Lipid discovery enabled by sequence statistics and machine learning
Figures
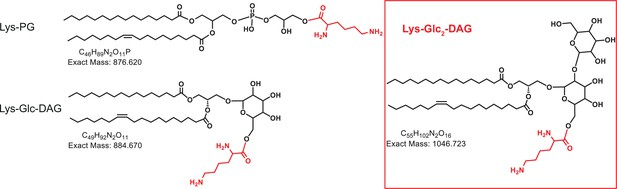
Chemical structures of lysine lipids.
Lys-PG, lysyl-phosphatidylglycerol; Lys-Glc-DAG, lysyl-glucosyl-diacylglycerol; Lys-Glc2-DAG, lysyl-diglucosyl-diacylglycerol.
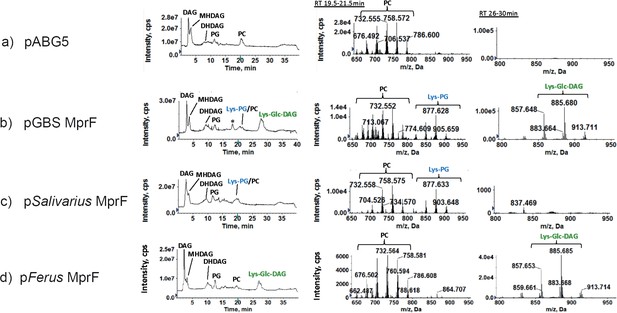
Synthesis of lysine lipids (Lys-PG and Lys-Glc-DAG) in S. mitis expressing mprFs from S. agalactiae, S. salivarus, and S. ferus.
(a) S. mitis NCTC12261 with empty vector control (pABG5) lacks lysine lipids; (b) S. agalactiae mprF (pGBSMprF) produces both Lys-PG and Lys-Glc-DAG; (c) S. salivarius mprF produces only Lys-PG; (d) S. ferus mprF produces only Lys-Glc-DAG. Left panels: total ion chromatograms (TIC); middle panels: mass spectra of retention time 19.5–21.5 min showing Lys-PG and PC; right panels: mass spectra of retention time 26–30 min showing Lys-Glc-DAG. Note: ‘*’ is an extraction artifact due to chloroform used. DAG, diacylglycerol; MHDAG, monohexosyldiacylglycerol; DHDAG, dihexosyldiacylglycerol; PG, phosphatidylglycerol; Lys-PG, lysyl-phosphatidylglycerol; Lys-Glc-DAG, lysyl-glucosyl-diacylglycerol; PC, phosphatidylcholine.
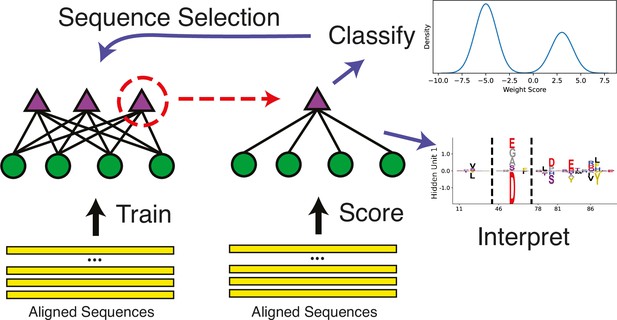
Schematic of the restricted Boltzmann machine (RBM) methodology.
An aligned set of protein sequences is first used to learn a hidden unit representation that best describes the statistics of the sequence dataset given restrictions on the hidden unit representation. Then, the individual hidden units can be studied to find particular units which allow useful enzyme classification, and additionally, these weights can be meaningfully interpreted as statistically covarying sequence configurations. Additionally, the classification can be used to create filtered datasets to train more models.
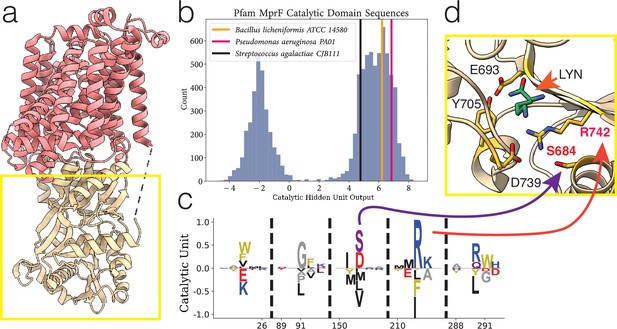
Example of hidden unit analysis and usage.
