A metabolic modeling-based framework for predicting trophic dependencies in native rhizobiomes of crop plants
Figures
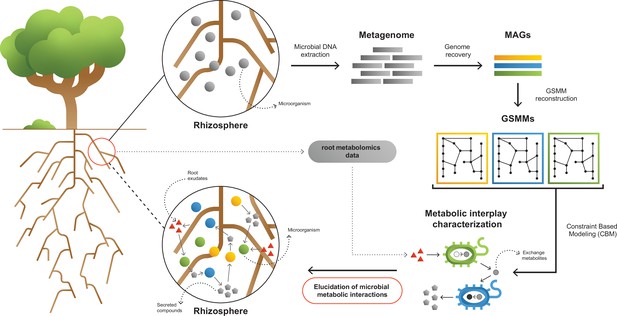
A framework for interpreting and characterizing the network of metabolic interactions of root-associated bacteria.
Going from obscure rhizosphere (upper circle) to the elucidated rhizosphere (lower circle): Microbial genomic DNA extracted from rhizosphere soil serves the construction of a rhizosphere-derived metagenome and the recovery of rhizosphere community metagenome assembled genomes (MAGs). For each MAG, a genome scale metabolic model (GSMM) is built, representing a specific species in the microbial community. Apple-root exudate profiles based on metabolomics data are used for the construction of a simulation environment. Via constraint-based modeling (CBM), applied on GSMMs, interactions are characterized in the simulated root environment, yielding predictive information regarding potential trophic exchanges within the native rhizosphere microbial community.
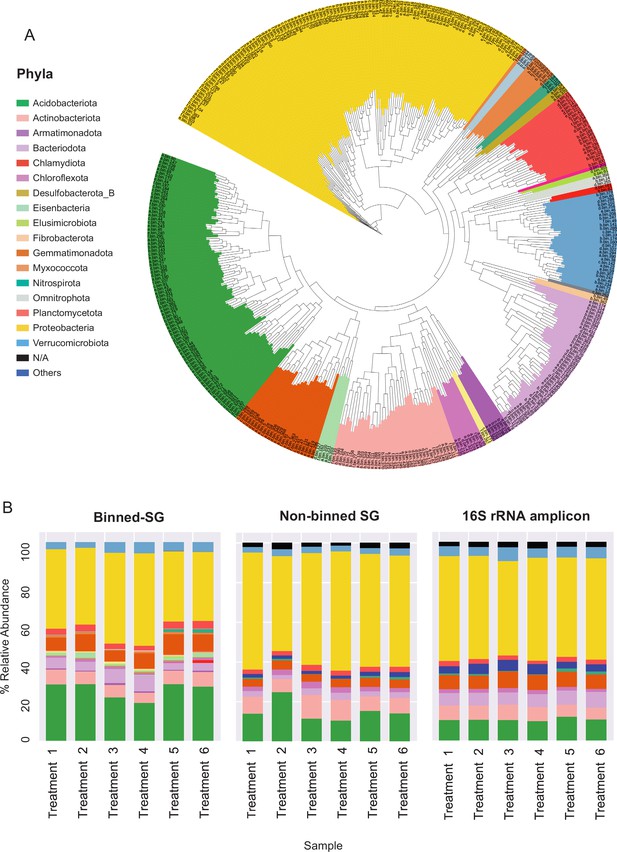
Phylogenomic surveys of the genomic collection assembled from sequence data derived from apple rhizosphere samples.
(A) A phylogenomic tree constructed from 395 de-replicated metagenome assembled genomes (MAGs) extracted from the metagenomes. The tree is based on concatenated marker proteins according to GTDB-Tk (Chaumeil et al., 2019). (B) Relative abundance of bacterial taxa at the phylum level as inferred from different phylogenomic classification approaches applied for the same samples: Binned-SG (MAGs derived from shotgun metagenomics); non-binned SG (contigs derived from shotgun metagenomics); 16S rRNA amplicon derived from the same samples. Taxonomic classifications of rRNA amplicon data and non-binned-SG sequences are taken from Somera et al., 2021 and Berihu et al., 2023, respectively. Shotgun metagenomics data from Berihu et. al was used here for construction of MAGs catalogue. ‘Treatments 1–6’ relate to six specific combinations of rootstock and soil amendment as detailed in Figure 2—source data 2. Relative abundance was calculated as the average value in five replicates conducted for each treatment.
