zip
-
MDAR checklist
- https://cdn.elifesciences.org/articles/86695/elife-86695-mdarchecklist1-v1.pdf