Quantification of anti-parasite and anti-disease immunity to malaria as a function of age and exposure
Figures
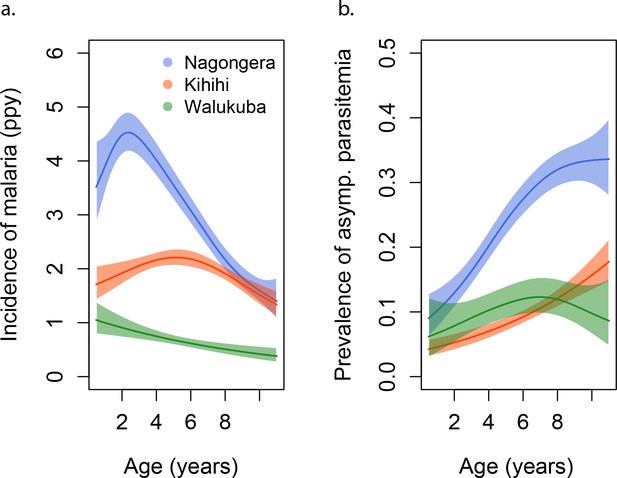
Incidence and prevalence of malaria as a function of age.
(a) and prevalence of asymptomatic parasitemia (b) in the three study sites as a function of age, modeled using generalized additive models (GAMS). Shaded areas represent 95% confidence bounds.
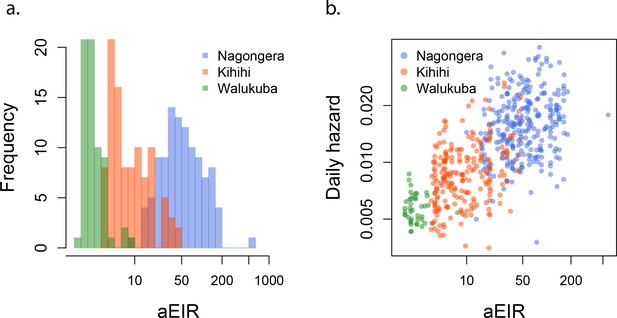
aEIR as a metric of individual exposure.
(a) Distribution of the average annual entomological inoculation rate (aEIR) experienced by the study households in the three study sites. (b) Correlation between the measured aEIRs and the estimated individual hazards of infection.
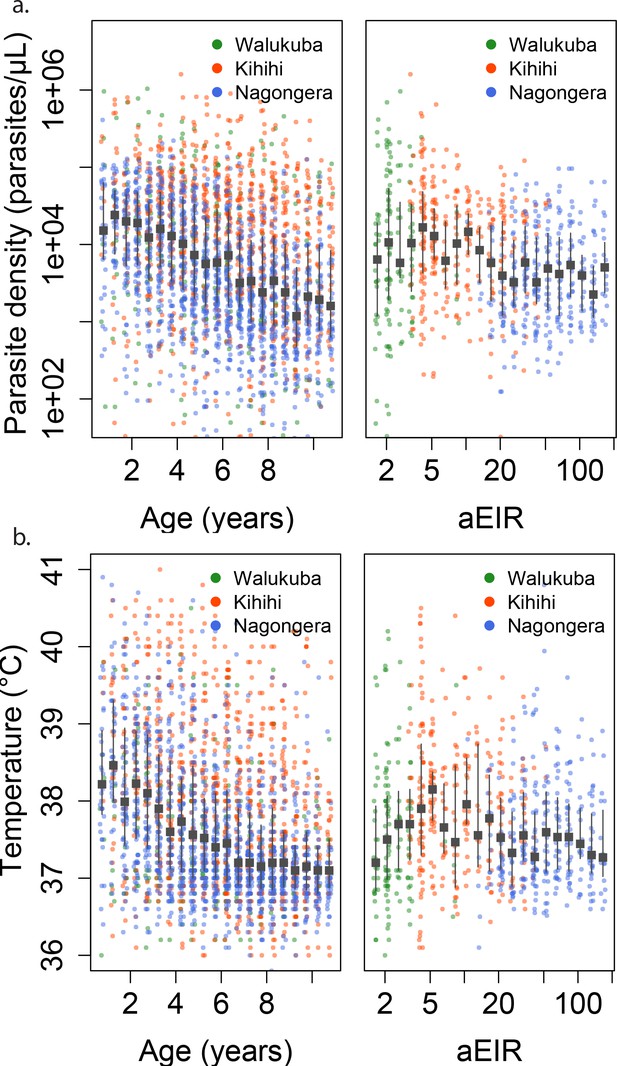
Changes in parasite densities and objective temprature as a function of age and exposure.
Trends in parasite densities. (a) recorded during symptomatic (passive surveillance) infections and routine (active surveillance) visits as a function of age (left) and aEIR (right); and trends in the objective temperature (b) recorded during visits in which participants were found to have a parasite density between 50,000 parasites/μL and 200,000 parasites/μL, as a function of age (left) and aEIR (right). Each point represents a measurement obtained during a study visit. The median and interquartile range are shown in black.
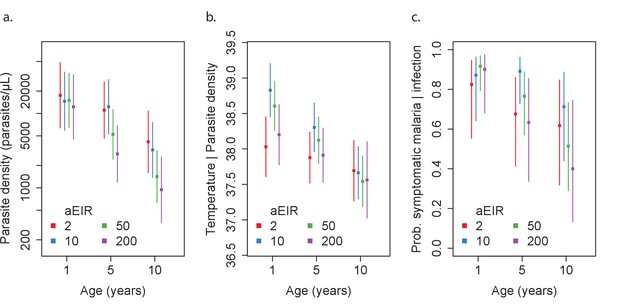
Results of best fitting models quantifying immunity.
