Molecular consequences of peripheral Influenza A infection on cell populations in the murine hypothalamus
Figures
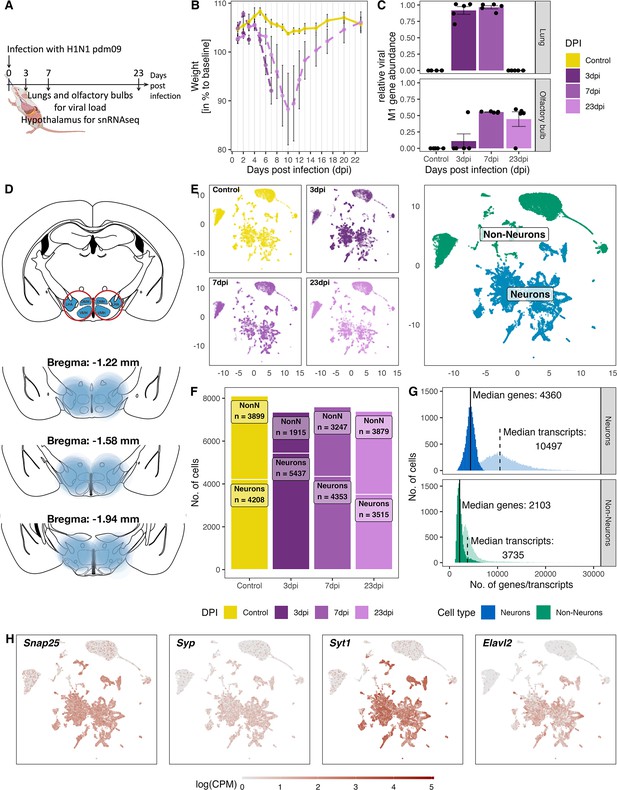
Overview of the experiment, development of the infection, and the resulting snRNA-seq dataset.
(A) Schematic representation of the experiment. (B) Loss of body weight of mice during disease progression until full recovery (n=5 per group). (C) Viral M1 gene abundance in lung and olfactory bulb tissue at the three time points during disease progression and in controls. (D) Location of microdissection punches of the hypothalamus mapped to the mouse brain atlas at distances from bregma of −1.22, –1.58 and −1.94. (E) UMAP dimensional reduction of 30.452 cells, color-coded based on their sample group (different time points and control) membership (left) or their neuronal and non-neuronal identity (right). (F) Bar graph showing the cell counts of neuronal and non-neuronal cells in the different sampling groups. (G) Histograms depicting the distribution of transcript (lighter shading) and genes (solid shading) in all non-neuronal and neuronal cells. (H) Normalized expression of neuronal marker genes (Snap25, Syp, Syt1, Elval2) in all cells shown on a UMAP plot.
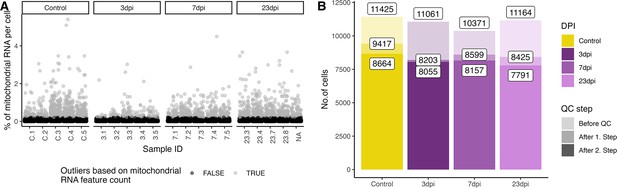
Quality control plots for the generated snRNA-seq dataset.
(A) Percentages of mitochondrial RNA in the different samples. Light gray dots were categorised as outliers and removed form the downstream analysis. (B) Cell counts per time point after different filter steps.
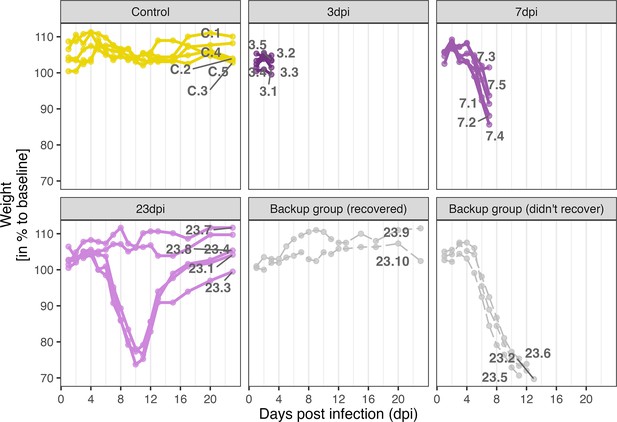
Weight curves depicting the weight-loss due to H1N1 pdm09 infection in the different individuals.
Weight curves are group by time point samples. Grey coloured animals were not used for snRNA-sequencing.

Punching location.
Location of 4 mm punches for RNA extraction and snRNA-seq in the hypothalamus in the different sampling groups.

UMAP embeddings per hash-tagged sample.
UMAP embeddings show the distribution of cells across the different cell types of the individual hash-tagged samples. Colours depict and shadings depict the time points.
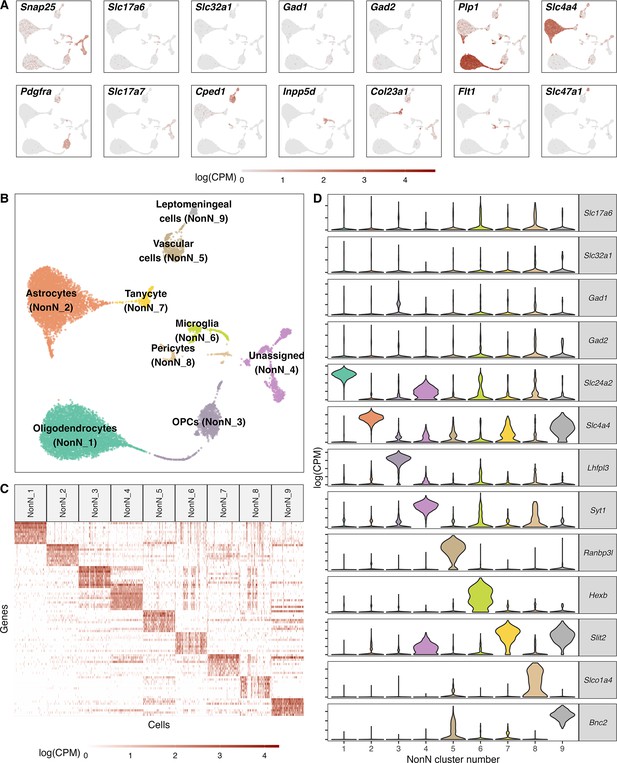
Classification of non-neuronal cell types.
