Multimodal analysis of methylomics and fragmentomics in plasma cell-free DNA for multi-cancer early detection and localization
Figures
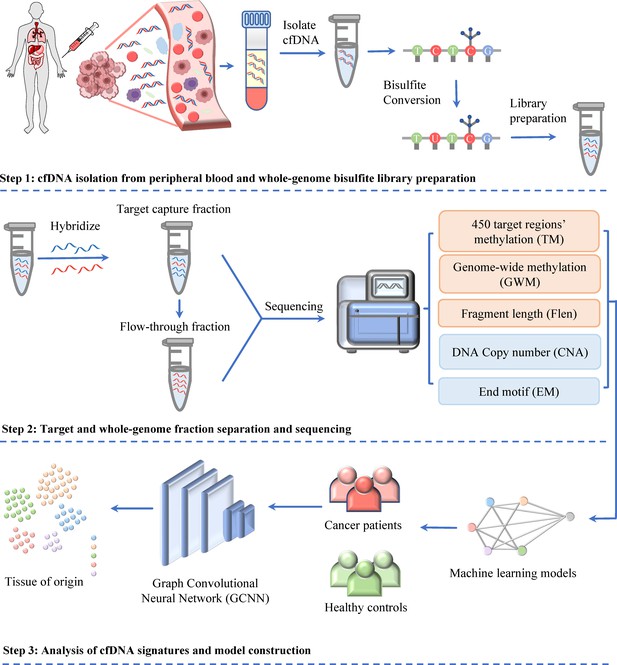
Workflow of SPOT-MAS (screening for the presence of tumor by methylation and size) assay for multi-cancer detection and localization.
There are three main steps in the SPOT-MAS assay. First, cell-free DNA (cfDNA) is isolated from peripheral blood, then treated with bisulfite conversion and adapter ligation to make whole-genome bisulfite cfDNA library. Second, whole-genome bisulfite cfDNA library is subjected to hybridization by probes specific for 450 target regions to collect the target capture fraction. The whole-genome fraction was retrieved by collecting the ‘flow-through’ and hybridized with probes specific for adapter sequences of DNA library. Both the target capture and whole-genome fractions were subjected to massive parallel sequencing and the resulting data were pre-processed into five different features of cfDNA: target methylation (TM), genome-wide methylation (GWM), fragment length profile (FLEN), DNA copy number (CNA), and end motif (EM). Finally, machine learning models and graph convolutional neural networks are adopted for classification of cancer status and identification tissue of origin.
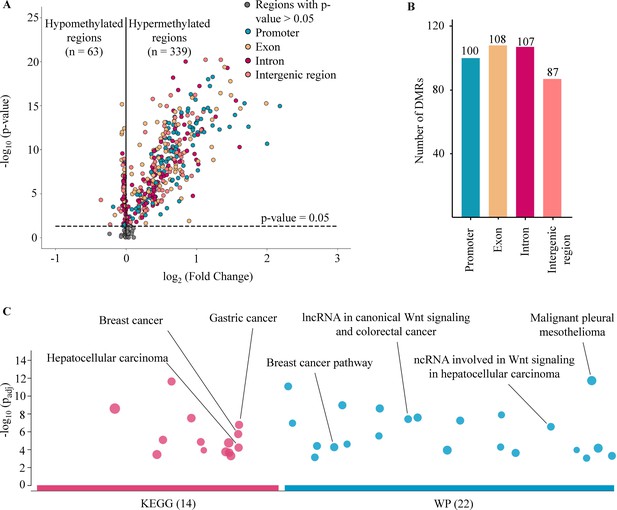
Analysis of targeted methylation in cell-free DNA (cfDNA).
(A) Volcano plot shows log2 fold change (logFC) and significance (-log10 Benjamini-Hochberg adjusted p-value from Wilcoxon rank-sum test) of 450 target regions when comparing 499 cancer patients and 1076 healthy controls in the discovery cohort. There are 402 DMRs (p-value <0.05), color-coded by genomic locations. (B) Number of differentially methylated regions (DMRs) in the four genomic locations. (C) Kyoto Encyclopedia of Genes and Genomes (KEGG) and WikiPathway (WP) pathway enrichment analysis using g:Profiler for genes associated with the DMRs. A total of 36 pathways are enriched, suggesting a link between differences in methylation regions and tumorigenesis.
