Spike-timing-dependent ensemble encoding by non-classically responsive cortical neurons
Figures
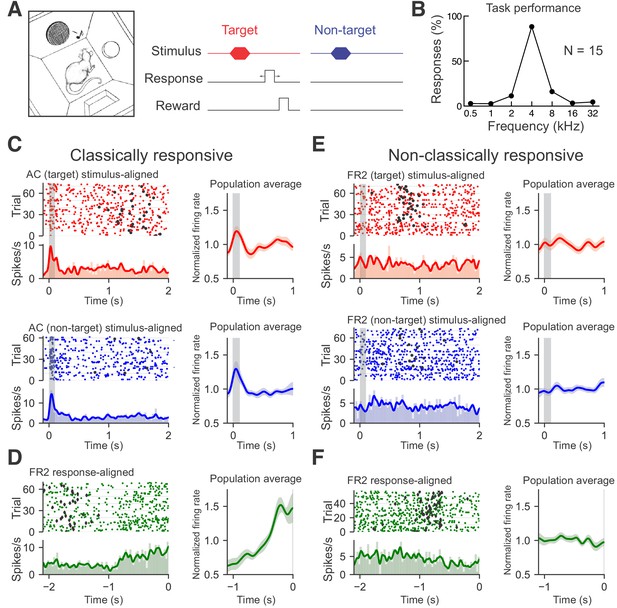
Recording from AC or FR2 during go/no-go audiomotor task.
(A) Behavioral schematic for the go/no-go frequency recognition task. Animals were rewarded with food for entering the nose port within 2.5 s after presentation of a target tone (4 kHz) or given a 7 s time-out if they incorrectly responded to non-target tones (0.5, 1, 2, 8,16, or 32 kHz). (B) Behavioral responses (nose pokes) to target and non-target tones (hit rates: 88 ± 7%, false alarms: 7 ± 5%, N = 15 rats). (C) Left, AC unit with significant tone modulated responses during target trials (red; top panel, average evoked spikes = 0.55) and non-target trials (blue; bottom panel, average evoked spikes = 0.92). Rasters of individual trials as well as the firing rate histogram and moving average are shown. Histograms of average firing rate during a trial were constructed using 25 ms time bins. A moving average of the firing rate was constructed using a Gaussian kernel with a 20 ms standard deviation. Black circles represent behavioral responses. Right, population averages for all target (n = 23) or nontarget (n = 34) classically responsive singe-units from AC. (D) Left, FR2 unit with ramping activity (green; ramp index = 2.82). Trials here are aligned to response time. Diamonds indicate stimulus onset. Right, population average for all ramping single-units from FR2 (n = 21). (E) Left, FR2 unit that was not significantly modulated during target trials (red; average evoked spikes = 0.041, p<0.001, 2000 bootstraps). Black circles here represent behavioral responses. Right, population averages for all target (n = 44) or non-target (n = 44) non-classically responsive single-units from FR2 (F) Left, FR2 unit lacking ramping activity (green, ramp index = −1.0, p<0.001, 2000 bootstraps). Right, population average for all non-ramping single-units from FR2 (n = 44).
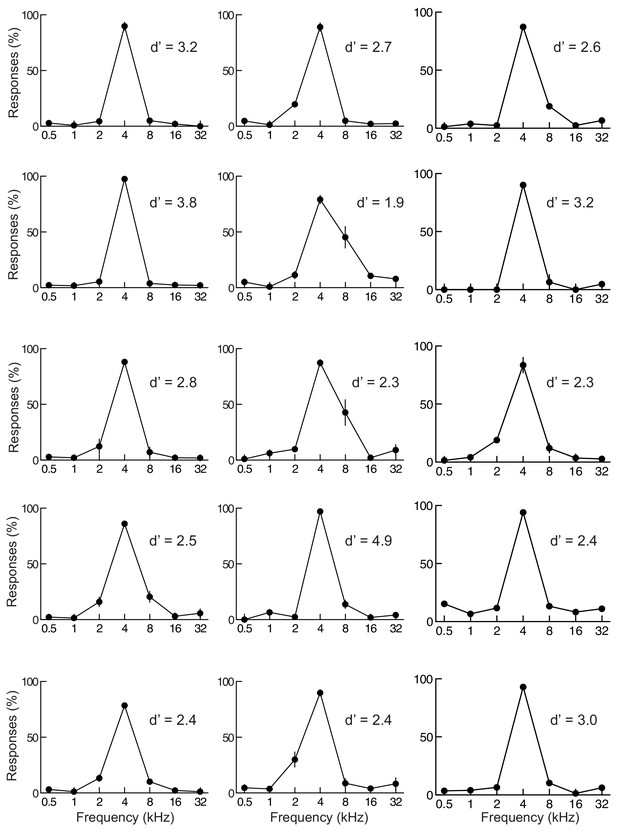
Individual response curves from 15 animals included in this study.
Each panel shows data from a different animal including behavioral d' for distinguishing target from non-target tones. We used a criteria of d'≥1 for inclusion in this study. Response curves here are for an average of three to four sessions. Error bars represent S.E.M.
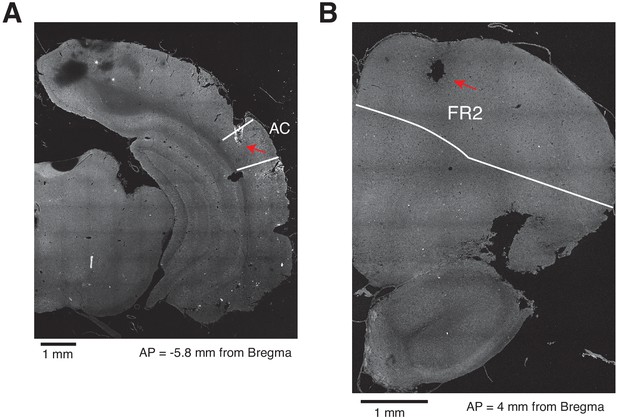
Histological placement of cannulas in AC and FR2.
(A) Example of a coronal section of a rat implanted with cannulas in primary auditory cortex (AC). The white lines represent the borders of AC38. (B) Example of a coronal section of a rat implanted with cannulas in FR2. The white lines represent the borders of FR238.
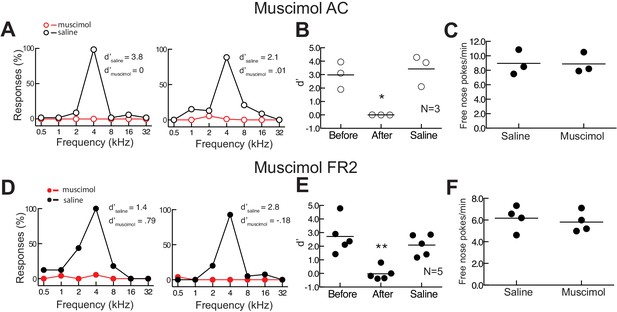
Bilateral infusion of muscimol into either AC or FR2 significantly impairs task performance.