(A) Normalized expression values of different neuronal and non-neuronal cell type markers (pan-neuronal marker - Snap25; Glutamatergic marker - Slc17a6; GABAergic markers - Slc32a1, Gad1, Gad2; Oligodendrocyte marker – Plp1; Astrocyte markers – Slc4a4; Oligodendrocyte precursor marker – Pdgfra; Cped1 – Vascular and leptomeningeal cell markers – Cped1, Slc47a1, Microglia marker – Inpp5d, Tanycytic marker – Col23a1, Pericyte marker – Flt1) in all non-neuronal cells (n=12.940) mapped on a UMAP. (B) Unsupervised clustering of non-neuronal cell types shown in a UMAP embedding and color-coded and annotated by potential cell type annotations. (C) Heatmap of normalized expression values showing discriminatory cell type markers of 9 non-neuronal cell populations. (D) Violin plots showing distribution of normalized expression values of neurotransmitters and best discriminatory cell type markers.
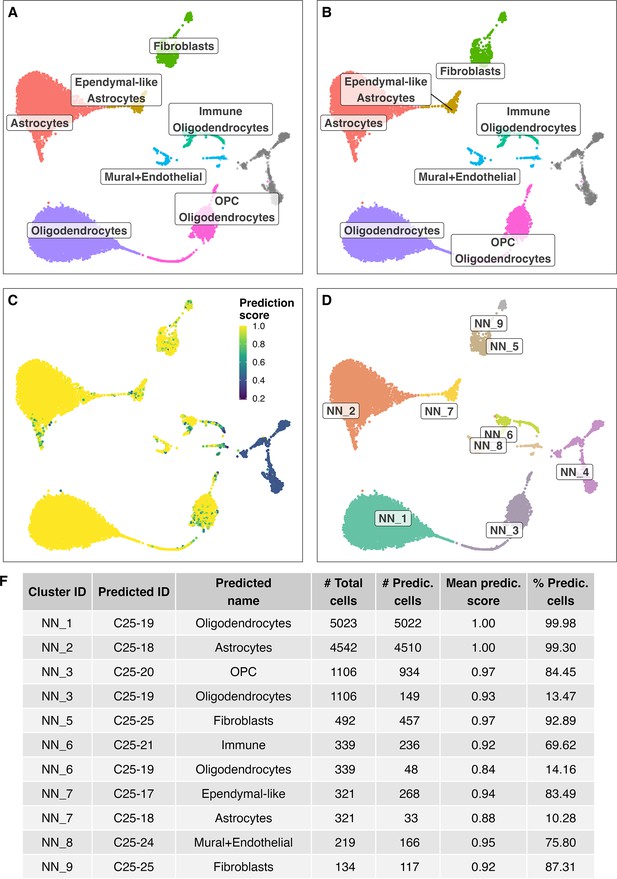
Label transfer of cell-type labels from the HypoMap (Steuernagel et al., 2022) annotations (C7) to the Non-neuronal cell cluster here identified.
(A/B) Shows the cell label transfer for HypoMap cell-type level C7. (C) Depicts prediction scores. (D) Original cell-clusters and labels identified in the here presented study. (F) Detailed overview of predicted names for the different identified cell clusters.
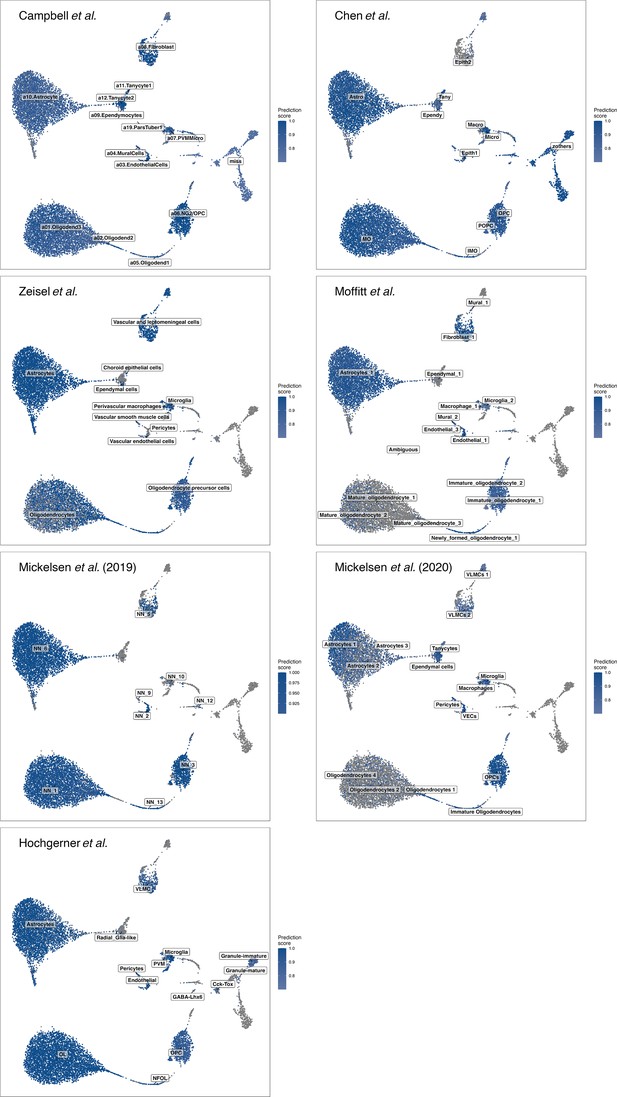
Cell-type label transfer for non-neuronal cells.
Depicting the prediction scores and potential cell-type labels in non-neuronal cells from different published datasets (Campbell et al., 2017; Chen et al., 2017; Hochgerner et al., 2018; Mickelsen et al., 2019; Mickelsen et al., 2020; Moffitt et al., 2018; Zeisel et al., 2018).
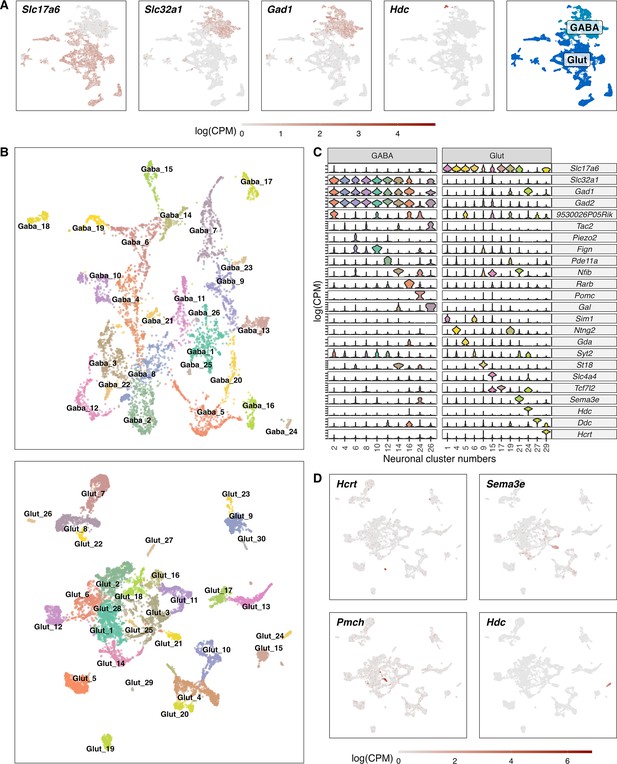
Classification of GABAergic and glutamatergic neuronal cell types in the hypothalamus.