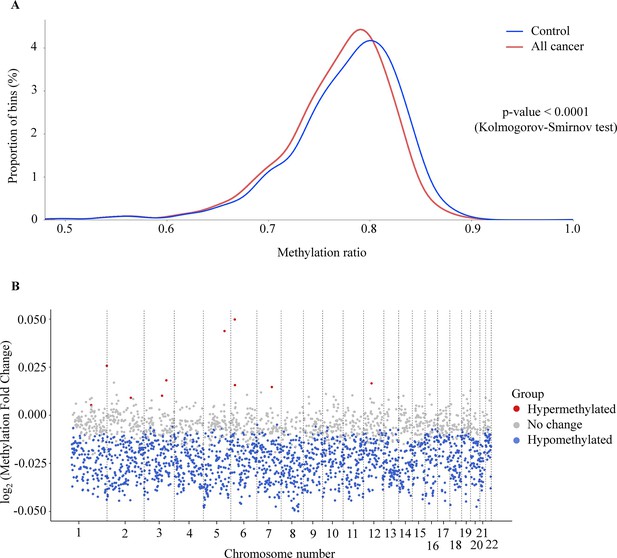
Genome-wide methylation changes in cell-free DNA (cfDNA) of cancer patients.
(A) Density plot showing the distribution of genome-wide methylation ratio for all cancer patients (red curve, n=499) and healthy participants (blue curve, n=1076). The left-ward shift in cancer samples indicates global hypomethylation in the cancer genome (p<0.0001, two-sample Kolmogorov-Smirnov test). (B) Log2 fold change of methylation ratio between cancer patients and healthy participants in each bin across 22 chromosomes. Each dot indicates a bin, identified as hypermethylated (red), hypomethylated (blue), or no significant change in methylation (gray).
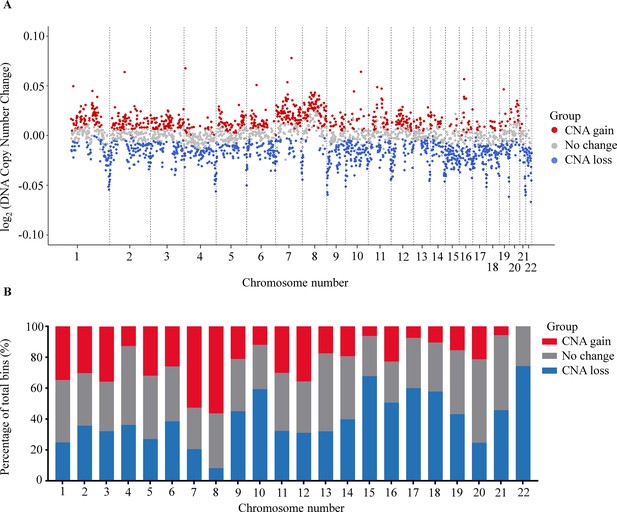
Analysis of copy number aberration (CNA) in cell-free DNA (cfDNA).
(A) Log2 fold change of DNA copy number in each bin across 22 autosomes between 499 cancer patients and 1076 healthy participants in the discovery cohort. Each dot represents a bin identified as gain (red), loss (blue), or no change (gray) in copy number. (B) Proportions of different CNA bins in each autosomes.
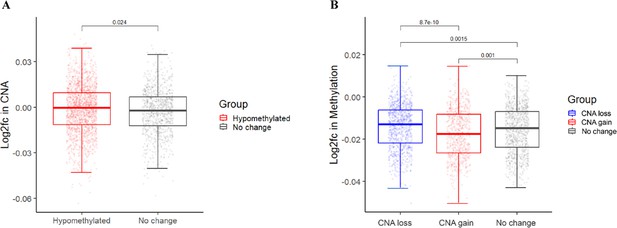
Association between methylation changes and copy number aberration (CNA).
(A) Box plot indicates the log2 fold change in CNA of hypomethylated bins and bins with unchanged methylation. (B) Box plot shows log2 fold change in methylation of bins with CNA gain, loss, or unchanged CNA. p-Value estimated by the one-tailed Mann-Whitney U test.