(a), anti-disease immunity (b) and overall immunity against symptomatic malaria (c). Each plot shows, for specific ages and aEIRs, the expected parasite density (/μL) (a), objective temperature given a density of 40,000 parasites/μL (b) and the probability of developing symptomatic malaria upon infection (c), estimated using the best fitting model. 95% confidence intervals of the estimates are also shown.
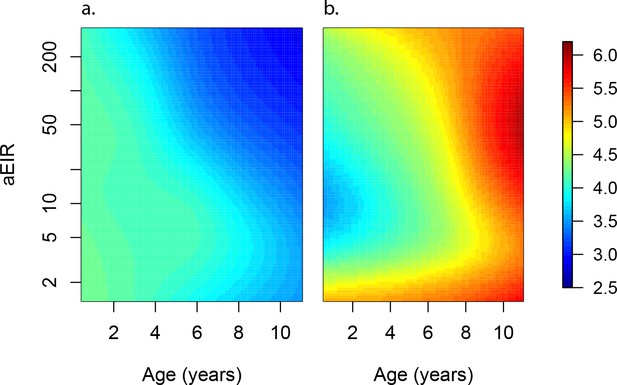
Anti-parasite and Anti-disease immunity as a function of age and exposure.
(a) and anti-disease immunity (b). These results are similar to those presented in Figure 4, but for the full range of ages and aEIRs included in the data. Panel (a) shows expected parasite densities (parasites/μL, log 10) upon infection for different ages and levels of exposure (aEIR). Panel (b) shows the ‘fever threshold’ or ‘pyrogenic density’, the minimum parasite densities (parasites/μL, log 10) associated with fever (temperature 38°C or greater), again as a function of age and exposure.
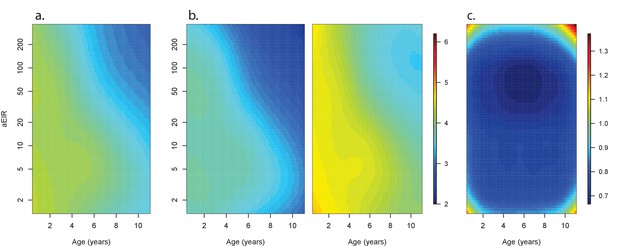
Confidence bounds of best fitting model quantifying anti-parasite immunity.
Left panel (a) is equivalent to Figure 5a, and shows the expected parasite densities (log 10, parasites/μL) after infection at different ages and levels of exposure (aEIR). Panel (b) shows the lower and upper 95% confidence bounds. Panel (c) shows the width of the confidence intervals (2*1.96*standard error) for different levels of age and exposure.
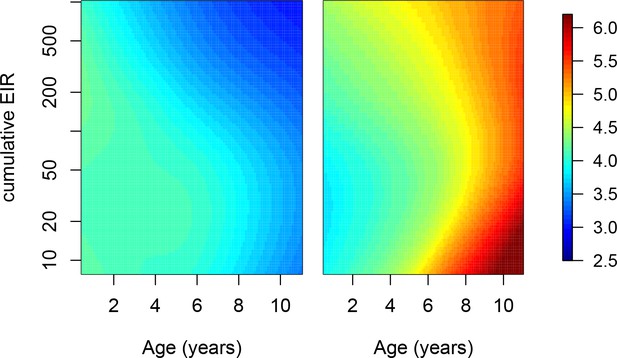
Adjusting for cumulative exposure.
To further explore the independent contribution of age on the development of anti-parasite and anti-disease immunity, we fit models where, instead of adjusting for aEIR, we explicitly adjusted for the cumulative aEIR (cumEIR) as a metric of cumulative exposure. Cumulative aEIR was calculated as the product of age and aEIR. We fit the following models. Anti-parasite immunity. . Anti-disease immunity. . Figure shows the results of these models. These results are similar to those presented in Figure 5, but adjusted for cumulative aEIR rather than for EIR. Left panel shows expected parasite densities (log 10, parasites/μL) after infection at different ages and levels of cumulative exposure. Right panel shows the expected fever thresholds (parasite densities required to develop a temperature 38°C or greater).
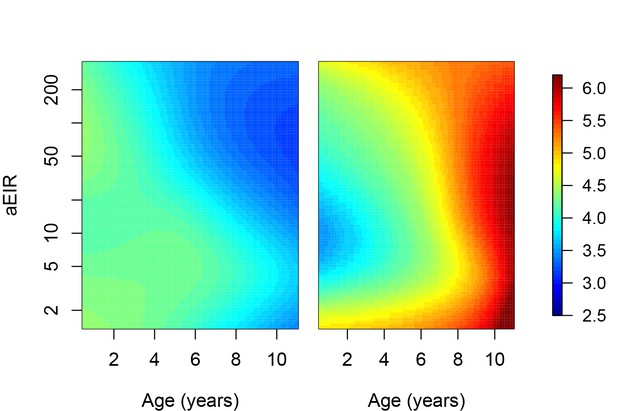
Adjusting for the number of infections in the past 3 months: Results of models.
quantifying anti-parasite (left) and anti-disease immunity (right). These results are similar to those presented in Figure 5, but adjusted for the number of P. falciparum positive visits in the last 3 months. Left panel shows expected parasite densities (log 10, parasites/μL) after infection at different ages and levels of exposure (aEIR). Right panel shows the expected fever thresholds (parasite densities required to develop a temperature 38°C or greater). Appendix 2 shows the estimated coefficients for these and additional models exploring the impact of recent exposure.