(A) Normalized expression values of different neurotransmitters (Glutamatergic marker - Slc17a6; GABAergic markers - Slc32a1, Gad1, Gad2) in all neuronal cells (left) and a color-coded UMAP projection based on their GABAergic or glutamatergic identity (right) (n=17.512). (B) Un-supervised clustering of GABAergic (upper, n=6.032) and glutamatergic (lower, n=11.481) cells in UMAP plots. Cell type clusters are color-coded and annotated with labels. (C) Violin plots showing normalized expression values of neurotransmitters and discriminating marker genes of selected GABAergic (left) and glutamatergic (right) cell type clusters. (D) Glutamatergic UMAP plots showing normalized expression values of distinct markers for hypothalamic neuron populations (HCRT neurons – upper left, PMCH neurons – lower left, GnRH neurons – upper right, Histaminergic neurons – lower right).
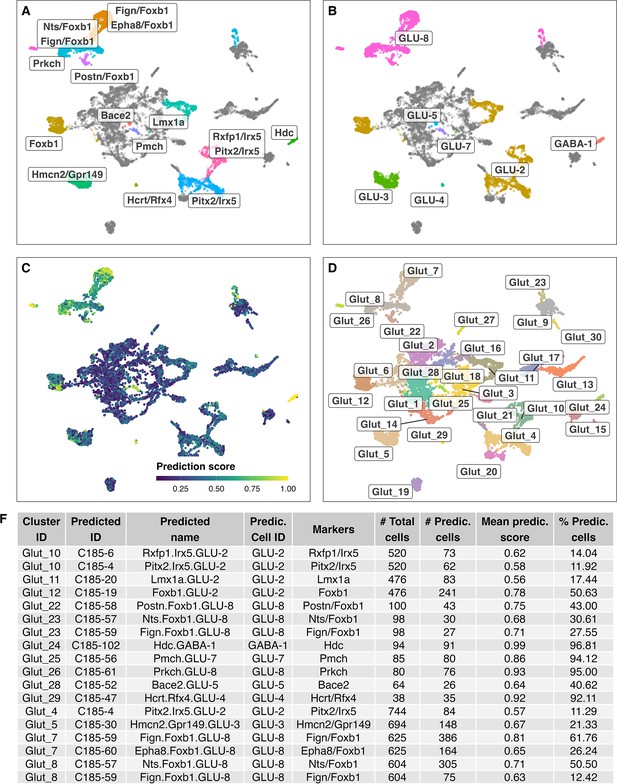
Label transfer of cell-type labels from the HypoMap (Steuernagel et al., 2022) annotations (C285) to glutamatergic cell cluster here identified.
(A/B) Shows the cell label transfer for HypoMap cell-type level C285 (_named).( C) Depicts prediction scores.( D) Original cell-clusters and labels identified in the here presented study.( F) Detailed overview of predicted names for the different identified cell clusters.
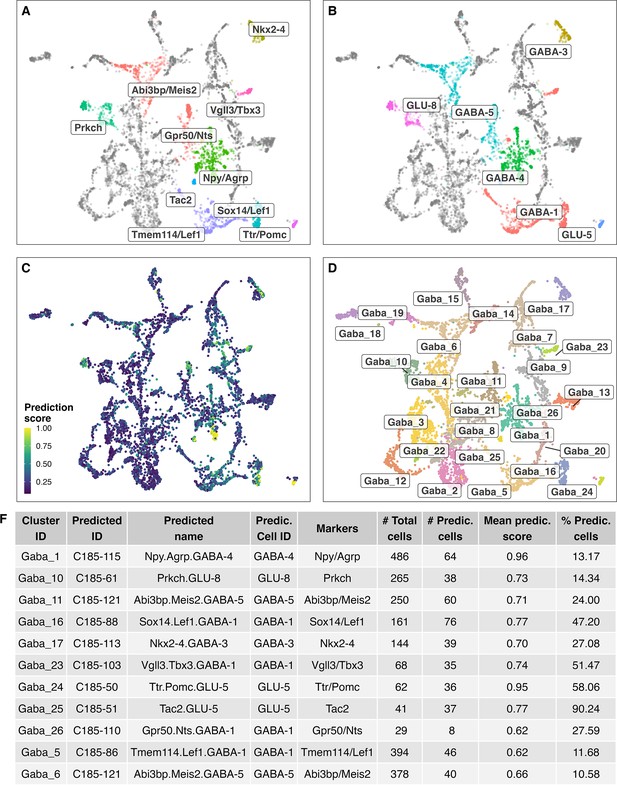
Label transfer of cell-type labels from the HypoMap (Steuernagel et al., 2022) annotations (C285) to GABAergic cell cluster here identified.
(A/B) Shows the cell label transfer for HypoMap cell-type level C285 (_named).( C) Depicts prediction scores.( D) Original cell-clusters and labels identified in the here presented study.( F) Detailed overview of predicted names for the different identified cell clusters.
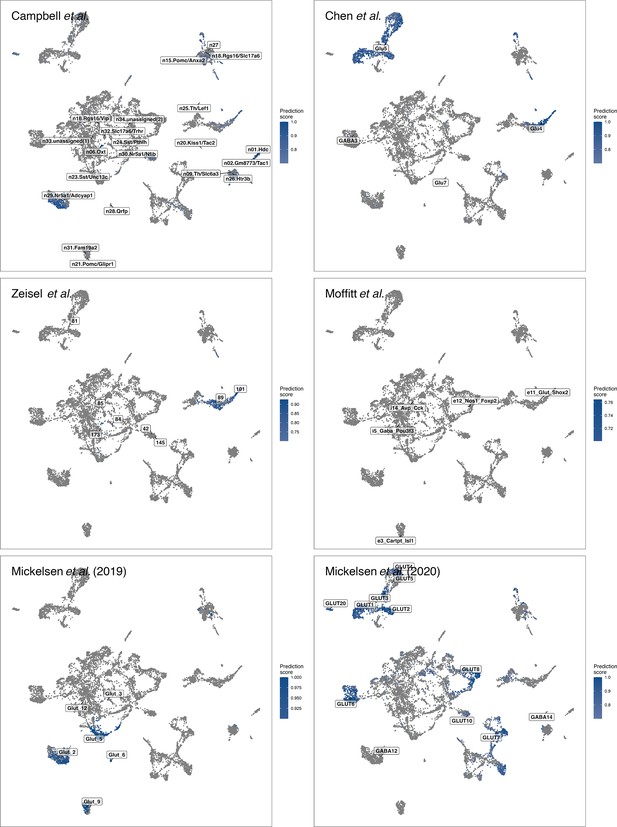
Cell-type label transfer for glutamatergic cells.
