Discovering and deciphering relationships across disparate data modalities
Figures
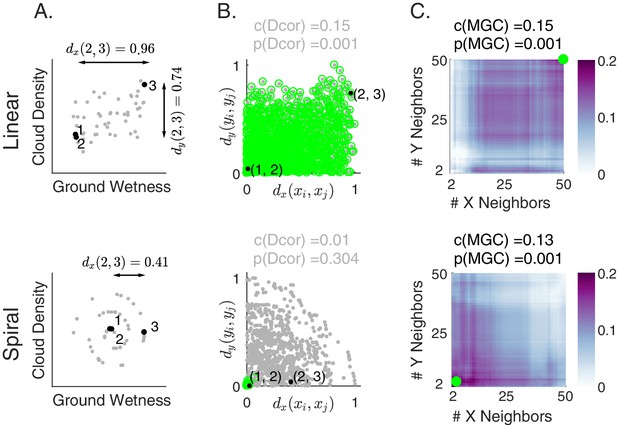
Illustration of Multiscale Graph Correlation (Mgc) on simulated cloud density () and grass wetness ().
We present two different relationships: linear (top) and nonlinear spiral (bottom; see Materials and methods for simulation details). (A) Scatterplots of the raw data using pairs of samples for each scenario. Samples , , and (black) are highlighted; arrows show distances between these pairs of points while their distances are almost 0. (B) Scatterplots of all pairs of distances comparing and distances. Distances are linearly correlated in the linear relationship, whereas they are not in the spiral relationship. Dcorr uses all distances (gray dots) to compute its test statistic and p-value, whereas Mgc chooses the local scale and then uses only the local distances (green dots). (C) Heatmaps characterizing the strength of the generalized correlation at all possible scales (ranging from to for both and ). For the linear relationship, the global scale is optimal, which is the scale that Mgc selects and results in a p-value identical to Dcorr. For the nonlinear relationship, the optimal scale is local in both and , so Mgc achieves a far larger test statistic, and a correspondingly smaller and significant p-value. Thus, Mgc uniquely detects dependence and characterizes the geometry in both relationships.
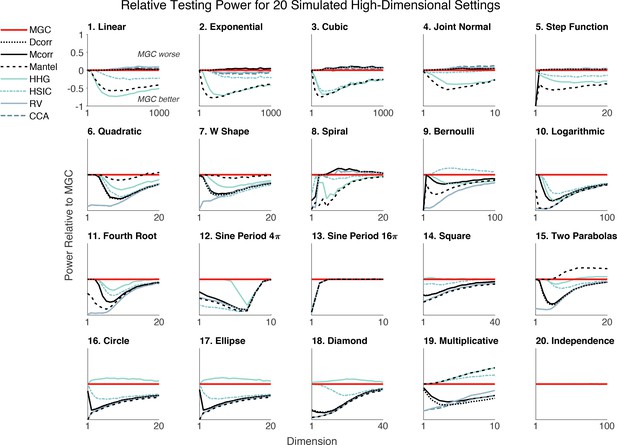
An extensive benchmark suite of 20 different relationships spanning polynomial, trigonometric, geometric, and other relationships demonstrates that Mgc empirically nearly dominates eight other methods across dependencies and dimensionalities ranging from 1 to 1000 (see Materials and methods and Figure 2—figure supplement 1 for details).
Each panel shows the testing power of other methods relative to the power of Mgc (e.g. power of Mcorr minus the power of Mgc) at significance level versus dimensionality for . Any line below zero at any point indicates that that method’s power is less than Mgc’s power for the specified setting and dimensionality. Mgc achieves empirically better (or similar) power than all other methods in almost all relationships and all dimensions. For the independent relationship (#20), all methods yield power as they should. Note that Mgc is always plotted ‘on top’ of the other methods, therefore, some lines are obscured.
