Modeling resource allocation strategies for insecticide-treated bed nets to achieve malaria eradication
Figures
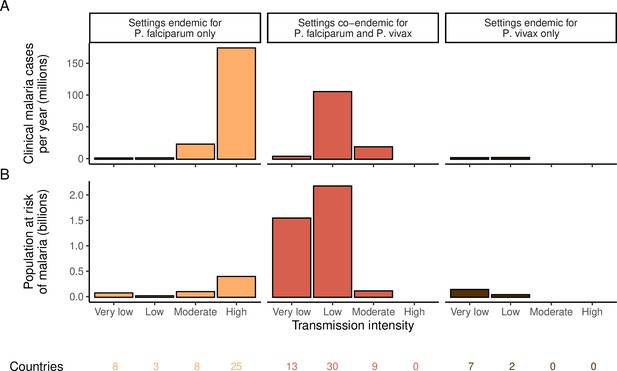
Global distribution of P. falciparum and P. vivax malaria burden in 2000 (in the absence of insecticide-treated nets) obtained from the Malaria Atlas Project (Weiss et al., 2019; Battle et al., 2019).
(A) The annual number of clinical cases and (B) the population at risk of malaria across settings with different transmission intensities and endemic for P. falciparum, P. vivax, or co-endemic for both species. The number of countries in each setting is indicated below the figure.
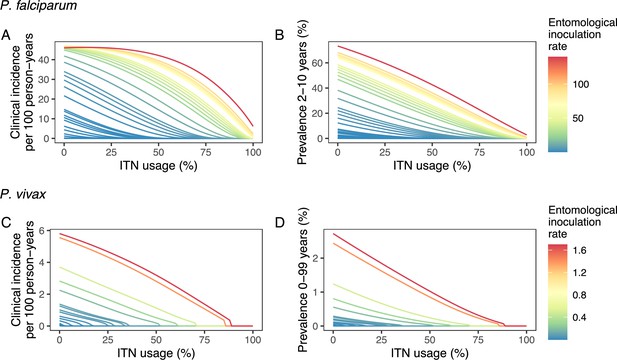
Modeled impact of insecticide-treated net (ITN) usage on malaria epidemiology by the setting-specific transmission intensity, represented by the baseline entomological inoculation rate.
The impact on the clinical incidence and prevalence of P. falciparum malaria (panels A and B) and on the clinical incidence and prevalence of P. vivax malaria (panels C and D) is shown. Panels A and C represent the clinical incidence for all ages.
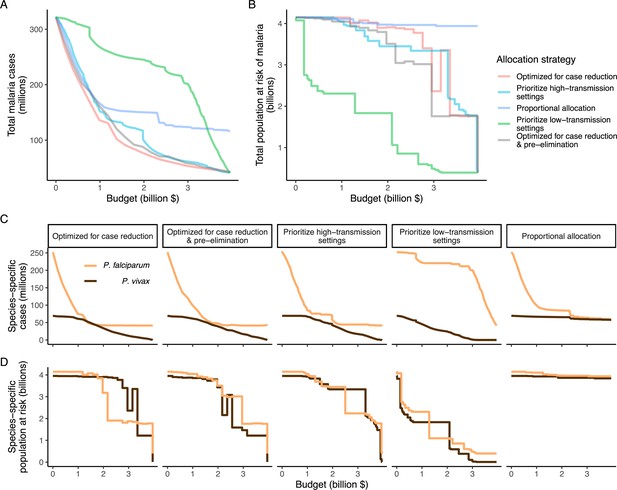
Global clinical cases and population at risk of malaria under different allocation strategies at varying budgets.
The impact on total malaria cases (panel A), total population at risk (panel B), individual P. falciparum and P. vivax cases (panel C), and population at risk of either species (panel D) are shown. Budget levels range from 0, representing no usage of insecticide-treated nets, to the budget required to achieve the maximum possible impact. Optimizing for case reduction generally leads to declining populations at risk as the budget increases, but this is not guaranteed due to the possibility of redistribution of funding between settings to minimize cases. The strategy optimizing case reduction and pre-elimination shown here places the same weighting (1:1) on reaching pre-elimination in a setting as on averting total cases, but conclusions were the same for weights of 0.5–100 on pre-elimination.
