Distinguishing mutants that resist drugs via different mechanisms by examining fitness tradeoffs
Figures
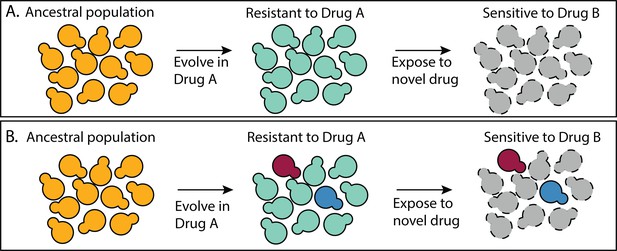
A multidrug treatment strategy that relies on all mutants having the same tradeoffs.
(A) All of the mutants that resist Drug A do so via a similar mechanism such that all are sensitive to Drug B. (B) There are multiple different types of mutants that resist Drug A, not all of which are sensitive to Drug B.
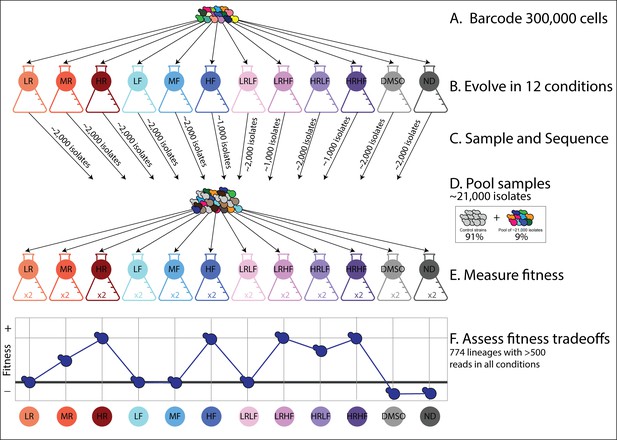
An overview of the experimental design.
(A) Yeast cells were barcoded to create 300,000 lineages. (B) These lineages were evolved in 12 different conditions (Table 1). (C) A small sample of evolved isolates were taken from each evolution experiment and their barcodes were sequenced. These ~21,000 isolates do not represent as many unique, adaptive lineages because many either have the same barcode or do not possess adaptive mutations. (D) These samples of evolved isolates were all pooled together with control strains representing the ancestral genotype. (E) Barcoded fitness competition experiments were then performed on this pool in each of the 12 evolution conditions. Fitness was measured by tracking changes in each barcode’s frequency over time relative to control strains. Two replicates per condition were performed. (F) The overall goal is to investigate fitness tradeoffs for hundreds of adaptive lineages. For example, the adaptive lineage depicted in dark blue has higher fitness than the ancestor in some environments (HR, HF) but lower fitness in others (DMSO, ND). We were able to investigate fitness tradeoffs for 774 adaptive lineages. We excluded lineages when we did not observe their associated barcode at least 500 times in all 12 environments. In other words, we only included lineages for which we obtained high-quality fitness estimates in all 12 environments.
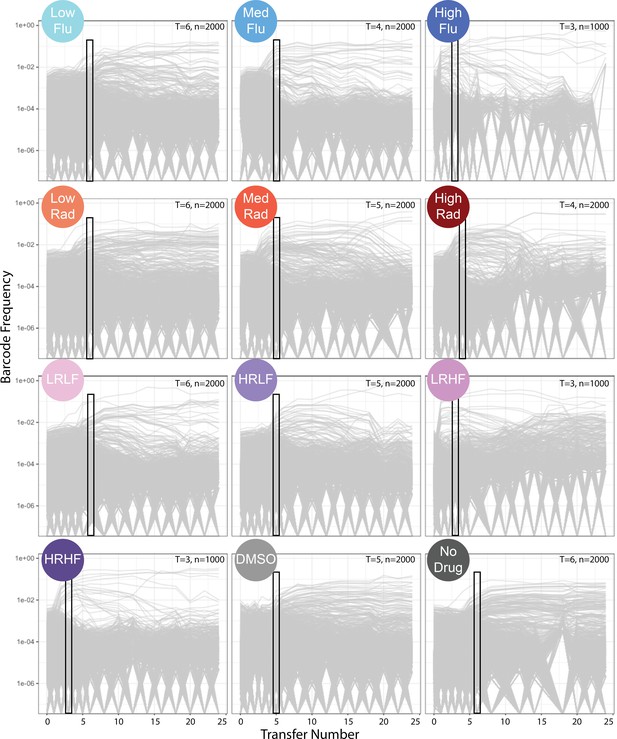
Twelve barcoded evolution experiments track ~300,000 lineages as they adapt to different drug concentrations and combinations.
In each plot, every line represents a unique barcoded lineage. The vertical axis represents barcode frequency. When lineages reach a frequency of zero it means they were not sampled at that time point of the experiment. The black boxes indicate the transfer number of each evolution experiment from which evolved lineages are sampled; the number of cells sampled (colonies picked) is in the upper right hand corner.