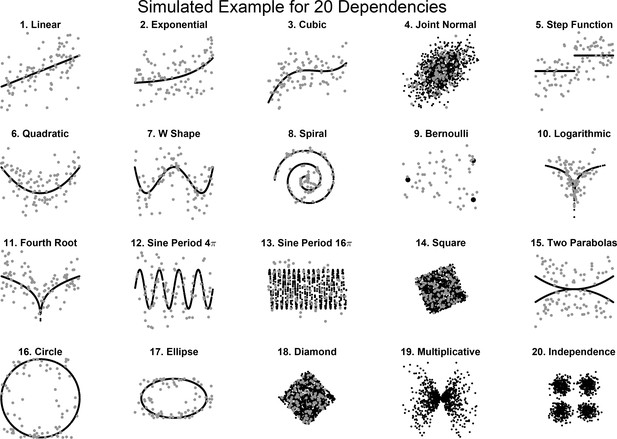
Visualization of the dependencies at .
For each, points are sampled with noise () to show the actual sample data used for one-dimensional relationships (gray dots). For comparison purposes, points are sampled without noise () to highlight each underlying dependency (black dots). Note that only black points are plotted for type 19 and 20, as they do not have the noise parameter .
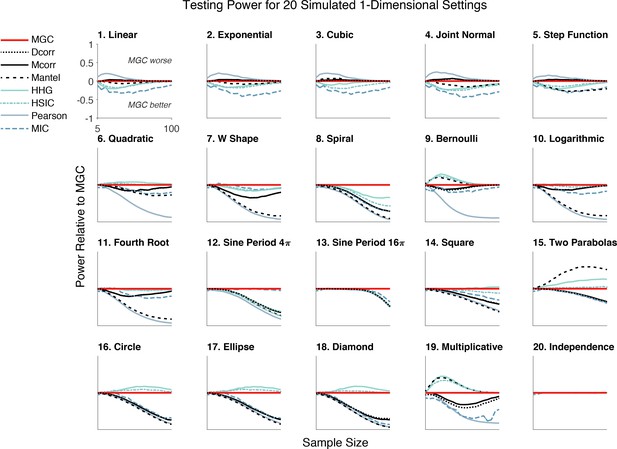
The same power plots as in Figure 2, except the 20 dependencies are one-dimensional with noise, and the x-axis shows sample size increasing from 5 to 100.
Mgc empirically achieves similar or better power than the previous state-of-the-art approaches on most problems. Note that Mic is included in 1D case; RV and Cca both equal Pearson in 1D; Kendall and Spearman are too similar to Pearson in power and thus omitted in plotting.
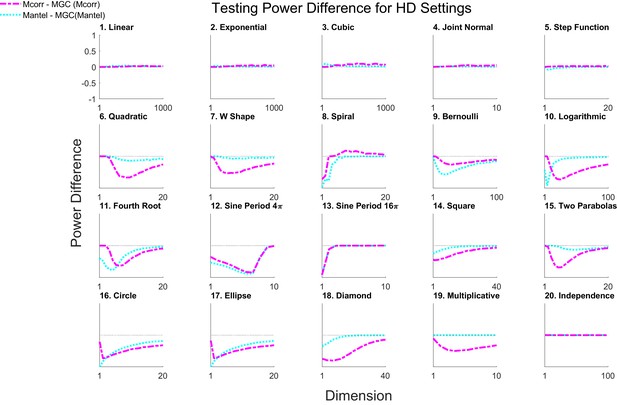
The same set-ups as in Figure 2, comparing different Mgc implementations versus its global counterparts.
The default Mgc builds upon Mcorr throughout the paper, and we further consider Mgc on Mantel to illustrate the generalization. The magenta line shows the power difference between Mcorr and Mgc , and the cyan line shows the power difference between Mantel and the Mgc version of Mantel. Indeed, Mgc is able to improve the global counterpart in testing power under nonlinear dependencies, and maintains similar power under linear and independent dependencies.
