Transcriptional cartography integrates multiscale biology of the human cortex
Figures
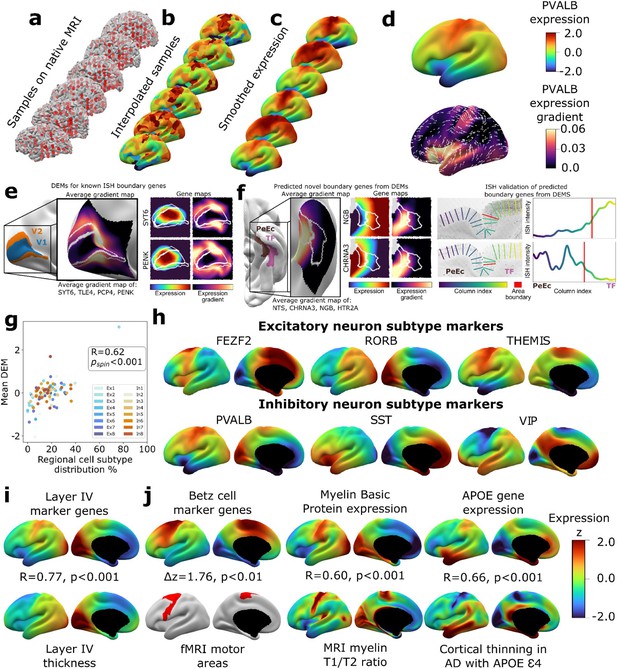
Creating and benchmarking spatial dense gene expression maps in the human cortex.
(a) Spatially discontinuous Allen Human Brain Atlas (AHBA) microarray samples (red points) were aligned with MRI-derived cortical surface mesh reconstructions. (b) AHBA vertex expression values were propagated using nearest-neighbor interpolation and subsequently smoothed (c). (d) Subject-level maps were z-normalized and averaged to generate a single reference dense expression map (DEM) for each gene, as well as the associated expression gradient map (shown here for PVALB: top and bottom, respectively). (e) DEMs can recover known expression boundaries in in situ hybridization (ISH) data. Four canonical V1 area markers (Zeng et al., 2012) show a significantly sharp DEM expression gradient at the V1/V2 boundary (inset cortical map and Figure 1—figure supplement 2a, b), which is also evident in all four individual gene DEMs and DEM gradients (SYT6, PENK, and Figure 1—figure supplement 2c). (f) DEMs can discover previously unknown expression boundaries. Genes with high DEM gradients across the PeEc (parahippocampal) and TF (fusiform) gyri (inset cortical map) were validated in ISH data, showing sharp expression changes in both directions at this boundary (CHRNA3, NGB, and Figure 1—figure supplement 2d-f). (g) Illustrative comparisons of selected DEMs against regional variation in microscale measures of cellular composition: scatterplot showing the global correlation of regional cellular proportions from single nucleus RNAseq (snRNAseq) across 16 cells and 6 regions (Lake et al., 2016) with DEM values for corresponding cell-type marker genes (R = 0.48, pspin<0.001, excluding Ex3-V1 and In8-BA10 outlier samples). (h) DEMs for markers of six neuronal subtypes (three excitatory: FEZF2, RORB, THEMIS; three inhibitory: PVALB, SST, VIP) based on recently validated subtype marker genes (Bakken et al., 2021; Hodge et al., 2019). (i) Illustrative comparison of layer IV marker DEMs with corresponding mesoscale cortical measure of layer IV thickness from a 20 μm 3D histological atlas of cortical layers. (j) Illustrative comparisons of selected DEMs with corresponding macroscale cortical measures from independent neuroimaging markers.
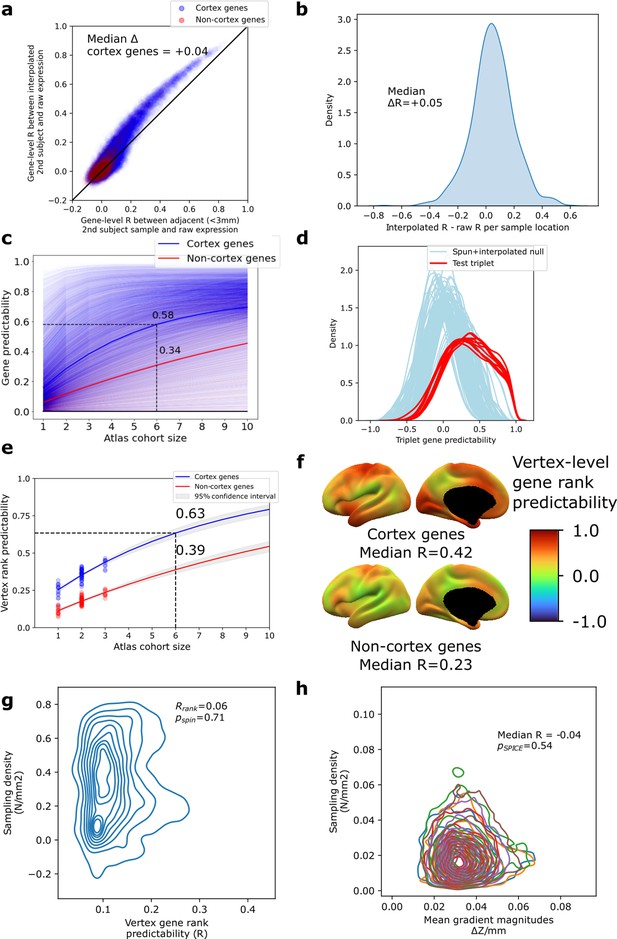
Reproducibility of Dense Expression Maps (DEMs) interpolated from spatially sparse postmortem measures of cortical gene expression.