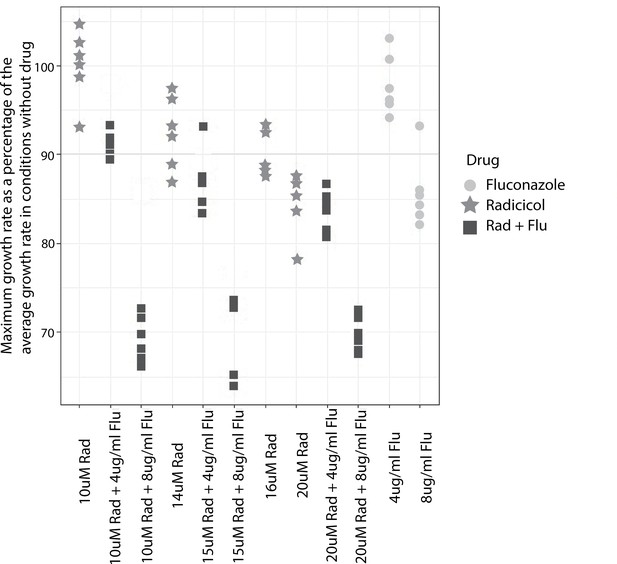
Chosen drug concentrations do not dramatically reduce yeast’s maximum growth rate.
We measured the growth rate of a single barcoded yeast lineage, prior to the evolution experiment, in different concentrations and combinations of drugs using a plate reader to track changes in optical density over time. Maximum growth rate is reported as a percentage of the maximum growth rate in conditions lacking FLU or RAD. Maximum growth rate was calculated as the maximum log-linear slope of the change in optical density over time.
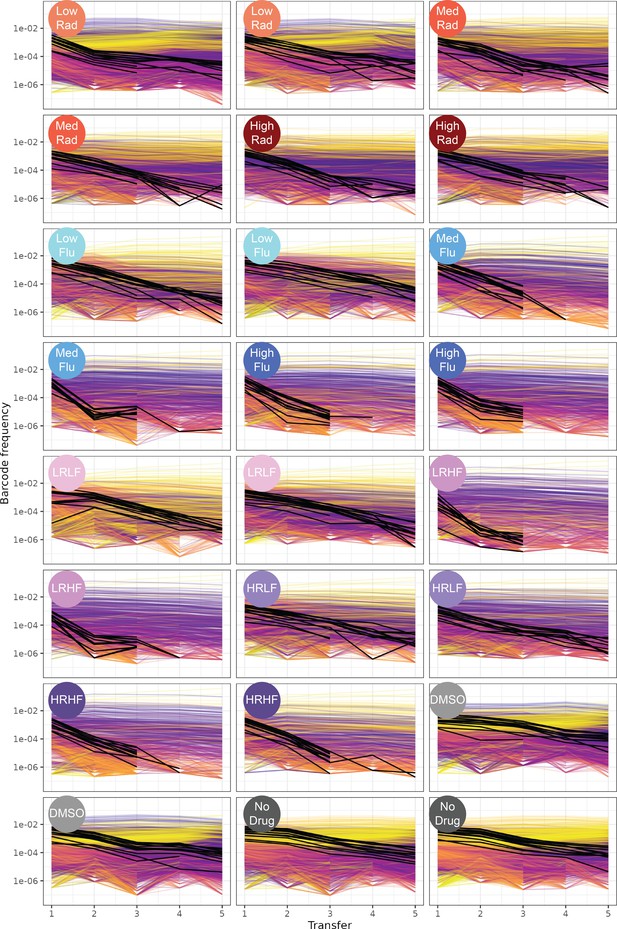
Twenty-four fitness competitions track evolved lineages as their barcodes change frequency.
In each plot, every line represents a barcoded lineage. Lineages with >5 reads per experiment are shown (4815 lineages shown). Control lineages, known to possess no fitness differences relative to the ancestor, are highlighted in black. Evolved lineages with higher fitness than controls are yellow, similar fitness to controls (i.e. relative fitness ~0) are purple, and lower fitness than controls are orange. When a lineage declines in frequency so much that its associated barcode is no longer observed, its line abruptly ends. Some lineages appear to decline and then increase in frequency; this happens because low-frequency lineages are subject to sampling noise.
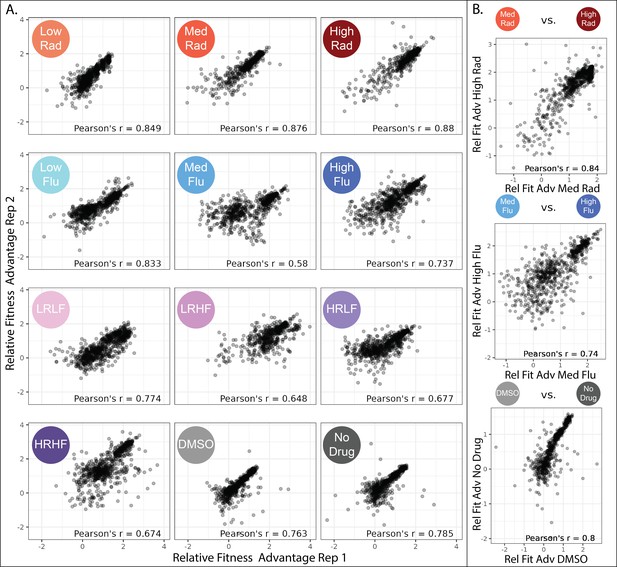
Fitness measurements are reproducible between replicates and closely related conditions.