-
Figure 2—source data 1
Genomic characteristics of metagenome assembled genomes (MAGs).
- https://cdn.elifesciences.org/articles/94558/elife-94558-fig2-data1-v1.xlsx
-
Figure 2—source data 2
Summary table of the mapping of reads to metagenome assembled genomes (MAGs) across treatments.
Treatment types are fully described in Berihu et al., 2023.
- https://cdn.elifesciences.org/articles/94558/elife-94558-fig2-data2-v1.xlsx
-
Figure 2—source data 3
Pathway completion analysis (Kyoto Encyclopedia of Genes and Genomes [KEGG] pathway decoder) of metagenome assembled genomes (MAGs).
- https://cdn.elifesciences.org/articles/94558/elife-94558-fig2-data3-v1.xlsx
-
Figure 2—source data 4
Genome scale metabolic model (GSMM) MEMOTE tests results.
- https://cdn.elifesciences.org/articles/94558/elife-94558-fig2-data4-v1.xlsx
-
Figure 2—source data 5
Genome scale metabolic model (GSMM) attributes.
- https://cdn.elifesciences.org/articles/94558/elife-94558-fig2-data5-v1.xlsx
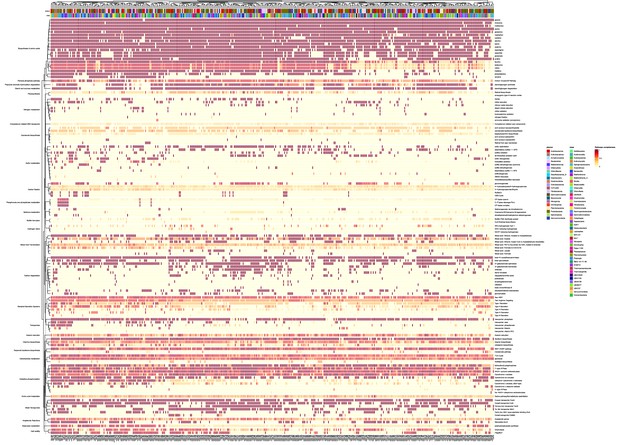
Metabolic pathway completion analysis of community de-replicated metagenome assembled genomes (MAGs).
The presented cluster map links the MAGs with different metabolic pathways based on completion values of a specific metabolic pathway. Values are concluded based on the ratio between the count of EC numbers present in an MAG, which correspond to a specific pathway (derived from annotated genes), and the total EC numbers in that specific KEGG pathway. Left axis represents the KEGG KO of a set of specific functions, which are indicated on the right axis. Top and bottom color bars on the upper axis represent the taxonomic annotation of the MAGs (phylum and class, respectively), which are indicated on the bottom axis.
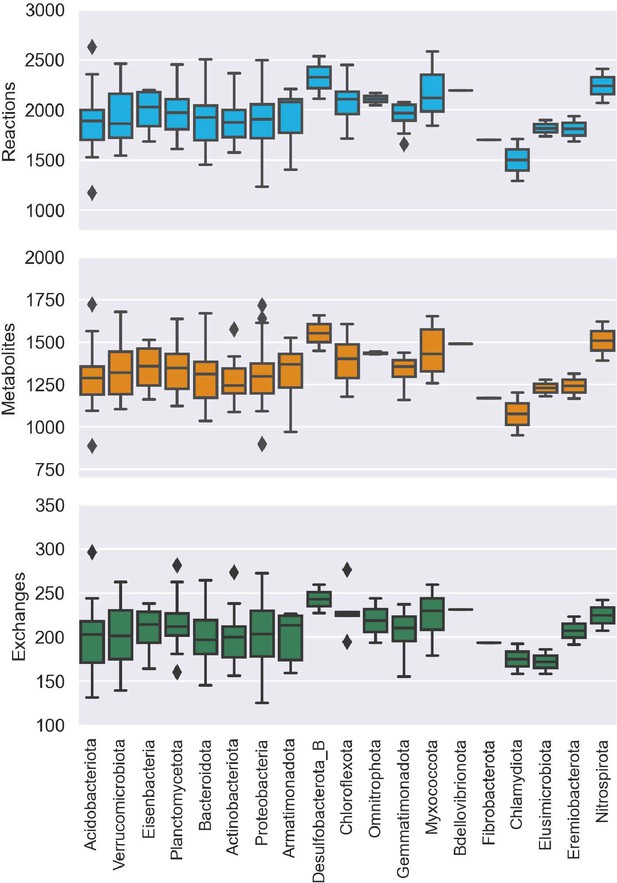
Distribution of genome scale metabolic model (GSMM) attributes at the phylum level.