(a) Signal boost in the interpolated DEM dataset vs. spatially sparse expression data. Restricting to samples taken from approximately the same cortical location in pairs of individuals (within 3mm geodesic distance), there was an overall improvement in intersubject spatial predictability in the interpolated maps. Furthermore, genes with lower predictability in the interpolated maps were less predictable in the raw dataset, suggesting these genes exhibit higher underlying biological variability rather than methodologically introduced bias. (b) Similarly at the paired sample locations, gene-rank predictability was generally improved in DEMs vs. sparse expression data (median change in R from sparse samples to interpolated data for each pair of subjects, +0.5). (c) Gene-level reproducibility of spatial patterns from DEMs independently generated by subsampling the 6 donor cohort into groups of 1, 2 and 3 subjects (median across splits). Learning curve analyses of median gene DEM predictability using DEMs created with subsamples of the full cohort, were generated separately for cortically expressed and non-cortical genes. The spatial reproducibility of DEMs increases with the number of sample donors and can be extrapolated to estimate reproducibility using the full sample size of 6, giving an estimated r of 0.58. (d) Gene predictability was higher across all triplet-triplet pairs than when compared to the spun+interpolated null. (e) Vertex-level reproducibility of gene rankings by scaled expression at each cortical vertex calculated for using the subsampling processes as above. Learning curves were fit to median per vertex predictability of relative gene ranks with different sample cohort sizes. Using the full sample size of 6, cortically expressed genes had an estimated reproducibility of 0.63. (f) Ranking of genes by scaled expression at each cortical vertex is generally reproducible and more so for cortically expressed vs. non-cortically expressed genes. (g) The magnitudes of gradients of DEMs were not associated with the local sampling density. (h) Comparison of the sampling density with the reliability of gene expression rankings showed no relationship.
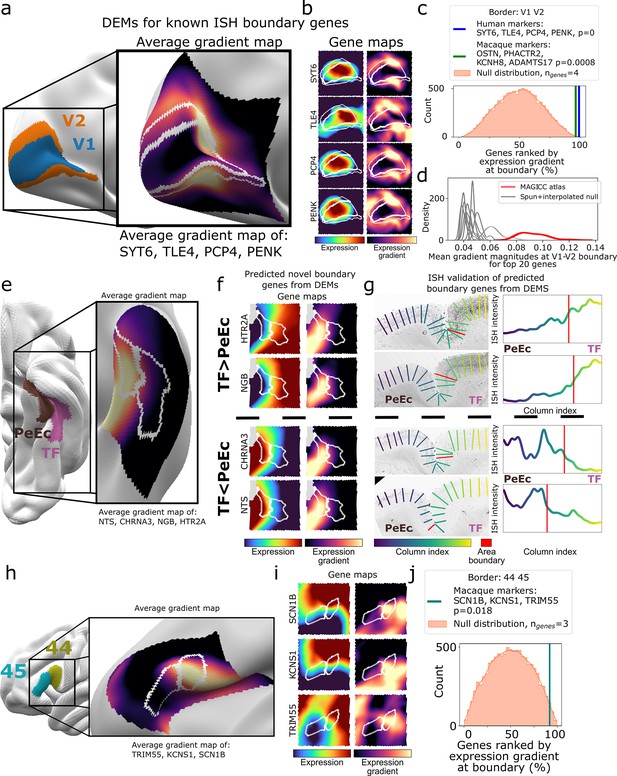
Validating and discovering area marker genes.