(A) Every point depicts one of the evolved lineages that was observed more than 500 times in both replicates of the fitness competition experiment for a given condition. The average Pearson correlation across all pairs of replicates in all conditions is 0.75. (B) Every point depicts one of the 774 lineages that was observed more than 500 times in at least one replicate fitness competition. Here, instead of comparing the fitness of replicates, we compare fitness across conditions for three closely related pairs of conditions. Comparisons across conditions may be less noisy than those across replicates because fitness in a particular condition represents the average fitness across replicates in that condition.
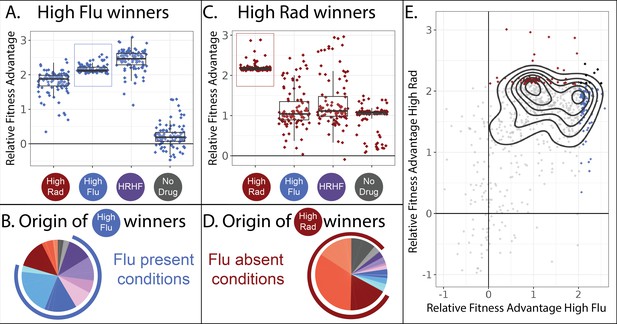
Two different classes of FLU-resistant mutants with unique tradeoffs.
(A) This panel describes the 100 mutant lineages with the highest fitness relative to the control strains in the high FLU environment (8 μg/ml FLU). The vertical axis depicts the fitnesses (log-linear slopes relative to control strains) for these 100 strains in four selected environments, including the high FLU environment (boxed). Boxplots summarize the distribution across all 100 lineages for each environment, displaying the median (center line), interquartile range (IQR) (upper and lower hinges), and highest value within 1.5 × IQR (whiskers). (B) The 100 lineages with highest fitness in high FLU were most often sampled from evolution experiments in which FLU was present. In this pie-chart, colors correspond to the evolution conditions listed in Table 1 and the blue outer ring highlights evolution conditions that contain FLU. The size of each slice of pie represents the relative frequency with which these 100 lineages were found in each evolution experiment. (C) Similar to panel A, this panel describes the 100 mutant lineages with the highest fitness relative to the control strains in the high RAD environment (20 µM Rad). (D) The 100 lineages with highest fitness in high RAD were most often sampled from evolution experiments that did not contain FLU. (E) A pairwise correlation plot showing that all 774 mutants, not just the two groups of 100 depicted in panels A and C, to some extent fall into two groups defined by their fitness in high FLU and high RAD. The contours (black curves) were generated using kernel density estimation with bins = 7. These contours describe the density of the underlying data, which is concentrated into two clusters defined by the two smallest black circles. The 100 mutants with highest fitness in high FLU are blue, highest fitness in high RAD are red, and the seven that overlap between the two aforementioned categories are black.
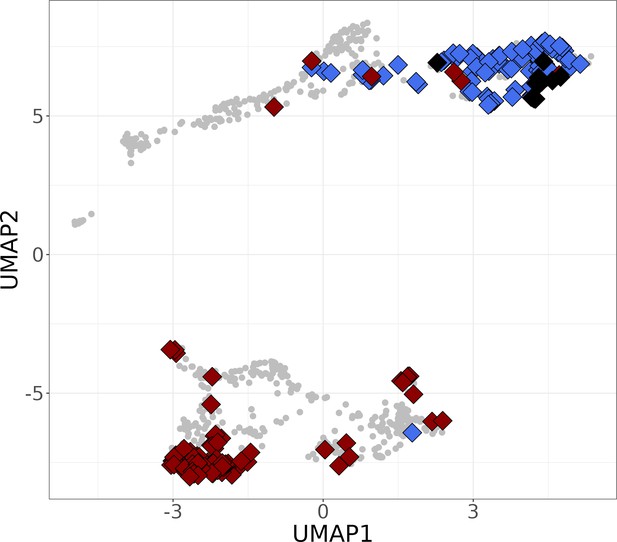
The two types of adaptive mutants depicted in Figure 3 sort into different clusters on the UMAP.
The top 100 highest fitness lineages in high FLU (blue) and high RAD (red) largely cluster into different groups, with the 7 overlapping mutants (black) falling into the uppermost group.
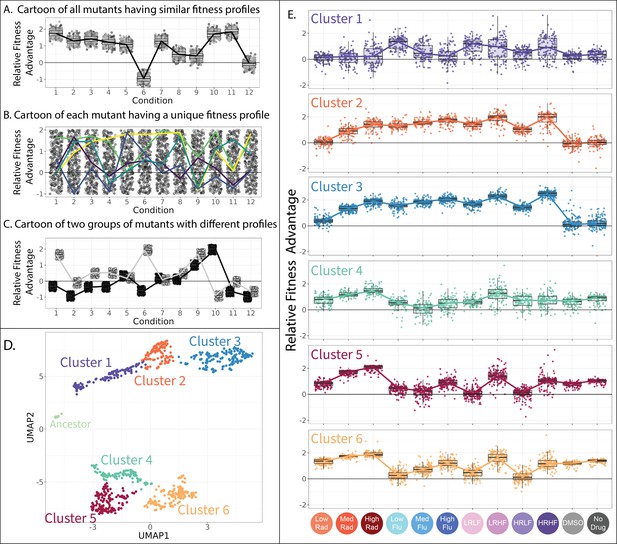
Clustering evolved lineages with similar fitness profiles.