(A) Behavioral performance after muscimol infusion (red) or saline control (black) in AC from two individual animals. (B) Summary of performance on day before infusion, after muscimol infusion into AC, and after saline control infusion (N = 3 animals). Performance was impaired after muscimol infusion (p=0.03 Student’s paired two-tailed t-test, *p<0.05). (C) Behavior of one animal allowed to freely nose poke for food without tones being presented. This behavior was not affected by muscimol inactivation (average of three sessions, p>0.99 Wilcoxon matched-pairs signed rank test). Error bars represent S.E.M. (D) Behavioral performance for two animals infused bilaterally with muscimol into FR2. (E) Summary of performance before, during, and after muscimol infusion into FR2 (N = 5 animals). Performance was impaired after muscimol infusion (p=0.009 Student’s paired two-tailed t-test, **p<0.01). (F) Muscimol in FR2 did not impair free nose poking for food without tones being presented in two animals (average of four sessions, p=0.62, Wilcoxon matched-pairs signed rank test).
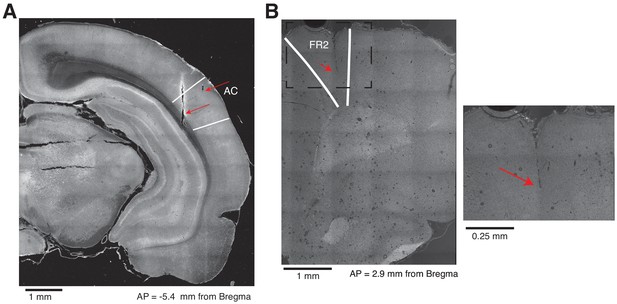
Histological placement of electrodes in AC and FR2.
(A) Example of electrode tracks and electrolytic lesions in AC. The white lines represent the borders of AC. (B) Example of an electrode track in FR2. The white lines represent the borders of FR2. Left, section imaged at 10X. Right, the same section imaged at 40X.
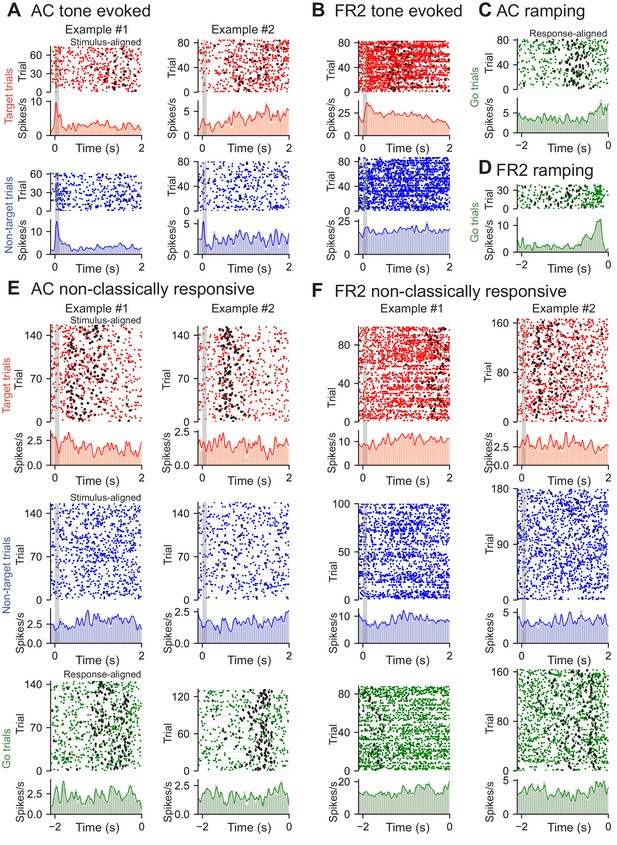
Examples of tone-evoked, ramping, and non-classically responsive cells from AC and FR2.
(A) Two example tone-evoked cells recorded from AC. Rasters and PSTHs of target (red) and non-target (blue) trials shown. Stimulus shown as grey bar and black circles represent behavioral response. (Example #1: average evoked spikes on target tones = 0.55, on non-target = 0.92. Example #2: average evoked spikes on target tones = 0.096, on non-target = 0.12; note that example #2 is only non-target tone evoked). (B) Example target tone-evoked cell recorded from FR2. Rasters and PSTHs of target (red) and non-target (blue) trials shown (average evoked spikes on target tones = 0.37, on non-target = 0.20). (C) Example ramping cell recorded from AC. Rasters and PSTH of go trials (green) shown (ramp index = 2.8). (D) Example ramping cell recorded from FR2 (ramp index = 4.9). Rasters and PSTH of go trials (green) shown. (E) Two example non-classically responsive cells recorded from AC. Rasters and PSTH of target (red), non-target (blue), and go (green) trials shown. (Example #1: average evoked spikes on target tones = 0.12, on non-target = −0.12, ramp index = −0.85; ptone <0.001, pramp = 0.010, 2000 bootstraps; Example #2: average evoked spikes on target tones = −0.020, on non-target = −0.038, ramp index = 1.1; ptone <0.001, pramp = 0.004, 2000 bootstraps). (F) Two example non-classically responsive cells recorded from FR2 (Example #1: average evoked spikes on target tones = 0.081, on non-target = −0.15, ramp index = 1.4; ptone <0.001, pramp = 0.019, 2000 bootstraps; Example #2: average evoked spikes on target tones = 0.046, on non-target = −0.070, ramp index = 1.8; ptone <0.001, pramp = 0.033, 2000 bootstraps). Rasters and PSTH of target (red), non-target (blue), and go (green) trials shown.
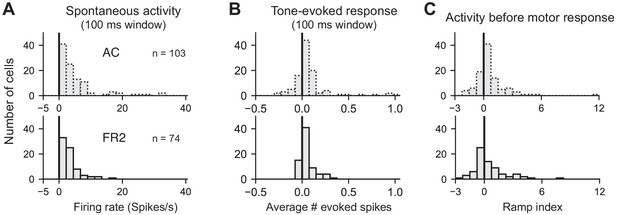
Summary statistics.
Histograms of (A) spontaneous firing rate, (B) average number of tone-evoked spikes for preferred stimulus category, and (C) ramp index for AC (top) and FR2 (bottom).
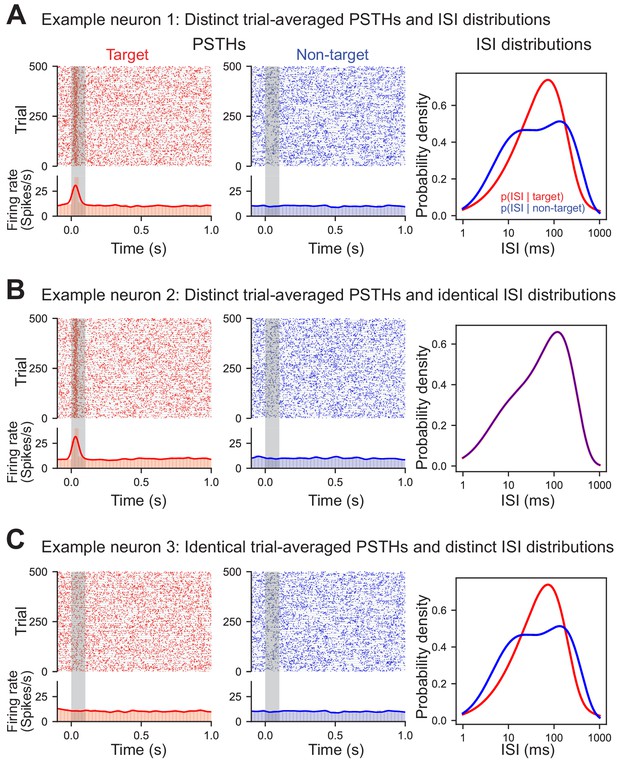
ISIs capture information distinct from trial-averaged rate.