(a) Validation local DEM gradients through areal marker genes. Gene expression gradients were averaged along the border between V1 and V2, shown on an inflated cortical surface. The average of four recognised V1 area markers (Zeng et al., 2012 Cell) exhibits high changes in expression along the boundary between V1 and V2. (b) Each marker gene exhibits a clear peak of expression within V1 with high expression gradients in mm outside the region. (c) Mean gradient for known marker genes at the V1 V2 border in both macaques angmd humans were significantly highly ranked relative to randomly sampled groups of genes. (d) Mean of gradient magnitudes for 20 genes with largest gradients along V1-V2 border, compared to values along the same boundary on the spun+interpolated null atlas. Gradients were higher in the actual dataset than in all spun version indicating this high gradient feature is not primarily due to the effects of calcarine sulcus morphology on interpolation. (e) DEM gradients used for discovery of novel area markers. Quantified along the sulcal border between areas PeEc (parahippocampal gyrus) and TF (fusiform gyrus), (f) Each of the four DEM gradient genes exhibited clear expression changes along this border. (g) Putative border genes were validated using available ISH data capturing the same dividing sulcus. These genes exhibited visible and quantifiable changes in expression in the predicted location. (h) DEM gradients in relatively cytoarchitectonically homogeneous frontal regions, 44 and 45, mark areal boundary genes identified in the macaque. (i) DEM gradients for known marker genes at the area 44 / 45 border in macaque were significantly highly ranked relative to randomly sampled groups of genes.
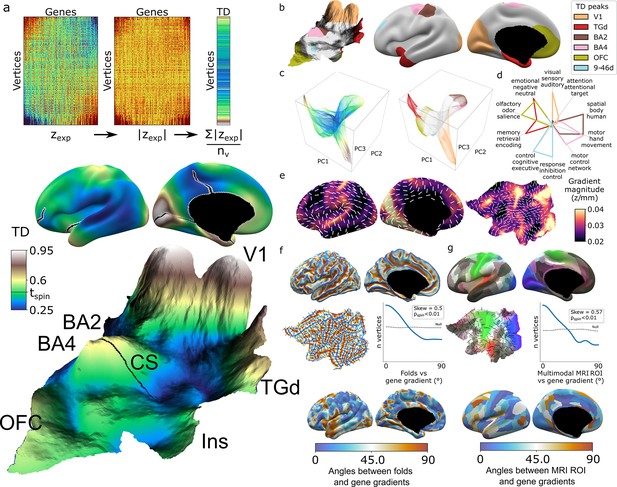
Mapping transcriptional distinctiveness (TD) in the human cortex and its alignment with macroscale structure and function.
(a) Regional TD can be quantified as the mean absolute z-score of dense expression map (DEM) values at each vertex (top) and visualized as a continuous cortical map (middle, TD encoded by color) or in a relief map of the flattened cortical sheet (bottom, TD encoded by color and elevation, Video 1). Black lines on the inflated view identify cuts for the flattening procedure. The cortical relief map is annotated to show the central sulcus (CS), and peaks of TD overlying dorsal sensory and motor cortices (Brodmann areas, BA2, BA4), the primary visual cortex (V1), temporal pole (TGd), insula (Ins), and ventromedial prefrontal cortex (OFC). (b) Thresholding the TD map through spatial permutation of DEMs (tspin ; ‘Materials and methods’) and clustering significant vertices by their expression profile defined six TD peaks in the adult human cortex (depicted as colored regions on terrain and inflated cortical surfaces). (c) Cortical vertices projected into a 3D coordinate system defined by the first three principal components (PCs) of gene expression, colored by the continuous TD metric (left) and TD peaks (right). TD peaks are focal anchors of cortex-wide expression PCs. (d) TD peaks show statistically significant functional specializations in a meta-analysis of in vivo functional MRI data. (e) The average magnitude of local expression transitions across genes (color) and principal orientation of these transitions (white bars) varies across the cortex. (f) Cortical folds in Allen Human Brain Atlas (AHBA) donors (top surface maps and middle flat map) tend to be aligned with the principal orientation of TD change across cortical vertices (p<0.01, middle histogram, sulci running perpendicular to TD change), and the strength of this alignment varies between cortical regions. (g) Putative cortical areas defined by a multimodal in vivo MRI parcellation of the human cortex (Glasser et al., 2016) (top surface maps and middle flat map) also tend to be aligned with the principal direction of gene expression change across cortical vertices (p<0.01, middle histogram, sulci running perpendicular to long axis of area boundaries), and the strength of this alignment varies between cortical areas.
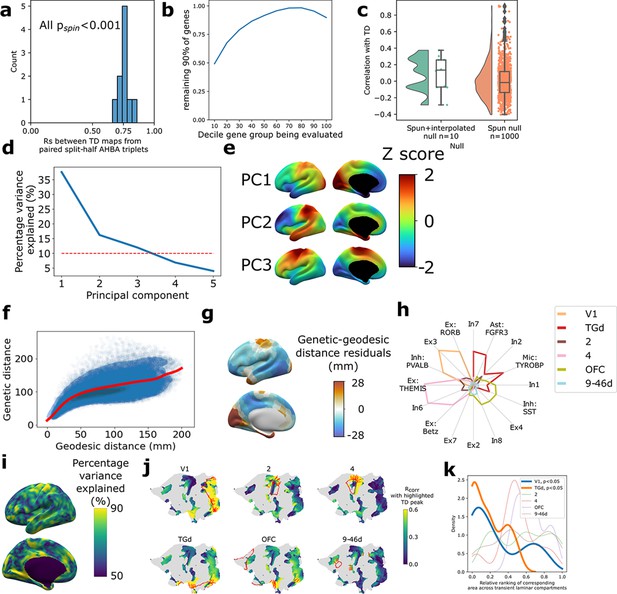
Characterizing bulk transcriptome.