(A–C) Simulated data showing potential fitness profiles when (A) all mutants have similar responses to environmental change and thus a similar fitness profile, (B) every mutant has a different profile (five unique profiles are highlighted in color), or (C) every mutant has one of a small number of unique profiles (two unique profiles are depicted). (D) Every point in this plot represents one of the barcoded lineages colored by cluster; clusters were identified using a gaussian mixture model. The 774 adaptive lineages cluster into 6 groups based on variation in their fitness profiles; the control lineages cluster separately into the leftmost cluster in light green. (E) The fitness profiles of each cluster of adaptive lineages. Boxplots summarize the distribution across all lineages within each cluster in each environment, displaying the median (center line), interquartile range (IQR) (upper and lower hinges), and highest value within 1.5×IQR (whiskers).
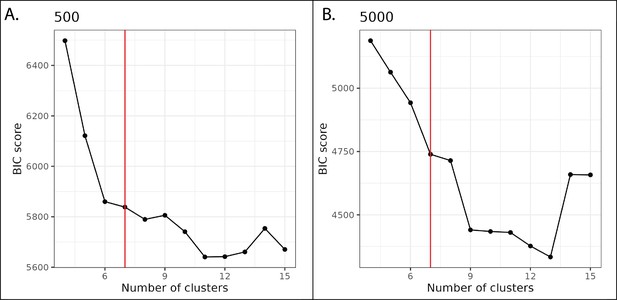
Bayesian information criteria (BIC) scores suggest the 774 mutants cluster into between 6 and 13 groups.
We used a gaussian mixture model to distinguish clusters of mutants with unique fitness profiles. (A) BIC scores for analysis performed on 774 mutant lineages that each were observed at minimum 500 times per environment. (B) BIC scores for analysis performed on 617 mutant lineages that each were observed at minimum 5000 times per environment.
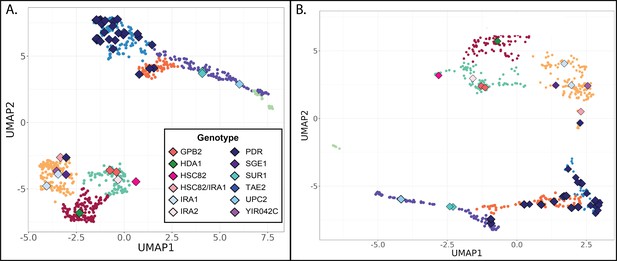
UMAP structure is robust.
(A) In this UMAP plot, relative fitness of the 774 lineages is fed directly into UMAP prior to normalizing the data to have equal variance across all environments as was done in Figure 4D. This has little impact on the appearance of the UMAP. (B) UMAP made with 617 lineages that were observed more than 5000 times in at least one replicate. While the appearance of the UMAP is inverted relative to Figure 4D, the clusters largely retain the same lineages. As lower coverage (noisier) data points are removed from this dataset, the observation that the overall patterns are maintained suggests our original clusters were not based on noisy fitness measurements.
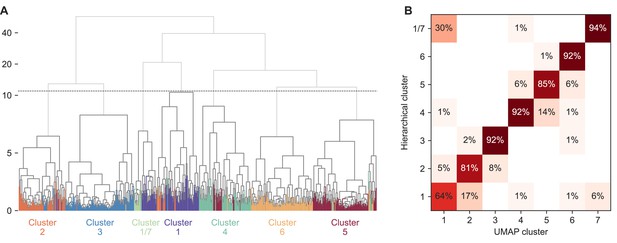
Clusters are robust to a hierarchical clustering method.
(A) In order to assess whether clusters identified from the UMAP are robust to alternative clustering methods, we used hierarchical clustering to identify clusters of mutants with similar fitness profiles. This figure depicts a dendrogram where the branch length between each lineage (or group of lineages) represents the distance between the clustered groups as measured across all measured conditions. The terminal branch leading to each lineage is colored by that lineage’s cluster from the UMAP clustering used in Figure 4 of the main text. Dark gray lines show clusters identified using a between-cluster cutoff of 11, which creates 7 clusters, consistent with the number of clusters we identified in Figure 4 via clustering on the UMAP space. Hierarchical clusters are labeled and colored corresponding to whichever of the UMAP clusters they share the most overlapping lineages with. Note that the y-axis is linearly scaled between 0 and 10 and log2 scaled above 10 for visualization purposes. (B) A matrix quantifying how well the results of this hierarchical clustering method correspond with those of the UMAP method presented in Figure 4, depicting the percentage of a given UMAP cluster that ends up with each corresponding hierarchical cluster. For the most part, mutants that are clustered together in the UMAP in Figure 4 are also clustered together in (A). Even though it appears that mutants in cluster 1 (which are those most resistant to low FLU) move to a different cluster, these mutants move together to the cluster pertaining to the control strains as they have the least severe effects on fitness.