(a) The structure of PDB:7DUW, with the red colored region being the transmembrane flippase domain and the yellow boxed region the cytosolic domain which we focus on. (b) The activations produced by inputting a sequence into a hidden unit, producing a single number as output which corresponds to a summation of negatively and positively weighted residues. Performed on entire training set (histogram in blue), highlighting sequences corresponding to predominantly positive weighted residues. (c) Hidden unit from a restricted Boltzmann machine (RBM) trained on the Pfam DUF2156 domain. The MSA positions 152 and 212 correspond to residues S684 and R742, respectively. (d) Residues (in yellow) in the MprF cytosolic domain which form the binding pocket for Lys-tRNALys (the ligand analogue L-lysine amide shown in green), from PDB:4V36. LYN, L-lysine amide.
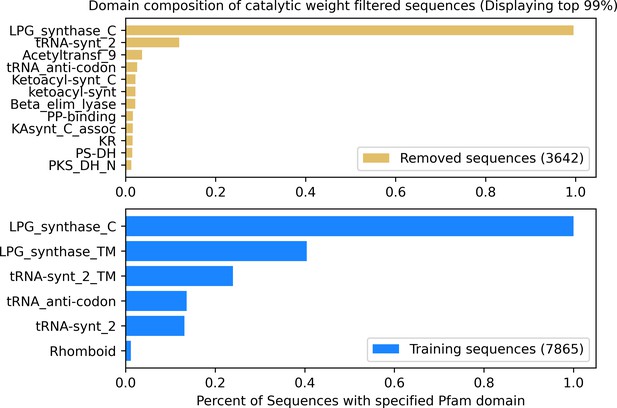
The full sequences of our pre-filtered multiple sequence alignment were analyzed to identify which Pfam domains were present in them.
We then split these results based on the procedure in Figure 3, where Removed sequences indicate domain composition of sequences removed by this procedure, and Training sequences for the data we trained our final model on. We remove domains from this plot which occur in less than 1% of the sequences of their respective sets for clarity.

Proposed set of hidden units for classifying lipid specificity.
(A) Two hidden units found in a restricted Boltzmann machine (RBM) trained on the filtered Pfam dataset. The hidden unit residues are highlighted in the PDB:4V34 structure, with arrows pointing to their corresponding residue sets. (B) The activations of the hidden units when scoring the sequences used in the training set and sequences from NCBI not used during training (N=23,138). S. agalactiae produces Lys-Glc-DAG and Lys-PG, while B. licheniformis and P. aeruginosa produces only aminoacylated-PG. Q1-Q4 are quadrant labels which we refer to throughout the paper. Lys-PG, lysyl-phosphatidylglycerol; Lys-Glc-DAG, lysyl-glucosyl-diacylglycerol.
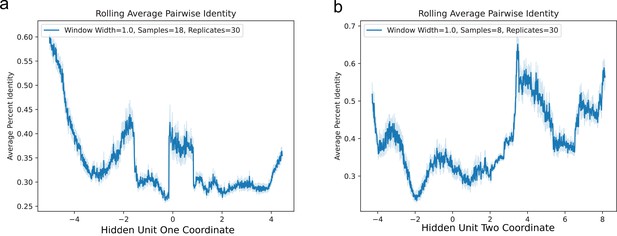
Assessment of restricted Boltzmann machine (RBM) hidden unit’s reliance on total sequence identity in classification.
A sliding window average is performed, where a window of width 1 is slid from the negative end to the positive of the hidden unit activation, where at each position sequence coordinates are sampled from the window. These sampled sequences are compared pairwise between themselves, computing the Hamming distance across their entire sequence length to produce an average. This sampling procedure is repeated 30 times for each window, with the light blue shading representing the 95% confidence interval. Panels (a) and (b) correspond to the sliding window average on hidden units one and two.
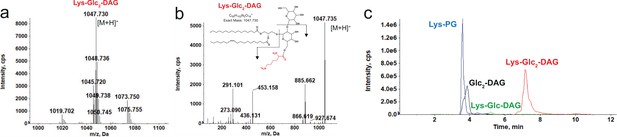
Identification of Lys-Glc2-DAG in E. dispar.
(a) Positive ion mass spectrum of Lys-Glc2-DAG species in E. dispar. Major Lys-Glc2-DAG species with carbon atoms (before colon) and double bonds (after colon). (b) MS/MS product ions and fragmentation scheme of the most abundant Lys-Glc2-DAG ion at m/z 1047.73. (c) Extracted ion chromatograms of LC/MS of lysine lipids (Lys-PG, Lys-Glc-DAG, Lys-Glc2-DAG) and Glc2-DAG separated on an amino HPLC column. Glc2-DAG, diglucosyl-diacylglycerol; Lys-PG, lysyl-phosphatidylglycerol; Lys-Glc-DAG, lysyl-glucosyl-diacylgylcerol; Lys-Glc2-DAG, lysyl-diglucosyl-diacylglycerol.