(a) Triplet reproducibility of cortical Transcriptomic Distinctiveness (TD). Across all ten combinations of triplets from the 6-subject cohort, the median correlation between pairs of triplets was r=0.77, pspin<0.001. (b) Genes were grouped into deciles according to the reproducibility of their spatial patterns in independent sub-cohorts (Figure 1—figure supplement 1c). Each decile’s TD map was compared to the map from the remaining 9 deciles. (c) Correlations between TD and TD maps regenerated on datasets spun using two independent nulls, one where the rotation is applied prior to interpolation and smoothing (spun+interpolated) and one where it is applied to the already-created DEMs. In each null, the same rotation matrix is applied to all genes. (d) Percentage variance explained by the first five principal components of cortical gene expression, compared to a spatially permuted null (red-dashed line). (e), DEMs of statistically significant first 3 PCs, shown on an inflated cortical surface. (f), Pairwise inter-regional transcriptomic distances against geodesic cortical distances. Red line shows the prediction from a Generalized Additive Model. (g) Median residual transcriptomic distances for each region, characterizing areas that are transcriptomically further from other areas than their cortical physical embedding would predict. (h) Cortical cell type overlaps with TD peak regions. 21 of 24 DEMs for cortical cell marker genes had peaks located in TD peak regions (pspin<0.001), with TD peaks showing distinctive overlaps. (i) Percentage variance explained by the first principal component of the gene expression gradient vectors. High percentages indicate concerted, anisotropic gene expression gradients. (j) Flattened representations of the cortical sheet with areas of significantly high magnitude gradients. Vertex colors are given by rank correlation of gene gradient magnitudes with gene ranks at the labeled TD peak (outlined). The principal orientation of gene expression gradients are shown in red for selected high magnitude vertices, and can be seen to run perpendicular to TD peak boundaries. (k) The mean expression z-score of high-ranking genes in each TD peak was calculated for measures of gene expression in the fetal cortex at 21 PCW, sampled at six transient fetal layers with an average of 29 regional samples per layer. Because these regional measures of fetal expression sampled multiple cortical regions at different transient fetal layers, we could rank regions within a layer by their expression of genes characterizing a given TD peak and then ask if high-ranking fetal regions for a given TD tended to include regions overlapping that TD (i.e. do fetal visual cortex samples tend to be amongst the highest ranked for V1 TD marker genes from adult DEMs?). These relative intra-layer rankings are shown as density plots for each TD, with bold lines for those two adult TDs (V1 and TGd) that already have localized transcriptional identities at 21 PCW.
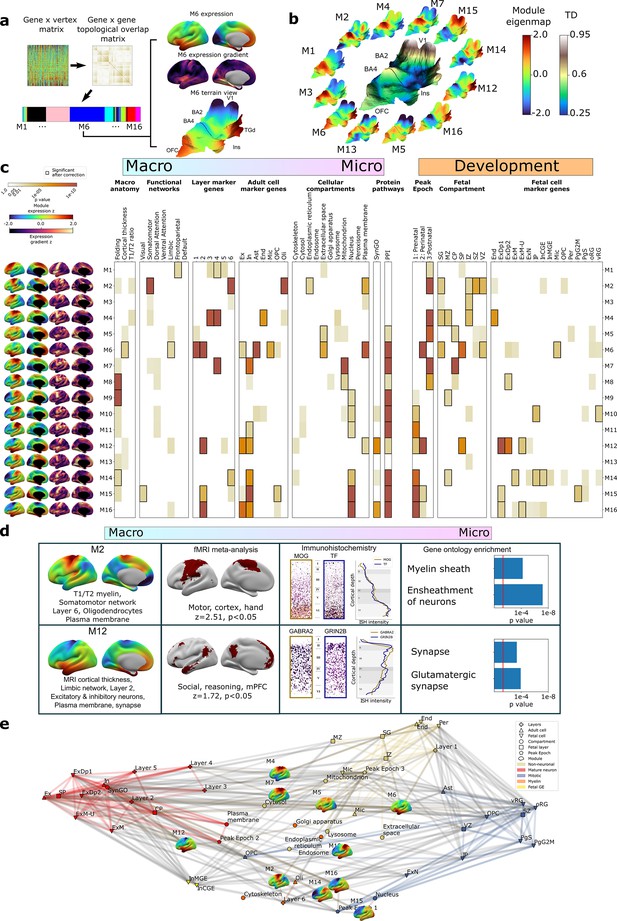
Cortex-wide gene co-expression patterns reflect multiple spatial scales and developmental epochs of brain organization.