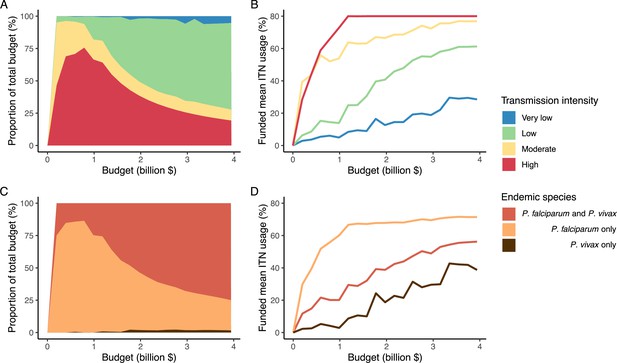
Optimal strategy for funding allocation across settings to minimize malaria case burden at varying budgets.
Panels show optimized allocation patterns across settings of different transmission intensities (panels A and B) and different endemic parasite species (panels C and D). The proportion of the total budget allocated to each setting (panels A and C) and the resulting mean population usage of insecticide-treated nets (ITNs) (panels B and D) are shown.
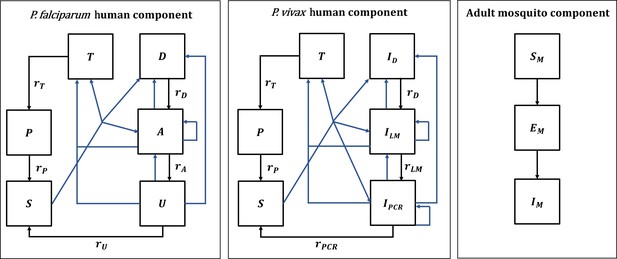
Malaria transmission model; diagram adapted from Griffin et al., 2016 and White et al., 2018.
Humans move through six states in the models for both species: S (susceptible), D (untreated symptomatic infection), T (successfully treated symptomatic infection), A (asymptomatic infection), U (asymptomatic sub-patent infection), and P (prophylaxis) in the P. falciparum model, and S (susceptible), ID (untreated symptomatic infection), T (successfully treated symptomatic infection), ILM (asymptomatic light microscopy detectable blood-stage infection), IPCR (asymptomatic sub-microscopic PCR detectable blood-stage infection) and P (prophylaxis) in the P. vivax model. New infections (including superinfections) are highlighted in blue but parameters are not shown. Rates rD, rA, rU, rT, rP, rLM, and rPCR determine the mean duration of each state. Hypnozoites states in the P. vivax model are not shown on the diagram. Adult female mosquitoes move through three model compartments: Sm (susceptible), Em (exposed), and Im (infected).
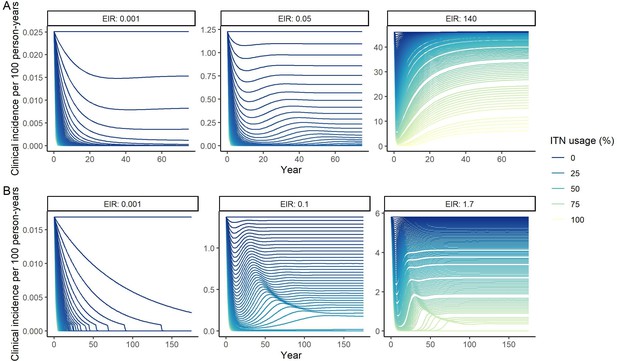
Modeled impact of insecticide-treated net (ITN) usage on clinical incidence over time for three representative entomological inoculation rates (EIR) for (A) P. falciparum and (B) P. vivax.
EIRs represent the minimum, median, and maximum EIRs of the global population distribution. Lines represent increments of 1% of ITN usage.