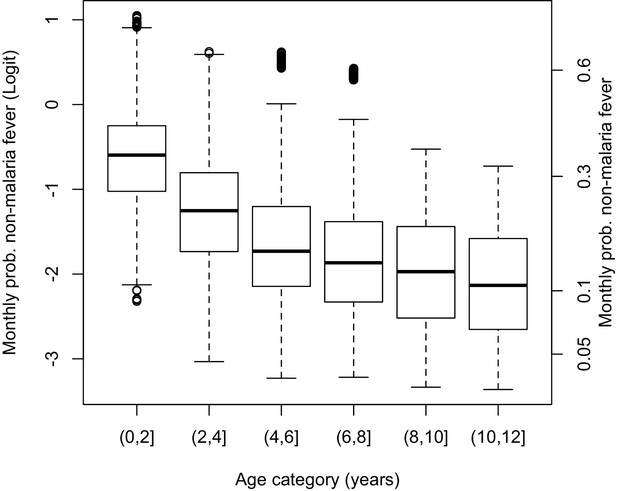
Adjusting for the probability of non-malaria fevers.
Since it is known that the probability of developing fever (not due to malaria) decreases with age, we performed sensitivity analyses where we adjusted for the probability of non-malaria fevers. Using data from instances when participants consulted for fever and/or were objectively febrile but were found to be smear negative, we fit a model to estimate the monthly probability of non-malaria fever as a function of age. This model was specified as: where i is an index for individuals and j is an index for month. The model also included a random effect to account for clustering within individuals. Figure shows the distribution of estimated probabilities of non-malaria for the different age-groups in the dataset. We then used this model to predict the probability of non-malaria fever for each individual at each time point, and re-estimated our anti-parasite and anti-disease models adjusting for this probability (on the logit scale). See Figure 5—figure supplement 5.
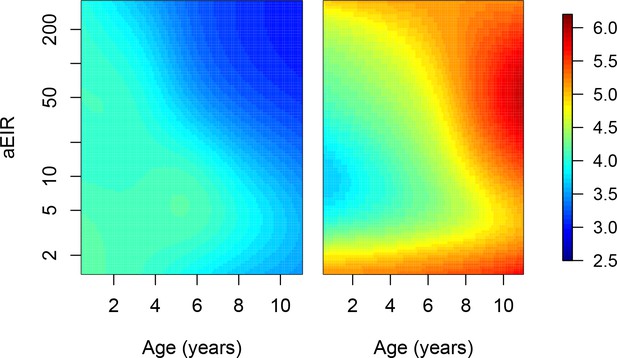
Adjusting for the probability of non-malaria fevers.
Results of models quantifying anti-parasite (left) and anti-disease immunity (right). Left panel shows expected parasite densities (log 10, parasites/μL) after infection at different ages and levels of exposure (aEIR). Right panel shows the expected fever thresholds (parasite densities required to develop a temperature 38°C or greater). These results are similar to those presented in Figure 5, but adjusting for the probability of non-malaria fever experienced by the different age-groups. See Figure 5—figure supplement 4.
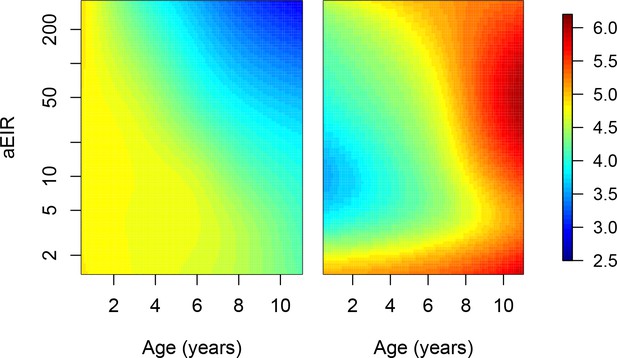
Adjusting for the probability of observation.
Since the study design only included 'routine' samples collections every 3 months, it is likely that we missed several events of asymptomatic infection, particularly in the moderate and high transmission settings. Moreover, since infections were detected using microscopy, we lack knowledge about the complexity of each infection. To account for the lower probability of observing asymptomatic, as compared to symptomatic infections, we conducted sensitivity analyses where we up-weighted asymptomatic observations to account for potentially missed infections. Weights were calculated as the expected number of infections experienced by the participant during the period of time comprised between the last visit (where the participant was tested for parasites) and the current visit. The expected number of infections (M) were derived using estimates of the average daily individual hazard of infection as. where D is the number of days between the last visit when the participant was tested and is the daily probability of infection. Figure shows results of models quantifying anti-parasite (left) and anti-disease immunity (right). Left panel shows expected parasite densities (log 10, parasites/μL) after infection at different ages and levels of exposure (aEIR). Right panel shows the expected fever thresholds (parasite densities required to develop a temperature 38°C or greater). These results are similar to those presented in Figure 5, but weighted for the probability of observation of asymptomatic infections.