(a) Overview of weighted gene co-expression network analysis (WGCNA) pipeline applied to the full dense expression map (DEM) dataset. Starting top left: the pairwise DEM spatial correlation matrix is used to generate a topological overlap matrix between genes (middle top), which is then clustered. Of the 23 WGCNA-defined modules, 7 were significantly enriched for non-cortical genes and removed, leaving 16 modules. Each module is defined by a set of spatially co-expressed genes, for which the principal component of expression can be computed and mapped at each cortical point (eigenmap). M6 is shown as an example projected onto an inflated left hemisphere (M6 z-scored expression and M6 expression change), and the bulk transcriptional distinctiveness (TD) terrain view from Figure 2 (M6 expression). (b) The extremes of WGCNA eigenmaps highlight different peaks in the cortical terrain: the main TD terrain colored by TD value (center, from Figure 2), surrounded by TD terrain projections of selected WGCNA eigenmaps. (c) WGCNA modules (eigenmaps and gradient maps, rows) are enriched for multiscale aspects of cortical organization (columns). Cell color intensity indicates pairwise statistical significance (p<0.05), while black outlines show significance after correction for multiple comparisons across modules. Columns capture key levels of cortical organization at different spatial scales (arranged from macro- to microscale) and developmental epochs: spatial alignment between module eigenmaps and in vivo MRI maps of cortical folding orientation, cortical thickness and T1/T2 ratio, fMRI resting-state functional networks; enrichment for module gene sets for independent annotations (Supplementary file 2) marking: cortical layers (He et al., 2017; Maynard et al., 2021); cell types (Darmanis et al., 2015; Habib et al., 2017; Hodge et al., 2019; Lake et al., 2018; Lake et al., 2016; Li et al., 2018; Ruzicka et al., 2021; Velmeshev et al., 2019; Zhang et al., 2016); subcellular compartments (Binder et al., 2014); synapse-related genes (Koopmans et al., 2019); protein–protein interactions between gene products (Szklarczyk et al., 2019); temporal epochs of peak expression (Werling et al., 2020) (‘fetal’: 8–24 21 post conception weeks [PCW]/’‘perinatal’' 24 PCW–6 mo/‘postnatal’ > 6 mo); transient layers of the mid-fetal human cortex at 21 PCW (Miller et al., 2014) (subpial granular zone [SG], marginal zone [MZ], cortical plate [CP], subplate [SP], intermediate zone [IZ], subventricular zone [SZ], and ventricular zone [VZ]); and fetal cell types at 17–18 PCW (Polioudakis et al., 2019). (d) Independent validation of multiscale enrichments for selected modules M2 and M12. M2 significantly overlaps the Neurosynth topic associated with the terms motor, cortex, and hand. Two high-ranking M2 genes, MOG and TF, exhibit clear layer VI peaks on in situ hybridization (ISH) and GO enrichment analysis myelin-related annotations. M12, overlapping the limbic network most closely overlapped the Neurosynth topic associated with social reasoning. Two high-ranking M22 genes GABRA2 and GRIN2B showed layer II ISH peaks and GO enrichment analysis revealed synaptic annotations. (e) Network visualization of pairwise overlaps between annotational gene sets used in (c), including WGCNA module gene sets (inset expression eigenmaps).
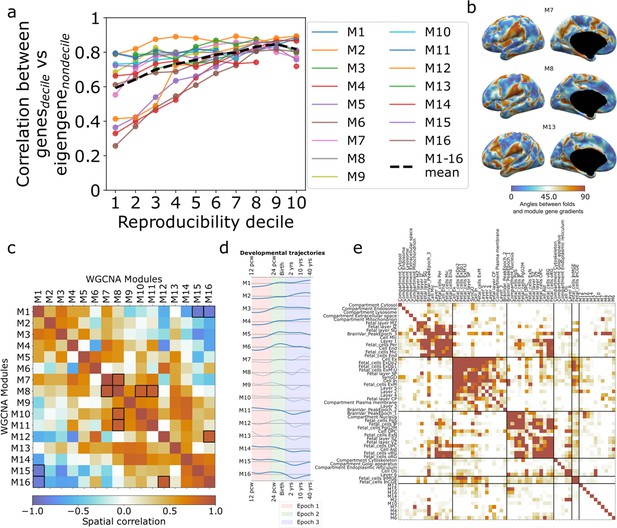
Characterization of WGCNA spatial, developmental and gene-set relationships.