Subplots of reactions, metabolites, and exchanges representing the distribution of the respective attributes for each group of bacteria, affiliated with a specific phylum.
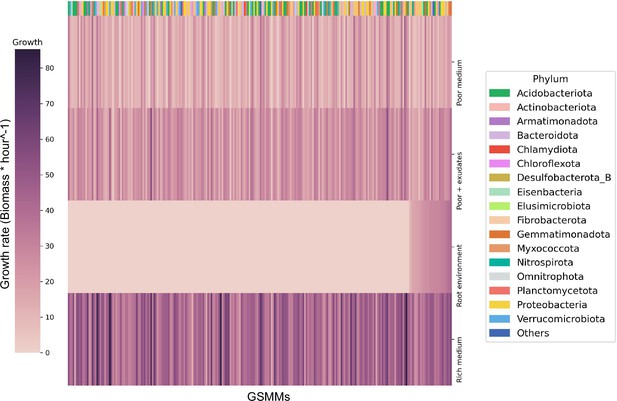
Bacterial growth rates in different environments.
Each row represents the growth rate (biomass increase * hour−1) of species in a specialized medium; from top to bottom: poor medium, poor medium supplemented with root exudates, root environment medium, and rich medium. Each column represents a genome scale metabolic model (GSMM). Models are sorted by their growth score in the root environment medium. Poor, rich, and roor + exudates media are model specific, thus sustain growth of all models. The horizontal color bar on top of the plot represents the phyla of the corresponding GSMM. Actual growth values are provided in Figure 3—source data 1.
-
Figure 3—source data 1
Genome scale metabolic model (GSMM) growths in different environments.
- https://cdn.elifesciences.org/articles/94558/elife-94558-fig3-data1-v1.xlsx
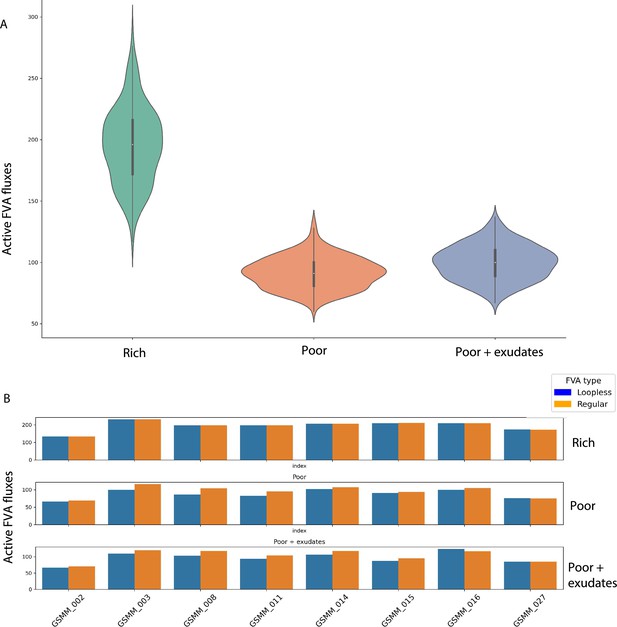
Flux Variability Analysis (FVA) performances of genome scale metabolic models (GSMMs) in different environments (Figure 3—source data 1).
(A) Distribution of potentially active exchange reactions (non-zero minimum FVA flux) in the different environments. Solid line inside each violin indicates the interquartile range (IQR). White point in IQR indicates the median value. Whiskers extending from the IQR indicate the range within 1.5 times the IQR from the quartiles. Violin width at a given value represents the density of data points at that value. (B) Loopless FVA scores compared to regular FVA for models in the three model-specific environments (Figure 3; poor medium, poor medium supplemented with root exudates, and rich). Bars indicate the count of active fluxes (non-zero minimum FVA flux). Only a subset of models was used for this analysis.
