Automated workflow for the cell cycle analysis of (non-)adherent cells using a machine learning approach
Figures
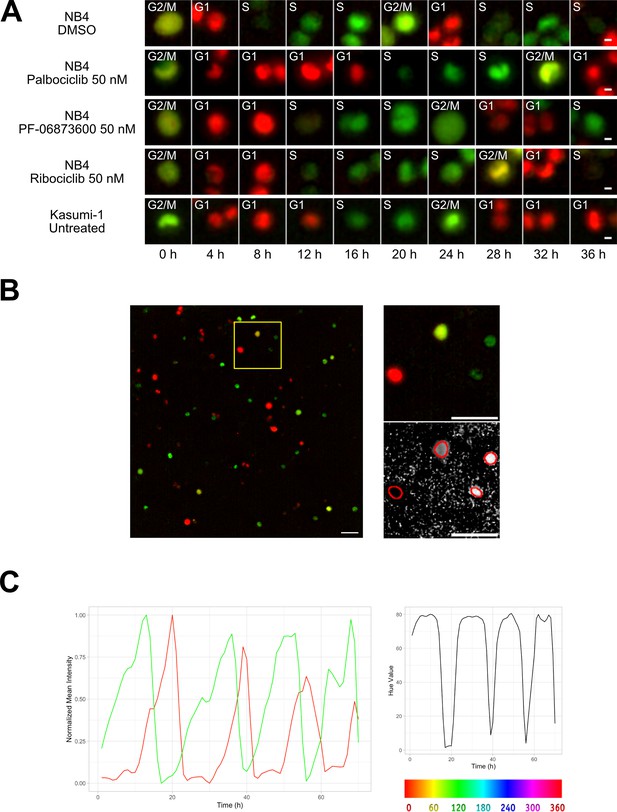
Cell tracking.
(A) Examples from Kasumi-1 cell and NB4 cell lines (upon the different conditions: DMSO, palbociclib 50 nM, PF-0606873600 50 nM, ribociclib 50 nM, untreated), showing a tracked cell that explores the different cell cycle phases (scale bar is 5 µm). (B) Fluorescence images with R and G channels were processed in Fiji to create an HSB stack, from which the brightness and hue channel were extracted to be used respectively as tracking channel and cell phase identification channel (scale bar is 50 µm). (C) Plot curves generated using the data extracted from the TrackMate script execution. The variations over time of mCherry (red curve) and mVenus (green curve) fluorescence intensities, normalized between minimum and maximum values (left plot), and of the hue scale (right plot) of a single NB4 cell in DMSO condition are shown.
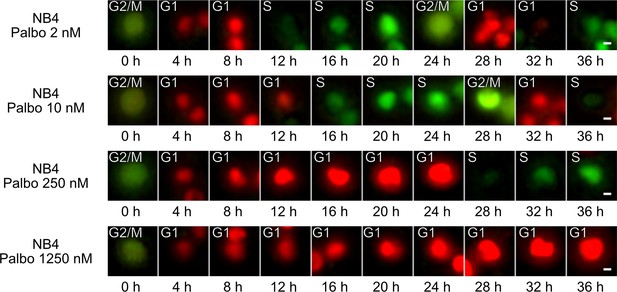
Cell cycle phases in treated NB4 cells.
Examples from NB4 cell line upon different palbociclib treatment concentrations showing a cell that explores the cell cycle phases (scale bar is 5 µm). For palbociclib 250 nM and palbociclib 1250 nM conditions, the cell was manually tracked for presentation purposes.
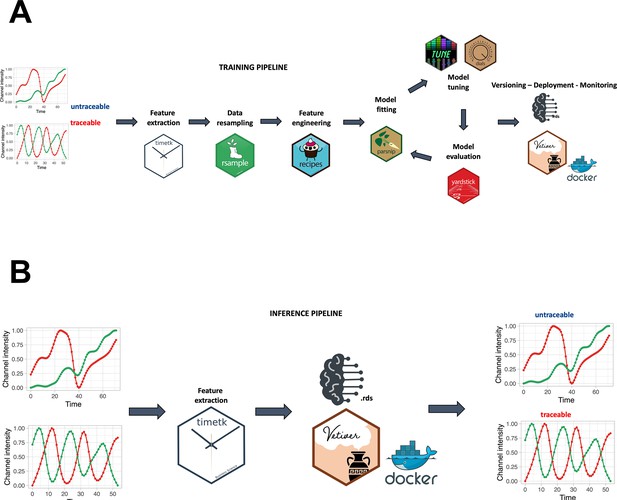
Training and inference pipelines.
