Connectomics: A network for swimming
One of the fundamental aims of neuroscience is to understand how circuits of neurons interact to generate complex behavior. Toward that end, efforts are underway to generate complete maps of how all the neurons in a nervous system connect to each other. A complete human 'connectome' is many years away. Therefore, networks of neurons in simpler nervous systems, such as those from fruit flies and nematodes, are being mapped and analyzed as prototypes for understanding the network structure and circuit principles that may underlie bigger brains.
Now, in eLife, Gáspár Jékely and colleagues at the Max Planck Institute for Developmental Biology – including Csaba Verasztó as first author – report details of the connectome responsible for the locomotion of the larvae of a marine worm called Platynereis dumerilii (Verasztó et al., 2017).
The larvae of P. dumerilii swim by moving hair-like structures called cilia in a coordinated manner in response to visual and other sensory cues. These cilia are organized into several bands along the body of the animal known as the prototroch, metatroch and paratroch (Figure 1A). The prototroch sits between the head and the trunk, while the metatroch and three paratroch bands are spread along the trunk. Several patches of cilia are also found in the head of the larva, including on a specialized cell called the crescent cell.
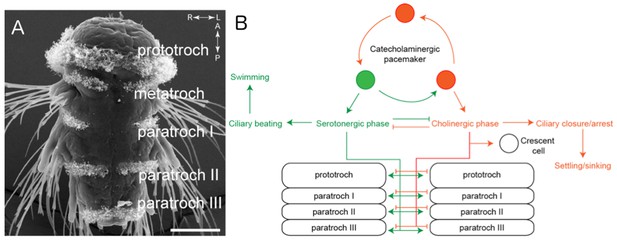
The ciliomotor circuitry of Platynereis dumerilii.
(A) The larvae of Platynereis dumerilii swim by coordinating the beating of cilia on the surface of the body. The cilia are arranged into several bands known as the prototroch, metatroch and paratroch I, II and III. Scale bar, 50 μm. (B) Verasztó et al. mapped the connections between the three classes of motor neurons involved in swimming. The first class of neurons produce a neurotransmitter called acetylcholine and stop the cilia in the prototroch and the three bands of the paratroch beating during the cholinergic phase. These cholinergic neurons also connect to the crescent cell in the head, which has cilia that beat alternately to the cilia in the body. The second class of neurons produce a neurotransmitter called serotonin and make the cilia in the prototroch and paratroch beat faster during the serotonergic phase. The third class of neurons – known as catecholaminergic/peptidergic neurons – form a rhythmic pacemaker system that modulates cilia activity. Figure adapted from Verasztó et al., 2017.
The visual/motor system in the larvae contains only 71 cells (including the photoreceptors that collect visual cues, sensory neurons and the motor neurons that control the beating of the cilia). The wiring of the sensory layers of this system have been mapped previously by a technique called serial electron microscopy reconstruction (Randel et al., 2014), but the connections between the motor neurons and the ciliated cells had not been mapped.
Using neurochemical labeling techniques, Verasztó et al. mapped the neural inputs to the different bands of cilia, subdividing the neurons into three main classes based on the types of neurotransmitter molecules used to relay information between them. The first class (which rely on a neurotransmitter called acetylcholine) is composed of both sensory and motor neurons, including a neuron known as the MC neuron (which is connected to all the cells in the prototroch) and “Loop” neurons that communicate with all of the bands of cilia except the metatroch. Furthermore, these Loop neurons are the only input to the crescent cell.
The neurons in the second class – which produce a neurotransmitter called serotonin – include two “Ser-tr1” cells that connect to cells in the prototroch, metatroch and paratroch. Finally, the third class, known as catecholaminergic/peptidergic neurons, contains three cMN cells that produce different combinations of neurotransmitters, including dopamine, noradrenaline and neuropeptides. These three cells form connections amongst themselves, and also to the prototroch and the MC neuron.
Verasztó et al. then examined the roles these newly-mapped connections play in locomotion using neurochemical and live imaging approaches. This revealed that the MC neuron and the Loop neurons were active when the prototroch cells were active and the cilia were not beating. This suggests that these neurons stop all the cilia on the larva from beating. Consistent with this idea, using a drug to block acetylcholine receptors in the larvae caused the cilia to beat continuously.
The second class of neurons appear to play the opposite role in locomotion because treating the larvae with serotonin caused the cilia to beat faster and without stopping. Also, the Ser-tr1 cells became more active when the cilia in the prototroch band were beating more slowly, presumably to stimulate the cilia to beat faster. Lastly, the three cMN cells from the third class of neurons showed spontaneous rhythmic patterns of activity, with the activity of two of them increasing in synchrony with the activity of the cilia on the prototroch cells, whereas the activity of the third cMN cell was negatively correlated with this activity. Taken together, the results indicate that neurons producing different neurotransmitters are activated in alternating phases to control the beating of the cilia (Figure 1B).