Three simulated example neurons demonstrating that differences in the ISI are not necessary for differences in the trial-averaged firing rate to occur (and vice versa). Each trial was generated by randomly sampling from the appropriate conditional ISI distribution. Evoked responses were generated by shifting trials without altering the ISI distributions such that one spike during stimulus presentation is found at approximately 30 ms (with a variance of 10 ms). (A) Example neuron with both an evoked target response and a difference in the conditional ISI distributions on target and non-target trials. (B) Example neuron with an evoked target response but identical conditional ISI distributions. (C) Example non-classically responsive neuron with no distinct trial-averaged activity relative to the pre-stimulus period that nevertheless is generated by distinct ISI distributions.
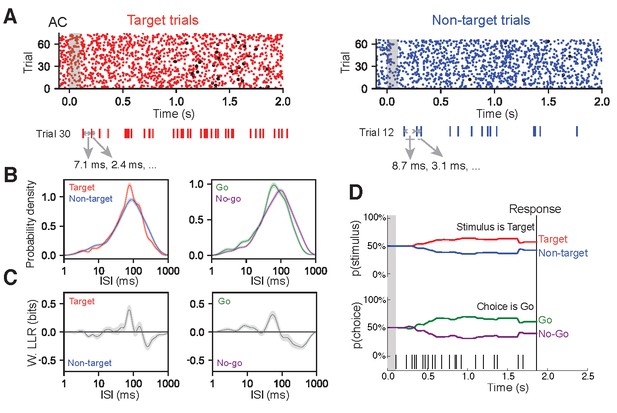
ISI-based algorithm for decoding behavioral variables from AC and FR2 single-units.
(A) Single-unit activity was first sorted by task condition, here for target trials (red) and non-target trials (blue). All ISIs following stimulus onset and before behavioral choice were aggregated into libraries for each condition (average response time is used on no-go trials) as shown for a sample trial. (B) Probability density for observing a given ISI on each condition was generated via Kernel Density Estimation on libraries from (A) Left, target (red) and non-target (blue) probabilities. Right, go (green) and no-go (purple). (C) Relative differences between the two stimulus conditions (or choice conditions) was used to infer the actual stimulus category (or choice) from an observed spike train, in terms of weighted log likelihood ratio (W. LLR) for stimulus category (p(ISI)*(log2p(ISI|target) - log2(ISI|non-target)); on left) and behavioral choice (p(ISI)*(log p2(ISI|go) - log2(ISI|no-go)); on right). When curve is above zero the ISI suggests target (go) and when below zero the ISI suggests non-target (no-go). (D) Probability functions from B were used as the likelihood function to estimate the prediction of a spike train on an individual trial (bottom). Bayes’ rule was used to update the probability of a stimulus (top) or choice (bottom) as the trial progressed and more ISIs were observed. The prediction for the trial was assessed at the end of the trial as the probability of stimulus category (or choice). In this example trial, p(target|ISI)=61%.
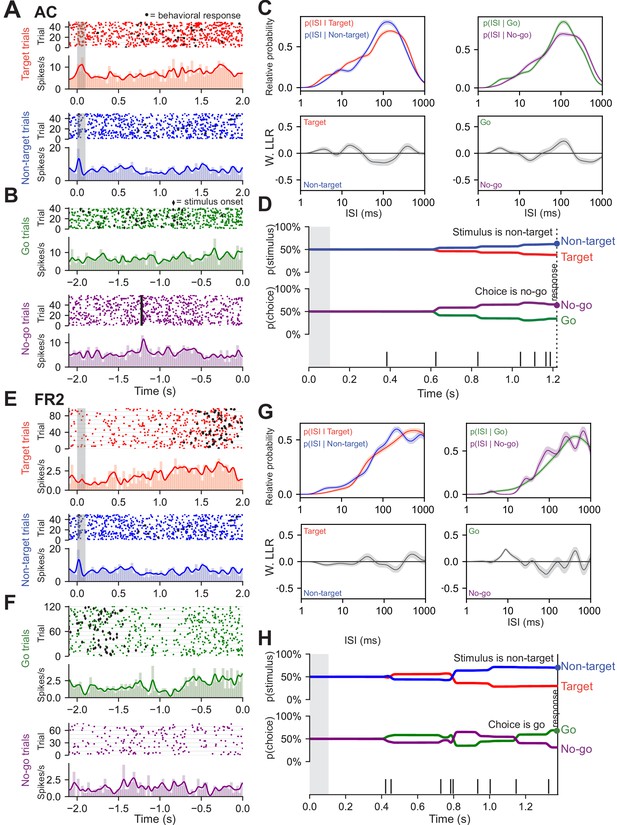
Decoding algorithm to determine stimulus category and choice in single-unit ISIs from AC and FR2 for two additional neurons.
(A–D) Decoding algorithm applied to a sample neuron in AC. (A) Single-unit activity sorted by stimulus condition: target trials (red) and non-target trials (blue). Black circles represent the behavioral response. (B) Trials aligned to behavioral response: go (green) and no-go (purple). Black diamonds in both go and no-go trials represent stimulus onset. (C) All ISIs during the trial (following stimulus onset and before behavioral choice) are aggregated into libraries for each condition (average response time is used on no-go trials). Probability of observing a given ISI on each condition was generated by using Kernel Density Estimation on the libraries from (A). Top left are target (red) and non-target (blue) probabilities and on right are go (green) and no-go (purple). Below left (right) are the log likelihood ratios (LLR) for the ISIs conditioned on stimulus category (behavioral choice). When curve is above zero the ISI suggests target (go); when it is below zero the ISI suggests non-target (no-go). (D) Probability functions from c were used as the likelihood function to estimate the prediction of a spike train on an individual trial (bottom). Bayes’ rule was used to update the probability of a stimulus (top) or choice (bottom) as the trial progresses and more ISIs were observed. Prediction for the trial was assessed at the end of the trial as depicted by the highlighted dot. (E–H) as in (A–D) except the decoding algorithm is applied to a neuron from FR2.