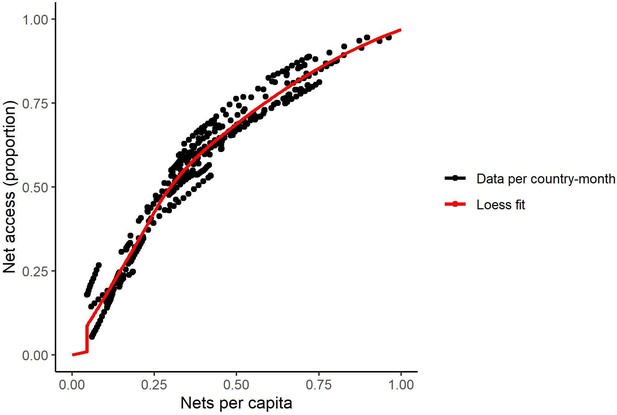
Relationship between access and nets per capita in 2020 (generated from data in Bertozzi-Villa et al., 2021).
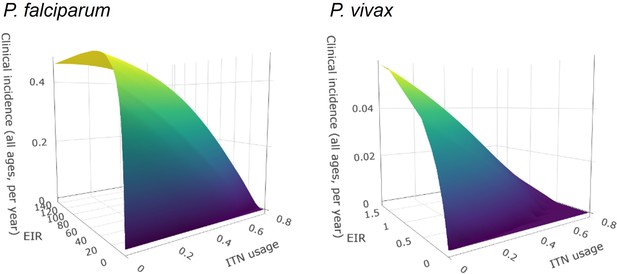
Surface plots of entomological inoculation rate (EIR) vs. insecticide-treated net (ITN) usage vs. clinical incidence.
Plots were fit using bivariate linear interpolation of gridded data EIR and ITN usage values taken from mathematical model simulations.
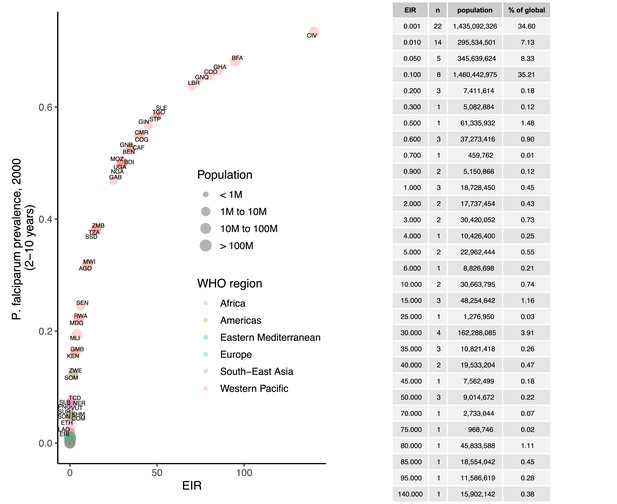
Prevalence of P. falciparum in children 2–10 years (2000), matched to entomological inoculation rate (EIR) values by country.
Points are sized by total population and colored by World Health Organization region.
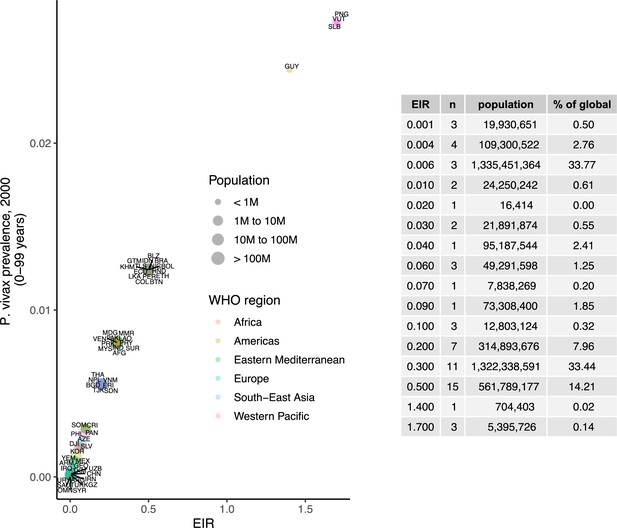
Prevalence of P. vivax in people 0–99 years (2000), matched to entomological inoculation rate (EIR) values by country.