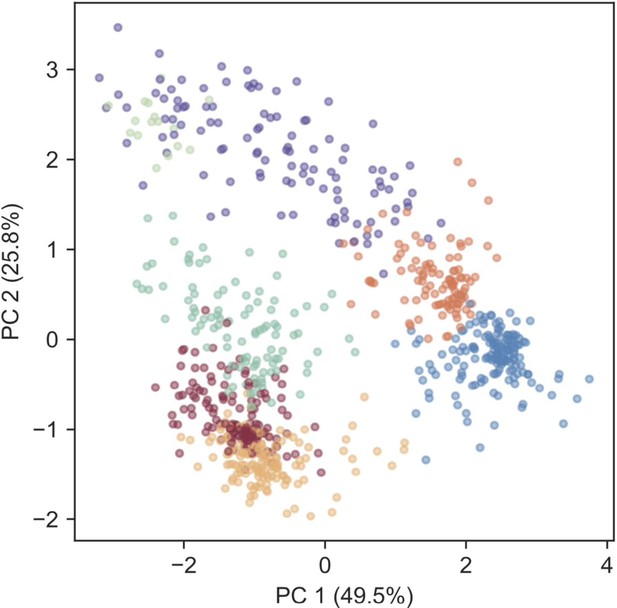
Clusters are robust to principal component analysis.
In order to assess whether clusters identified in the UMAP are robust to alternative clustering methods, we used principal component analysis and plotted PC1 vs PC2 to visualize mutants in two dimensional space. Points representing each mutant are colored corresponding to their cluster identity in Figure 4. The amount of variation captured by each component is stated in the axis label.
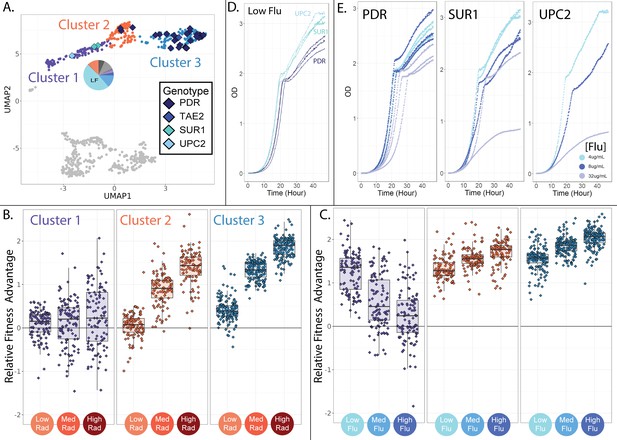
Evolved lineages comprising cluster 1 have different genotypes and phenotypes from neighboring clusters.
(A) The three clusters on the top half of the UMAP differ in their genetic targets of adaptation with cluster 1 being unique in that it does not contain mutations to PDR1 or PDR3. Cluster 1 is also unique in that it contains lineages that predominantly originated from the low fluconazole evolution condition; the pie chart depicts the fraction of lineages originating from each of the 12 evolution environments with colors corresponding to Table 1. (B) Evolved lineages comprising cluster 1 do not have consistent fitness advantages in conditions containing RAD, while lineages comprising clusters 2 and 3 are uniformly adaptive in medium and high RAD. Boxplots summarize the distribution across all lineages within each cluster in each environment, displaying the median (center line), interquartile range (IQR) (upper and lower hinges), and highest value within 1.5×IQR (whiskers). (C) Lineages comprising cluster 1 are most fit in low concentrations of FLU, and this advantage dwindles as the FLU concentration increases. Lineages comprising clusters 2 and 3 show the opposite trend. (D) In low FLU (4 μg/ml), Cluster 1 lineages (UPC2 and SUR1) grow faster and achieve higher density than lineages from cluster 3 (PDR). This is consistent with bar-seq measurements demonstrating that cluster 1 mutants have the highest fitness in low FLU. (D) Cluster 1 lineages are sensitive to increasing FLU concentrations (SUR1 and UPC2). This is apparent in that the dark blue (8 μg/ml flu) and grey (32 μg/ml flu) growth curves rise more slowly and reach lower density than the light blue curves (4 μg/ml flu). But this is not the case for the PDR mutants. These observations are consistent with the bar-seq fitness data (Figure 4E).
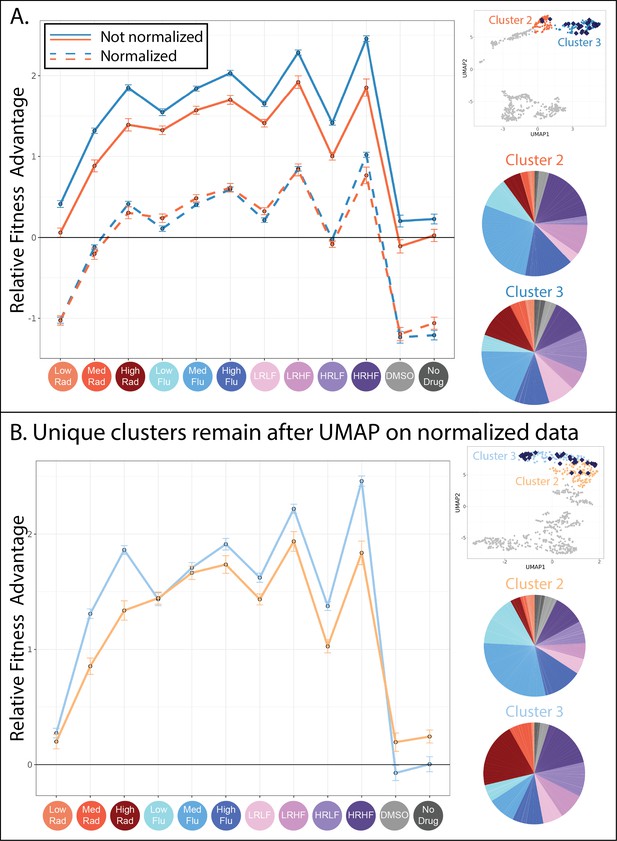
Evolved lineages in clusters 2 and 3 have characteristic differences despite similarities at the genetic level.