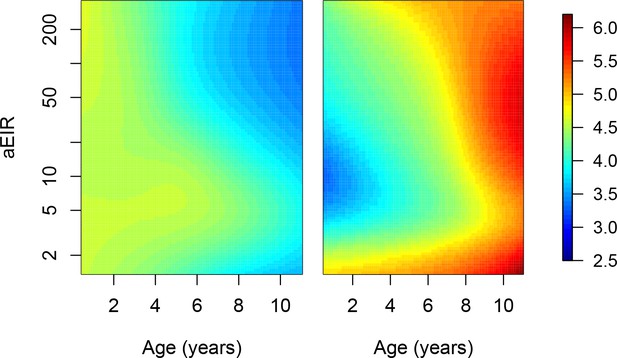
Limiting the analysis to ‘incident’ infections.
Since we don’t have data on the genotypes of parasites, it is also possible that consecutive asymptomatic infections represent persistent, rather than new infections. To assess the potential impact of including these persistent infections in the analyses, we conducted sensitivity analyses where we limited the dataset to ‘incident’ infections. We considered the following samples as belonging to ‘Incident’ infections. All instances of symptomatic malaria infection not preceded by an asymptomatic infection within 10 days. All instances of asymptomatic infection when. The previous blood smear (from routine or active surveillance) was negative. The previous blood smear (from routine surveillance) was positive, but the participant received malaria treatment between that visit and the current one. Limiting the analyses to incident infections implied excluding 700/1431 instances of asymptomatic parasitemia. Figure shows results of models quantifying anti-parasite (left) and anti-disease immunity (right). Left panel shows expected parasite densities (log 10, parasites/μL) after infection at different ages and levels of exposure (aEIR). Right panel shows the expected fever thresholds (parasite densities required to develop a temperature 38°C or greater). These results are similar to those presented in Figure 5, but limiting the dataset to ‘incident’ infections.
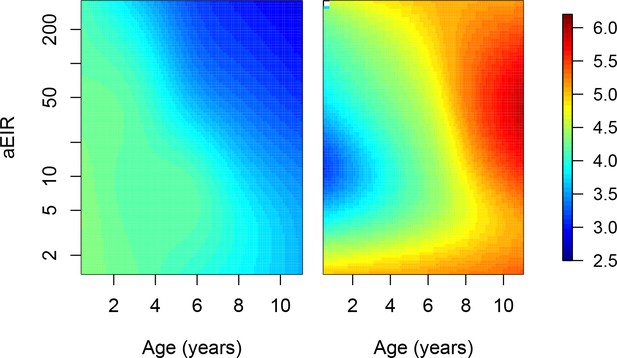
Limiting the analysis to individuals without the sickle hemoglobin mutation (β globin E6V).
This analysis only included data from 618/773 individuals without the sickle hemoglobin mutation (β globin E6V). Figure shows results of models quantifying anti-parasite (left) and anti-disease immunity (right). Left panel shows expected parasite densities (log 10, parasites/μL) after infection at different ages and levels of exposure (aEIR). Right panel shows the expected fever thresholds (parasite densities required to develop a temperature 38°C or greater). These results are similar to those presented in Figure 5, but limited to children without the sickle hemoglobin mutation (β globin E6V).
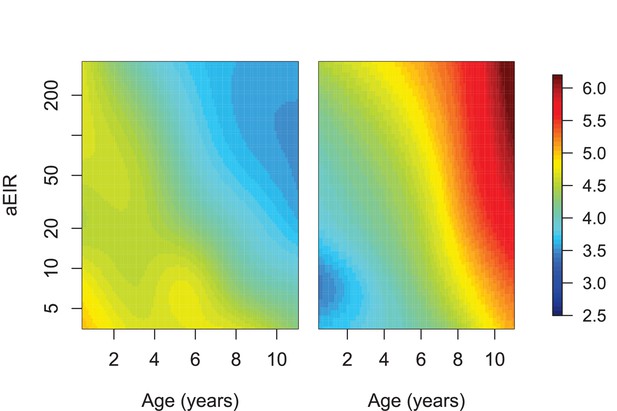
Limiting the analysis to individuals from Tororo and Kanungu.
This analysis only included data from 634/773 individuals living in Tororo (Nagongera) and Kanungu (Kihihi). Figure shows results of models quantifying anti-parasite (left) and anti-disease immunity (right). Left panel shows expected parasite densities (log 10, parasites/μL) after infection at different ages and levels of exposure (aEIR). Right panel shows the expected fever thresholds (parasite densities required to develop a temperature 38°C or greater). These results are similar to those presented in Figure 5, but limited to children living in Tororo (Nagongera) and Kanungu (Kihihi).
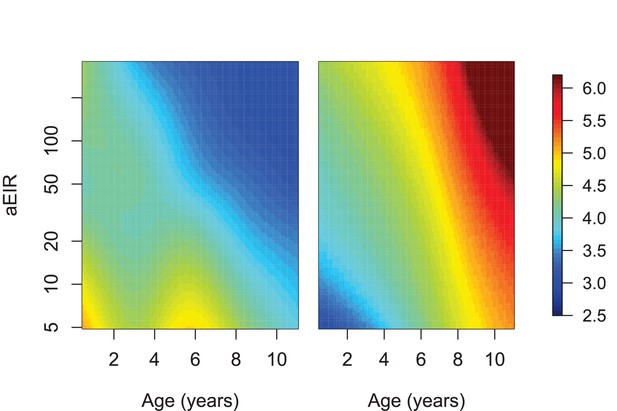
Limiting the analysis to individuals living in settings with aEIR ≥5.