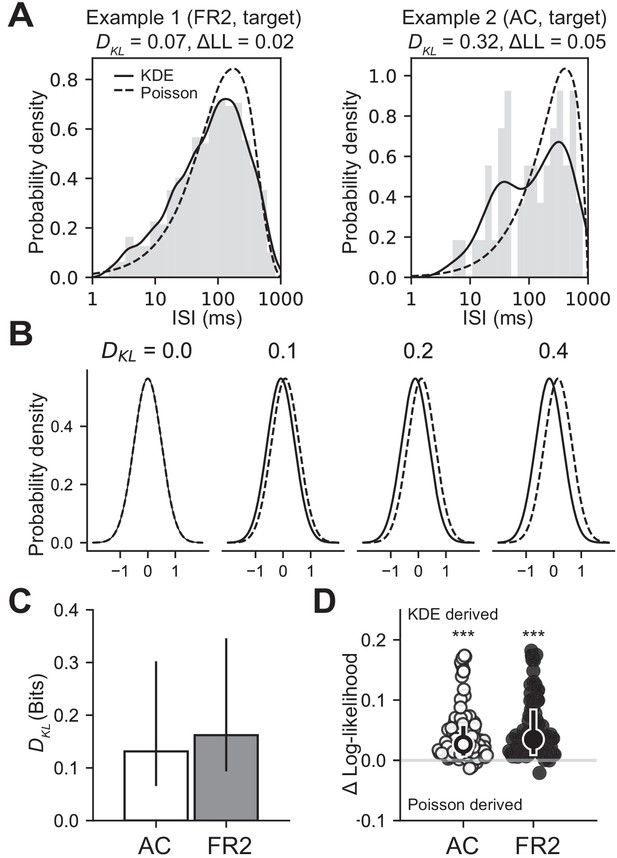
Empirical ISI distributions are better modeled using non-parametric methods.
(A) ISI histograms from two example cells on target trials with the corresponding non-parametric Kernel Density Estimate (KDE) distribution (solid lines) and the distribution derived from a rate-modulated Poisson process (dashed lines). Above each example is the Kullback-Leibler divergence (DKL) quantifying the difference between these two distributions, and the difference in the average log-likelihood of the data (ΔLL) where positive values indicate that the data is better described by the non-parametric KDE distribution. (B) Constructed examples of the KL divergence for four pairs of normal distributions with equal standard deviations and various mean offsets as a visual reference (C) Summary of all KL divergence values for both stimulus and choice in AC (white) and FR2 (grey). Bar indicates median and error bars indicate bottom and top quartiles. (D) Summary of difference between log-likelihood of observed data under non-parametric KDE and rate-modulated Poisson distributions. Positive values indicate KDE distributions are generically a superior fit for the data (AC: p=1.1 × 10−15 FR2: p=1.2 × 10−16, Wilcoxon signed-rank test).
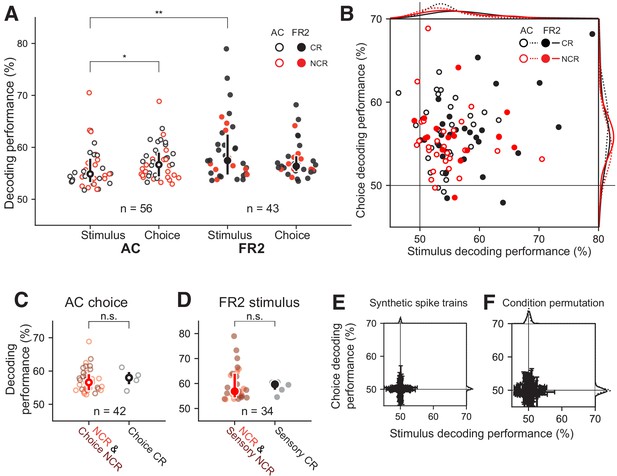
Decoding performance of single-units recorded from AC or FR2.
(A) Decoding performance of single-units for stimulus category and behavioral choice in AC (open circles) and FR2 (filled circles) restricted to those statistically significant relative to synthetically-generated spike trains (p<0.05, permutation test, two-sided). Note that decoding performance values reflect the algorithm’s prediction certainty on individual trials. Central symbol with error bars represents group medians and top and bottom quartiles (*p=0.02, **p=0.001, Mann-Whitney U test, two-sided). Black symbols, classically responsive cells; red symbols, non-classically responsive cells. (B) Weighted decoding performance for choice versus stimulus, restricted to those statistically significant relative to synthetically generated spike trains for either stimulus, choice, or both (p<0.05, permutation test, two-sided). Black symbols, classically responsive cells; red symbols, non-classically responsive cells. This performance metric is weighted by the prediction certainty on individual trials and can be thought of as a confidence measure. (C) Choice decoding performance in AC of non-classically responsive cells (red) and choice non-classically responsive (dark-red) versus choice classically responsive cells (black; i.e. ramping cells). Decoding performance was not statistically different (p=0.32 Mann-Whitney U test, two-sided). Central symbol with error bars represents group medians and top and bottom quartiles. (D) Stimulus decoding performance in FR2 for non-classically responsive cells (red) and sensory non-classically responsive (dark-red) versus choice responsive cells (black; i.e. ramping cells). Decoding performance was not statistically different (p=0.29, Mann-Whitney U test, two-sided). Central symbol with error bars represents group medians and top and bottom quartiles. (E) Decoding performance for choice versus stimulus, applied to spike trains synthetically generated from sampling (with replacement) over all ISIs observed without regard to stimulus category or behavioral choice. Black, classically responsive cells; red, non-classically responsive cells. Error bars represent standard deviation. (F) Decoding performance for choice versus stimulus, applied to spike trains left intact but trial conditions (stimulus category and behavioral choice) were randomly permuted (1000 permutations per unit). Error bars represent standard deviation.
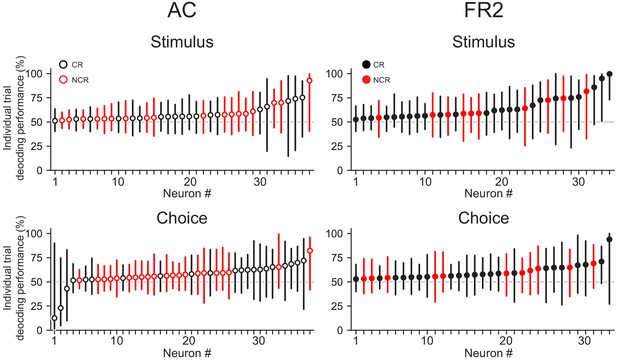
Decoding performance of single cells on individual trials.
Median decoding performance on individual trials with error bars denoting top and bottom quartiles. Neurons ordered according to median performance for each region and task variable. Black symbols, classically responsive units; red symbols, non-classically responsive units.