Depicting the prediction scores and potential cell-type labels in glutamatergic cells from different published datasets (Campbell et al., 2017; Chen et al., 2017; Mickelsen et al., 2019; Mickelsen et al., 2020; Moffitt et al., 2018; Zeisel et al., 2018).

Cell-type label transfer for GABAergic cells.
Depicting the prediction scores and potential cell-type labels in GABAergic cells from different published datasets (Campbell et al., 2017; Chen et al., 2017; Mickelsen et al., 2019; Mickelsen et al., 2020; Moffitt et al., 2018; Zeisel et al., 2018).
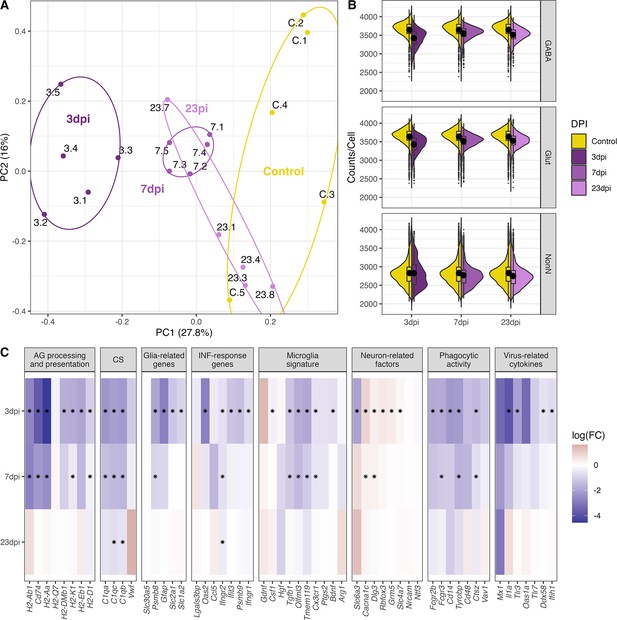
General changes in the transcriptomic landscape of the hypothalamus during peripheral IAV infections.
(A) Principial component plot of combined counts per sample. Each sample snRNA-library were down-sampled to a total of 300 cells (100 non-neuronal, 100 GABAergic, 100 glutamatergic cells) before normalized counts were aggregated before principal component analysis. Samples are color-coded according to their group membership and the numbers correspond to the individual animal IDs. (B) Violin plot showing the distribution of counts per cell at the different time points. The three points of infections are compared to the control group. (C) Heatmap showing log-transformed log-fold changes of known immune-related genes. Black stars indicate significant expression (FDR ≤ 0.05).
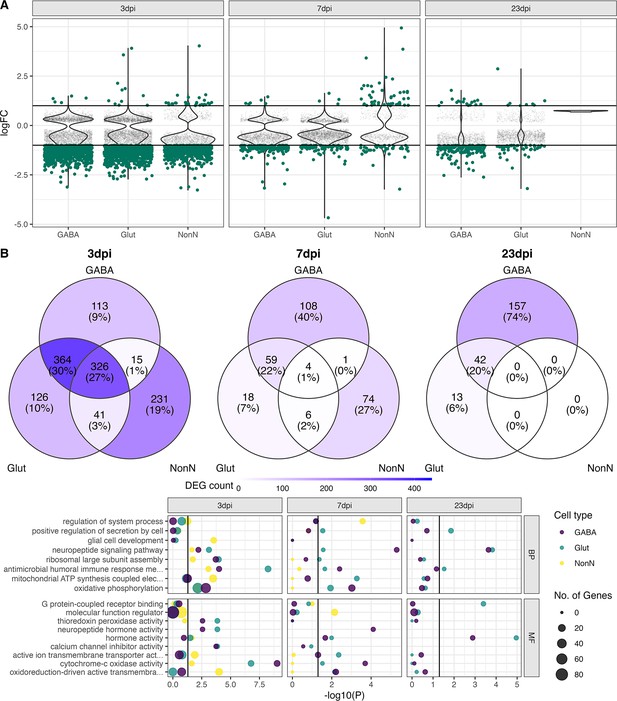
Transcriptional changes in GABAergic and glutamatergic neurons of the hypothalamus during peripheral IAV infections.
(A.) Violin plots showing the log-transformed fold changes (logFC) per gene calculated for the GABAergic, glutamatergic, and non-neuronal cells at 3, 7, and 23 dpi. Dark-green dots represent significantly differential expressed genes (FDR ≤ 0.05) with a logFC greater than 1 or lower than –1. Small grey dots show significantly expressed genes with a logFC between –1 and 1. (B) Venn diagrams depicting the overlap of highly differential expressed genes (logFC >1 or logFC < –1) between the three main cell type clusters at each time point. (C) Gene ontology analysis showing the most significant ontology terms for the category of Biological Process (BP, top) and Molecular Function (MF, bottom). The two highest significant ontology’s for each time point and each cell type were chosen if it included more than 4 annotated genes. The size of the dots indicates the number of genes included in the ontology term and the colour show the cell type annotation.
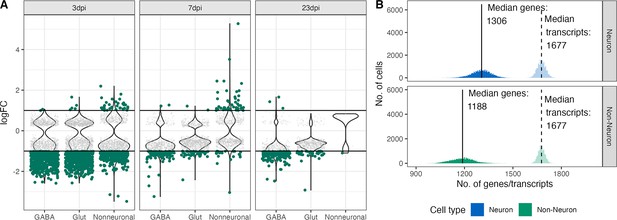
DEG analysis in down-sampled dataset.
lepsy onset is seasonal and incr(A) Scatter- and Violin-plot showing the results of a differential gene expression analysis comparing infected groups against control groups on all Glutamatergic, GABAergic and Non-neuronal cells on a down sampled data set. (B) Histogram showing the distribution of features (genes) and transcripts per cell in a down0sampled dataset (down-sampled to 1600 transcripts per cell).