This analysis only included data from 554/773 individuals living in households with aEIR >5. Figure shows results of models quantifying anti-parasite (left) and anti-disease immunity (right). Left panel shows expected parasite densities (log 10, parasites/μL) after infection at different ages and levels of exposure (aEIR). Right panel shows the expected fever thresholds (parasite densities required to develop a temperature 38°C or greater). These results are similar to those presented in Figure 5, but limited to children living in households with aEIR ≥5.
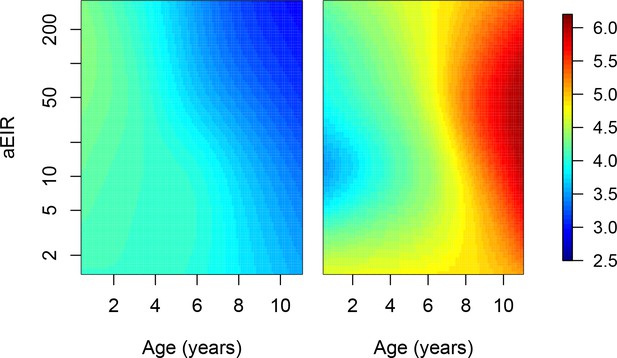
Using a metric of aEIR that only includes prior observations.
In our main analysis, the metric of exposure used was the mean aEIR over the whole study period, as a proxy of the mean exposure that each participant has experienced over their life-time. We performed a sensitivity analysis where instead, aEIR is calculated as the mean aEIR up to each point in time. This analysis only excluded data from 344/5640 observations where we did not have prior exposure information. Figure shows results of models quantifying anti-parasite (left) and anti-disease immunity (right). Left panel shows expected parasite densities (log 10, parasites/μL) after infection at different ages and levels of exposure (aEIR). Right panel shows the expected fever thresholds (parasite densities required to develop a temperature 38°C or greater). These results are similar to those presented in Figure 5, but with an alternative metric of aEIR as described above.
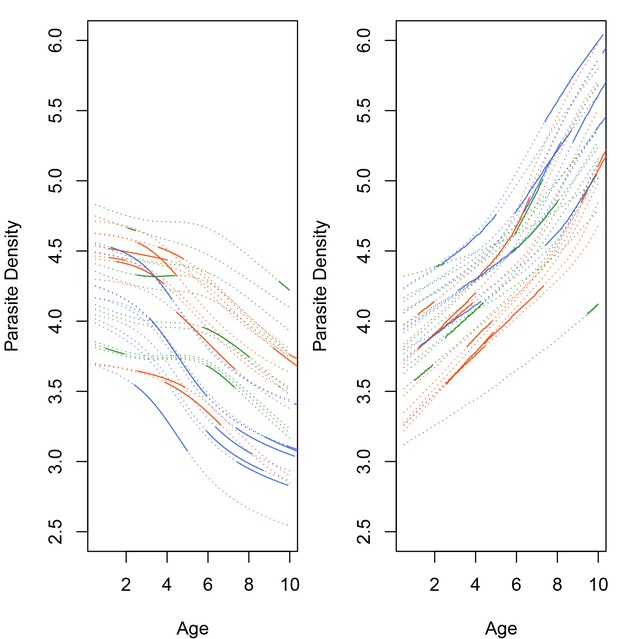
Predicted individual trajectories of anti-disease and anti-parasite immunity.
Figure showing predicted individual trajectories in the development of anti-parasite (a) and anti-disease (b) for a sample of study participants from each study site. (a) shows expected parasite densities (parasites/μL, log 10) after infection at different ages and levels of exposure (aEIR). (b) shows the expected fever thresholds (parasite densities required to develop a temperature 38°C or greater). Each line represents the predicted trajectory, but the solid portion represents the period of time when the individual contributed to the dataset.
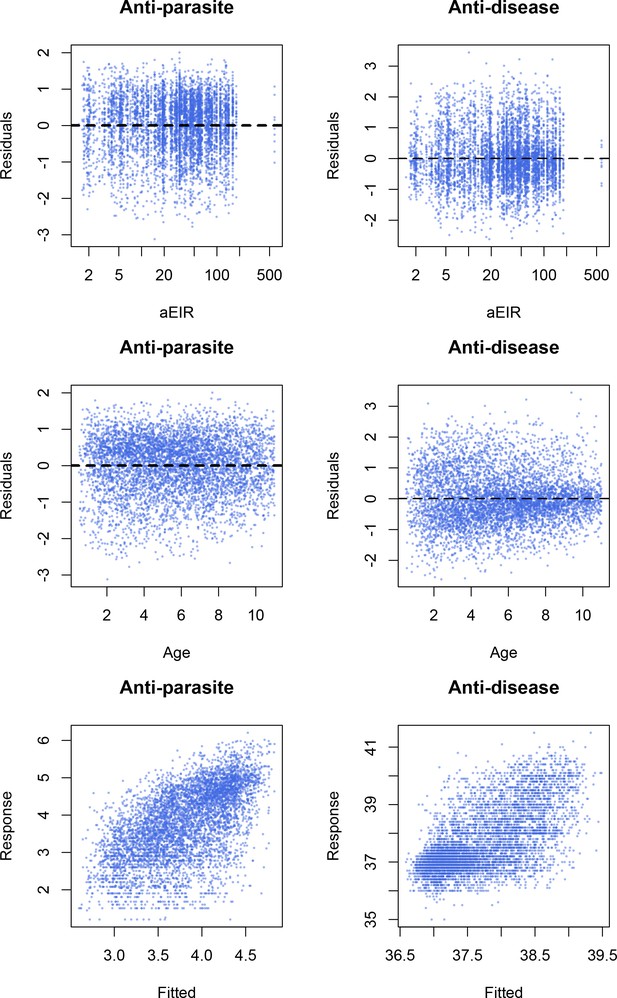
Model checks.