This work demonstrates the power of connectomics to understand how neuronal activity modulates behavior in a simple organism. An interesting contrast can be made with C. elegans, a nematode that also has a simple connectome. While a map of the junctions between the neurons in C. elegans has been available for decades (White et al., 1986) and the pathways of dopamine, noradrenaline and serotonin activity are largely mapped (Bentley et al., 2016), its neuropeptide systems are extremely complicated and thus mostly uncharacterized. This is due to the worm neurons producing a vast number of different neuropeptides, many with uncharacterized activity, unidentified receptors, or unknown expression patterns.
On the other hand, all of the neuropeptides found in Platynereis have been identified and the other components of its neuropeptide systems are better understood. Thus, there is a real prospect of obtaining a complete connectome for Platynereis that could shed light on how neurotransmitters, which often signal between cells that are not directly connected by synapses, interact with the wired circuitry that makes up the connectome. Another difference between Platynereis and C. elegans is that the simplicity of the C. elegans nervous system probably evolved from a more complex nematode ancestor (Malakhov and Hope, 1994), whereas the Platynereis nervous system may more closely resemble that of a distant, more primitive ancestor (Jékely, 2011). If so, the structure of the Platynereis connectome may provide interesting insight into the origin of animal brains.
References
-
The multilayer connectome of Caenorhabditis elegansPLOS Computational Biology 12:e1005283.https://doi.org/10.1371/journal.pcbi.1005283
-
Origin and early evolution of neural circuits for the control of ciliary locomotionProceedings of the Royal Society B: Biological Sciences 278:914–922.https://doi.org/10.1098/rspb.2010.2027
-
BookNematodes: Structure, Development, Classification, and PhylogenyWashington: Smithsonian Institution Press.
-
The structure of the nervous system of the nematode Caenorhabditis elegansPhilosophical Transactions of the Royal Society B: Biological Sciences 314:1–340.https://doi.org/10.1098/rstb.1986.0056
Article and author information
Author details
Publication history
Copyright
© 2017, Chew et al.
This article is distributed under the terms of the Creative Commons Attribution License, which permits unrestricted use and redistribution provided that the original author and source are credited.
Metrics
-
- 1,200
- views
-
- 105
- downloads
-
- 1
- citations
Views, downloads and citations are aggregated across all versions of this paper published by eLife.
Download links
Downloads (link to download the article as PDF)
Open citations (links to open the citations from this article in various online reference manager services)
Cite this article (links to download the citations from this article in formats compatible with various reference manager tools)
Further reading
-
- Developmental Biology
- Neuroscience
Williams syndrome (WS; OMIM#194050) is a rare disorder, which is caused by the microdeletion of one copy of 25–27 genes, and WS patients display diverse neuronal deficits. Although remarkable progresses have been achieved, the mechanisms for these distinct deficits are still largely unknown. Here, we have shown that neural progenitor cells (NPCs) in WS forebrain organoids display abnormal proliferation and differentiation capabilities, and synapse formation. Genes with altered expression are related to neuronal development and neurogenesis. Single cell RNA-seq (scRNA-seq) data analysis revealed 13 clusters in healthy control and WS organoids. WS organoids show an aberrant generation of excitatory neurons. Mechanistically, the expression of transthyretin (TTR) are remarkably decreased in WS forebrain organoids. We have found that GTF2IRD1 encoded by one WS associated gene GTF2IRD1 binds to TTR promoter regions and regulates the expression of TTR. In addition, exogenous TTR can activate ERK signaling and rescue neurogenic deficits of WS forebrain organoids. Gtf2ird1-deficient mice display similar neurodevelopmental deficits as observed in WS organoids. Collectively, our study reveals critical function of GTF2IRD1 in regulating neurodevelopment of WS forebrain organoids and mice through regulating TTR-ERK pathway.
-
- Cell Biology
- Neuroscience
The assembly and maintenance of neural circuits is crucial for proper brain function. Although the assembly of brain circuits has been extensively studied, much less is understood about the mechanisms controlling their maintenance as animals mature. In the olfactory system, the axons of olfactory sensory neurons (OSNs) expressing the same odor receptor converge into discrete synaptic structures of the olfactory bulb (OB) called glomeruli, forming a stereotypic odor map. The OB projection neurons, called mitral and tufted cells (M/Ts), have a single dendrite that branches into a single glomerulus, where they make synapses with OSNs. We used a genetic method to progressively eliminate the vast majority of M/T cells in early postnatal mice, and observed that the assembly of the OB bulb circuits proceeded normally. However, as the animals became adults the apical dendrite of remaining M/Ts grew multiple branches that innervated several glomeruli, and OSNs expressing single odor receptors projected their axons into multiple glomeruli, disrupting the olfactory sensory map. Moreover, ablating the M/Ts in adult animals also resulted in similar structural changes in the projections of remaining M/Ts and axons from OSNs. Interestingly, the ability of these mice to detect odors was relatively preserved despite only having 1–5% of projection neurons transmitting odorant information to the brain, and having highly disrupted circuits in the OB. These results indicate that a reduced number of projection neurons does not affect the normal assembly of the olfactory circuit, but induces structural instability of the olfactory circuitry of adult animals.