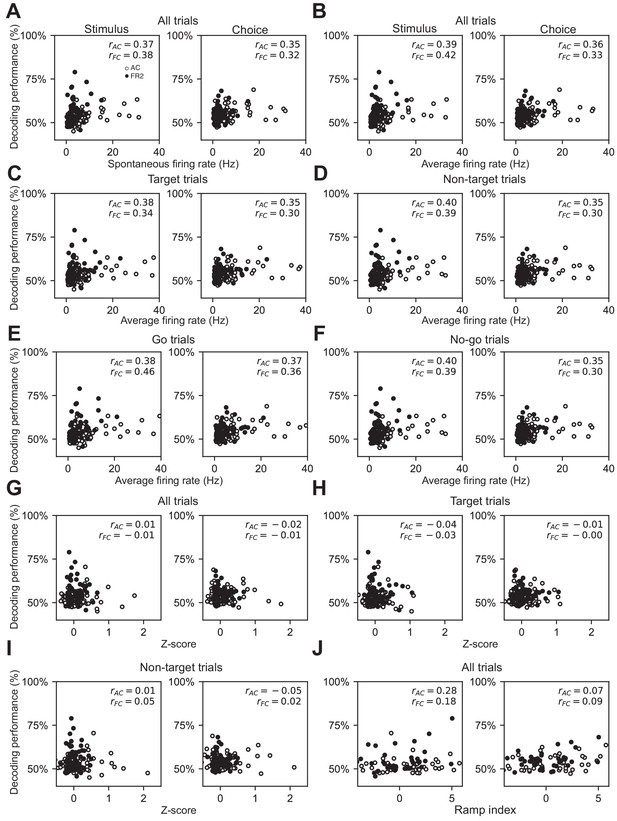
Lack of correlations between classical firing rate metrics and stimulus or choice decoding performance.
(A) Stimulus and choice decoding performance versus the spontaneous firing rate for both target and non-target trials, (rAC = 0.37, 0.35; slopeAC = 2.3 × 10−3, 2.2×10−3; pAC = 1.7 × 10−4, 4.1 × 10−4; rFR2 = 0.38, 0.30; slopeFR2 = 8.9 × 10−3, 4.6 × 10−3; pFR2 = 8.2 × 10−4, 6.6 × 10−3). (B) Stimulus and choice decoding performance versus average firing rate for both target and non-target trials, (rAC = 0.39, 0.36; slopeAC = 2.3 × 10−3, 2.1 × 10−3; pAC = 7.8 × 10−5, 3.0 × 10−4; rFR2 = 0.42, 0.33; slopeFR2 = 8.7 × 10−3, 4.5 ×10−3; pFR2 = 2.3 × 10−4, 3.8 × 10−3). (C) Stimulus and choice decoding performance versus average firing rate for target trials only, (rAC = 0.38, 0.35; slopeAC = 1.9 × 10−3, 1.8 × 10−3; pAC = 1.0 × 10−4, 4.2 × 10−4; rFR2 = 0.34, 0.30; slopeFR2 = 5.4 × 10−3, 3.0 × 10−3; pFR2 = 3.2 × 10−3, 0.011). (D) Stimulus and choice decoding performance versus average firing rate for non-target trials only, (rAC = 0.40, 0.35; slopeAC = 2.2 × 10−3, 1.9 × 10−3; pAC = 4.0 × 10−5, 6.6 × 10−4; rFR2 = 0.39, 0.30; slopeFR2 = 5.3 × 10−3, 2.5 × 10−3; pFR2 = 2.9 × 10−4, 0.010). (E) Stimulus and choice decoding performance versus average firing rate for go trials only, (rAC = 0.38, 0.37; slopeAC = 1.9 × 10−3, 1.9 × 10−3; pAC = 1.4 × 10−4, 2.3 × 10−4; rFR2 = 0.46, 0.36; slopeFR2 = 7.6 × 10−3, 3.8 × 10−3; pFR2 = 3.6 × 10−5, 2.0 × 10−3). (F) Stimulus and choice decoding performance versus average firing rate for no-go trials only, (rAC = 0.40, 0.35; slopeAC = 2.1 × 10−3, 1.9 ×10−3; pAC = 6.1 × 10−5, 4.7 × 10−4; rFR2 = 0.39, 0.30; slopeFR2 = 7.5 × 10−3, 3.7 × 10−3; pFR2 = 5.9 × 10−4, 9.2 ×10−3). (G) Stimulus and choice decoding performance versus z-score for all trials, (rAC = 0.01,–0.02; slopeAC = 1.3 × 10−3, −2.4 × 10−3; pAC = 0.91, 0.83; rFR2 = 0.01,–0.01; slopeFR2 = −2.3 × 10−3, −1.8 ×10−3; pFR2 = 0.95, 0.94). (H) Stimulus and choice decoding performance versus z-score for target trials only, (rAC = −0.04,–0.01; slopeAC = −5.1 × 10−3, −1.6 ×10−3; pAC = 0.72, 0.91; rFR2 = −0.03,–0.002; slopeFR2 = −6.7 × 10−3, −1.9 × 10−4; pFR2 = 0.81, 0.99). (I) Stimulus and choice decoding performance versus z-score for non-target trials only, (rAC = 0.01,–0.05; slopeAC = 1.0 × 10−3, −4.3 × 10−3; pAC = 0.90, 0.61; rFR2 = 0.05, 0.02; slopeFR2 = 0.017, 4.3 × 10−3; pFR2 = 0.68, 0.87). (J) Stimulus and choice decoding performance versus ramp index, (rAC = 0.28, 0.07; slopeAC = 5.1 × 10−4, 1.3 × 10−4; pAC = 5.9 × 10−3, 0.49; rFR2 = 0.18, 0.09; slopeFR2 = 4.5 × 10−4, 1.4 × 10−4; pFR2 = 0.13, 0.47).
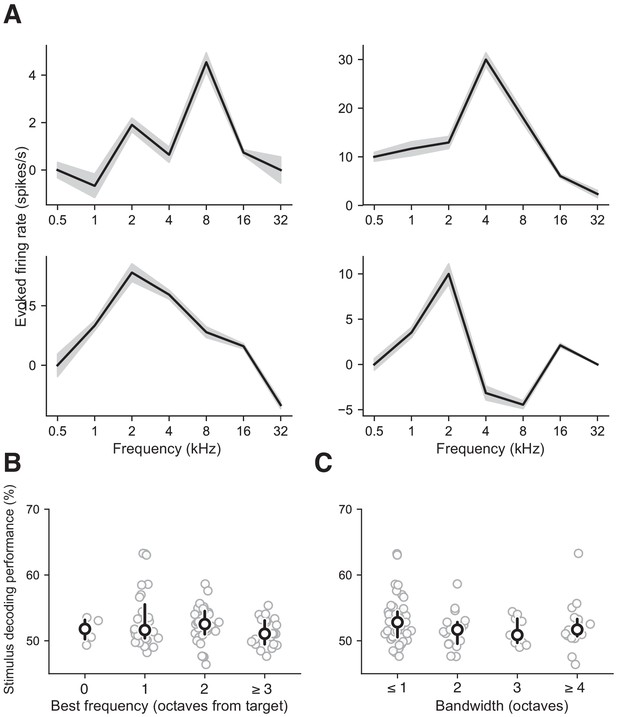
Stimulus decoding in AC independent of receptive field properties.
(A) Examples of tuning curves from four different neurons constructed from responses in AC. Gray regions represent S.E.M. (B) Stimulus decoding performance as a function of best frequency as measured relative to the target tone frequency. No significant differences were found between groups (p>0.2, Mann Whitney U test, two-sided). (C) Stimulus decoding performance as a function of receptive field bandwidth tuning. No significant differences were found between groups (p>0.1, Mann Whitney U test, two-sided).