(A) This panel shows the similarities between clusters 2 and 3. The upper right inset displays the same UMAP from Figure 4D with only clusters 2 and 3 highlighted and with lineages possessing mutations to the PDR genes depicted as blue diamonds. The line plot displays the same fitness profiles for clusters 2 and 3 as Figure 4E, plotting the average fitness for each cluster in each environment and a 95% confidence interval. Dotted lines represent the same data, normalized such that every lineage has an average fitness of 0 across all environments. These line plots show that the fitness profiles for clusters 2 and 3 have a very similar shape. Pie charts display the relative frequency with which lineages in clusters 2 and 3 were sampled from each of the 12 evolution conditions, colors match those in the horizontal axis of the line plot and Table 1. (B) This panel shows the differences between the new clusters 2 and 3 created after all fitness profiles were normalized to eliminate magnitude differences. The upper right inset displays a new UMAP (also see Figure 6—figure supplement 1) that summarizes variation in fitness profiles after each profile was normalized by setting its average fitness to 0. The line plot displays the fitness profiles for the new clusters 2 and 3, which look different from those in panel A because 37% of mutants in the original clusters 2 and 3 switched identity from 2 to 3 or vice versa. The new clusters 2 and 3 are depicted in slightly different shades of blue and orange to reflect that these are not the same groupings as those depicted in Figure 4. Pie charts display the relative frequency with which lineages in new clusters 2 and 3 were sampled from each of the 12 evolution conditions, colors match those in the horizontal axis of the line plot and Table 1.
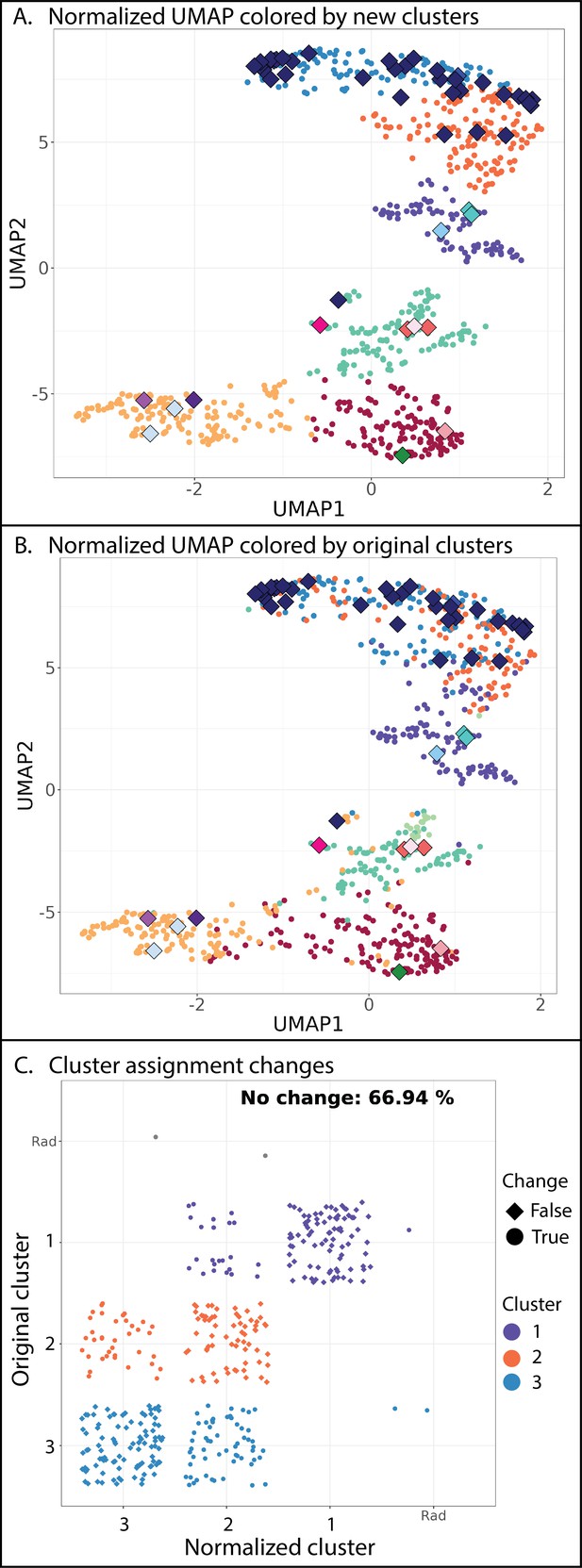
UMAP on data that were normalized to account for magnitude differences (row means set to 0).