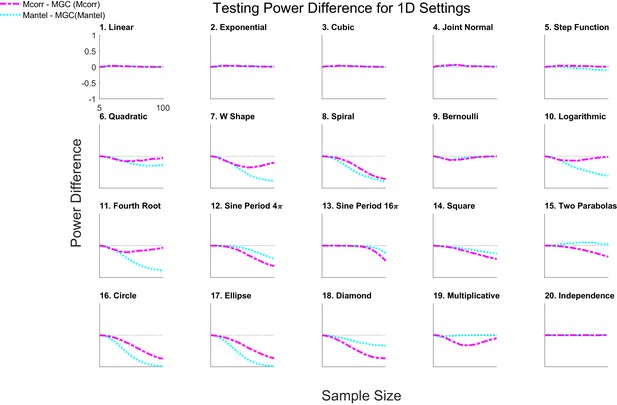
The same power plots as in Figure 3, except the 20 dependencies are one-dimensional with noise, and the x-axis shows sample size increasing from 5 to 100.
https://doi.org/10.7554/eLife.41690.008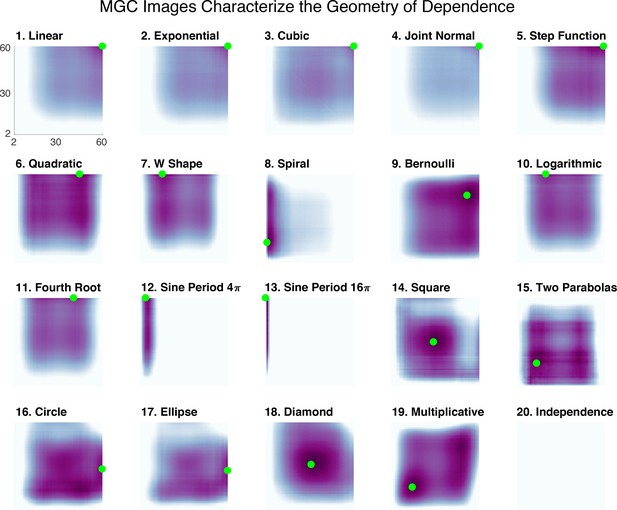
The Mgc-Map characterizes the geometry of the dependence function.
For each of the 20 panels, the abscissa and ordinate denote the number of neighbors for and , respectively, and the color denotes the magnitude of each local correlation. For each simulation, the sample size is 60, and both and are one-dimensional. Each dependency has a different Mgc-Map characterizing the geometry of dependence, and the optimal scale is shown in green. In linear or close-to-linear relationships (first row), the optimal scale is global, that is the green dot is in the top right corner. Otherwise the optimal scale is non-global, which holds for the remaining dependencies. Moreover, similar dependencies often share similar Mgc-Maps and similar optimal scales, such as (10) logarithmic and (11) fourth root, the trigonometric functions in (12) and (13 , 16) circle and (17) ellipse, and (14) square and (18) diamond. The Mgc-Maps for high-dimensional simulations are provided in Figure 3—figure supplement 1.
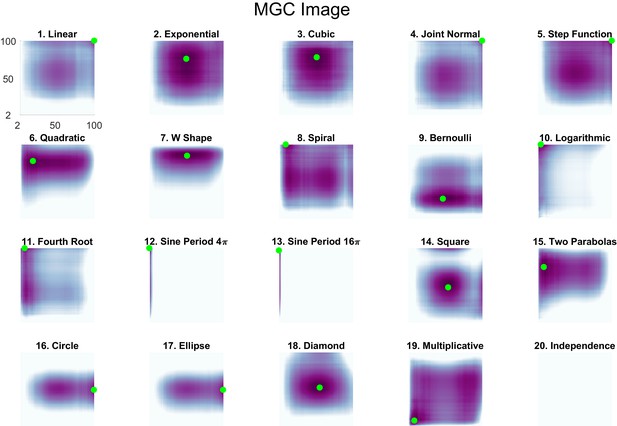
The Mgc-Map for the 20 panels for high-dimensional dependencies.
For each simulation, the sample size is 100, and the dimension is selected as the dimension such that Mgc has a testing power above 0.5. It has similar behavior and interpretation as the one-dimensional power maps in Figure 3, that is the linear relationships optimal scales are global, and similar dependencies share similar Mgc-Maps.