(A) The machine learning pipeline followed to create the quality model. Using timetk, time series-associated features are extracted from the list of manually annotated tracks. A random forest model is then trained to predict whether a track is cycling or not. (B) An unannotated track can be fed to the model to predict whether it is cycling or not.
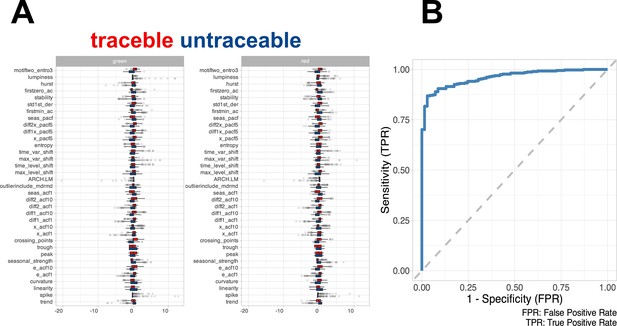
Feature distribution and model performance.
(A) Boxplot showing the distribution of the feature extracted from the training set using timetk. (B) ROC curve of the random forest model computed in the test set.
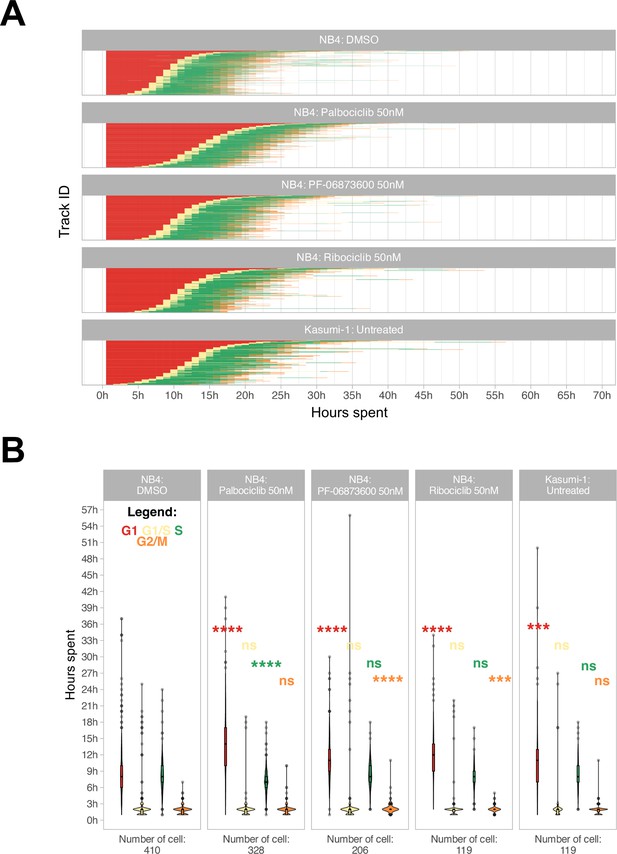
Cell cycle phase assignment.
(A) Waterfall plot of treated and untreated acute myeloid leukemia (AML) cells. Each row corresponds to a single cell. (B) Boxplot of the cell phase duration of the first cell cycle, as obtained by the pipeline, without excluding of the outliers. The asterisks are the adjusted significance level given by Wilcox test of the sample for each over the NB4 DMSO for each phase according to the color (ns: p>0.05, *p≤0.05, **p≤0.01, ***p≤0.001, ****p≤0.0001).
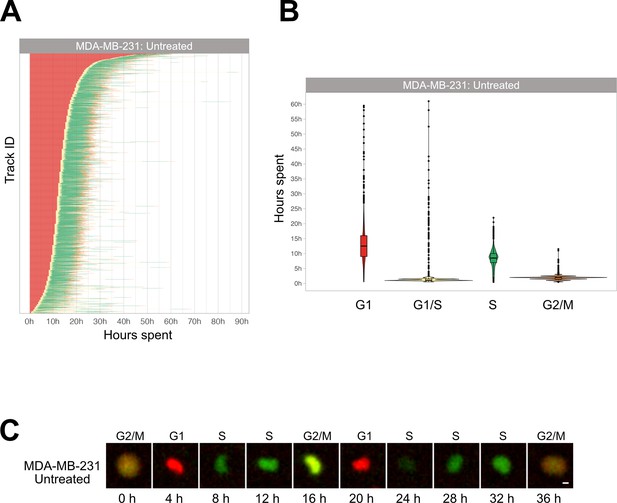
Cell cycle phase assignment in MDA-MB-231 cells.
