Disseminating cells in human oral tumours possess an EMT cancer stem cell marker profile that is predictive of metastasis in image-based machine learning
Figures
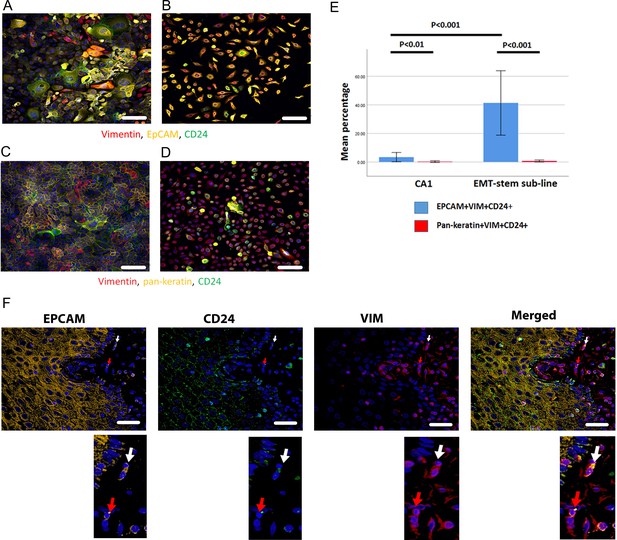
Immunofluorescent co-staining for EpCAM, Vimentin and CD24 identifies the EMT stem cell state.
(A–D) Immunofluorescent staining for EpCAM, Vimentin and CD24 (A, B) and pan-keratin, Vimentin and CD24 (C, D) in the CA1 cell line (A, C) and the EMT-stem CA1 sub-line (B, D). (E) Quantification of the percentage of EpCAM+Vim+CD24+ and pan-keratin+Vim+CD24+ cells in the CA1 cell line and EMT-stem sub-line. Significance is obtained from a two-tailed student t-test. The graph shows mean +/-95% confidence interval. n=3. (F) Detection of EpCAM+Vim+CD24+ cells in the stroma surrounding an oral cancer tumour specimen. The white arrow highlights an EpCAM+Vim+CD24+ cell in the stroma. The red arrow highlights an EpCAM+Vim+CD24- cell in the stroma. DAPI nuclear stain is blue. Below inset; enlargement of the highlighted cells for each marker. Scale bars = 100 µm.
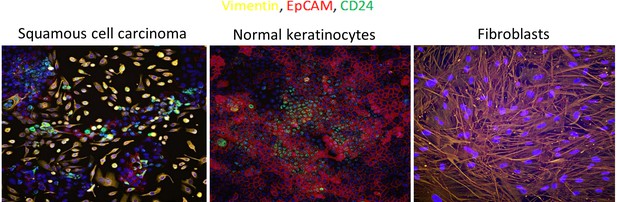
EpCAM, Vimentin and CD24 immunofluorescent staining in the CA1 OSCC cell line (left), normal keratinocytes (centre) and oral cancer associate fibroblasts (right).
Yellow = Vimentin, Red = EpCAM, Green = CD24.
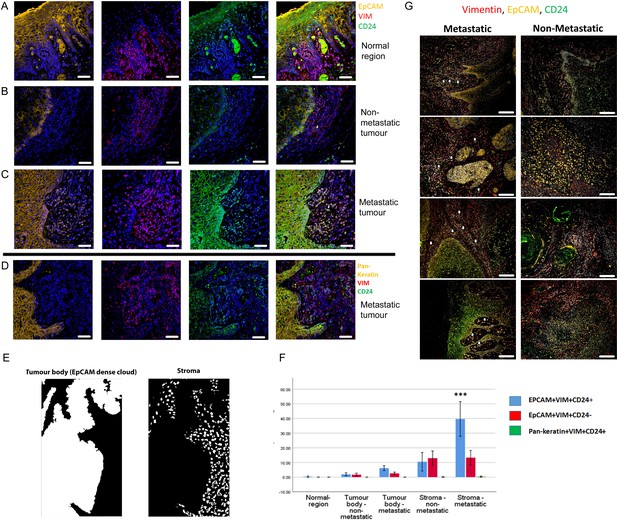
Enrichment of EpCAM+Vim+CD24+ cells in the stroma surrounding metastatic tumours.