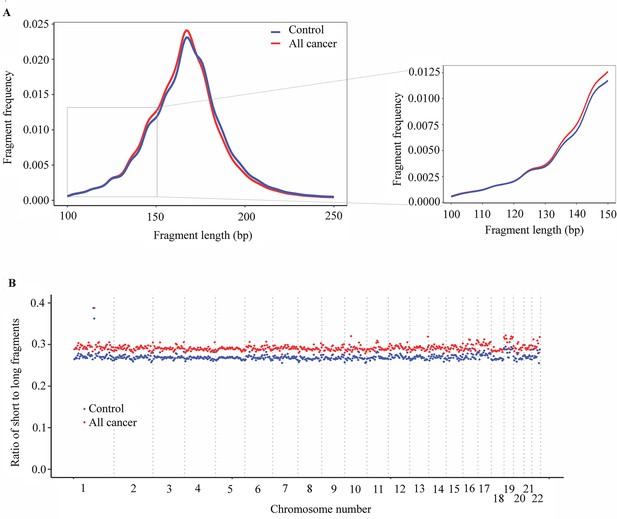
Analysis of fragment length patterns of circulating tumor DNA (ctDNA) in plasma.
(A) Density plot of fragment length between cancer patients (red, n=499) and healthy participants (blue, n=1076) in the discovery cohort. Inset corresponds to an x-axis expansion of short fragment (<150 bp). (B) Ratio of short to long fragments across 22 autosomes. Each dot indicates a mean ratio for each bin in cancer patients (red) and healthy participants (blue).
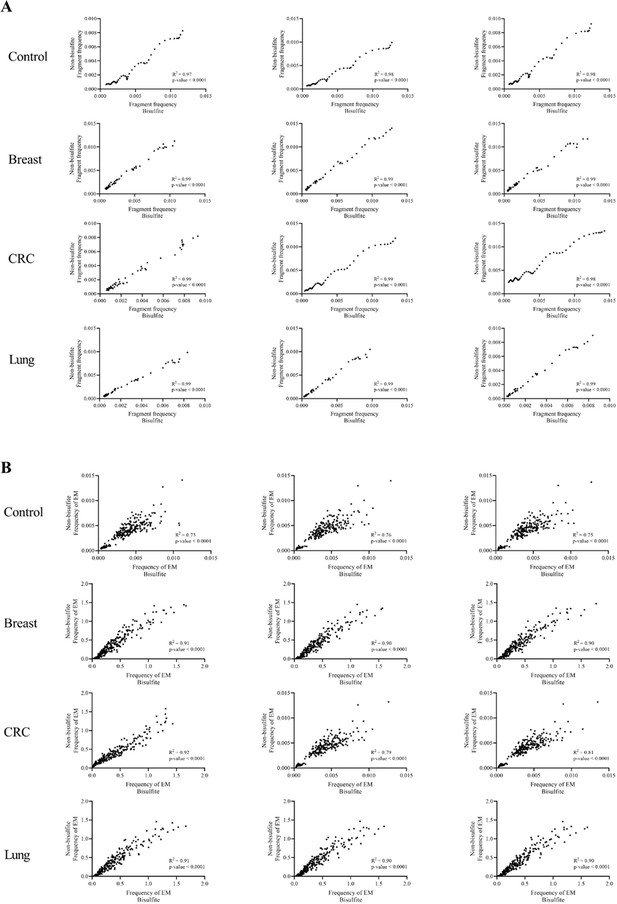
Correlations between bisulfite and non-bisulfite converted data.
Pearson’s correlation analysis shows correlations of fragment length patterns (A) or end motifs (B) between bisulfite and non-bisulfite-treated cell-free DNA (cfDNA) from controls (n=3) and cancer samples (n=9).
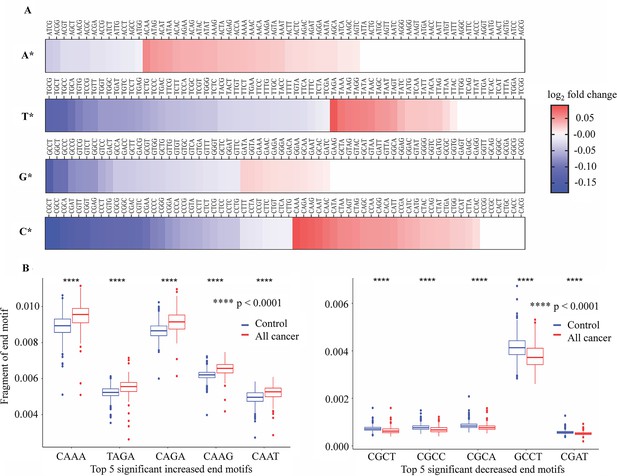
Differences in 4-mer end motif between cancer and healthy cell-free DNA (cfDNA).
(A) Heatmap shows log2 fold change of 256 4-mer end motifs in cancer patients (n=499) compared to healthy controls (n=1076). (B) Box plots showing the top 10 motifs with significant differences in frequency between cancer patients (red) and healthy controls (blue) using Wilcoxon rank-sum test with Bonferroni-adjusted p-value <0.0001.