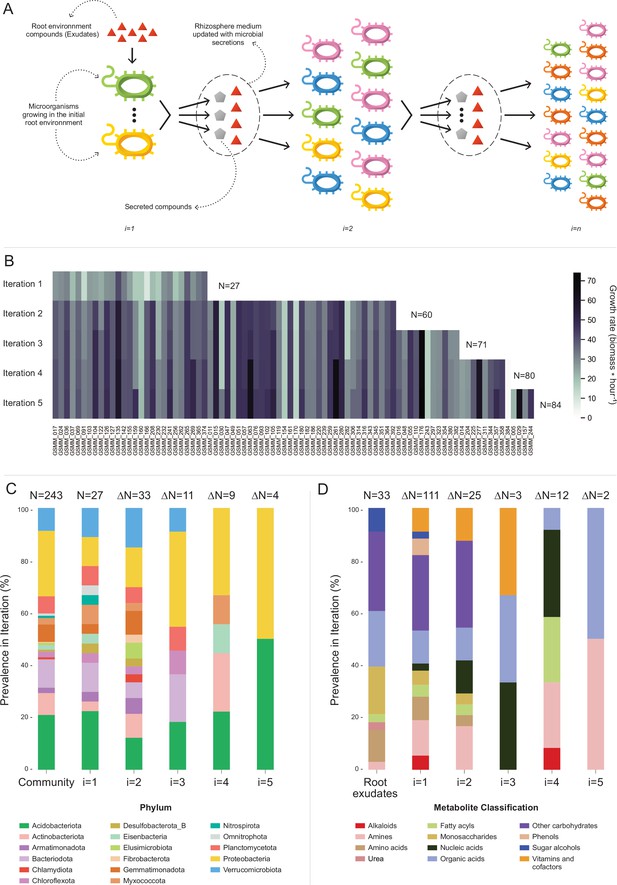
Microbial community succession module (MCSM), and characterization of trophic dynamics in the community along iterations.
(A) An illustration of the iterative microbial community growth module, representing the growth of community members along iterations starting in the ‘Rhizosphere environment’ and updated with microbial secretion outputs. (B) Growth rate characterization of genome scale metabolic models (GSMMs) in the community along iterations. Each column in the heat-map represents a different GSMM (only models which have grown in the rhizosphere environment are presented); growth rate is indicated by the color bar. Iterations are represented by rows. Blank spaces indicate models not growing at that specific iteration. N is the total number of species that grew after each iteration. (C) Distribution of GSMMs growing along iterations at the phylum level. (D) Distribution of organic compounds secreted along iterations, classified into biochemical groups. Root exudates bar (far left) represents the classification of organic compounds in the initial ‘Rhizosphere environment’. Numbers on top of bars in both C and D (designated by N) denote the number of new entries in a specific iteration, with respect to the previous iteration.
-
Figure 4—source data 1
Table of compounds used throughout iterations in the microbial community succession module (MCSM).
- https://cdn.elifesciences.org/articles/94558/elife-94558-fig4-data1-v1.xlsx
-
Figure 4—source data 2
Genome scale metabolic model (GSMM) secretion data derived from microbial community succession module (MCSM).
- https://cdn.elifesciences.org/articles/94558/elife-94558-fig4-data2-v1.xlsx
-
Figure 4—source data 3
Initial medium compounds used in microbial community succession module (MCSM) cellulose degradation process.