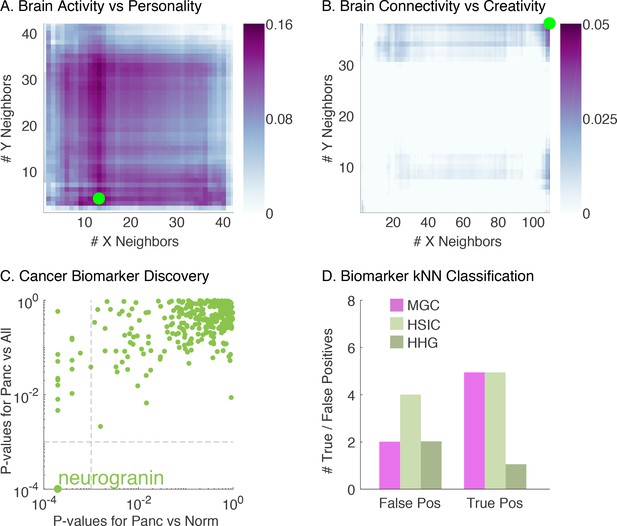
Demonstration that Mgc successfully detects dependency, distinguishes linearity from nonlinearity, and identifies the most informative feature in a variety of real data experiments.
(A) The Mgc-Map for brain activity versus personality. Mgc has a large test statistic and a significant p-value at the optimal scale (13, 4), while the global counterpart is non-significant. That the optimal scale is non-global implies a strongly nonlinear relationship. (B) The Mgc-Map for brain connectivity versus creativity. The image is similar to that of a linear relationship, and the optimal scale equals the global scale, thus both Mgc and Mcorr are significant in this case. (C) For each peptide, the x-axis shows the p-value for testing dependence between pancreatic and healthy subjects by Mgc, and the y-axis shows the p-value for testing dependence between pancreatic and all other subjects by Mgc. At critical level , Mgc identifies a unique protein after multiple testing adjustment. (D) The true and false positive counts using a k-nearest neighbor (choosing the best ) leave-one-out classification using only the significant peptides identified by each testing method. The peptide identified by Mgc achieves the best true and false positive rates, as compared to the peptides identified by Hsic or Hhg.
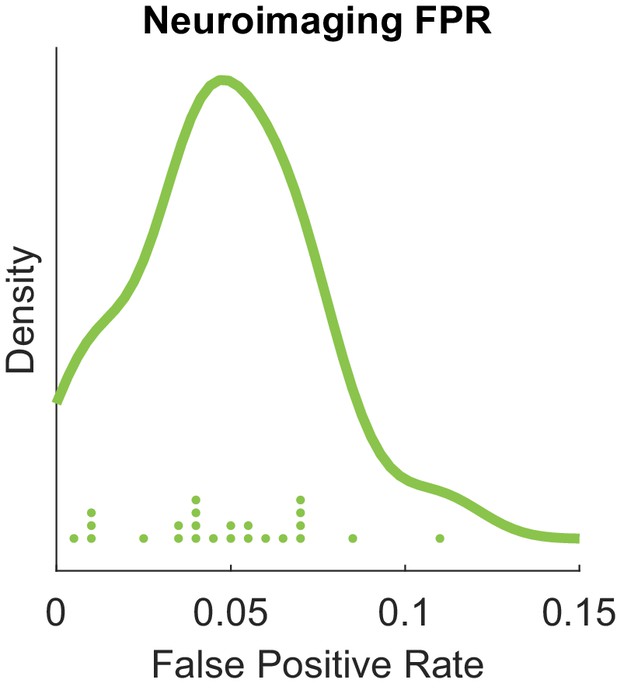
We demonstrate that Mgc is a valid test that does not inflate the false positives in screening and variable selection.
This figure shows the density estimate for the false positive rates of applying Mgc to select the 'falsely significant' brain regions versus independent noise experiments; dots indicate the false positive rate of each experiment. The mean ± standard deviation is 0.0538 ± 0.0394.