(a) Gene DEMs for each module were stratified into deciles according to their reproducibility score and correlated with their module’s eigengene. Higher reproducibility genes tended to resemble the module eigengene more closely. (b) Strength of cortical folding alignment with WGCNA modules M7, M8 and M13. (c) Spatial correlations between WGCNA module eigenmaps. Significant correlations after controlling for multiple comparisons are outlined in black. (d) Average developmental trajectory of genes in modules. In bold are modules where gene-level trajectories exhibit significantly greater intra-modular correlation than expected by chance. (e) Matrix representation of significant pairwise overlaps between annotational gene sets used in Figure 3c, including WGCNA module gene sets and a UMAP embedding of the matrix is presented in Figure 3e. Black lines demarcate clusters of annotations.
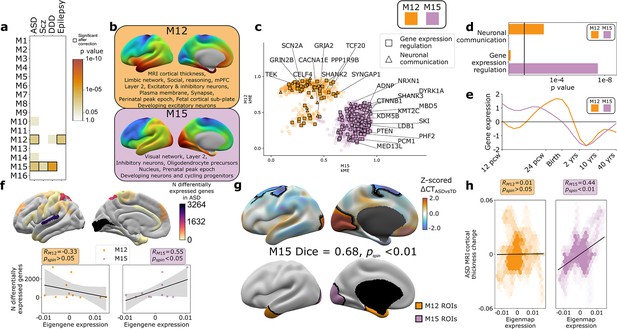
Autism spectrum disorder (ASD) risk genes follow two different spatial patterns of cortical gene expression which differentially predict cortical changes in ASD.
(a) Enrichment of weighted gene co-expression network analysis (WGCNA module gene sets for risk genes associated with atypical brain development through enrichment of rare deleterious variants in studies of ASD, schizophrenia (Scz), severe developmental disorders (DDD, deciphering developmental disorders study), and epilepsy. Cell color intensity indicates pairwise statistical significance (p<0.05)), while outlined matrix cells survived correction for multiple comparisons across modules. (b) Summary of multiscale and developmental annotations from Figure 3c for M12 and M15: the only two WGCNA modules enriched for risk genes of more than one neurodevelopmental disorder. (c) M12 and M15 genes clustered by the strength of their membership to each module. Color encodes module membership. Shape encodes annotations for two GO Biological Process annotations that differ between the module gene sets: neuronal communication and regulation of gene expression. Text denotes specific ASD risk genes. (d) Contrasting GO enrichment of M12 and M15 for neuronal communication and regulation of gene expression GO Biological Process annotations. (e) M12 and M15 differ in the developmental trajectory of their average cortical expression between early fetal and mid-adult life (Li et al., 2018). (f) Regional differences in intrinsic expression of the M15 module (but not the M12 module) in adult cortex is correlated with regional variation in the severity of altered cortical gene expression (number of differentially expressed genes) in ASD (Haney et al., 2020). (g) Statistically significant regional alterations of cortical thickness (CT) in ASD compared to typically developing controls from in vivo neuroimaging (Di Martino et al., 2017) (top). Areas of cortical thickening show a statistically significant spatial overlap (Dice overlap = 0.68, pspin<0.01) with regions of peak intrinsic expression for M15 in adult cortex (bottom). (h) M15 eigenmap expression (but not M12 eigenmap) shows significant spatial correlation with relative CT change in ASD.
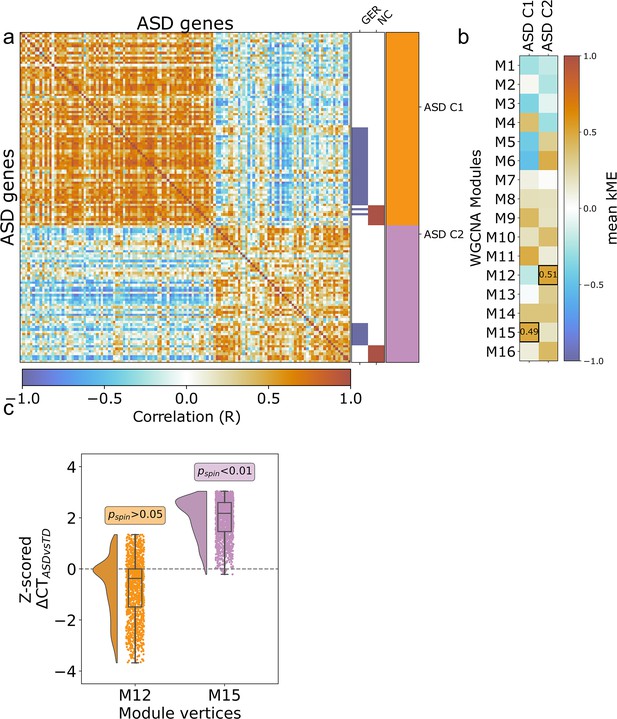
Additional characterisations of Autism Spectrum Disorder (ASD) risk genes and modules.