(A–C) Immunofluorescent four-colour staining of oral tumour specimens for EpCAM (yellow), Vimentin (red) and CD24 (green) with DAPI nuclear stain (blue). Representative imaging fields from a normal epithelial region (A), a non-metastatic tumour (B) and a metastatic tumour (C). (D) Staining of a metastatic tumour for pan-keratin, Vimentin and CD24. (E) Image segmentation was performed, with generation of an ‘EpCAM dense cloud’ to distinguish the tumour body from the stroma. Grey level intensities for EpCAM, Vimentin and CD24 were obtained for every nucleated cell in each imaging field. (F) Quantification of the percentage of EpCAM+Vim+CD24+, EpCAM+Vim+CD24- and pan-keratin+Vim+CD24+ cells in normal region (epithelium distant from the tumour), tumour body, and stromal region from metastatic and non-metastatic tumours in the first cohort of specimens. A student t-test was performed comparing the mean percentage of EpCAM+Vim+CD24+ co-expressing cells in the metastatic stroma compared to the other fractions. *** signifies p<0.001. The graph shows mean +/-95% confidence interval. (G) Immunofluorescent four-colour staining of oral tumours from the second cohort of specimens, showing tumours with a range of invasive front presentations. White arrows highlight single EpCAM+Vim+CD24+ cells in the stroma. Scale bars = 100 µm.
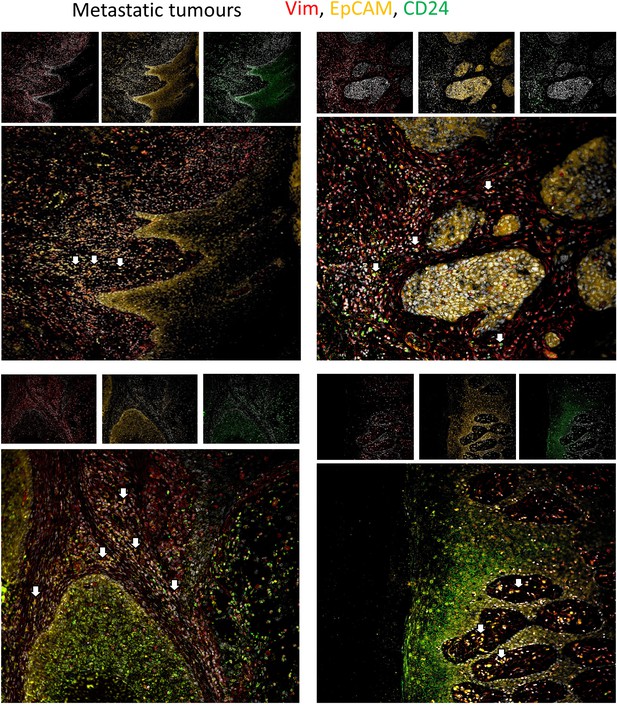
The metastatic tumour fields from Figure 2G, shown with separate channels at the top and the merge below.
The separate channels are Vimentin (left, red), EpCAM (centre, yellow) and CD24 (right, green). All include DAPI nuclear stain. In the merge, white arrows highlight individual EpCAM + Vim + CD24 + cells.

The non-metastatic tumour fields from Figure 2G, shown with separate channels at the top and the merge below.
The separate channels are Vimentin (left, red), EpCAM (centre, yellow), and CD24 (right, green). All include DAPI nuclear stain.

Enrichment of EpCAM+Vim+CD24+ cells in the stroma surrounding metastatic tumours in the second cohort of specimens.