- https://cdn.elifesciences.org/articles/94558/elife-94558-fig4-data3-v1.xlsx
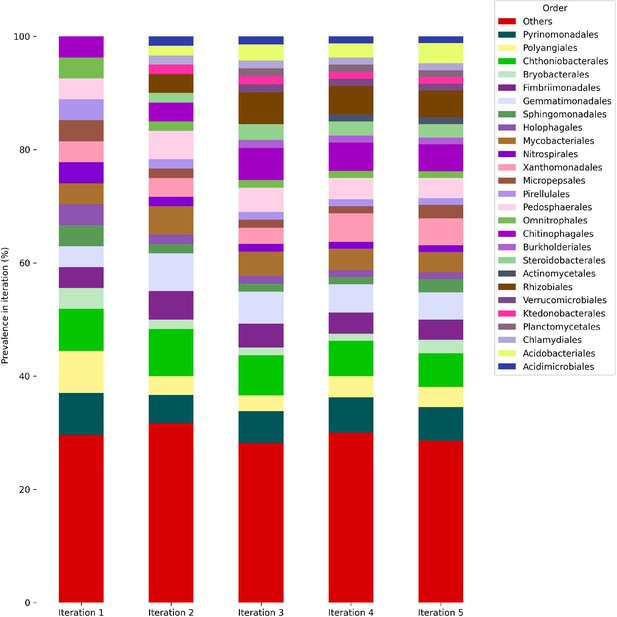
Phylogenetic distribution of models growing along iterations at the order level.
Bars represent the taxonomic distribution of the community (i.e., overall models that grew along iterations, with respect to the corresponding iteration). Colors are indicative of different orders.
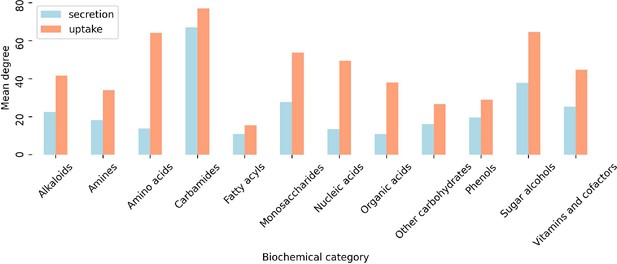
Uptake and secretion degrees of biochemically classified metabolite nodes, as inferred from the communal interaction network.
Values indicate mean amount of links between community genome scale metabolic models (GSMMs) and nodes classified to the corresponding category. Biochemical classification was conducted both based on BRITE database and manually.
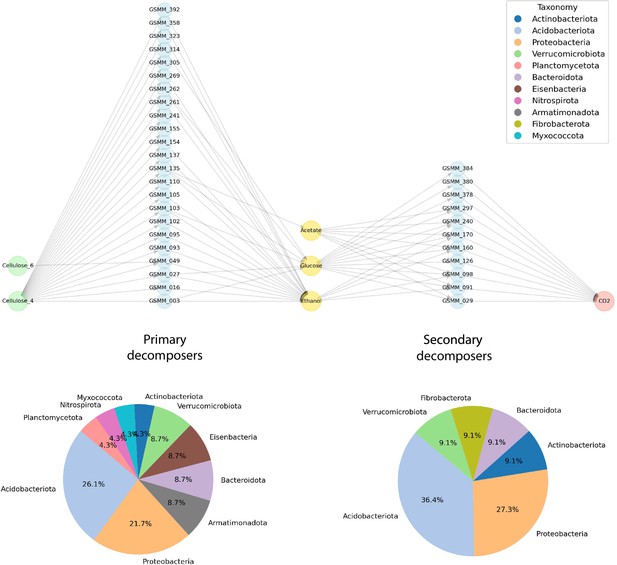
Application of microbial community succession module (MCSM) over the process of cellulose decomposition as described by Kato et al., 2005.
Five-partite network exhibiting the uptake of cellulose oligomers (4 and 6 units of connected D-glucose) by primary decomposers, through secretion of intermediate compounds and their metabolization by secondary decomposers to CO2. Distribution of phyla of primary and secondary decomposers is denoted by pie charts. Though metagenome assembled genomes (MAGs) were not constructed for the original species as in Kato et al., among the primary consumers, species corresponding to the Acidobacteria (Acidobacteriales) (Kulichevskaya, 2012), Actinobacteria (McCarthy, 1987), Bacteriodetes (Thomas et al., 2011), Proteobacteria (Xanthomonadales) (Dow and Daniels, 1994), and Verrucobacteria (Bergmann et al., 2011) groups are found to be capable of degrading cellulose compounds via enzymatic mechanisms.