Residual plots and response vs. fitted plots for the best fitting models (Models AP4 and AD4).
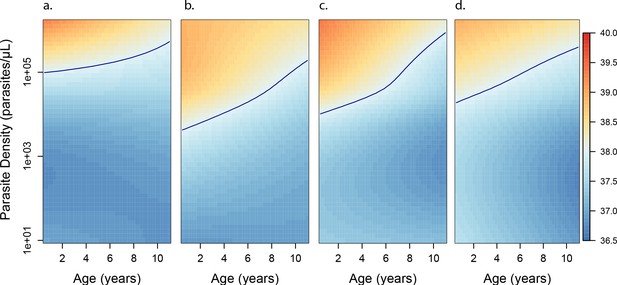
Anti-disease immunity as a function of age and exposure.
Each panel shows how the expected objective temperature (°C) varies as a function of age and parasite density, for different transmission settings. (a) aEIR = 2; (b) aEIR = 10; (c) aEIR = 50; (d) aEIR = 200. Contours indicating the fever threshold (38°C) are also shown. Confidence bounds for these plots are presented in Figure 6—figure supplement 1.
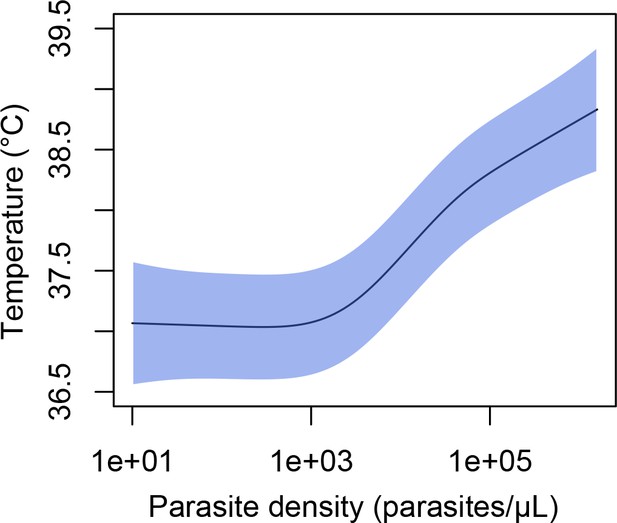
Association between parasite density (parasites/μL) and objective temperature (in °C).
https://doi.org/10.7554/eLife.35832.024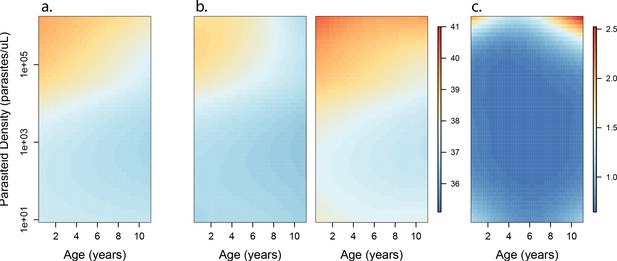
Confidence bounds of best fitting model quantifying anti-disease immunity.
Left panel (a) is equivalent to Figure 6c, and shows how the expected objective temperature (°C) varies as a function of age and parasite density, in a setting with aEIR of 50. Panel (b) shows the lower and upper 95% confidence bounds. Panel (c) shows the width of the confidence intervals (2*1.96*standard error) for different levels of age and exposure.
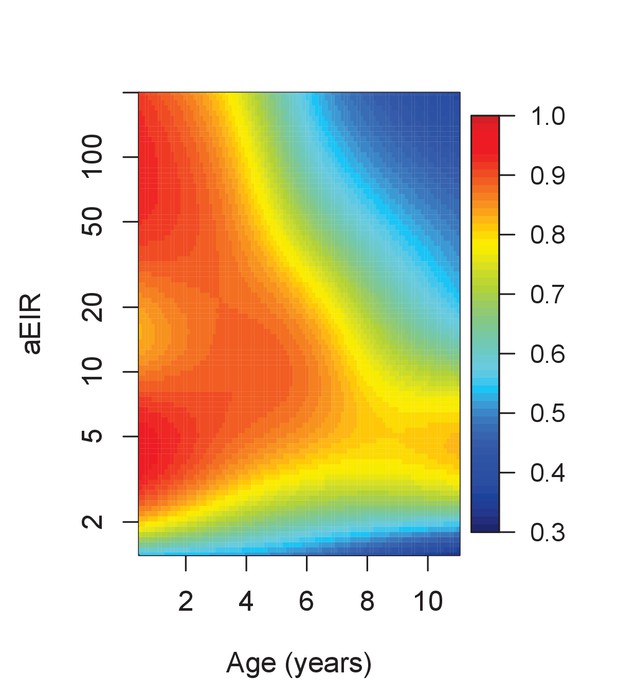
Results of the best model quantifying overall immunity against symptomatic malaria (upon microscopically detectable infection), as a function of age and exposure (aEIR).