(A) Tiling of a stained and imaged slide into 20 x fields of view and selection of a single field of view for segmentation. (B) Segmentation into single nucleated cells using DAPI staining. (C) Segmentation of cells in the stromal region using co-localisation of Vimentin and DAPI staining. (D) This segmentation pipeline separates tumour body from stroma in image analysis. (E) Cell identity measurements in the second cohort of specimens. Quantification of the percentage of EpCAM+Vim+CD24+ and EpCAM+Vim+CD24- cells in normal region (epithelium distant from the tumour), tumour body, and stromal region from metastatic and non-metastatic tumours. Recorded as the total percentage across the entire manually curated selection from the cohort (tumour-stroma interface and normal region fields of view), rather than the average percentage per field of view (as shown in Figure 2F for the first cohort).
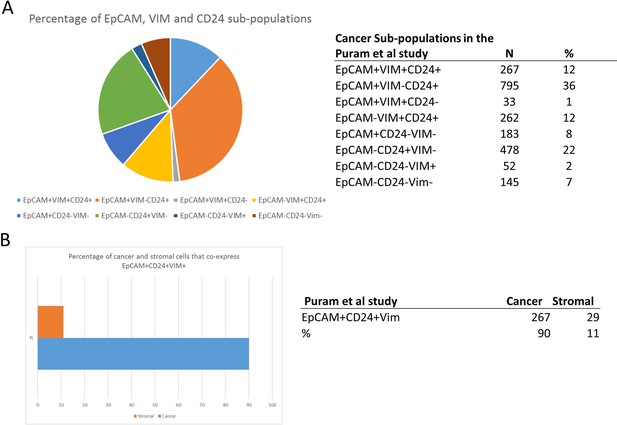
Analysis of EpCAM, CD24, and Vimentin expression in a published head and neck cancer scRNAseq dataset (Puram et al., 2017).
(A) Percentage of the cancer cells (excluding non-cancer cells) expressing each possible combination of EpCAM, CD24, and Vimentin, shown as a pie chart (left) and table (right). (B) The percentage of EpCAM+Vim+CD24+ cells within the whole dataset that are annotated as cancer (blue bar on bar chart) and non-cancer/stromal (orange bar on bar chart), shown as a bar chart (left) and table (right).
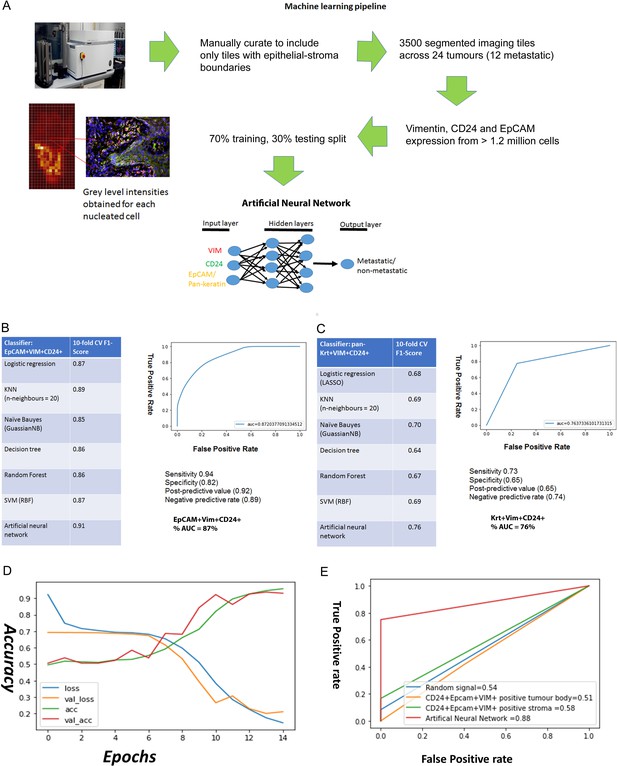
Predicting metastasis using EpCAM, Vimentin and CD24 immunofluorescent staining and a supervised machine learning approach.