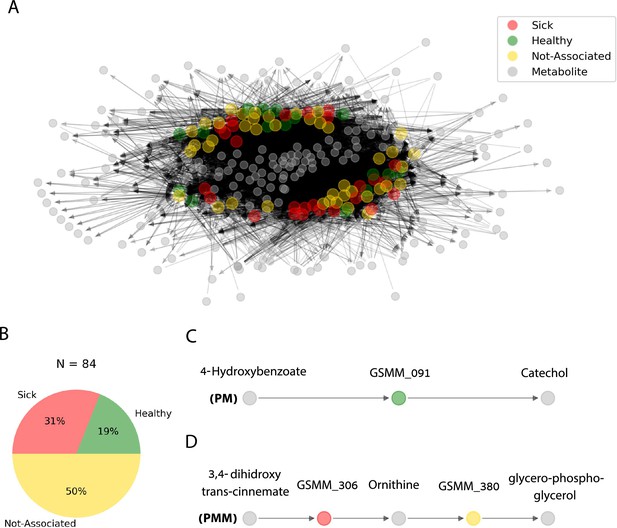
Trophic interactions based on community exchange fluxes predicted along iterations in the simulated rhizosphere environment.
(A) Network representation of potential metabolite exchanges between rhizosphere community members. Edges in the network are directional; arrowhead from a gray node (metabolite) pointing toward a colorful node (genome scale metabolic model, GSMM) indicates uptake; arrowhead from a colorful node (GSMM) pointing toward a gray node (metabolite) indicates secretion. Node colors correspond to differential abundance classification of GSMMs in the different plots: healthy, sick, and not-associated. Metabolites found in the center of the network are of a higher connectivity degree (i.e., are involved in more exchanges). Only organic compounds are included in the network. Gray rectangles illustrate a zoom-in to specific five- and three-partite sub-networks (C, D). (B) Pie chart distribution of GSMMs classified according to differential abundance of reads mapped to the respective metagenome assembled genomes (MAGs); N is the total number of GSMMs in the network. (C, D) Examples of specific sub-network motifs derived from the community network; plant–microbe (PM; a three component sub-network motif, upper); plant–microbe–microbe (PMM; a five component sub-network motif, lower), respectively. The full list of PM and PMM sub-network motifs is found in Figure 6—source data 1.
-
Figure 5—source data 1
Differential abundance scores of genome scale metabolic models (GSMMs) (metagenome assembled genomes, MAGs).
- https://cdn.elifesciences.org/articles/94558/elife-94558-fig5-data1-v1.xlsx
-
Figure 5—source data 2
Trophic network information, nodes degree.
- https://cdn.elifesciences.org/articles/94558/elife-94558-fig5-data2-v1.xlsx
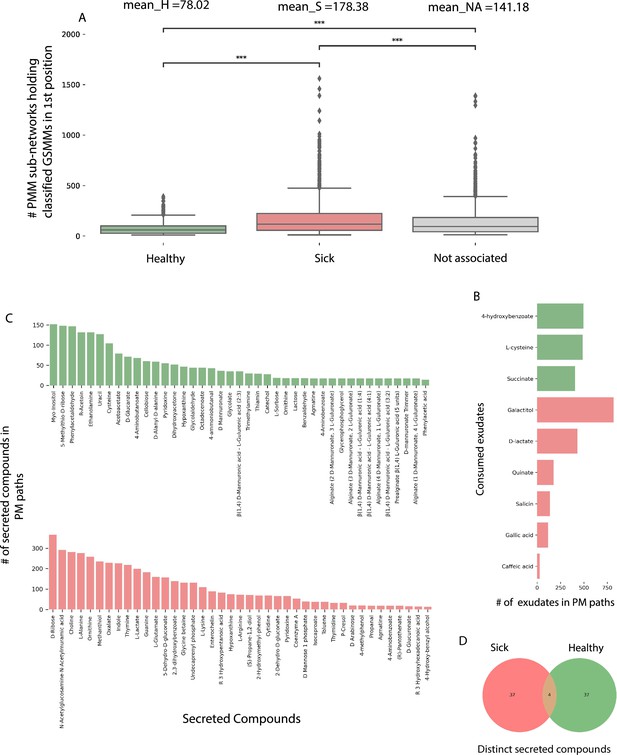
Characterization of plant–microbe (PM) and plant–microbe–microbe (PMM) sub-network motifs’ features associated with differentially abundant (DA) genome scale metabolic models (GSMMs).