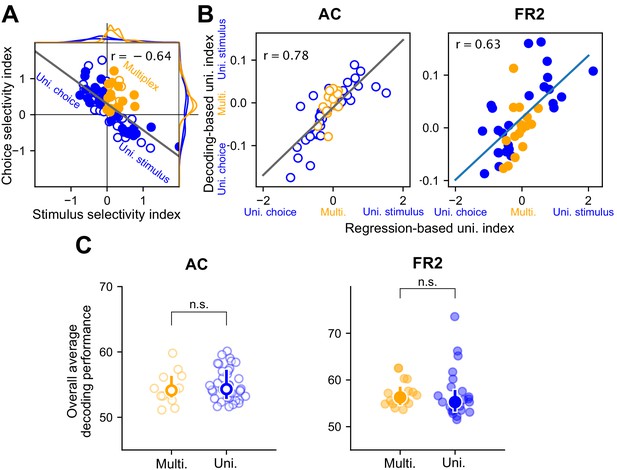
Decoding performance is a sufficient measure of uni/multiplexing.
Given the correlation between stimulus category and behavioral choice we used a regression based analysis to determine whether decoding performance alone was sufficient to establish whether cells were multiplexed for both behavioral variables. We used multiple regression to create an alternative definition of multiplexing and uniplexing and then demonstrated this definition coincides with the one used in the paper based solely on decoding performance. (A) Choice selectivity index versus stimulus selectivity index for single cells. Each index quantifies the extent to which the corresponding variable was predictive of decoding performance. Multiplexed cells (orange symbols) have positive values on both indices. Uniplexed cells (blue symbols) are only positive for one of the two indices. Each cell was projected on the linear regression (grey line) to construct a regression-based uniplexing index. Multiplexed cells were close to zero on this measure and cells uniplexed for stimulus or choice were positive or negative, respectively. (B) The decoding-based uniplexing index (difference between stimulus and choice decoding performance) versus the regression-based index defined in a for AC (left, open symbols) and FR2 (right, filled symbols). In both regions, these two measures of uni/multiplexing were correlated. (C) Overall decoding performance (average of stimulus and choice decoding) for multiplexed cells versus uniplexed cells in AC (left) and FR2 (right). There were no systematic differences in decoding performance between multiplexed and uniplexed units (n.s. pAC = 0.22, pFR2 = 0.11, Mann-Whitney U test, two-sided).
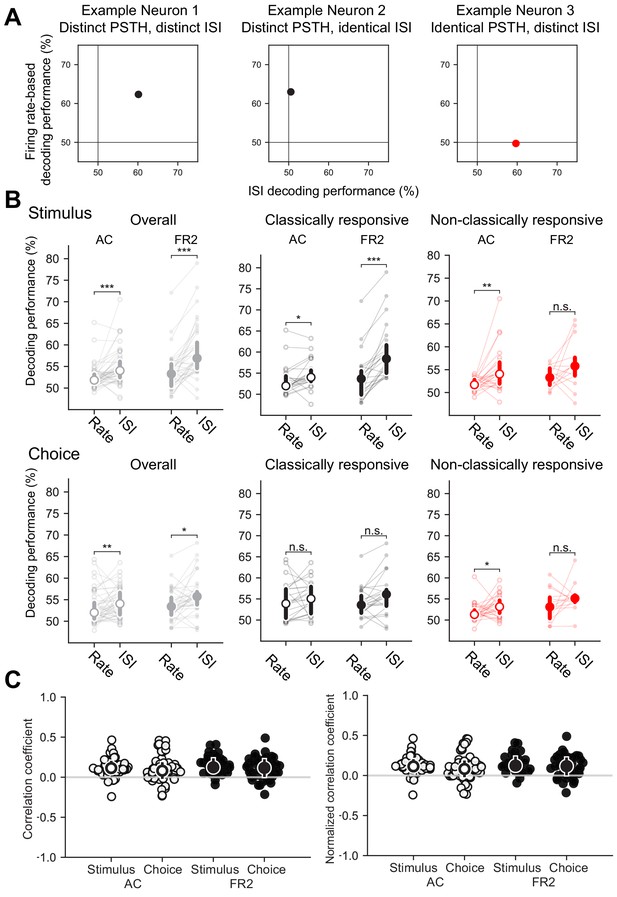
Information captured by ISI-based decoder distinct from conventional rate-modulated (inhomogeneous) Poisson decoder.
(A) Decoding performance comparison for example neurons shown in Figure 2. Left, Both the trial-averaged firing rate and the ISI distributions can be used to decode stimulus category for this example neuron. Middle, Only the firing rate can be used to decode this example. Right, In this case, the ISI distributions can be used to decode even when the trial-averaged firing rate cannot. (B) Comparison of decoding performance for conventional rate-modulated decoder to our ISI-based decoder. Top row, stimulus decoding, bottom row, choice decoding. Left, Overall comparison for all cells. Right, Comparison for classically responsive and non-classically responsive cells (Stimulus Overall: ***pAC = 0.0001, ***pFR2 = 8 × 10−6, Stimulus Repsonsive: *pAC = 0.031, ***pFR2 = 4 × 10−5, Stimulus non-classically responsive: **pAC = 0.0019, n.s. pFR2 = 0.096, Choice Overall: **pAC = 0.0057, *pFR2 = 0.02, Choice Repsonsive: n.s. pAC = 0.031, n.s. pFR2 = 0.08, Choice non-classically responsive: *pAC = 0.004, n.s. pFR2 = 0.19, Wilcoxon signed-rank test). Individual cells shown and median with error bars designating bottom and top quartiles superimposed. (C) Left, Matthews correlation coefficient (MCC) between correct predictions of our ISI-based decoder and a conventional rate-modulated firing rate decoder. A MCC value of 1 indicates each decoder correctly decodes exactly the same set of trials, whereas −1 indicates each decoder is correct on complementary trials. Values close to 0 indicate that that the relationship between the decoders is close to chance. Typically, values from −0.5 to 0.5 are considered evidence for weak to no correlation (stimulus median and interquartile range: AC = 0.10, 0.09, FR2 = 0.11, 0.12; choice median and interquartile range: AC = 0.06, 0.15, FR2 = 0.08, 0.17). Right, Matthews correlation coefficient (MCC) rescaled by the maximum possible correlation given the decoding performance of each method remains fixed. This control demonstrates that the correlation values are not a result of weak decoding performance for one of the decoding methods (stimulus median and interquartile range: AC = 0.11, 0.11, FR2 = 0.12, 0.15; choice median and interquartile range: AC = 0.08, 0.17, FR2 = 0.11, 0.19). Source data has been provided in the spreadsheet titled ‘figure_5.csv’.