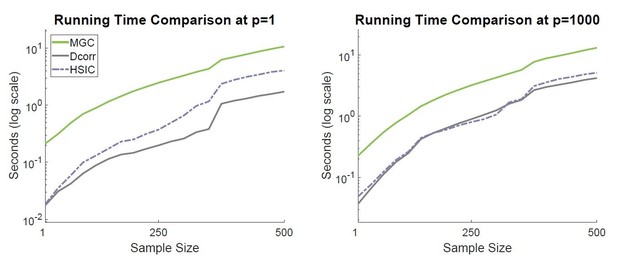
Compute the test statistics of MGC, DCORR, and HSIC for 100 replicates, and then plot the average running time in log scale (clocked using Matlab 2017a on a Windows 10 machine with I7 six-core CPU).
The sample data are repeatedly generated using the quadratic relationship in Appendix, the sample size increases from 25 to 500, and the dimensionality is fixed at p = 1 on the left and p = 1000 on the right. In either panel, the three lines differ by some constants in the log scale, suggesting the same running time complexity but different constants. MGC has a higher intercept than the other two, which translates to about a constant of 6 times of DCORR and 3 times of HSIC at n = 500 and p = 1, and about 3 at p = 1000.
Tables
The median sample size for each method to achieve power 85% at type one error level 0.05, grouped into monotone (type 1–5) and non-monotone relationships (type 6–19) for both one- and ten-dimensional settings, normalized by the number of samples required by Mgc.
In other words, a 2.0 indicates that the method requires double the sample size to achieve 85% power relative to Mgc. Pearson, Rv, and Cca all achieve the same performance, as do Spearman and Kendall. Mgc requires the fewest number of samples in all settings, and for high-dimensional non-monotonic relationships, all other methods require about double or triple the number of samples Mgc requires.
Dimensionality | One-Dimensional | Ten-Dimensional | ||||
---|---|---|---|---|---|---|
Dependency type | Monotone | Non-Mono | Average | Monotone | Non-Mono | Average |
Mgc | 1 | 1 | 1 | 1 | 1 | 1 |
Dcorr | 1 | 2.6 | 2.2 | 1 | 3.2 | 2.6 |
Mcorr | 1 | 2.8 | 2.4 | 1 | 3.1 | 2.6 |
Hhg | 1.4 | 1 | 1.1 | 1.7 | 1.9 | 1.8 |
Hsic | 1.4 | 1.1 | 1.2 | 1.7 | 2.4 | 2.2 |
Mantel | 1.4 | 1.8 | 1.7 | 3 | 1.6 | 1.9 |
Pearson / Rv / Cca | 1 | >10 | >10 | 0.8 | >10 | >10 |
Spearman / Kendall | 1 | >10 | >10 | n/a | n/a | n/a |
Mic | 2.4 | 2 | 2.1 | n/a | n/a | n/a |
-
Table 1—source data 1
Testing power sample size data in one dimension.
- https://doi.org/10.7554/eLife.41690.010
-
Table 1—source data 2
Testing power sample size data in high-dimensions.
- https://doi.org/10.7554/eLife.41690.011
The p-values for brain imaging vs mental properties.
Mgc always uncovers the existence of significant relationships and discovers the underlying optimal scales. Bold indicates significant p-value per dataset.
Testing Pairs/Methods | Mgc | Dcorr | Mcorr | Hhg | Hsic |
---|---|---|---|---|---|
Activity vs Personality | 0.043 | 0.667 | 0.441 | 0.059 | 0.124 |
Connectivity vs Creativity | 0.011 | 0.010 | 0.011 | 0.031 | 0.092 |
-
Table 2—source data 1
p-value data for activity vs personality.
- https://doi.org/10.7554/eLife.41690.016
-
Table 2—source data 2
p-value data for connetivity vs creativity.
- https://doi.org/10.7554/eLife.41690.017
Results for cancer peptide screening.
The first two rows report the p-values for the tests of interest based on all peptides. The next four rows report the number of significant proteins from individual peptide tests; the Benjamini-Hochberg procedure is used to locate the significant peptides by controlling the false discovery rate at 0.05.