Points are sized by total population and colored by World Health Organization region.
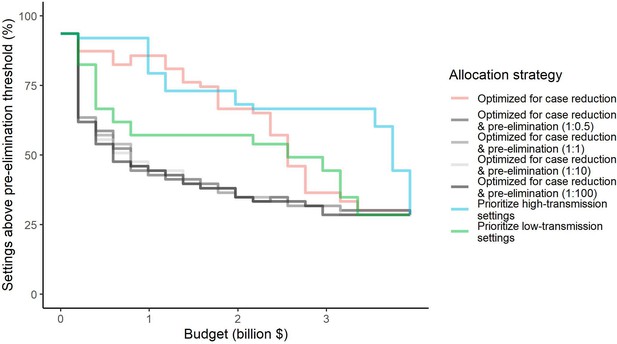
Percentage of settings not having reached pre-elimination (<1 case per 1000 population at risk) under different allocation strategies at varying budgets.
Budget levels range from 0, representing no usage of insecticide-treated nets, to the budget required to achieve the maximum possible impact. For the strategies optimizing for case reduction and pre-elimination, brackets show the weight placed on averting total cases vs on reaching pre-elimination in the optimization (i.e. 1:1 represents equal weight on both).
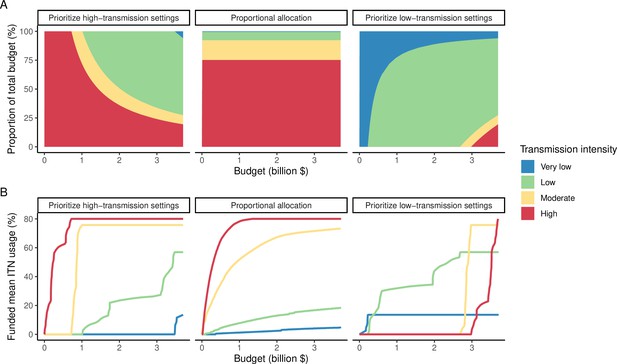
Illustration of resource allocation patterns under the three modeled policy strategies at varying budgets.
(A) Percentage of global funding allocated to each transmission setting. (B) Mean funded insecticide-treated net (ITN) usage in each transmission setting. Transmission intensity groups represent transmission settings with varying population sizes proportional to the global distribution.
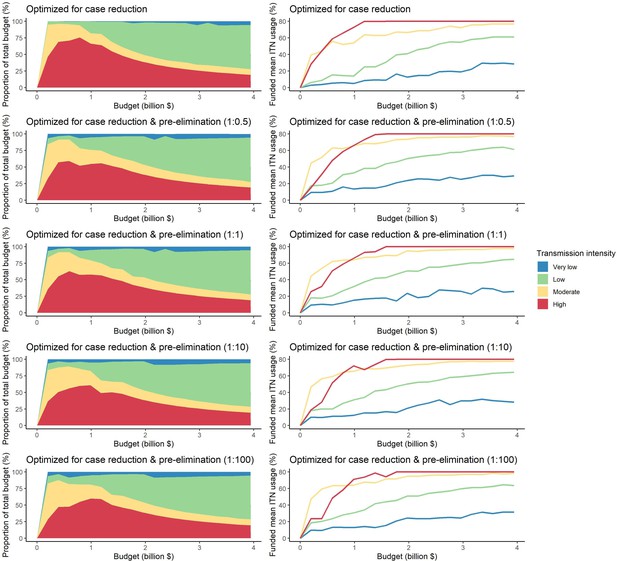
Optimal strategies for funding allocation across settings to minimize malaria case burden (top panels) and to minimize malaria cases and increase the number of settings reaching a pre-elimination phase at varying budgets.