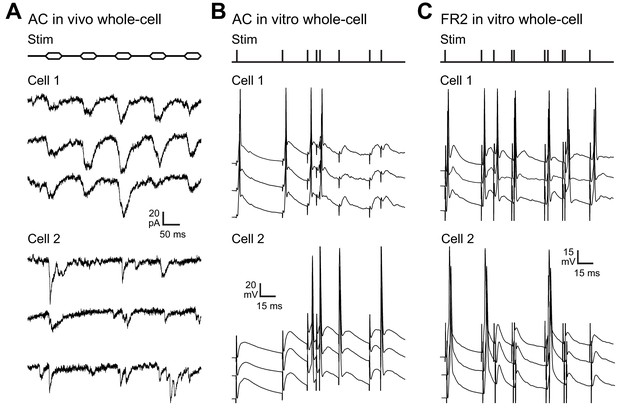
Whole-cell recordings from AC and FR2 neurons showing that different cells can have distinct responses to the same input pattern- necessary for ISI-based decoding by biological networks.
In each case, note the reliability of response across trials but differences in response patterns across cells. (A) Two of eight in vivo whole-cell recordings from anesthetized adult rat primary AC, presenting trains of pure tones at the best frequency for each cell (top, ‘Stim’). (B) Two of nine whole-cell recordings from adult rat AC in brain slices. Extracellular stimulation was used to present input patterns previously recorded from cortex with tetrode recordings in behaving rats during the auditory task used here, and responses recorded in current-clamp near spike threshold. (C) Two of 11 whole-cell recordings from adult rat FR2 in brain slices.
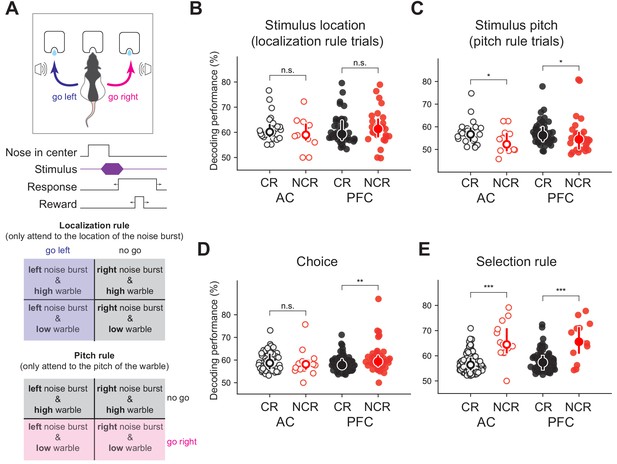
Non-classically responsive cells in both auditory cortex and prefrontal cortex (PFC) encode behavioral variables including the selection rule in a task switching paradigm.
(A) Schematic of novel auditory stimulus selection task. Animals were presented with two simultaneous tones (a white noise burst and warble) and trained to respond to the location of the sound in the ‘localization’ context while ignoring pitch and respond to the pitch while ignoring the location in the ‘pitch’ context (figure adapted from Rodgers and DeWeese, 2014), Neuron). Decoding performance for (B) stimulus localization on localization trials (pAC = 0.24, pPFC = 0.21, Mann-Whitney U test, two-sided), (C) stimulus pitch on pitch trials (pAC = 0.48, pPFC = 0.47, Mann-Whitney U test, two-sided), and (D) choice (**pAC = 0.0064, pPFC = 0.22, Mann-Whitney U test, two-sided) for classically responsive cells (black) and non-classically responsive cells (red; no stimulus modulation or ramping activity) in auditory (open symbols) and prefrontal cortex (closed symbols) previously reported but not further analyzed in this study. (E) Decoding performance for the selection rule for classically responsive (black) and non-classically responsive cells (red; similar pre-stimulus firing rates for both pitch and localization blocks; ***pAC = 5 × 10−6, ***pPFC <0.0002, Mann-Whitney U test, two-sided).
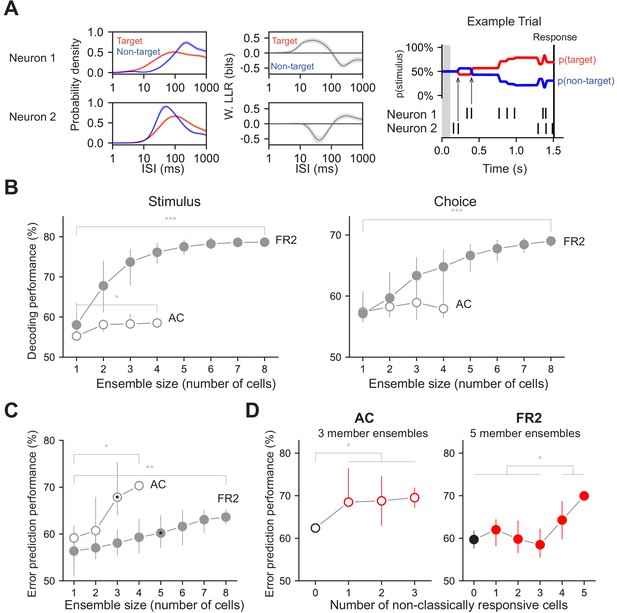
Decoding performance of neuronal ensembles recorded in AC or FR2.
(A) Schematic of ensemble decoding. Left, conditional ISI distributions and corresponding weighted LLR shown for two simultaneously recorded neurons. Right, an example trial where each neuron’s ISIs and LLRs are used to independently update stimulus category according to Bayes’ rule. Arrows indicate the first updates from each neuron. (B) Stimulus and choice decoding performance for ensembles in AC and FR2 for ensembles of increasing size (Comparing smallest with largest ensembles. Stimulus: *pAC = 0.04, ***pFR2 = 1 × 10−5, Choice: pAC = 0.29, ***pFR2 = 7 × 10−5, Mann-Whitney U test, two-sided). (C) Error prediction performance in AC and FR2 as a function of ensemble size (*pAC = 0.03, **pFR2 = 0.002; comparison between AC and FR2, for 3-member ensembles: p=1.2 × 10−5, for 4-member ensembles: p=0.03, Mann-Whitney U test, two-sided). Chance performance is 50%. (D) Error prediction performance in AC and FR2 as a function of the number of non-classically responsive cells in the ensemble (*pAC = 0.037, Welch’s t-test with Bonferroni correction for multiple comparisons; *pFR2 = 0.015, Student’s t-test with Bonferroni correction), 3 and 5 member ensembles in c. shown for AC and FR2 respectively. Chance performance is 50%.
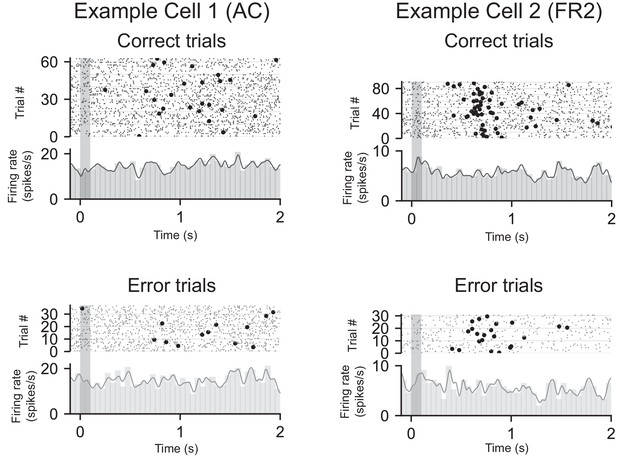
PSTHs from two example cells recorded in either AC or FR2 separated by correct (top) and error (bottom) trials.