(a) Clustering of DEMs associated with ASD risk genes identified two contrasting clusters, ASD C1 & ASD C2. C1 was primarily enriched for genes associated with the Gene Expression Regulation, with C2 for Neuronal Communication. (b) Consistent with findings from Figure 4, across all 16 WGCNA modules, DEMs for genes in C1 had the highest mean kME with M15 eigenmap, while genes in C2 had the highest mean kME with M12. (c) The region of peak M15 expression (but not M12 expression) shows significantly greater cortical thickening in ASD than other cortical regions.

Figure S1d: Gene predictability was higher across all triplet-triplet pairs than when compared to spun+interpolated null.
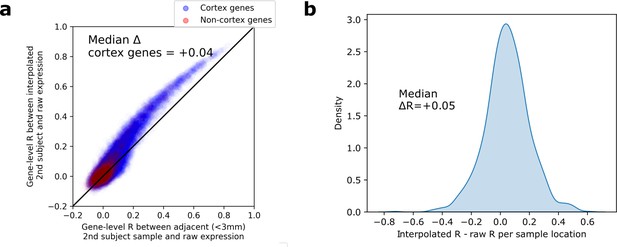
Figure S1: Reproducibility of Dense Expression Maps (DEMs) interpolated from spatially sparse postmortem measures of cortical gene expression.
(a) Signal boost in the interpolated DEM dataset vs. spatially sparse expression data. Restricting to samples taken from approximately the same cortical location in pairs of individuals (within 3mm geodesic distance), there was an overall improvement in intersubject spatial predictability in the interpolated maps. Furthermore, genes with lower predictability in the interpolated maps were less predictable in the raw dataset, suggesting these regions exhibit higher underlying biological variability rather than methodologically introduced bias. (b) Similarly at the paired sample locations, gene-rank predictability was generally improved in DEMs vs. sparse expression data (median change in R from sparse samples to interpolated for each pair of subjects, +0.5).
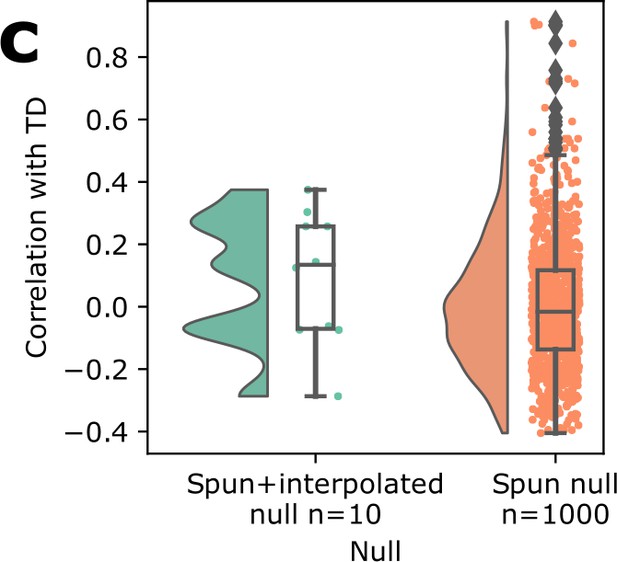
Figure S3c: Correlations between TD and TD maps regenerated on datasets spun using two independent nulls, one where the rotation is applied prior to interpolation and smoothing (spun+interpolated) and one where it is applied to the already-created DEMs.
In each null, the same rotation matrix is applied to all genes.
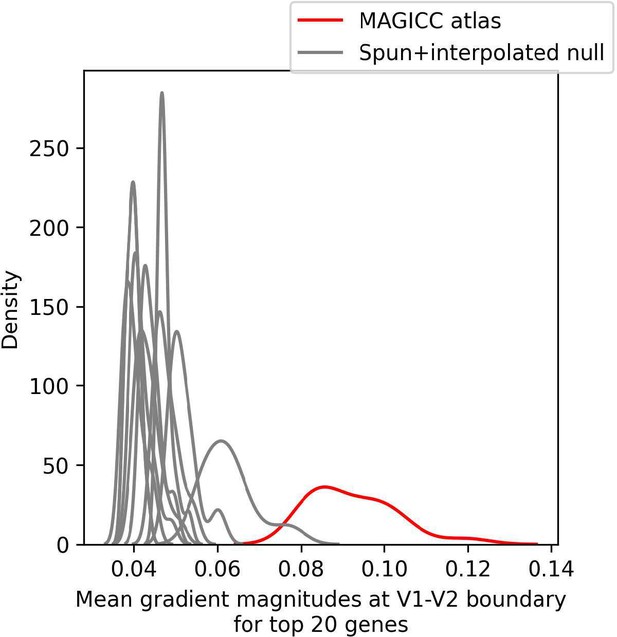
Figure S2d: Mean of gradient magnitudes for 20 genes with largest gradients along V1-V2 border, compared to values along the same boundary on the spun+interpolated null atlas.