Testing pairs / Methods | Mgc | Mantel | Dcorr | Mcorr | Hhg | |
---|---|---|---|---|---|---|
1 | Ovar vs. Norm: p-value | 0.0001 | 0.0001 | 0.0001 | 0.0001 | 0.0001 |
2 | Ovar vs. Norm: # peptides | 218 | 190 | 186 | 178 | 225 |
3 | Pancr vs. Norm: p-value | 0.0082 | 0.0685 | 0.0669 | 0.0192 | 0.0328 |
4 | Panc vs. Norm: # peptides | 9 | 7 | 6 | 7 | 11 |
5 | Panc vs. All: # peptides | 1 | 0 | 0 | 0 | 3 |
6 | # peptides unique to Panc | 1 | 0 | 0 | 0 | 2 |
7 | # false positives for Panc | 0 | n/a | n/a | n/a | 1 |
-
Appendix 1—table 1—source data 1
Ovarian testing results.
- https://doi.org/10.7554/eLife.41690.021
-
Appendix 1—table 1—source data 2
Pancreatic testing results.
- https://doi.org/10.7554/eLife.41690.022
-
Appendix 1—table 1—source data 3
Peptide screening results for pancreatic.
- https://doi.org/10.7554/eLife.41690.023
For each of Mgc, Dcorr, Mcorr, Hhg, Hsic, Mantel, Pearson, and Mic, list the top four peptides identified for Panc vs All and the respective corrected p-value using Benjamini-Hochberg.
Bold indicates a significant peptide at type 1 error level 0.05. The top candidates are very much alike except Mic. In particular, neurogranin is consistently among the top candidates for all methods, but is only significant while using Mgc, Hsic, and Hhg; there are two other significant proteins from Hsic and Hhg, but they do not further improve the classification performance comparing to just using neurogranin. Note that the p-values from Mantel and Pearson are always 1 after Benjamini-Hochberg correction, so their respective top peptides are identified using raw p-values without correction.
method | Top four identified peptides | |||
---|---|---|---|---|
Mgc | neurogranin | fibrinogen protein 1 | tropomyosin alpha-3 | ras suppressor protein 1 |
p-value | 0.03 | 0.33 | 0.49 | 0.52 |
Dcorr | neurogranin | fibrinogen protein 1 | kinase 6 | twinfilin-2 |
p-value | 0.41 | 0.60 | 0.60 | 0.93 |
Mcorr | neurogranin | fibrinogen protein 1 | kinase 6 | tropomyosin alpha-3 |
p-value | 0.45 | 0.80 | 0.80 | 0.83 |
Hsic | neurogranin | tropomyosin alpha-3 | kinase 6 | tripeptidyl-peptidase 2 |
p-value | 0.01 | 0.01 | 0.09 | 0.09 |
Hhg | neurogranin | fibrinogen protein 1 | tropomyosin alpha-3 | platelet basic protein |
p-value | 0.03 | 0.03 | 0.03 | 0.11 |
Mantel | neurogranin | adenylyl cyclase | tropomyosin alpha-3 | alpha-actinin-1 |
p-value | 1 | 1 | 1 | 1 |
Pearson | neurogranin | adenylyl cyclase | tropomyosin alpha-3 | alpha-actinin-1 |
p-value | 1 | 1 | 1 | 1 |
Mic | kinase B | S100-A9 | ERF3A | thymidine |
p-value | 0.15 | 0.15 | 0.15 | 0.15 |
The actual testing time (in seconds) on real data.
https://doi.org/10.7554/eLife.41690.026Data | Personality | Creativity | Screening |
---|---|---|---|
Mgc | 2.5 | 7.5 | 1.9 |
Dcorr | 0.2 | 0.4 | 0.18 |
Hsic | 0.5 | 1.7 | 0.23 |
Hhg | 6.3 | 53.4 | 12.3 |
Pearson | NA | NA | 0.03 |
Mic | NA | NA | 0.1 |
Mrule |
Additional files
-
Transparent reporting form
- https://doi.org/10.7554/eLife.41690.018
-
Appendix 1—table 1—source data 1
Ovarian testing results.
- https://doi.org/10.7554/eLife.41690.021
-
Appendix 1—table 1—source data 2
Pancreatic testing results.
- https://doi.org/10.7554/eLife.41690.022
-
Appendix 1—table 1—source data 3
Peptide screening results for pancreatic.
- https://doi.org/10.7554/eLife.41690.023