Panels show the proportion of the budget allocated and the resulting mean population usage of insecticide-treated nets (ITNs) across settings of different transmission intensities. For the strategies optimizing for case reduction and pre-elimination, brackets show the weight placed on averting total cases vs on reaching pre-elimination in the optimization (i.e. 1:1 represents equal weight on both).
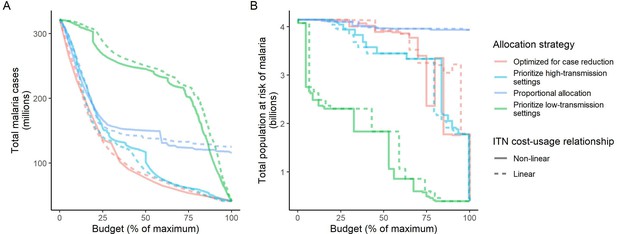
Influence of different assumptions about the relationship between the cost and population usage of insecticide-treated nets (ITNs) on the impact of the allocation strategies.
The global clinical malaria cases (panel A) and the population at risk of malaria (panel B) under different allocation strategies are shown at varying budgets. Results with the more realistic non-linear assumption are presented throughout the main manuscript. Budget levels are expressed relative to the maximum budget required to achieve the largest possible impact with ITNs, but note that this maximum budget was different depending on the ITN costing assumption ($3,698,727,241 for non-linear vs. $6,902,923,309 for linear).
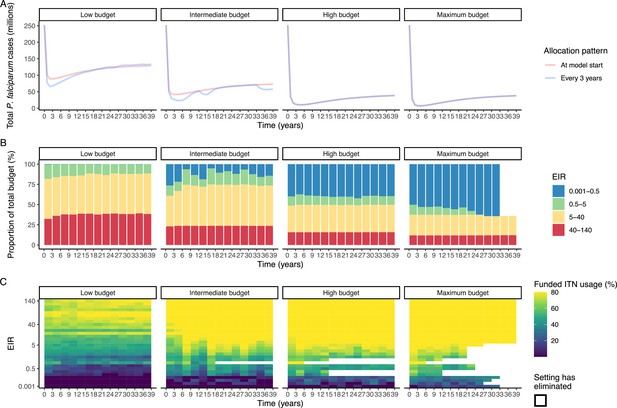
Resource allocation patterns over time for P. falciparum.
Panel A shows the number of cases over time for re-allocation of insecticide-treated nets (ITNs) every 3 years compared to the one-time allocation of a constant ITN usage to minimize the final (year 39) case burden. Panels B and C show the optimal allocation pattern for each 3 year distribution cycle across settings of different transmission intensities. The maximum budget was 26.6 million for ITN distributions every 3 years over 39 years.
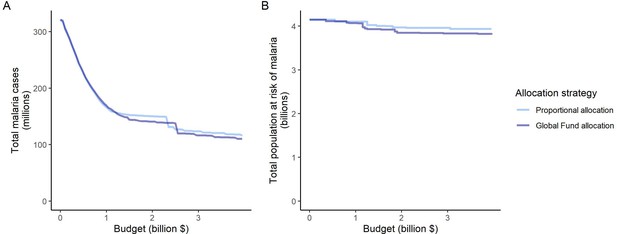
Impact of the proportional allocation strategy and the 2020–2022 Global Fund allocation on global malaria cases (panel A) and the total population at risk of malaria (panel B) at varying budgets.
Both strategies use the same algorithm for budget share allocation based on the malaria disease burden in 2000–2004, but the Global Fund allocation additionally involves an economic capacity component and specific strategic priorities (The Global Fund, 2023).

Impact of the proportional allocation strategy and the 2020-2022 Global Fund allocation on global malaria cases (panel A) and the total population at risk of malaria (panel B) at varying budgets.
Both strategies use the same algorithm for budget share allocation based on malaria disease burden in 2000-2004, but the Global Fund allocation additionally involves an economic capacity component and specific strategic priorities.
Tables
Relative reduction in malaria cases and population at risk under different allocation strategies.