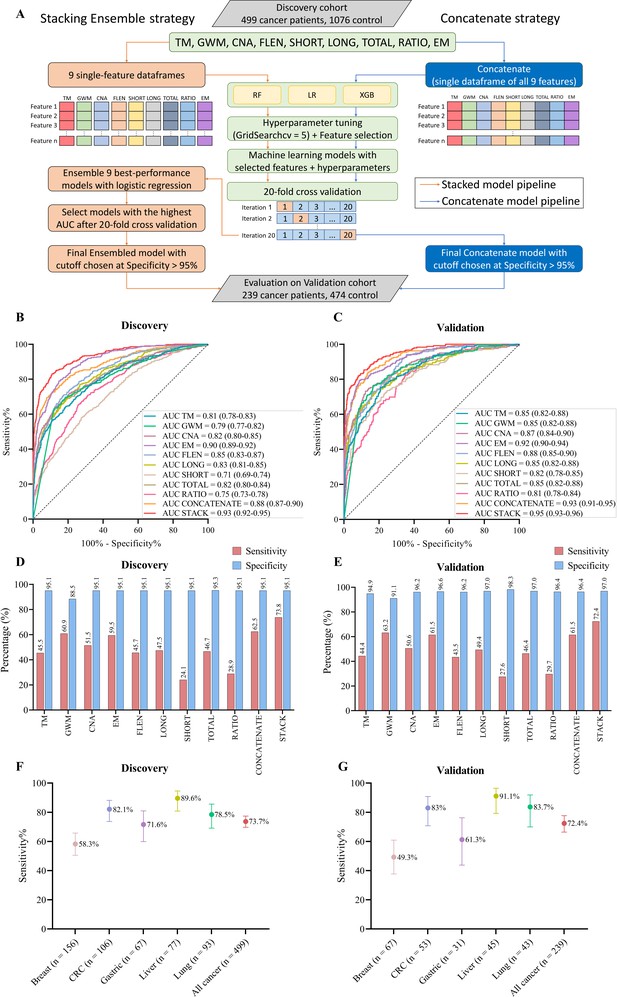
Model construction and performance validation for SPOT-MAS (screening for the presence of tumor by methylation and size).
(A) Two-model construction strategies for cancer detection. (B, C) Receiver operating characteristic (ROC) curves comparing the performance of single-feature models, and two combination models (concatenate and ensemble stacking) in the discovery (B) and validation cohorts (C). (D, E) Bar charts showing the specificity and sensitivity of single-feature models and two combination models (concatenate and ensemble stacking) in the discovery (D) and validation cohorts (E). (F, G) Dot plots showing the sensitivity of SPOT-MAS assay in detection of five different cancer types in the discovery (F) and validation cohorts (G). The points and error bars represent the sensitivity and 95% confidence intervals. Feature abbreviations as follows: TM – target methylation density, GWM – genome-wide methylation density, CNA – copy number aberration, EM – 4-mer end motif, FLEN – fragment length distribution, LONG – long fragment count, SHORT – short fragment count, TOTAL – all fragment count, RATIO – ratio of short/long fragment.
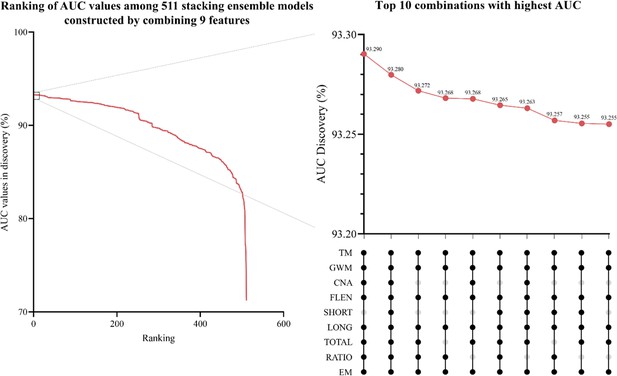
Exhaustive search for the optimal stacking ensemble model.
The red line indicates the area under the curve (AUC) ranking of 511 ensemble combinations. The inset shows the top 10 combinations with the highest AUC value.