Gradients were higher in the actual dataset than in all spun version indicating this high gradient feature is not primarily due to the effects of calcarine sulcus morphology on interpolation
Videos
Visualisation of Transcriptional Distinctiveness (TD) in the human cortex, encoded by both color and elevation.
Tables
Statistical tests used to compare spatial maps and gene sets derived from the Allen Human Brain Atlas with independent multiscale neuroscientific resources.
Input data | Test statistic | Significance test |
---|---|---|
Comparison of two cortical maps, e.g., Figure 1e | Pearson’s R (e.g., Figure 1e and f), Spearman Rrank (Figure 3), delta Z for binary and continuous comparison (Figures 1e and 3c and d), Dice score for two binary maps (Figures 2d and 4g), skew in distribution of angles (Figures 2f and c and 3cFigures 2f & g and 3c). Counts for peak expression locations overlapping ROIs (Figure 2—figure supplement 1h). | Spin test: generate null distribution for test statistic by independently spinning spherical projections of spatial maps and recalculating test statistic on spun maps (Alexander-Bloch et al., 2018). |
Intrasubject alignment of multimodal maps | Pearson’s R (e.g., Figure 1—figure supplement 1g). | Simple permutation-based intermodal correspondence (SPICE) test (Weinstein et al., 2021). |
Comparison of gene–gene connectivity matrix, e.g., protein–protein interaction vs. spatial correlation, gene–gene spatial correlation vs. developmental trajectory correlation | If continuous, threshold matrix at 95%. Fisher’s exact test for significant edge-level overlap. | Fisher’s exact test p-values corrected for multiple comparisons using the Holm–Sidak step down procedure (Holm, 1979). |
Overlap of two gene lists, e.g., Figure 3e | Fisher’s exact test. | Fisher’s exact p-value corrected for multiple comparisons. |
Cortical thickness changes in pathology (in AD, Figure 1e, in ASD, Figure 4g) | Linear model: Vertex cortical thickness ~ Age + sex + group + mean cortical thickness. | Cluster-wise correction. Calculate maximum size of significant clusters on 1000 randomly permuted cohorts, using the 95th centile size as a threshold on the test cohort (Hagler et al., 2006). |
Intramodular trajectory correlation | Pairwise intramodular median rank correlation. | Randomly sampled gene sets of comparable size. |
Protein–protein interaction | Intramodular connectivity. | Random resampling of gene sets with decile-matching for degree. |
-
AD, Alzheimer’s disease; ASD, autism spectrum disorder; ROI, region of interest.
Interpolated | GP regression | |
---|---|---|
Fig 1g Cell types | R=0.62 | R=0.59 |
Fig 1i L4 | R=0.77 | R=0.63 |
Fig 1i Betz | Delta z=1.76 | Delta z=1.31 |
Fig 1i Myelin | R=0.60 | R=0.61 |
Fig 1i APOE | R=-0.52 | R=-0.48 |
Additional files
-
Supplementary file 1
Study participants demographics.
(1) Demographics of adult donors Allen Human Brain Atlas microarray data. (2) Demographics of fetal samples from Allen Institute’s fetal Laser Microarray Dataset. (3) Demographics of included participants for Alzheimer’s disease APOE analysis from the Open Access Series of Imaging Studies (OASIS). (4) Demographics of included participants for autism spectrum disorder analysis from the Autism Brain Imaging Data Exchange (ABIDE) I and II.
- https://cdn.elifesciences.org/articles/86933/elife-86933-supp1-v1.xlsx
-
Supplementary file 2
Gene lists used in the study.
(1) Gene list assignments for enrichment analyses including WGCNA modules. (2) Meta module assignments.
- https://cdn.elifesciences.org/articles/86933/elife-86933-supp2-v1.xlsx
-
Supplementary file 3
Transcriptomically distinctive (TD) peaks.
(1) TD genes, GO, cellular, fetal, and functional annotations. (2) Remaining sheets describe significant Biological Process and Cellular Compartment Gene Ontology annotations for TD peaks.
- https://cdn.elifesciences.org/articles/86933/elife-86933-supp3-v1.xlsx
-
Supplementary file 4
WGCNA module enrichments.
(1) WGCNA module spatial and gene set enrichment p-values. (2) Remaining sheets describe significant Biological Process and Cellular Compartment Gene Ontology annotations for WGCNA modules.
- https://cdn.elifesciences.org/articles/86933/elife-86933-supp4-v1.xlsx
-
MDAR checklist
- https://cdn.elifesciences.org/articles/86933/elife-86933-mdarchecklist1-v1.docx