Colors represent the expected probability of developing symptomatic malaria upon infection. Confidence bounds for these plots are presented in Figure 7—figure supplement 1.
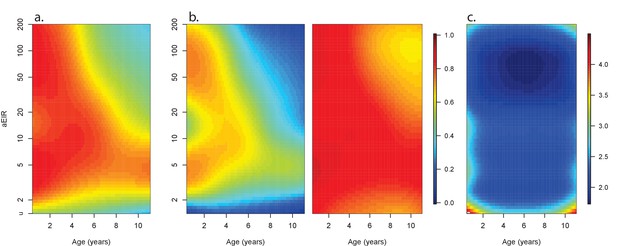
Confidence bounds of best fitting model quantifying overall immunity against symptomatic malaria.
Left panel (a) is equivalent to Figure 7. Colors represent the expected probability of developing symptomatic malaria upon infection, as a function of age and exposure. Panel (b) shows the lower and upper 95% confidence bounds. Panel (c) shows the width of the confidence intervals (2*1.96*standard error), on the logit scale, for different levels of age and exposure.
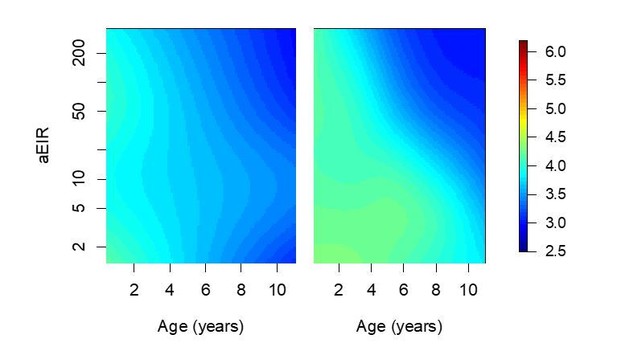
Anti-parasite immunity.
Results of models quantifying anti-parasite immunity. Left panel shows result when models are fit to data from routine visits only (Active) while right panel shows result when models are fit to non-routine (Passive) visits.

Anti-disease immunity.
Results of models quantifying disease immunity when fit to data from non-routine (Passive) visits only. It is not possible to fit this model using only data from routine visits as the majority (~95%) of participants are asymptomatic during these visits and therefore it is not possible to estimate a fever threshold.
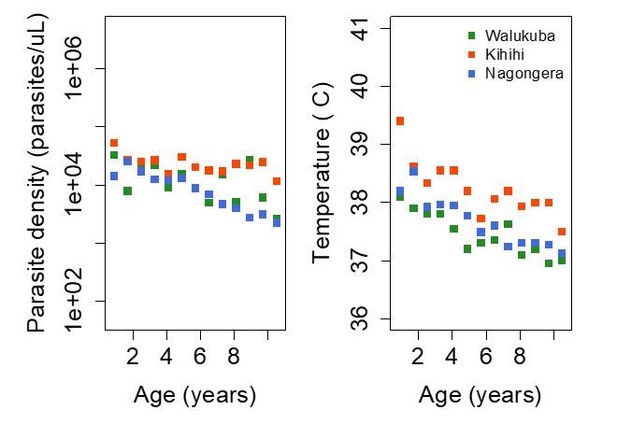
Trends in parasite densities recorded during symptomatic (passive surveillance) infections and routine (active surveillance) visits as a function of age; and trends in the objective temperature recorded during visits in which participants were found to have a parasite density between 50,000 parasites/μL and 200,000 parasites/μL, as a function of age Each point represents a measurement obtained during a study visit.
Dark squares represent the mean of the observations from each site.
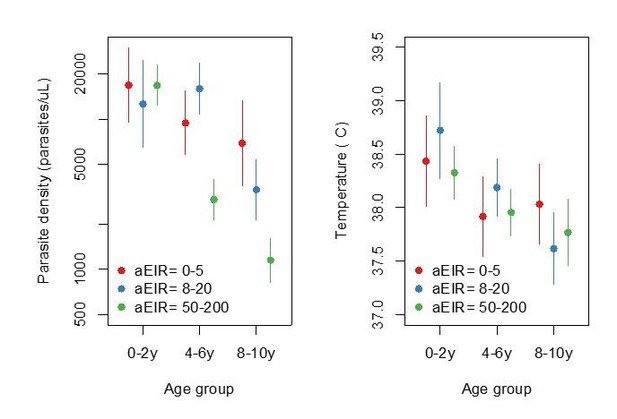
Figure summarizing evidence for anti-parasite immunity (left) and anti-disease immunity (right) in the raw data.
Each plot shows, for specific ages and aEIRs, the mean parasite density (/μL) (left), and the mean objective temperature given a parasite density between 10,000 to 80,000 parasites/μL (right). Means and 95% confidence intervals are shown. This plot corresponds to Figure 4 in the manuscript but was created using raw data (rather than model output).
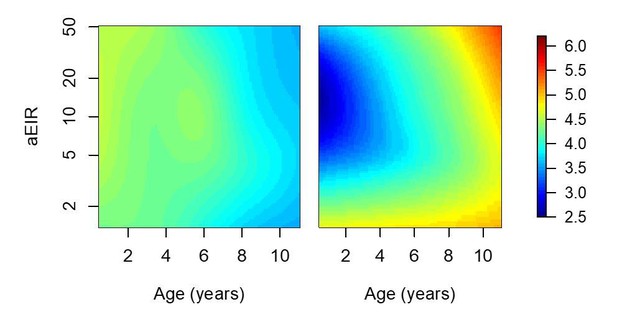
Results of models quantifying anti-parasite and anti-disease immunity.