Reductions are shown relative to the baseline of 321 million clinical cases and 4.1 billion persons at risk in the absence of interventions. Low, intermediate, and high budget levels represent 25%, 50%, and 75% of the maximum budget, respectively. The strategy optimizing case reduction and pre-elimination shown here places the same weighting (1:1) on reaching pre-elimination in a setting as on averting total cases.
Clinical cases | Population at risk | ||||
---|---|---|---|---|---|
Scenario | Budget level | Number (millions) | Relative reduction (%) | Number (billions) | Relative reduction (%) |
Optimized for case reduction | Low | 136.1 | 58 | 4.1 | 0 |
Intermediate | 77.0 | 76 | 3.9 | 6 | |
High | 53.9 | 83 | 2.4 | 42 | |
Maximum | 41.5 | 87 | 0.4 | 91 | |
Optimized for case reduction & pre-elimination | Low | 161.3 | 50 | 4.0 | 3 |
Intermediate | 87.8 | 73 | 3.5 | 16 | |
High | 58.8 | 82 | 1.8 | 58 | |
Maximum | 41.5 | 87 | 0.4 | 91 | |
Prioritize high-transmission settings | Low | 153.9 | 52 | 4.0 | 2 |
Intermediate | 109.5 | 66 | 3.4 | 17 | |
High | 61.8 | 81 | 3.3 | 19 | |
Maximum | 41.5 | 87 | 0.4 | 91 | |
Proportional allocation | Low | 166.9 | 48 | 4.1 | 1 |
Intermediate | 150.4 | 53 | 4.0 | 4 | |
High | 123.8 | 61 | 4.0 | 4 | |
Maximum | 116.0 | 64 | 3.9 | 5 | |
Prioritize low-transmission settings | Low | 268.2 | 17 | 2.3 | 44 |
Intermediate | 245.2 | 24 | 1.8 | 56 | |
High | 202.1 | 37 | 0.5 | 88 | |
Maximum | 41.5 | 87 | 0.4 | 91 |
Overview of modeled scenarios for allocation of funding to different transmission settings.
Strategies 1A-1E compare resource allocation scenarios using clinical incidence values from each transmission setting at equilibrium after insecticide-treated net (ITN) coverage has been introduced. Strategies 2A-2B are compared as part of the allocation over time sub-analysis. EIR: entomological inoculation rate.
Strategy | Modeling approach/assumptions | |
---|---|---|
1A | Optimized for total malaria case reduction | Generalized simulated annealing is used to determine the optimal allocation of a given budget to minimize the total number of global malaria cases. |
1B | Optimized for total malaria case reduction and pre-elimination | Generalized simulated annealing is used to determine the optimal allocation of a given budget to minimize the total number of global malaria cases while placing a premium on the pre-elimination phase being reached in a setting. |
1C | Prioritize high-transmission settings | Funding is allocated to groups of countries according to transmission intensity (P. falciparum + P. vivax entomological inoculation rate, EIR). For a given budget, the transmission settings with the highest EIR are prioritized, increasing ITN coverage in increments of 1% in each setting until malaria is eliminated or until an increase in coverage leads to no further decrease in cases, before allocating to the next-highest EIR setting. |
1D | Prioritize low-transmission (near-elimination) settings | Funding is allocated to groups of countries according to transmission intensity (P. falciparum + P. vivax EIR). For a given budget, the transmission settings with the lowest EIR are prioritized, increasing ITN coverage in increments of 1% in each setting until malaria is eliminated or until an increase in coverage leads to no further decrease in cases, before allocating to the next-lowest EIR setting. |
1E | Proportional allocation | Funding is allocated to groups of countries in proportion to their disease burden. Budget shares are calculated using country data from the World Malaria Report (World Health Organization, 2007; World Health Organization, 2020) and account for the country-specific total malaria cases (P. falciparum and P. vivax), deaths, incidence and mortality rate in 2000–2004, scaled by the subsequent increase in the population at risk (The Global Fund, 2019). |
2A | One-time optimized allocation for P. falciparum case reduction | Generalized simulated annealing is used to determine the optimized allocation at a given budget, minimizing the total number of global P. falciparum cases after 39 years, resulting in constant ITN usage in each setting over this time period. |
2B | Optimized allocation every three years for P. falciparum case reduction | Generalized simulated annealing is used to determine the optimized allocation at a given budget, minimizing the total number of global P. falciparum cases after every 3 year period for 39 years, allowing ITN usage to vary in each setting every 3 years. |
P. falciparum human model parameter values.
Full details can be found in the original publication (Griffin et al., 2016), including references for parameters and intervals for the prior and posterior distributions (median values of the posterior distribution are used in model simulations).