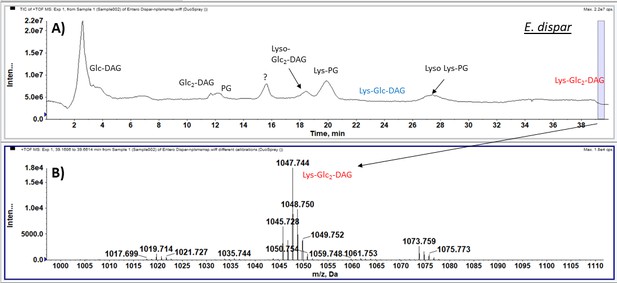
Identification of Lys-Glc2-DAG which is highly retentive on a silica HPLC column.
(A) The total ion chromatogram of normal phase LC/MS of E. dispar lipids separated on a silica HPLC column. (B) The positive ion mass spectrum of Lys-Glc-2DAG eluting at the end of the LC gradient. Glc-DAG, glucosyl-diacylglycerol; Glc-DAG, diglucosyl-diacylglycerol; PG, phosphatidylglycerol; Lyso-Glc2-DAG, lyso diglucosyl-diacylglycerol; Lys-PG, lysyl-phosphatidylglycerol; Lys-Glc-DAG, lysyl-glucosyl-diacylglycerol; Lyso Lys-PG, lyso lysyl-phosphatidylglycerol; Lys-Glc-DAG, lysyl-diglucosyl-diacylglycerol.
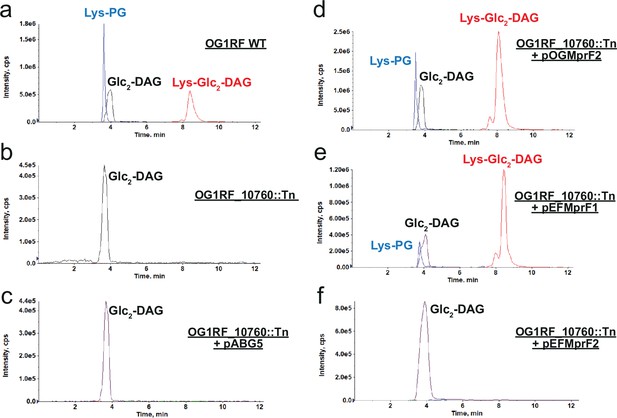
E. faecalis MprF2 and E. faecium MprF1 confer Lys-Glc2-DAG synthesis.
(a) OG1RF-WT; (b) OG1RF_10760::Tn lacks lysine lipids; (c) OG1RF_10760::Tn+pABG5 lacks lysine lipids; (d) OG1RF_10760::Tn+pOGMprF2 restores lysine lipids; (e) OG1RF_10760::Tn+pEFMprF1 restores lysine lipids; (f) OG1RF_10760::Tn+pEFMprF2 lacks lysine lipids. Expression of OGMprF2 and EFMprF1 in OG1RF_10760 Tn mutant restores Lys-Glc2-DAG synthesis. Shown are the extracted ion chromatograms of lysine lipids (Lys-PG, Lys-Glc2-DAG) and Glc2-DAG separated on an amino HPLC column. Note: Lys-Glc-DAG was found in trace amounts or missing from lipid extractions. Glc2-DAG, diglucosyl-diacylglycerol; Lys-PG, lysyl-phosphatidylglycerol; Lys-Glc2-DAG, lysyl-diglucosyl-diacylglycerol.
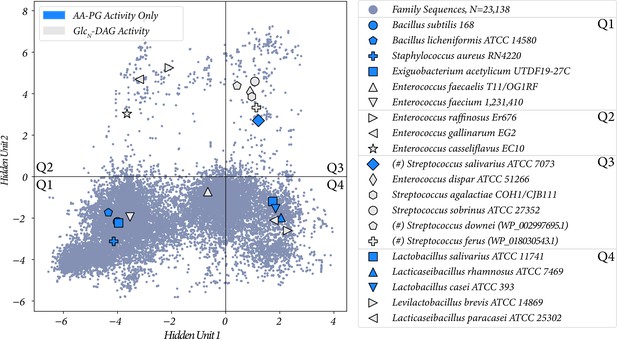
The two proposed hidden unit activations with all of the sequences from Table 2 labeled.