(A) Waterfall plot of untreated MDA-MB-231 cells. Each row corresponds to a single cell. (B) Boxplot of the cell phase duration of the first cell cycle, as obtained by the pipeline, without excluding of the outliers. A total of 1116 cells were analyzed, obtaining a mean (± SD) cell cycle duration of 24.5 ± 8.5 hr. (C) Example of an adherent cell in control condition tracked with the described pipeline (scale bar is 5 µm).
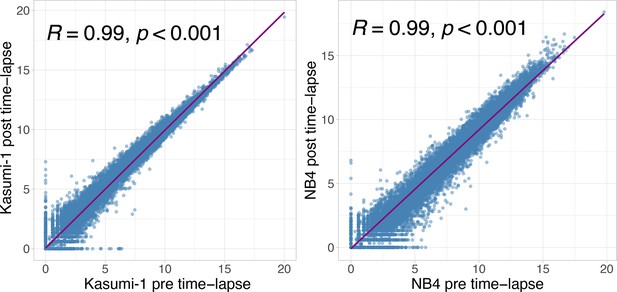
Transcriptome correlation analysis of acute myeloid leukemia (AML) cells seeded on Smart BioSurface (SBS).
Pearson correlation among pre- and post time-lapse acquisition of Kasumi-1 (left) and NB4 (right) cell lines. In the x-axis is reported the log1p read counts mean between the two replicates before the acquisition, while on the y-axis the log1p read counts mean between the two replicates after the acquisition.
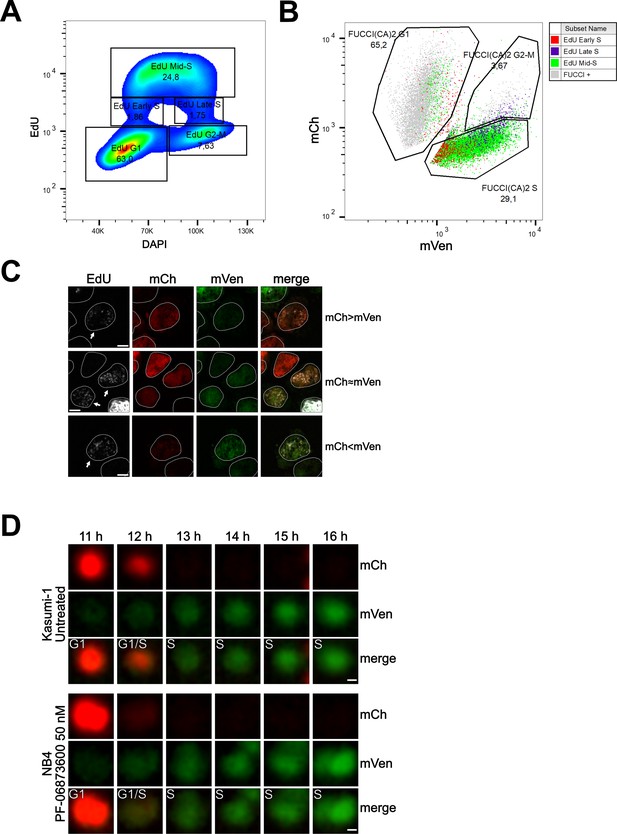
Cell cycle profiling and G1 to S transition analysis.
(A) Density plot showing the cell cycle profiling of FUCCI(CA)2-Kasumi-1 cells based on EdU vs DAPI. (B) Scatter plot of the same cells as in (A) showing the cell cycle profiling based on FUCCI(CA)2. Cells identified to be in early, mid, and late S phase by EdU are highlighted in red, green, and purple, respectively. Due to the chosen gates and the 2 hr EdU pulse, a small percentage of EdU-positive cells leaked into the G1 and G2-M FUCCI(CA)2-defined groups. (C) Representative images of nuclei during the G1 to S transition are indicated by arrows. Depending on the exact time after S phase initiation, the overlay of the mCherry and mVenus channels can show a red prevalence (first row), a yellow color (middle row), or a green prevalence (third row). The scale bar represents 5 µm. (D) The frames, including the transition from G1 to S phase of the untreated Kasumi-1 cell and the NB4 PF-06873600-treated cell shown in Figure 1A, are presented from the 11 hr to the 16 hr frame. In these image sequences, the G1/S label was assigned to the first frame showing dim but detectable mVenus fluorescence and dim but still detectable mCherry fluorescence. The scale bar represents 2 µm.