Parameter | Symbol | Estimate |
---|---|---|
Human infection duration (days) | ||
Latent period | 12 | |
Patent infection | 195 | |
Clinical disease (untreated) | 5 | |
Treatment of clinical disease | 5 | |
Sub-patent infection | 110.299 | |
Prophylaxis | 15 | |
Age and heterogeneity | ||
Age-dependent biting parameter | 0.85 | |
Age-dependent biting parameter | 8 years | |
Variance of the log heterogeneity in biting rates | 1.67 | |
Pre-erythrocytic immunity reducing probability of infection | ||
Duration of refractory period in which immunity is not boosted | 7.19919 days | |
Duration of pre-erythrocytic immunity | 10 years | |
Maximum probability of infection due to no immunity | 0.590076 | |
Maximum relative reduction in probability of infection due to immunity | 0.5 | |
Scale parameter | 43.8787 | |
Shape parameter | 2.15506 | |
Immunity reducing probability of clinical disease | ||
Duration of refractory period in which immunity is not boosted | 6.06349 days | |
Duration of clinical immunity | 30 years | |
New-born immunity relative to mother’s clinical immunity | 0.774368 | |
Duration of maternal immunity | 67.6952 days | |
Maximum probability of clinical disease due to no immunity | 0.791666 | |
Maximum relative reduction in probability of clinical disease due to immunity | 0.000737 | |
Scale parameter | 18.02366 | |
Shape parameter | 2.36949 | |
Immunity reducing probability of detection | ||
Duration of refractory period in which immunity is not boosted | 9.44512 days | |
Duration of detection immunity | 10 years | |
Minimum probability of detection due to maximum immunity | 0.160527 | |
Scale parameter | 1.577533 | |
Shape parameter | 0.476614 | |
Scale parameter relating age to immunity | 21.9 years | |
Time-scale at which immunity changes with age | 0.007055 | |
Shape parameter relating age to immunity | 4.8183 |
P. vivax human model parameter values.
Full details can be found in the original publication (White et al., 2018) including references for parameters and intervals for the prior and posterior distributions.
Parameter | Symbol | Estimate |
---|---|---|
Human infection duration (days) | ||
Latent period | 10 | |
Light microscopy-detectable asymptomatic infection | 10 | |
Clinical disease (untreated) | 5 | |
Treatment of clinical disease | 1 | |
Prophylaxis | 28 | |
Age, heterogeneity, and probability of infection | ||
Age-dependent biting parameter | 0.85 | |
Age-dependent biting parameter | 8 years | |
Variance of the log heterogeneity in biting rates | 1.29 | |
Probability of blood-stage infection upon infectious mosquito bite | 0.5 | |
Hypnozoite parameters | ||
Relapse rate | 0.024 per day | |
Clearance rate | 0.0026 per day | |
Maternal immunity | ||
New-born immunity relative to mother’s clinical immunity | 0.421 | |
Duration of maternal immunity | 35.148 days | |
Anti-parasite immunity reducing probability of light microscopy-detectable infection and duration of PCR-detectable infection | ||
Duration of refractory period in which immunity is not boosted | 19.77 days | |
Duration of anti-parasite immunity | 10 years | |
Maximum probability of detectability by light microscopy due to no immunity | 0.8918 | |
Minimum probability of detectability by light microscopy due to full immunity | 0.0043 | |
Scale parameter for detectability by light microscopy | 27.52 | |
Shape parameter for detectability by light microscopy | 2.403 | |
Maximum duration of PCR-detectable infection due to no immunity | 70 days | |
Minimum duration of PCR-detectable infection due to full immunity | 10 days | |
Scale parameter for duration of PCR-detectable infection | 9.9 | |
Shape parameter for duration of PCR-detectable infection | 4.602 | |
Clinical immunity reducing probability of clinical disease | ||
Duration of refractory period in which immunity is not boosted | 7.85 days | |
Duration of detection immunity | 30 years | |
Maximum probability of clinical disease due to no immunity | 0.8605 | |
Minimum probability of clinical disease due to full immunity | 0.018 | |
Scale parameter for clinical disease | 11.538 | |
Shape parameter for clinical disease | 2.250 |
Mosquito model and insecticide-treated net (ITN) parameter.