Protein ID of highlighted sequences are listed in Supplementary file 1. (#) indicates the lipid activity was confirmed through heterologous expression.
Tables
Summary of different MprF variants expressed in S. mitis and the lysine lipids they produce.
Percentage of amino acid identity and similarity compared to S. agalactiae COH1 MprF; data obtained from BLASTp. The lipids each strain synthesize are denoted by a checkmark or an x. Lys-PG, lysyl-phosphatidylglycerol; Lys-Glc-DAG, lysyl-glycosyl-diacylglycerol.
Bacteria strains | % Amino acid identity (similarity) | Lys-PG | Lys-Glc-DAG |
---|---|---|---|
SM61(pGBSMprF) | 100.0 (100.0) | ✓ | ✓ |
SM61(pFerus) | 61.9 (79.0) | X | ✓ |
SM61(pSobrinus) | 61.4 (79.0) | X | ✓ |
SM61(pDownei) | 61.0 (79.0) | X | ✓ |
SM61(pSalivarius) | 43.1 (66.0) | ✓ | X |
SM61(pABG5) | - (-) | X | X |
Table of all strains studied, the quadrant they occupy, and the lipids they synthesize.
** trace amounts Lys-Glc-DAG present in lipid extractions. * indicates heterologous expression of mprF in S. mitis. Lys-Glc-DAG, lysyl-glucosyl-diacylglycerol; Lys-Glc2-DAG, lysyl-diglucosyl-diacylglycerol; Lys-PG, lysyl-phosphatidylglycerol.
Bacterial strains | Lys-Glc-DAG | Lys-Glc2-DAG | Lys-PG | |
---|---|---|---|---|
Q1 | Bacillus subtilis 168 | X | X | ✓ |
Bacillus licheniformis ATCC 14580 | X | X | ✓ | |
Staphylococcus aureus RN4220 | X | X | ✓ | |
Exiguobacterium acetylicum UTDF19-27C | X | X | ✓ | |
Enterococcus faecalis T11/OG1RF** | ✓ | ✓ | ✓ | |
Enterococcus faecium 1,231,410 | ✓ | ✓ | ✓ | |
Q2 | Enterococcus raffinosus Er676 | ✓ | ✓ | ✓ |
Enterococcus gallinarum EG2 | ✓ | ✓ | ✓ | |
Enterococcus casseliflavus EC10 | ✓ | ✓ | ✓ | |
Q3 | Streptococcus salivarius* ATCC 7073 | X | X | ✓ |
Enterococcus dispar ATCC 51266 | ✓ | ✓ | ✓ | |
Streptococcus agalactiae CJB111/COH1 | ✓ | X | ✓ | |
Streptococcus sobrinus* ATCC 27352 | ✓ | X | X | |
Streptococcus downei* (WP_002997695.1) | ✓ | X | X | |
Streptococcus ferus* (WP_018030543.1) | ✓ | X | X | |
Q4 | Ligilactobacillus salivarius ATCC 11741 | X | X | ✓ |
Lacticaseibacillus rhamnosus ATCC 7469 | X | X | ✓ | |
Lactobacillus casei ATCC 393 | X | X | ✓ | |
Levilactobacillus brevis ATCC 14869 | X | ✓ | ✓ | |
Lacticaseibacillus paracasei ATCC 25302 | X | ✓ | ✓ |
Additional files
-
Supplementary file 1
Sequence locus IDs for all sequences listed in Table 2.
Bold denotes confirmed mprF allele.
- https://cdn.elifesciences.org/articles/94929/elife-94929-supp1-v1.csv
-
Supplementary file 2
Fisher’s exact test for determining whether positive values of hidden unit 2 are predictive of GlcN-DAG specificity.
p=0.028.
- https://cdn.elifesciences.org/articles/94929/elife-94929-supp2-v1.csv
-
Supplementary file 3
E. coli and plasmids used.
- https://cdn.elifesciences.org/articles/94929/elife-94929-supp3-v1.csv
-
Supplementary file 4
Primers used in this study, with columns indicating regions of sequence complementarity to pABG5.
- https://cdn.elifesciences.org/articles/94929/elife-94929-supp4-v1.csv
-
Supplementary file 5
mprF sequences synthesized by GeneWiz, with columns indicating regions of sequence complementarity to pABG5.
- https://cdn.elifesciences.org/articles/94929/elife-94929-supp5-v1.csv
-
MDAR checklist
- https://cdn.elifesciences.org/articles/94929/elife-94929-mdarchecklist1-v1.docx