(A) Count distribution of PMM sub-networks determined by GSMMs in the first microbial position classified as either healthy, sick, or not-associated, corresponding to the mean values H, S, and NA, respectively. Boxes extend from first quantile to the third quantile, middle line represents the median; dots outside whiskers indicate outliers. Distinction of groups was determined using ANOVA followed by the Tukey post hoc test. Asterisks indicate significance of test (***≤0.005) (B) Bar plot indicating the number of exudates significantly associated with H- or S-classified PM sub-networks (hypergeometric test; False Discovery Rate (FDR) ≤0.05; green: healthy – H, red: sick – S). (C) Bar plots indicate the number of secreted compounds in PM sub-networks, which are significantly associated with H-classified (upper, colored green) or S-classified (lower, colored red) (hypergeometric test; FDR ≤0.05). (D) Venn diagram represents the intersection of secreted compounds derived from both Sick and Healthy classified PM sub-networks.
-
Figure 6—source data 1
Sub-networks motifs data.
- https://cdn.elifesciences.org/articles/94558/elife-94558-fig6-data1-v1.xlsx
-
Figure 6—source data 2
Plant–microbe (PM) sub-networks functional characterization and statistical tests results.
- https://cdn.elifesciences.org/articles/94558/elife-94558-fig6-data2-v1.xlsx
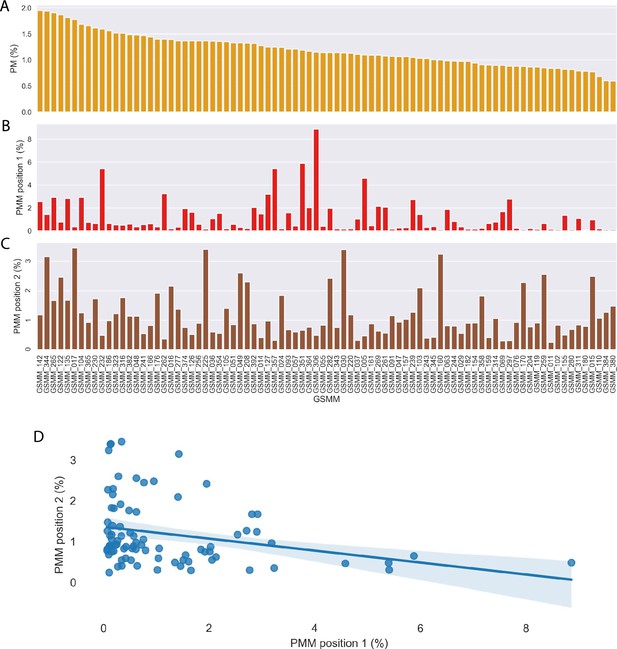
Community genome scale metabolic model (GSMM)-centric characterization of plant–microbe (PM) and plant–microbe–microbe (PMM) motifs.
(A–C) Frequency of community GSMMs in PM, PMM (first, second position) in sub-network motifs, respectively. (D) Correlation of GSMMs position frequency in PMM sub-networks; Pearson, p-value = 0.009.
Tables
Apple-root exudates adopted from the works of Leisso et al., 2017; Leisso et al., 2018, included in the rhizosphere environment.
Category | Compounds |
---|---|
Amino acids | L-Asparagine, L-aspartate, L-cysteine, L-valine, beta-alanine |
Monosaccharides | Rhamnose, glycerate, ribose, galactose, xylose, erythrose |
Sugar-alcohols | Sorbitol, galactitol, glycerol |
Other carbohydrates | 3,4-Dihydroxybenzoate, 3,4-dihydroxy-trans-cinnamate, 4-hydroxybenzoate, benzoate, esculin, ferulate, gallic acid, quinate, salicin, trans-cinnamate |
Organic acids | D-Galacturonate, D-lactate, D-malate, malonate, oxalate, pyruvate, succinate |
Carbamides | Urea |
Fatty acyls | Octadecanoic acid |