(A) Every point in this plot represents one of the 774 barcoded lineages colored by cluster; clusters were identified using a gaussian mixture model. After normalizing to account for magnitude differences, the 774 adaptive lineages cluster into 6 (not 7) groups based on variation in their fitness profiles. The number of clusters is reduced relative to the UMAP in Figure 4 because the control lineages no longer form a separate group; they cluster into the middle cluster in light green. (B) This plot shows the same UMAP as above in A, but the color of each of the 774 points is changed to reflect its color in the UMAP in Figure 4. Despite coloring based on a different UMAP, differently colored points still largely fall into separate clusters, except for those points colored blue or orange, which are discussed in Figure 6. (C) This plot depicts all lineages in clusters 1–3 in either the Figure 4 UMAP (not normalized to lessen magnitude differences) or the above UMAP (normalized to lessen magnitude differences). Lineages that do not switch from one cluster to another are represented by diamonds while those that switch are noted with circles. Across these three clusters, 67% of lineages remain in their original cluster.
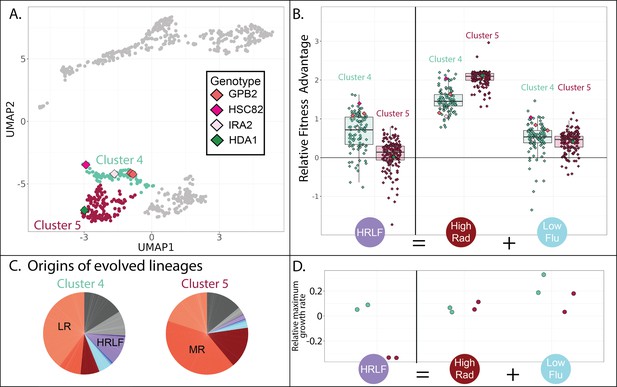
Evolved lineages in cluster 4 and 5 differ in response to combined drugs.
(A) Adjacent clusters 4 and 5 each contain a small number of sequenced isolates depicted as diamonds; diamond colors correspond to the genes containing adaptive mutations in each sequenced isolate. (B) Cluster 5 (red) has an unexpected fitness disadvantage in the HRLF multidrug environment relative to cluster 4 (green), given that cluster 5 lineages do not have a fitness disadvantage in the relevant single drug environments. Boxplots summarize the distribution across all lineages within each cluster in each environment, displaying the median (center line), interquartile range (IQR) (upper and lower hinges), and highest value within 1.5 × IQR (whiskers). (C) Pie charts display the relative frequency with which lineages in each cluster were sampled from each of the 12 evolution conditions, colors match those in Table 1. (D) The maximum exponential growth rate for a single lineage isolated from each of clusters 4 (green) and 5 (red), relative to the ancestor. The growth rate of each lineage in each condition was measured twice by measuring changes in optical density over time. Tested lineage from cluster 4 (in green) has a mutation to GBP2 (S317T) while the lineage from cluster 5 (in red) has mutation to HDA1 (S600S).
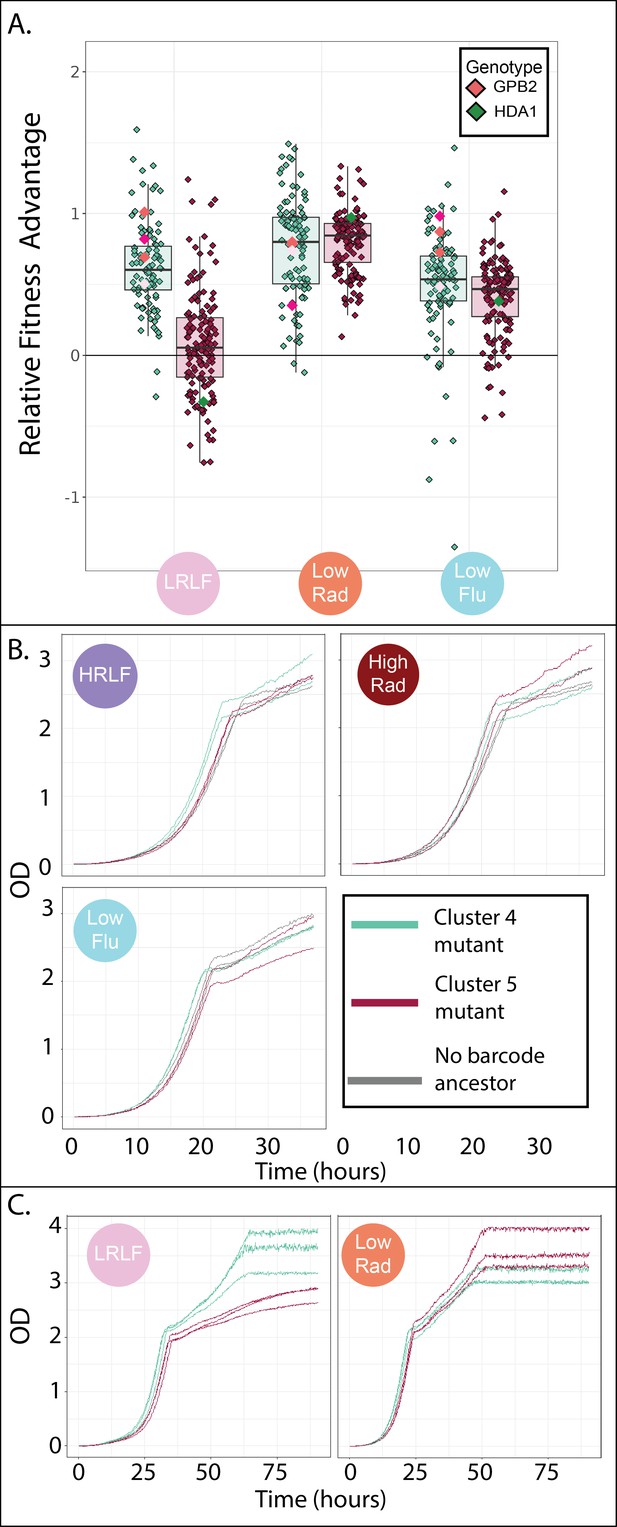
Unexpected tradeoffs in evolved lineages in cluster 4 and 5 in response to combined drugs.