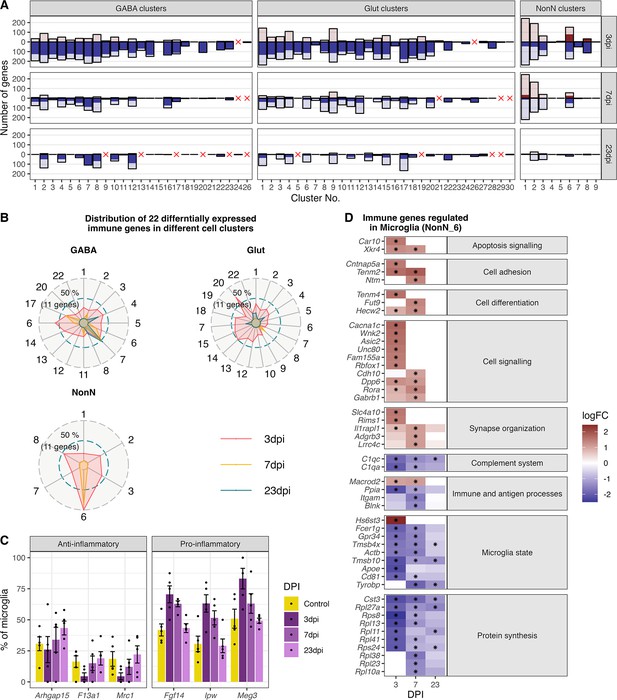
Gene expression changes in different cell type clusters and microglia activation during peripheral IAV infections.
(A) Bar plot showing the number of significantly differentially expressed genes in each cell type cluster at 3, 7, and 23 dpi. Dark solid blue and red bars show the number of highly differential expressed gene (FDR ≤ 0.05, logFC <= –1 or logFC ≥ 1) per cell type cluster and time point. Lighter shaded bars depict the number of all significant regulated genes (FDR ≤ 0.05, logFC ≥ 0 or logFC ≤ 0). Differentially expressed genes (DEGs) were only included for clusters containing at least three cells per sample and per time point in the cluster. A red cross indicates instances where these criteria were not met. (B) Radar plot showing the number of significantly and strongly regulated genes (FDR ≤ 0.05, logFC ≥ 1 or logFC<= –1: n=22) involved in immune processes (based on their gene ontology annotations) per cell cluster at the different time points (red: 3 dpi, yellow: 7 dpi, blue: 23 dpi). (C) Percentage of the number of cells expressing distinct anti- or pro-inflammatory signature genes in the microglia cluster. Cells with a raw count of 0 for a gene were assumed as non-expressing, all other cells were assumed to express this gene. Shown are percentages of microglia cells expressing a gene compared to all microglia at each time point across all samples. (D) Relative changes in expression levels of disease associated genes within the microglia cluster. Data is show as log-transformed fold changes (logFC) of each time point compared with control group. Black stars depict significant differentially expressed genes (FDR ≤ 0.05).

Cluster-based composition shifts calculated by Cacoa.
Box-plots shwoing cluster-based expression shifts of the different infected groups in comparison to the control calculated with the Cacoa package (Petukhov et al., 2022). Shifts on the x-axis in the negative space show a increase of cell proportions in the control samples, whereas a shift towards the positive space indicate an increase in the different infected groups (3, 7, and 23 dpi). Stars depict significance change in cell densities (p ≤ 0.05 after BH correction).

Cluster-based analysis of changes in expression magnitudes.
Boxplots show the shifts in expression magnitudes based on Cacoa package (Petukhov et al., 2022) in the different infected samples compared to the control group. Stars depict a significant change in expression in a cluster (p ≤ 0.05, after BH correction).
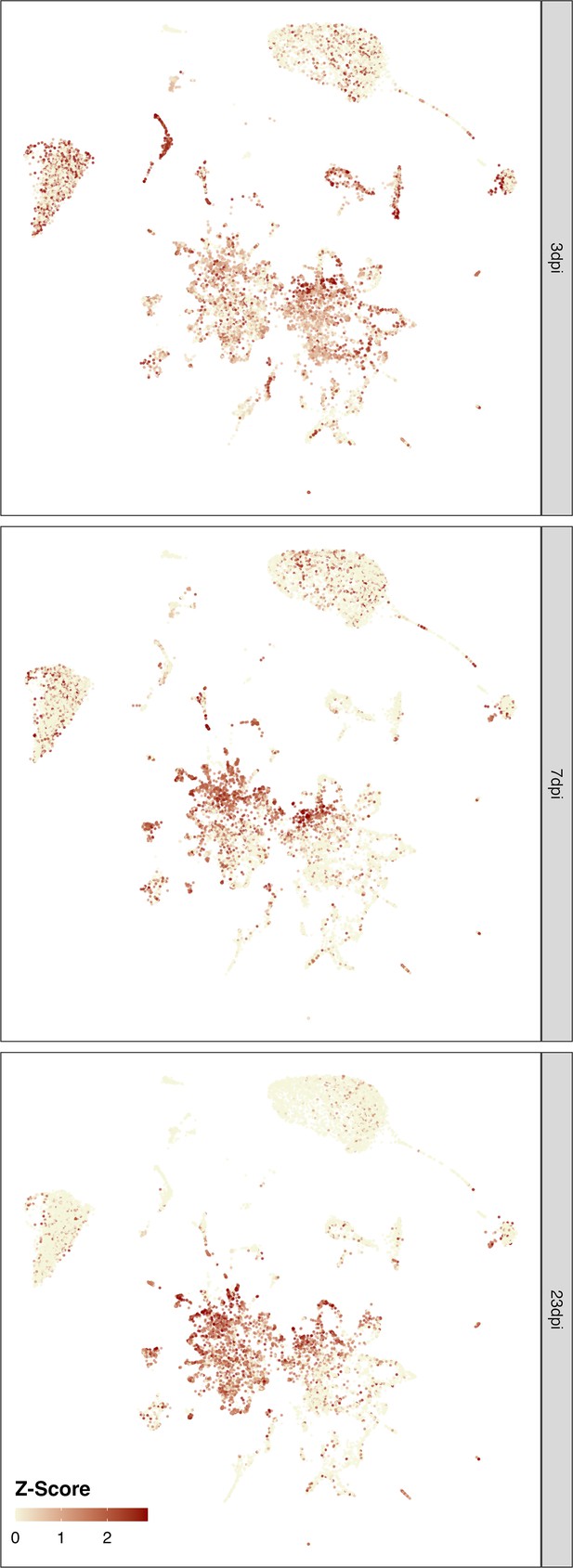
Cluster-free expression shifts.
UMAP embedding showing of the whole dataset showing adjusted statistical significance levels (color) of expression shift magnitudes. Analysis was performed based on Cacoa package (Petukhov et al., 2022).