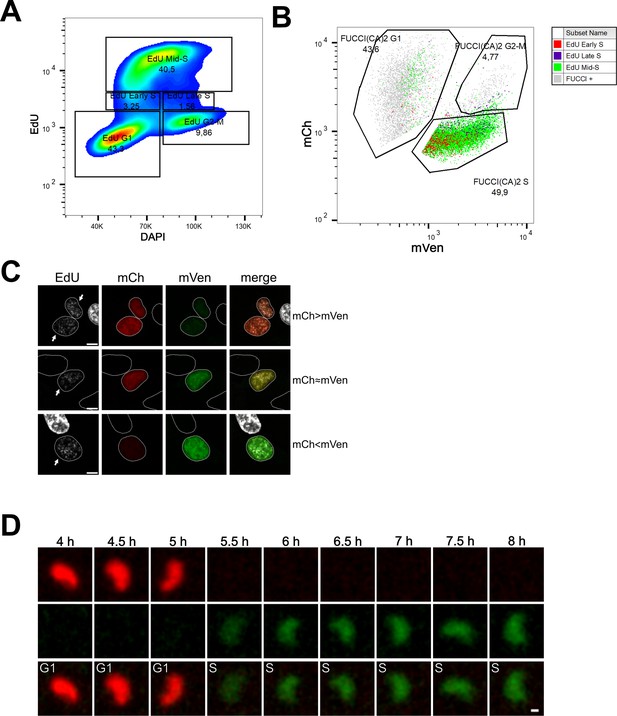
Cell cycle profiling and G1 to S transition analysis in MDA-MB-231 cells.
(A) Density plot showing the cell cycle profiling of FUCCI(CA)2-MDA-MB-231 cells based on EdU vs DAPI. (B) Scatter plot of the same cells in (A) showing the cell cycle profiling based on FUCCI(CA)2. Cells identified to be in early, mid, and late S phase by EdU are highlighted in red, green, and purple, respectively. Due to the chosen gates and the 2 hr EdU pulse, a small percentage of EdU-positive cells leaked into the G1 and G2-M FUCCI(CA)2-defined groups. (C) Representative images of nuclei in the G1 to S transition are indicated by arrows. Depending on the exact time after S phase initiation, the overlay of the mCh and mVen channels can show a red prevalence (first row), a yellow color (middle row), or a green prevalence (third row). Scale bar is 5 µm. (D) The frames of the untreated MDA-MB-231 cell shown in Figure 3—figure supplement 1C are presented from the 4 hr to the 8 hr frame. The scale bar represents 2 µm.
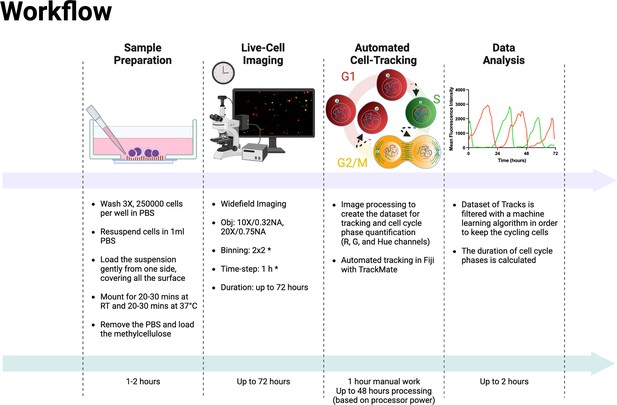
Experimental and analysis workflow.
Summary of the entire experimental workflow, from cell seeding to the calculation of cell cycle phases. Asterisks in the live-cell imaging section mean optional settings. This figure was created with BioRender.com.
Videos
Representative video of NB4 cells seeded on Smart BioSurface (SBS) + methylcellulose (MC).
Representative videos of a NB4 cell in control condition (DMSO) tracked with TrackMate expressing the FUCCI(CA)2 probe, showing the sequence of color changes (1 frame/hr).