Full details on parameter values can be found in the original publications (Griffin et al., 2010; Griffin et al., 2016; White et al., 2011; White et al., 2018), including references and intervals for the prior and posterior distributions for fitted parameters (median values of the posterior distribution are used in model simulations).
P. falciparum (Anopheles gambiae s.s.) | P. vivax (Anopheles punctulatus) | ||
---|---|---|---|
Infectiousness of humans to mosquitoes | |||
Lag from parasites to infectious gametocytes | 12.5 days | - | |
Untreated clinical disease | 0.068 | 0.8 | |
Treated clinical disease | 0.022 | 0.4 | |
Sub-patent infection | 0.0062 | - | |
Parameter for infectiousness of asymptomatic infection | 1.82425 | - | |
Light microscopy-detectable infection | - | 0.1 | |
PCR-detectable infection | - | 0.035 | |
Mosquito population model | |||
Daily mortality of adult mosquitoes with no interventions | 0.132 | 0.167 | |
Extrinsic incubation period | 10 days | 8.4 days | |
Larval model | |||
Early instar larval developmental period | 6.64 days | 6.64 days | |
Late instar developmental period | 3.72 days | 3.72 days | |
Pupal developmental period | 0.643 days | 0.643 days | |
Daily mortality rate of early-stage larvae (density-dependent) | 0.0338 | 0.0338 | |
Daily mortality rate of late-stage larvae (density-dependent) | 0.0348 | 0.0348 | |
Daily mortality rate of pupae (density-independent) | 0.249 | 0.249 | |
Effect of density dependence on late instars relative to early instars | 13.25 | 13.25 | |
Maximum number of eggs per oviposition per mosquito | 21.2 | 21.2 | |
Mosquito behavior | |||
Mean duration of host-seeking in the absence of vector control interventions | 0.69 days | 0.69 days | |
Mean duration of resting between blood meals | 2.31 days | 2.31 days | |
Proportion of bites taken on humans (anthropophagy) in the absence of vector control interventions | 0.92 | 0.5 | |
Proportion of bites taken on humans indoors and in bed | 0.89 | 0.9 | |
Effect of ITNs | |||
Maximum probability of a mosquito being repelled by a ITN with full insecticidal and barrier effect | 0.56 | 0.6 | |
Minimum probability of a mosquito being repelled by a ITN after decay | 0.24 | 0.2 | |
ITN half-life | - | 2.64 years | 2.64 years |
Maximum probability of a mosquito being killed by a ITN with full insecticidal and barrier effect | 0.41 | 0.3 |
Parameter values for the insecticide-treated net (ITN) costing conversion.
Parameter | Symbol | Value | Source |
---|---|---|---|
ITN usage | - | Varies in simulations | - |
ITN use rate (proportion) | - | 0.84 | Median across African countries in 2019 (Bertozzi-Villa et al., 2021) |
ITN half-life (years) | - | 1.64 | Median across African countries in 2020 (Bertozzi-Villa et al., 2021) |
ITN distribution frequency (years) | 3 | World Malaria Report (World Health Organization, 2007; World Health Organization, 2020) | |
Net loss function rate parameter | 20 | Bertozzi-Villa et al., 2021 |