All PSTHs are stimulus-aligned. The grey bar indicates stimulus presentation and circles represent behavioral response.
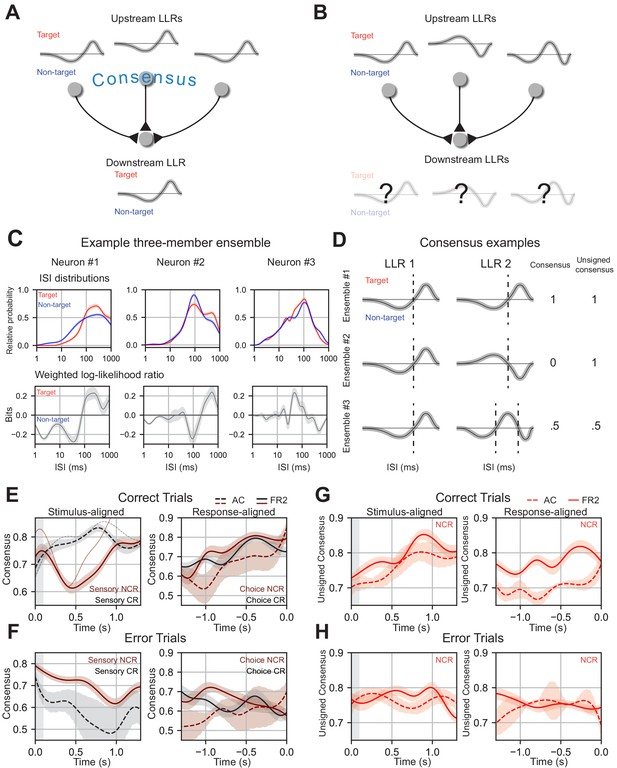
.
Ensemble consensus-building during behavior. (A) Schematic of consensus building in a three-member ensemble. When the LLRs of ensemble members are similar the meaning of any ISI is unambiguous to a downstream neuron. (B) Schematic of a three-member ensemble without consensus. The meaning of an ISI depends on the upstream neuron it originates from. (C) ISI distributions, and LLRs for three members of a sample ensemble. Note that despite differences in ISI distributions, neuron #1 and neuron #2 have similar weighted log-likelihood ratios (ISIs > 200 ms indicate target, ISIs < 200 ms indicate non-target). (D) Consensus values for three illustrative two-member ensembles. Ensemble 1 members have identical LLRs, agreeing on the meaning of all ISIs (consensus = 1) and on how the ISIs should be partitioned (unsigned consensus = 1). Ensemble 2 contains cells with LLRs where the ISI meanings are reversed, disagreeing on meaning of the ISIs (consensus = 0) but still agree on how the ISIs should be partitioned (unsigned consensus = 1). Ensemble 3 contains two cells with moderate agreement about the ISI meanings and partitioning, leading to intermediate consensus and unsigned consensus values (0.5 for each). (E) Left, mean consensus as a function of time from tone onset (stimulus-aligned) on correct trials for three-member sensory classically responsive ensembles in AC (two or more members sensory classically responsive; black dotted line; n=11 ensembles) and sensory non-classically responsive ensembles in FR2 (two or more members sensory non-classically responsive; dark red solid line; n=101 ensembles). Standard deviation shown around each mean trendline. Thin solid and dotted line represent an individual consensus trajectory from FR2 and AC, respectively. FR2 sensory non-classically responsive cells consistently reached consensus and then diverged immediately after stimulus presentation (Δconsensus, t = 0 to 0.42 s, pSNR = 3.9×10−4 Wilcoxon test with Bonferroni correction, two-sided). AC classically responsive ensembles (black) increase consensus until 750 ms (Δconsensus, t = 0 to 0.81 s, pSR = 0.14 Wilcoxon test with Bonferroni correction, two-sided). Right, mean consensus as a function of time to behavioral response (response-aligned) on correct trials for three-member choice classically responsive ensembles (two or more members choice classically responsive; black) in FR2 (solid line; n=47 ensembles) and choice non-classically responsive (two or more members choice non-classically responsive; dark red) in AC (dotted line; n=11 ensembles) and FR2 (solid line; n=57 ensembles). Standard deviation shown around each mean trendline. On correct trials, choice classically responsive (black) and choice non-classically responsive ensembles (dark red) in both regions reached high consensus values ~500 ms before response (Δconsensus, t = -1.0 to 0.0 s, pCNR = 2.0×10−5, pCR = 0.12 Wilcoxon test with Bonferroni correction, two-sided). (F) As in e, but for error trials (Δconsensus, correct vs. error trials, stimulus: pSNR= 0.007, pSR = 0.065, choice: pCNR = 0.0048, pCR = 0.065 Mann-Whitney U test, two-sided). (G) Unsigned consensus index for non-classically responsive ensembles (two or more members non-classically responsive) in AC (dotted line; n=13 ensembles) and FR2 (solid line; n=36 ensembles), stimulus-aligned (left, Δconsensus, t = 0 to 0.89 s, p = 5.1×10−5 Wilcoxon test with Bonferroni correction, two-sided) and response-aligned (right, Δconsensus, t = -1.0 to 0.0 s, p = 0.0033 Wilcoxon test with Bonferroni correction, two-sided). On correct trials, ensembles reach high values of unsigned consensus ~750 ms after tone onset and within 500 ms of behavioral response. (H) As in (G), but for error trials (Δconsensus, correct vs. error trials, p = 1.9×10−9 Mann-Whitney U test, two-sided). (E) – (G) Combinations analyzed and shown are those for which there are significant numbers in our dataset.
Tables
Number of classically responsive (CR) or non-classically responsive (NCR) neurons in AC and FR2 with significant stimulus or choice information.
https://doi.org/10.7554/eLife.42409.019# stimulus sig. | # choice sig. | Total # | ||
---|---|---|---|---|
AC | CR | 19 | 21 | 39 |
NCR | 18 | 21 | 64 | |
FR2 | CR | 20 | 22 | 31 |
NCR | 10 | 11 | 43 |
Reagent type (species) or resource | Designation | Source or reference | Identifiers | Additional information |
---|---|---|---|---|
Strain, strain background (Rattus norvegicus domesticus, males and females) | Sprague-Dawley, rats | Charles River, Taconic | NTac:SD | |
Chemical compound, drug | Muscimol | Sigma-Aldrich | InChi:ZJQHPWUVQPJPQT -UHFFFAOYSA-N; SID:24896662 | |
Software, algorithm | Single-trial Bayesian decoding algorithm | newly created | N/A | https://github.com/badralbanna/Insanally2017 |
Other | Rodgers and DeWeese, 2014 dataset | CRCNS | pfc-1 | http://crcns.org/data-sets/pfc/pfc-1 |
Additional files
-
Transparent reporting form
- https://doi.org/10.7554/eLife.42409.026