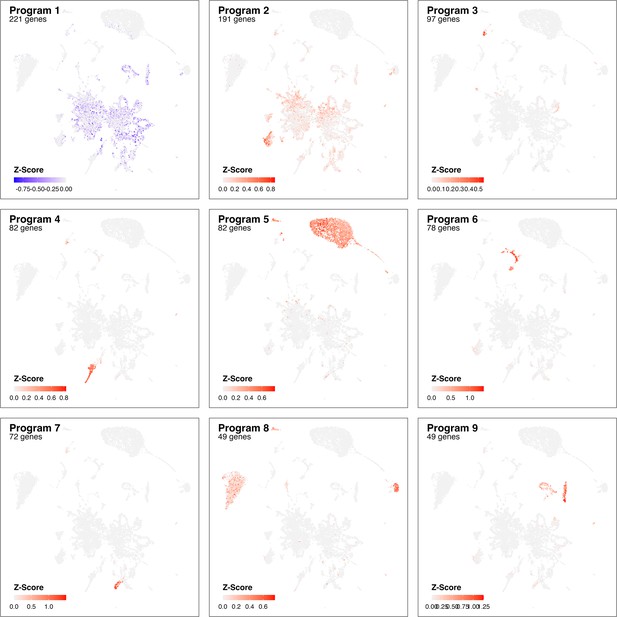
Identified gene programs at 3 dpi based on cluster-free genes expression analysis.
Adjusted z-scores are shown (color) for most pronounce genes programs identified comparing Control samples with infection at 3 dpi.
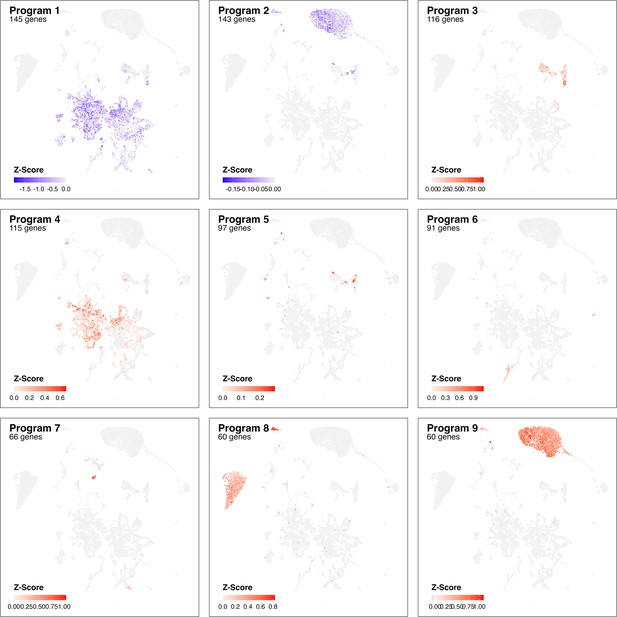
Identified gene programs at 3 dpi based on cluster-free genes expression analysis.
Adjusted z-scores are shown (color) for most pronounce genes programs identified comparing Control samples with infection at 7 dpi.
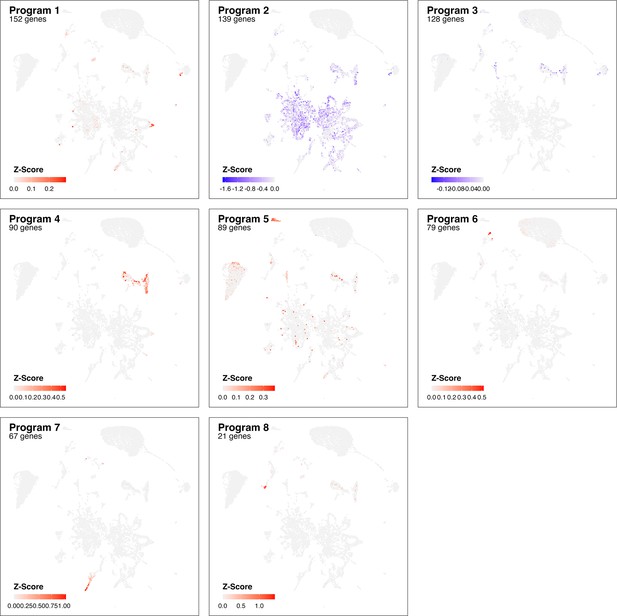
Identified gene programs at 3 dpi based on cluster-free genes expression analysis.
Adjusted z-scores are shown (color) for most pronounce genes programs identified comparing Control samples with infection at 23 dpi.
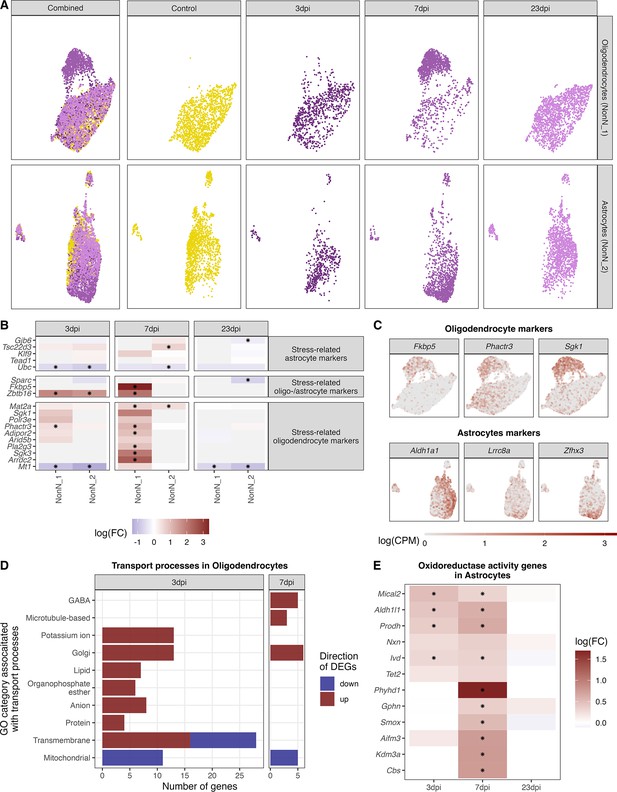
Identification of distinct oligodendrocyte and astrocyte sub-clusters at 7 dpi.
(A) Combined UMAP plot of all cells of the oligodendrocyte (upper right, n=5.023) and astrocyte (lower right, n=4.542) cluster in controls and at different time points. (B) Relative gene expression changes (in comparison of to the mock-infected group) of stress-related oligodendrocyte and astrocyte markers within the oligodendrocyte (NN_1) and astrocyte (NN_2) subcluster. Data is shown as log-transformed fold changes, an asterisks indicates statistical significance (FDR ≤ 0.05). (C) Normalized expression values of selected marker genes at 7-dpi in UMAP plots of all oligodendrocytes or astrocytes. (D) Number of differentially expressed genes (FDR ≤ 0.05, logFC <= –1 or logFC ≥ 1) annotated to gene ontologies associated with transport process in the oligodendrocytes at 3 and 7 dpi. (E) Expression dynamics of differential expressed oxidoreductase genes in the astrocyte cluster at 3, 7, and 23 dpi. A black star indicated significant differential expression (FDR ≤ 0.05).