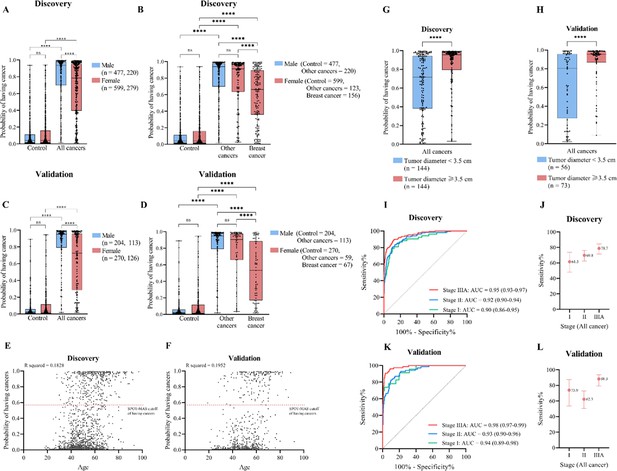
The effects of age, gender, tumor diameter, and cancer stages on model performance.
(A, C) Box plots show probability scores of having cancer for male and female participants in the discovery (A) and validation cohort (C). (B, D) Box plots show probability scores of having cancer for male and female participants when breast cancer samples are separated from the other four cancer types in the discovery (B) and validation cohort (D). (E, F) Pearson’s correlation analysis shows no correlation between age and model prediction scores. (G, H) Box plots show prediction scores of patients with tumor diameter <3.5 cm versus those with tumor diameter >3.5 cm in the discovery (G) and validation cohort (H). (I, K) Receiver operating characteristic (ROC) curves show the classification performance of the stacking ensemble model on cancer patients with different stages (I, II, and IIIA) in the discovery (I) and validation cohort (K). (J, L) Dot plots show the sensitivity and 95% confidence intervals of SPOT-MAS (screening for the presence of tumor by DNA methylation and size) assay in the detection of stage I, II, and IIIA cancer in the discovery (J) and validation cohort (L). (A–D, G–H) Boxes correspond to interquartile ranges (IQR) which include values between 25th to 75th percentile. The horizontal line inside the box indicated the median. The whiskers extended to the smallest or largest data points. The one-tailed Mann-Whitney U test was used to compare the prediction scores among different groups. ns: not significant; ****, p<0.0001.
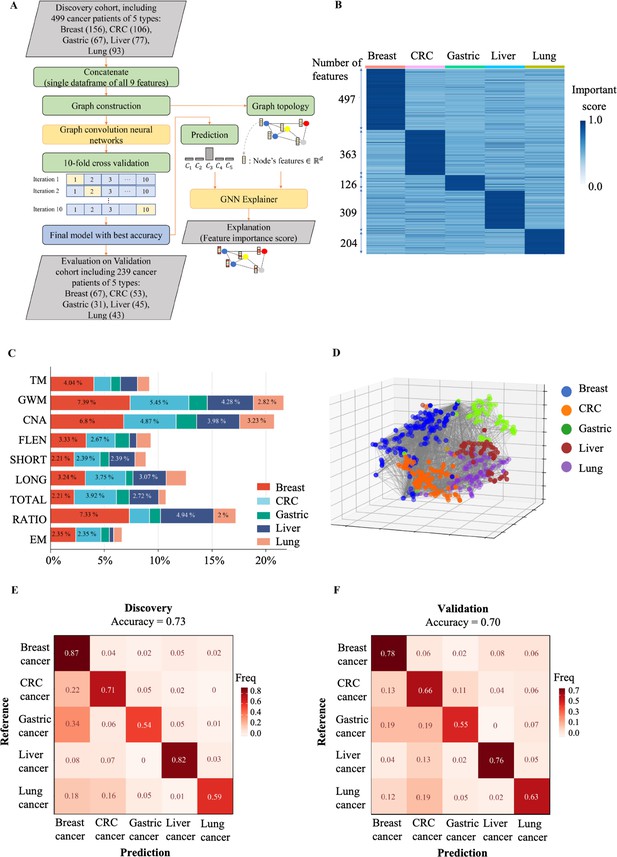
The performance of SPOT-MAS (screening for the presence of tumor by methylation and size) assay in prediction of the tissue of origin.
(A) Model construction strategy to predict tissue of origin by combining nine sets of cell-free DNA (cfDNA) features using graph convolutional neural networks. (B) Heatmap shows feature important scores of five cancer types. (C) Bar chart indicates the contribution of important features for classifying five different cancers. (D) Three dimensions graph represents the classification of five cancer types. (E, F) Cross-tables show agreement between the prediction (x-axis) and the reference (y-axis) to predict tissue of origin in the discovery cohort (E) and validation cohort (F).