This figure is similar to figure 5 in the manuscript, but with the analysis limited to 2238/5640 observations from Walukuba and Kihihi (thus the limited aEIR range).
Tables
Characteristics of the study participants.
https://doi.org/10.7554/eLife.35832.003Characteristic | Nagongera | Kihihi | Walukuba |
---|---|---|---|
Number of households | 106 | 100 | 76 |
Number of children | 329 | 305 | 139 |
Female, n (%) | 151(46) | 150 (49) | 66 (47) |
Mean age at enrollment, years (sd) | 4.4 (2.7) | 4.6 (2.6) | 4.3 (2.6) |
Mean follow up time, months (range) | 23.5 (0, 38.8) | 24.4 (0.8, 38.8) | 22.1 (2.3, 3.9) |
Symptomatic malaria | |||
Symptomatic Malaria episodes, n | 2447 | 1555 | 207 |
Median number of symptomatic malaria episodes/child, n (range) | 6 (0, 29) | 4 (0, 30) | 1 (0. 12) |
Median incidence of symptomatic malaria episodes ppy (range) | 2.6 (0, 10) | 1.6 (0, 15.2) | 0.6 (0, 5.1) |
Asymptomatic parasitemia | |||
Asymptomatic parasitemia episodes, n | 955 | 331 | 145 |
Median number of asymptomatic parasitemia episode/child, n (range) | 2 (0, 12) | 0 (0, 11) | 1 (0, 10) |
Median prevalence of asymptomatic parasitemia (range) | 0.12 (0.07–0.17) | 0.05 (0.02–0.10) | 0.07 (0.03–0.11) |
Household malaria exposure | |||
Household aEIR, median (range) | 51 (10–582) | 7.7 (3.6–47) | 2.1 (1.5–8.1) |
Results of linear models quantifying the association between age, aEIR and immunity outcomes.
https://doi.org/10.7554/eLife.35832.007All data | aEIR ≥ 5 (n = 5047) | aEIR < 5 (n = 593) | |
---|---|---|---|
Anti-parasite immunity | Fold change in parasite density (95% CI) | ||
Age (years) | 0.78 (0.77, 0.79) | 0.76 (0.75, 0.77) | 0.87 (0.83, 0.90) |
Log2 aEIR | 0.82 (0.79, 0.84) | 0.73 (0.69, 0.77) | 1.92 (1.69, 2.15) |
Anti-disease immunity* | Change in objective temperature C (95% CI) | ||
Age (years) | −0.07 (–0.06, –0.08) | −0.08 (–0.07, –0.1) | −0.04 (–0.07, –0.01) |
Log2 aEIR | −0.02 (–0.04, 0.0) | −0.07 (–0.05, –0.1) | 0.27 (0.11, 0.44) |
Overall immunity against symptomatic malaria | Odds ratio of symptomatic disease (95% CI) | ||
Age (years) | 0.78 (0.75, 0.82) | 0.77 (0.74, 0.80) | 0.90 (0.83, 0.99) |
Log2 aEIR | 0.91 (0.74, 1.13) | 0.62 (0.48, 0.80) | 3.83 (1.39, 10.6) |
-
*Model adjusted as well for Log parasite density.
Anti-parasite immunity Model fit.
https://doi.org/10.7554/eLife.35832.030Model | DF | % Deviance Explained | AIC |
---|---|---|---|
AP1 | 418.7 | 33.2 | 14052 |
AP2 | 414.2 | 33.1 | 14048 |
AP3 | 408 | 33 | 14046 |
AP4 | 404 | 33 | 14032 |
Anti-disease immunity Model fit.
https://doi.org/10.7554/eLife.35832.031Model | DF | % Deviance Explained | AIC |
---|---|---|---|
AD1 | 315 | 37.7 | 14946 |
AD2 | 285 | 39.0 | 14774 |
AD3 | 314 | 38.3 | 14894 |
AD4 | 296 | 39.4 | 14758 |
Overall immunity against symptomatic malaria Model fit.
https://doi.org/10.7554/eLife.35832.032Model | DF | % Deviance Explained | AIC |
---|---|---|---|
SM1 | 399.8 | 28 | 5382 |
SM2 | 385.3 | 28 | 5373 |
SM3 | 385.5 | 27.8 | 5380 |
SM4 | 362.6 | 27.3 | 5369 |
Anti-parasite immunity.
https://doi.org/10.7554/eLife.35832.034Time window | Functional form | Coefficient (95% CI) | p-value |
---|---|---|---|
3 months | Linear | −0.003 (−0.03–0.03) | 0.83 |
6 months | Linear | 0.003 (−0.02–0.02) | 0.75 |
6 months | Smooth | - | 0.77 |
Anti-disease immunity.
https://doi.org/10.7554/eLife.35832.035Time window | Functional form | Coefficient (95% CI) | p-value |
---|---|---|---|
3 months | Linear | −0.01 (−0.04–0.02) | 0.38 |
6 months | Linear | −0.01 (−0.03–0.01) | 0.15 |
6 months | Smooth | - | 0.15 |
Additional files
-
Transparent reporting form
- https://doi.org/10.7554/eLife.35832.028