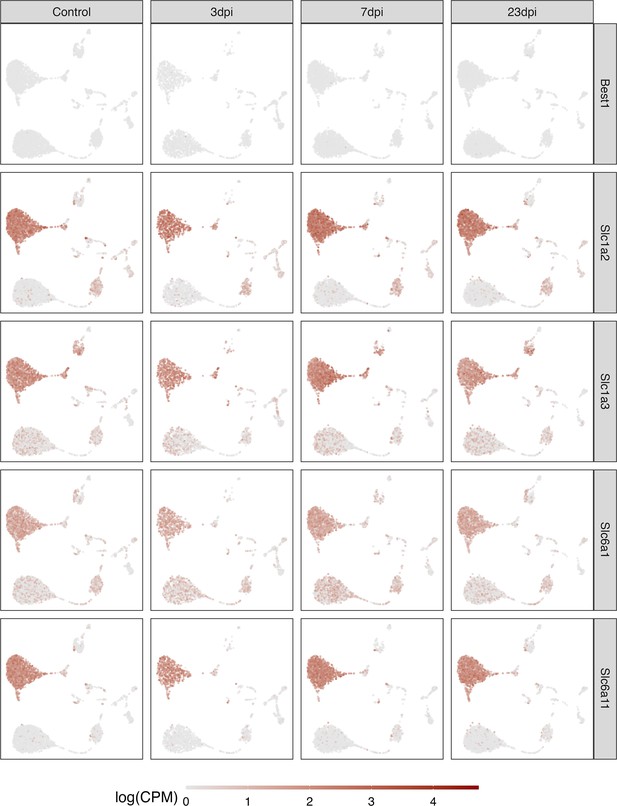
Expression of different GABAergic and glutamatergic transporters.
Depicted are normalized expression levels in non-neuronal cells of different GABAergic and glutamatergic transporters.
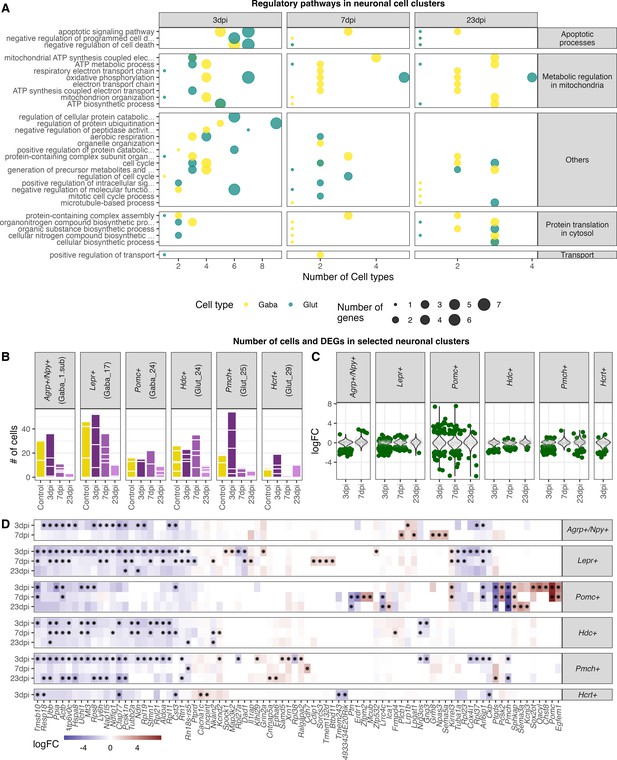
Highly enriched gene ontology categories in neuronal cell populations at different time points of infections.
(A) Dot-plot depicting the number of neuronal cell populations significantly enriched gene ontology categories. Only cell clusters with at least 10 significantly differently expressed genes (logFC <= –1 or logFC ≥ 1, FDR≤ 1) in all three analysed time points were included in the here presented plot. Neuronal cell clusters included are (GABA_2, 4, 6, 8, 10,12; Glut_1, 3, 4, 6, 9, 11, 13, 15, 17). Only significantly enriched (Pelim ≤ 0.05) gene ontology terms were chosen and for each cluster per time point the 10 most enriched GO terms were included. (B) Bar plot depicting the amount of captured nuclei in the individual animals at the different time points for known hypothalamic neuron populations. (C) Differential expressed genes in selected neuron populations. Dark green dots show significantly differential expressed genes (FDR ≤ 0.05). (D) Heatmap showing the five highest and lowest DEGs (FDR ≤ 0.05) in each neuronal population.
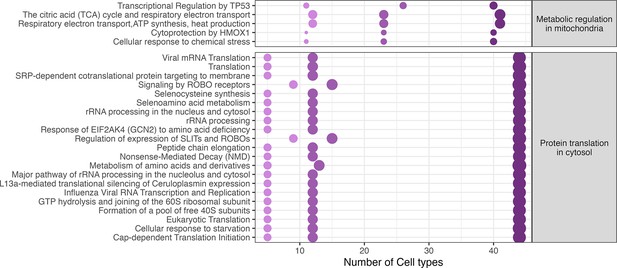
Reactome pathway enrichment of differentially expressed genes in neurons.
Dotplot shows significantly enriched Reachtome pathways in neuronal cell popluations across different timepoints. Gradients of purple depict reflect the time points of analysis. 3 dpi (dark purple) to 23dpi (light purple).
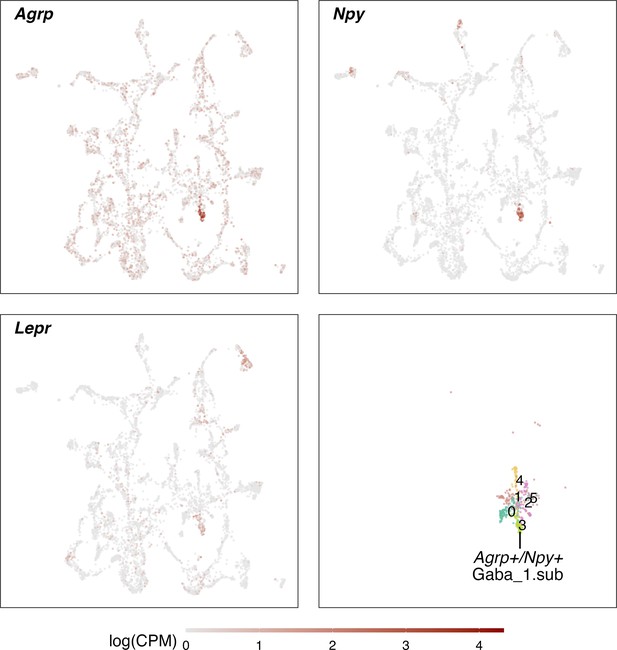
Identification of an Agrp+/Npy +neuron cluster.