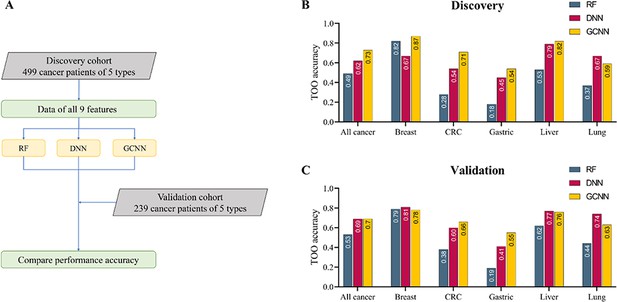
Construction of machine learning models for tissue of origin (TOO) identification.
(A) Model construction strategy. Random forest (RF), convolutional neural network (CNN), and graph convolutional neural network (GCNN) are used to classify the five cancer types from the input of concatenated nine sets of cell-free DNA (cfDNA) features. The performance of constructed models was evaluated on the validation cohort. (B, C) Bar charts comparing the performance accuracy of the three models in the discovery (B) and validation cohort (C).
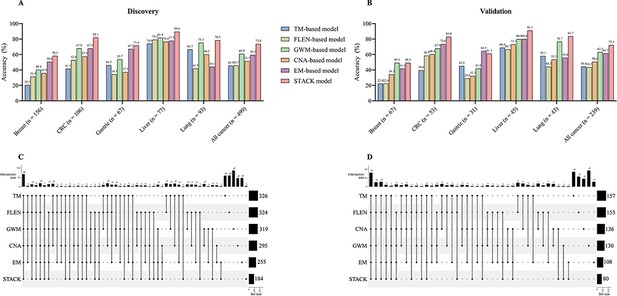
Comparison of accuracy for detecting five cancer types between single-feature model and stack model.
(A, B) The accuracy of single-feature models and stack model for detecting five cancer types in the discovery (A) and validation cohort (B). (C, D) The number of missed cases by the single-feature models and stack model in the discovery (C) and validation cohort (D).
Tables
Summary of clinical features of 738 cancer patients and 1550 healthy controls in discovery and validation cohorts.
Clinical features | Discovery cohort (N=1575) | Validation cohort (N=713) | |||||||||
---|---|---|---|---|---|---|---|---|---|---|---|
Cancer (N=499) | Healthy (N=1076) | p-Value (cancer vs healthy) | Cancer (N=239) | Healthy (N=474) | p-Value (cancer vs healthy) | ||||||
N | Percentage | N | Percentage | N | Percentage | N | Percentage | ||||
Gender | Female | 279 | 55.9% | 599 | 55.7% | 0.9281* | 126 | 52.72% | 270 | 56.1% | 0.2818* |
Male | 220 | 44.1% | 477 | 44.3% | 113 | 47.28% | 204 | 43.9% | |||
Age | Median | 58 | 47 | <0.0001† | 59 | 48 | <0.0001† | ||||
Min | 25 | 18 | 28 | 19 | |||||||
Max | 97 | 84 | 92 | 85 | |||||||
Stage | I | 52 | 10.4% | 23 | 9.6% | 0.4947* | |||||
II | 169 | 33.9% | 69 | 28.9% | |||||||
IIIA | 150 | 30.1% | 77 | 32.2% | |||||||
Non-metastasis with unknown staging information | 128 | 25.7% | 70 | 29.3% |
-
*
p-Values from Chi-square test.
-
†
p-Values from Mann-Whitney test.
Additional files
-
MDAR checklist
- https://cdn.elifesciences.org/articles/89083/elife-89083-mdarchecklist1-v1.docx
-
Supplementary file 1
Supplementary Tables.
Table S1. Detailed clinical information of all cancer and healthy subjects. Table S2. Summary of clinical information of patients with five cancer types. Table S3. Summary of sequencing quality metrics of samples in both cohorts. Table S4. List of 450 target regions. Table S5. List of significant pathways analyzed by g:Profiler. Table S6. List of 256 4-mer end motifs (EM). Table S7. Comparison of performance between single feature-based models and stacked model. Table S8. The sensitivity of SPOT-MAS (screening for the presence of tumor by methylation and size) model for detecting different cancer types and stages at a specificity of >95%. Table S9. The accurracy of random forest (RF), deep neural network (DNN), and graph convolutional neural network (GCNN) model for tissue of origin (TOO) identification. Table S10. List of significant features for TOO identification. Table S11. Overview of liquid biopsy assays for multi-cancer early detection described in recent publications.
- https://cdn.elifesciences.org/articles/89083/elife-89083-supp1-v1.xlsx