Representative video of a Kasumi-1 cell tracked with TrackMate expressing the FUCCI(CA)2 probe, showing the sequence of color changes (1 frame/hr).
Representative videos of NB4 cell treated with palbociclib 50 nM and tracked with TrackMate expressing the FUCCI(CA)2 probe, showing the sequence of color changes (1 frame/hr).
Representative video of NB4 cells seeded on Smart BioSurface (SBS) without methylcellulose (MC).
Tables
Reagent type (species) or resource | Designation | Source or reference | Identifiers | Additional information |
---|---|---|---|---|
Cell line (Homo sapiens) | NB4 | DSMZ | ACC 207 | |
Cell line (H. sapiens) | Kasumi-1 | DSMZ | ACC 220 | |
Cell line (H. sapiens) | MDA-MB-231 | ATCC | HTB-26 | |
Chemical compound, drug | Palbociclib | Selleckchem | S4482 | |
Chemical compound, drug | Ribociclib | Selleckchem | S7440 | |
Chemical compound, drug | PF-06873600 | Selleckchem | S8816 | |
Transfected construct (human) | tFUCCI(CA)2/pCSII-EF | RIKEN BRC through the National BioResource Project of the MEXT, Japan (Cat# RDB15446); Sakaue-Sawano, et al., Mol. Cell 68 (3): 626–640.e5. 2017 | RDB15446 | Lentivirus vector plasmid of mCherry-hCdt1(1/100)Cy(-)_P2A_mVenus-hGem(1/110). |
Commercial assay or kit | Click-iT EdU Alexa Fluor 647 Flow Cytometry Assay Kit | Thermo Fisher Scientific | C10419 | |
Commercial assay or kit | Click-iT EdU Cell Proliferation Kit for Imaging, Alexa Fluor 647 dye | Thermo Fisher Scientific | C10340 | |
Commercial assay or kit | TruSeq RNA Library Preparation Kit v2 | Illumina | RS-122-2001 and RS-122-2002 | |
Software, algorithm | LAS X | Leica Microsystems Inc | https://www.leica-microsystems.com/products/microscope-software/ | |
Software, algorithm | FIJI | Schindelin et al., 2012 | https://fiji.sc/ | |
Software, algorithm | TrackMate | https://doi.org/10.1016/j.ymeth.2016.09.016 | https://imagej.net/plugins/trackmate/ | |
Software, algorithm | R | R Development Core Team, 2021 | https://www.r-project.org/about.html | |
Software, algorithm | Tidyverse | Wickham et al., 2019 | https://www.tidyverse.org/ | |
Software, algorithm | Tidymodels | Kuhn and Wickham, 2020 | https://www.tidymodels.org/ | |
Software, algorithm | tsfeatures | Hyndman, 2023 | https://pkg.robjhyndman.com/tsfeatures/ | |
Software, algorithm | timetk | Dancho and Vaughan, 2024 | https://business-science.github.io/timetk/ | |
Other | DAPI stain | Sigma-Aldrich | 32670 | 5 μg/ml |
Other | Hoechst 33342 stain | Sigma-Aldrich | B2261 | 5 μg/ml |
Other | Leica Thunder Imager | Leica | https://www.leica-microsystems.com/products/thunder-imaging-systems/ | Widefield microscope |
Other | DFC9000 GTC | Leica | https://www.leica-microsystems.com/products/microscope-cameras/p/leica-dfc9000/ | sCMOS camera |
Other | Lumencor Spectra X | Lumencor | https://lumencor.com/products/spectra-x-light-engine | Multi-color illumination |
Other | Glass-bottom multiwell plate | MatTek Corporation | P12G-1.5-14F | |
Other | SBS-coated bottom-glass multiwell plates | Tethis S.p.A. | https://tethis-lab.com/contact/ | Titania nanostructured coating |
Additional files
-
MDAR checklist
- https://cdn.elifesciences.org/articles/94689/elife-94689-mdarchecklist1-v2.docx
-
Supplementary file 1
TrackMate features.
This is the table of the spots that is generated after the execution of the tracking script, that automatize TrackMate plugin. For more available parameters and information, please refer to: TrackMate (https://imagej.net/plugins/trackmate/) and Scripting TrackMate (https://imagej.net/plugins/trackmate/scripting/scripting).
- https://cdn.elifesciences.org/articles/94689/elife-94689-supp1-v2.xlsx