(A) Cluster 5 (red) has an unexpected fitness disadvantage in the LRLF multidrug environment relative to cluster 4 (green), given that cluster 5 lineages do not have a relative fitness disadvantage in the relevant single drug environments. This plot is similar to the one in Figure 7B except here the multidrug condition shown is LRLF rather than HRLF. Boxplots summarize the distribution across all lineages within each cluster in each environment, displaying the median (center line), interquartile range (IQR) (upper and lower hinges), and highest value within 1.5×IQR (whiskers). (B) Raw growth curve data from which relative maximum growth rates presented in Figure 7D were calculated. Two mutants, one to GBP2 (cluster 4; green) and one to HDA1 (cluster 5; red) were each grown individually in the conditions shown, as was the barcodeless ancestor strain (grey). All experiments were conducted in duplicate. We initiated all cultures from the same number of starting cells. The mutant in cluster 5 (red) has an obvious growth disadvantage in the combination condition (HRLF). (C) Raw growth curve data for the same two mutants in B in different conditions. These growth experiments were conducted similarly to those in B, except they were tracked for a longer period of time, lacked the ancestor strain, and involved three replicates per mutant. There appear to be obvious and reproducible differences in the growth curves of the two mutants surveyed.
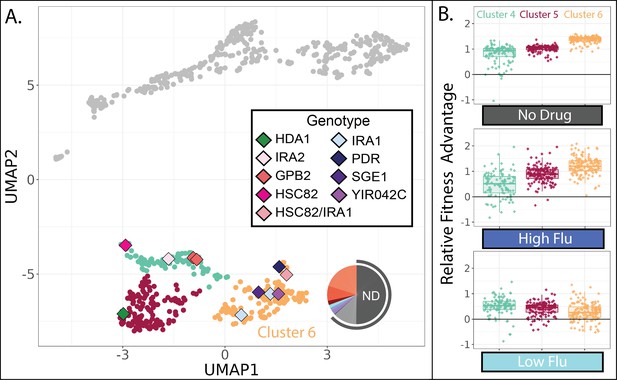
Evolved lineages in cluster 6 have higher fitness than other lineages in the absence of FLU and RAD.
(A) Same UMAP as Figure 4D with clusters 4, 5, and 6 highlighted and sequenced isolates in these clusters represented as diamonds. Diamond colors correspond to the targets of adaptation in the sequenced isolates. Pie charts display the relative frequency with which lineages in cluster 6 were sampled from each of the 12 evolution conditions; colors match those in Table 1. Grey outline depicts conditions lacking RAD and FLU. (B) Of the three clusters on the bottom half of the UMAP, cluster 6 lineages perform best in conditions without any drug and in the highest concentration of FLU. Yet they perform worst in the lowest concentration of FLU. Boxplots summarize the distribution across all lineages within each cluster in each environment, displaying the median (center line), interquartile range (IQR) (upper and lower hinges), and highest value within 1.5×IQR (whiskers).
Tables
A list of the environments included in this study and the symbol used to represent them in 242 subsequent figures.
Evolution Condition | Abbreviation | Symbol |
---|---|---|
Fluconazole | Low Flu | ![]() |
Fluconazole | Med Flu | ![]() |
Fluconazole | High Flu | ![]() |
Radicicol | Low Rad | ![]() |
Radicicol | Med Rad | ![]() |
Radicicol | High Rad | ![]() |
Radicicol Fluconazole | LRLF | ![]() |
Radicicol Fluconazole | LRHF | ![]() |
Radicicol Fluconazole | HRLF | ![]() |
Radicicol Fluconazole | HRHF | ![]() |
DMSO | DMSO | ![]() |
No Drug | No Drug | ![]() |
Additional files
-
Supplementary file 1
The sequenced mutations present in each of the adaptive lineages on which we performed whole genome sequencing.
- https://cdn.elifesciences.org/articles/94144/elife-94144-supp1-v1.xlsx
-
Supplementary file 2
Estimated percentage of diploid cells in each evolution condition at the time point we sampled, determined using nuclear staining and flow cytometry as described in the Methods.
*For the High Radicicol +Low Fluconazole condition, 95 instead of 96 samples were measured due to undetectable growth in one well.
- https://cdn.elifesciences.org/articles/94144/elife-94144-supp2-v1.xlsx
-
MDAR checklist
- https://cdn.elifesciences.org/articles/94144/elife-94144-mdarchecklist1-v1.docx