Agrp+/Npy +neurons were identified as a subcluster of the GABA_1 neuron population. Depicted are the normalized expression levels of marker genes Agrp, Npy and Lepr, showing their distinct expression in a cell cluster within the GABA_1 cluster. An additional cluster analysis of the GABA_1 cluster, identified them in the subcluster 3 (lower left).
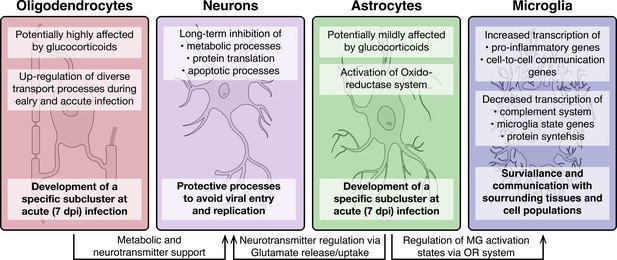
Overview of molecular processes occurring in different cell types within the hypothalamus during a peripheral H1N1 IAV infection.
Schematic overview of the main molecular mechanisms of oligodendrocytes, astrocytes, microglia and neurons during acute to late immune responses of a peripheral IAV infection with the H1N1 pdm09 Influenza A virus.
Additional files
-
Supplementary file 1
Number of cells in different cell populations across different cluster levels.
Sheet QC counts: The dataset underwent different quality control steps. This tables contains the amount of cells per animal at the different control and filter steps. Sheet Cell.counts_celltype.lev1: Table contains the amount of cells for the different sequenced animals in neuronal or non-neuronal cells. Sheet Cell.counts_celltype.lev2:This table contains the amount of cells per anima across all GABAergic, glutamatergic or non-neuronal cells. Sheet Cell.counts_cluster.id: This table shows the number of cells per animal for the different identified cell clusters.
- https://cdn.elifesciences.org/articles/87515/elife-87515-supp1-v2.xlsx
-
Supplementary file 2
Results from cell label transfer from different datasets (see Methods) on cell cluster level.
Containing information about the dataset, reference data ID, number of total cells, number of cells that fitted the prediction score criteria (score >0.5) and percent of cells in cell type tagged with selected prediction score cut-off. Sheet Glut: Results from the cell label transfer for glutamatergic clusters. Sheet Gaba: Results from the cell label transfer for GABAergic clusters. Sheet NonN: Results from the cell label transfer for non-neuronal clusters.
- https://cdn.elifesciences.org/articles/87515/elife-87515-supp2-v2.xlsx
-
Supplementary file 3
Predicted cell cluster annotations based on cell label transfer.
Sheet Selection criteria: Describes the criteria for transferring cell labels to the identified clusters. Sheet NonN: Cell labels for non-neuronal clusters. Sheet Glut: Cell labels for glutamatergic clusters. Sheet GABA: Cell labels for GABAergic clusters.
- https://cdn.elifesciences.org/articles/87515/elife-87515-supp3-v2.xlsx
-
Supplementary file 4
Marker genes identified using a receiver operating characteristic (ROC) method.
Sheet GABA: List of marker genes for GABAergic clusters. Sheet Glut: List of marker genes for glutamatergic clusters. Sheet NonN: List of marker genes for non-neuronal clusters.
- https://cdn.elifesciences.org/articles/87515/elife-87515-supp4-v2.xlsx
-
Supplementary file 5
Results from pseudobulb differential gene expression analysis. The analysis was performed 3 times on different cell type levels.
Sheet 3dpi - DEG - across all cells: Differential expressed genes comparing all control cells vs all cells at 3dpi. Sheet 7dpi - DEG - across all cells: Differential expressed genes comparing all control cells vs all cells at 7dpi. Sheet 23dpi - DEG - across all cells: Differential expressed genes comparing all control cells vs all cells at 23dpi. Sheet GABA - DEG - across celltype: Differential expressed genes from a pseudo-bulk analysis of GABAergic cells. Sheet Glut - DEG - across celltype: Differential expressed genes from a pseudo-bulk analysis of glutamatergic cells. Sheet NonN - DEG - across celltype: Differential expressed genes from a pseudo-bulk analysis of non-neuronal cells. Sheet GABA - DEG - across cellcluster: Differential expressed genes from a pseudo-bulk analysis on GABAergic cell cluster levels. Sheet Glut - DEG - across cellcluster: Differential expressed genes from a pseudo-bulk analysis on glutamatergic cell cluster levels. Sheet NonN - DEG - across cellcluster: Differential expressed genes from a pseudo-bulk analysis on non-neuronal cell cluster levels.
- https://cdn.elifesciences.org/articles/87515/elife-87515-supp5-v2.xlsx
-
Supplementary file 6
Results from pseudo-bulk differential gene expression analysis with less restrictive filter criteria on selected neuronal cell clusters (see Methods).
Sheet 3dpi: Analysis results for comparison Control vs 3dpi. Sheet 7dpi: Analysis results for comparison Control vs 7dpi. Sheet 23dpi: Analysis results for comparison Control vs 23dpi.
- https://cdn.elifesciences.org/articles/87515/elife-87515-supp6-v2.xlsx
-
Supplementary file 7
Results from Reactome pathway enrichment analysis.
- https://cdn.elifesciences.org/articles/87515/elife-87515-supp7-v2.xlsx
-
Supplementary file 8
Results from Gene Ontology (GO) enrichment analysis.
- https://cdn.elifesciences.org/articles/87515/elife-87515-supp8-v2.xlsx
-
Supplementary file 9
Gene programs from cluster free expression shift analysis from Cacoa package.
Analysis was performed for Control against the 3 different time points separately. Sheet Program scores UMAP embeddings: Contains the Adjusted z-scores from the identified genes programs at the different time points in all cells. Sheet 3 dpi - gene programs: Contains gene names, sim scores and loadings (for more information see Petukhov et al., 2022) for 9 different gene programs identified comparing cells from control samples against cells at 3 dpi. Sheet 7 dpi - gene programs: Contains gene names, sim scores and loadings (for more information see Petukhov et al., 2022) for 9 different gene programs identified comparing cells from control samples against cells at 7 dpi. Sheet 23 dpi - gene programs: Contains gene names, sim scores and loadings (for more information see Petukhov et al., 2022) for 8 different gene programs identified comparing cells from control samples against cells at 23 dpi.
- https://cdn.elifesciences.org/articles/87515/elife-87515-supp9-v2.xlsx
-
MDAR checklist
- https://cdn.elifesciences.org/articles/87515/elife-87515-mdarchecklist1-v2.pdf