(A) Pipeline for machine learning based on grey level intensities for the three markers in tumour cohort 1. The training tiles were classified as coming from a metastatic or non-metastatic tumour. (B, C) Performance of EpCAM, Vimentin and CD24 (B) and pan-keratin, Vimentin and CD24 (C) in the supervised learning task on tumour cohort 1. The tables show the 10-fold cross-validation F1 scores of different machine learning classification algorithms. To the right of each table is a receiver-of-operator curve (ROC) showing the area under the curve (AUC) of the artificial neural network (ANN) classifier. (D) Performance of EpCAM, Vimentin and CD24 in the supervised learning task on tumour cohort 2. An ANN classifier was trained and tested on cohort 2, independently of tumour cohort 1. Accuracy and loss scores are displayed for the training set (green and blue lines) and the validation set (red and yellow lines) drawn from within this cohort, for 14 training epochs on the ANN classifier. (E) ROCs comparing accuracy of the image-trained ANN (red line) with ANNs trained using the number of EpCAM+Vim+CD24+ cells for each field of view from the tumour stroma (green line) and tumour body (yellow line). Training with random gaussian signals provided a baseline (blue line).
Tables
Reagent type (species) or resource | Designation | Source or reference | Identifiers | Additional information |
---|---|---|---|---|
Cell line (Homo sapiens) | CA1 oral cancer line | Generated in house through explant culture of a human tumour specimen | ||
Cell line (Homo sapiens) | Cancer associated fibroblasts, primary culture | Generated in house through explant culture of a human tumour specimen | ||
Cell line (Homo sapiens) | N/TERT non-transformed epidermal keratinocyte cell line. hTERT immortalised | Smits et al., 2017 | ||
Biological sample (Homo sapiens) | FFPE blocks of human oral cancer specimens | Barts Health NHS Trust diagnostic archive | ||
Antibody | IgG2a mouse monoclonal CD24 antibody, clone ML5 | BD Bioscience | 1:100 | |
Antibody | IgG rabbit recombinant monoclonal EpCAM antibody | Abcam | EPR20532-225 | 1:100 |
Antibody | IgG1 mouse monoclonal Vimentin antibody, clone V9 | Dako | 1:100 | |
Antibody | IgG rabbit polyclonal wide spectrum cytokeratin antibody | Abcam | Ab9377 | 1:100 |
Antibody | Anti-mouse IgG2 Alexa Fluor 488 secondary antibody | Thermo Fisher | 1:500 | |
Antibody | Anti-rabbit IgG Alexa Fluor 555 secondary antibody | Thermo Fisher | 1:500 | |
Antibody | Anti-mouse IgG1 Alexa Fluor 647 secondary antibody | Thermo Fisher | 1:500 | |
Chemical compound, drug | DAPI nuclear dye, kept as a 1 mg/ml stock in DMSO | Sigma | 1:1000 | |
Software, algorithm | GE developer toolbox | GE | ||
Software, algorithm | Skikit-learn Python 3.6 libraries | Pedregosa et al., 2011 | ||
Software, algorithm | Tensorflow/Keras framework | https://www.tensorflow.org/api_docs/python/tf/keras/models |
Additional files
-
Supplementary file 1
Tumour characteristics for the 12 metastatic tumours (tumours 1–12) and 12 non-metastatic tumours (tumours 13–24) that were sectioned and stained for EpCAM, CD24 and Vimentin in the first cohort.
- https://cdn.elifesciences.org/articles/90298/elife-90298-supp1-v2.docx
-
Supplementary file 2
Clinical details and blinded analysis outcomes for second tumour cohort.
- https://cdn.elifesciences.org/articles/90298/elife-90298-supp2-v2.docx
-
MDAR checklist
- https://cdn.elifesciences.org/articles/90298/elife-90298-mdarchecklist1-v2.docx