Unimodal statistical learning produces multimodal object-like representations
Figures
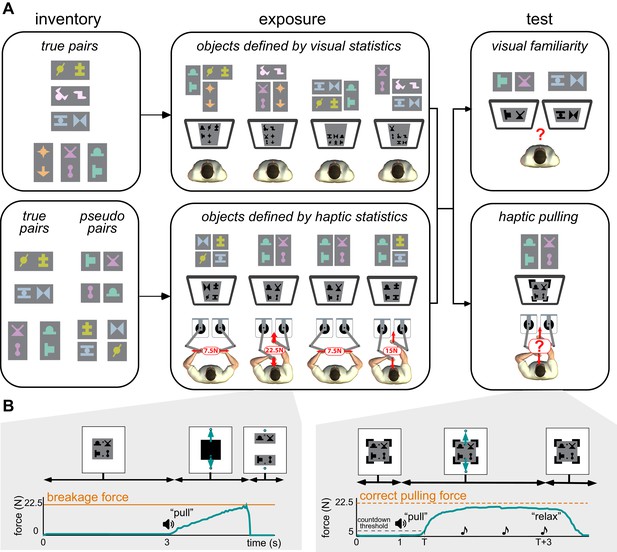
Experimental paradigm.
(A) Main phases of the experiments. Left. An inventory was constructed by arranging abstract shapes into horizontal and vertical pairs. True pairs behaved as objects: their shapes always appeared together, and in the same relative spatial configuration, and were hard to pull apart physically. Pseudo pairs served as controls: they had consistent visual statistics but were as easy to pull apart as two separate objects (indicated by the small separation between their shapes). Colouring and separation for illustration only, participants saw all shapes in grey-scale during exposure and testing, with no gaps between them, so that no visual cues separated the pairs of a compound scene (as shown on screens in the center and right panels). Center. During the exposure phase, participants experienced a sequence of visual scenes showing compound objects consisting of several pairs. The way the image displayed on the screen was constructed from the inventory is shown above each screen in colour for illustration. In the first experiment (top), participants observed compound scenes each constructed from three true pairs of the inventory. In the second experiment (bottom), on each trial, a compound scene consisting of two pairs (true or pseudo) was displayed and participants were required to pull the scenes apart in one of two directions as shown. A bimanual robotic interface (Howard et al., 2009) was used so that participants experienced the force at which the object broke apart (breakage force shown in red) but, crucially, visual feedback did not reveal the identity of true and pseudo pairs (see Materials and methods). Thus, only haptic information distinguished the true and pseudo pairs as the force required depended on the underlying structure of the scene. Right. In both experiments, participants finally performed two tests. First, in the haptic pulling test (bottom), participants were asked to pull with the minimal force which they thought would break apart a scene, composed of true or pseudo pairs (in both directions). We measured this force by ‘clamping’ the scene so that no haptic feedback was provided about the actual breakage force (black clamps at the corners of the scene). Crucially, the visual display also did not reveal the identity of true and pseudo pairs. Second, in the visual familiarity test (top), participants were asked to select which of two scenes presented sequentially appeared more familiar. One scene contained a true pair and the other a chimeric pseudo pair. Selecting the true pair counted as a correct response, but no feedback was given to participants as to the correctness of their choices. (B) Timeline of events in haptic exposure and test trials (displayed force traces are from representative single trials). Left. Haptic statistical exposure trials had scenes consisting of combinations of true and pseudo pairs of the inventory (top). After a fixed amount of time, the scene was masked (black square covering the scene), then pulling was initiated (‘pull’ instruction was played), and the scene was unmasked and shown as separated once the pulling force (green arrows and curve) exceeded the breakage force (orange line). Right. In the haptic pulling test, participants were asked to generate a pulling force which they thought would be just sufficient to break the scene apart (ideally the breakage force corresponding to the scene, orange dashed line). The scenes were constructed using the pairs of the inventory without any visible boundary between them and held together by virtual clamps at the corners of the scene (top). Pulling was initiated (‘pull’ instruction), and once the participant’s pulling force (green arrows and curve) exceeded a 5 N threshold (dashed black line), three beeps were played at 1 s intervals (notes). The clamps remained on until the end of the trial (top), so the scene never actually separated, and after the third beep (at which the pulling force was measured) participants were asked to ‘relax’. See Materials and methods for details of the variant used in the haptic exposure task.
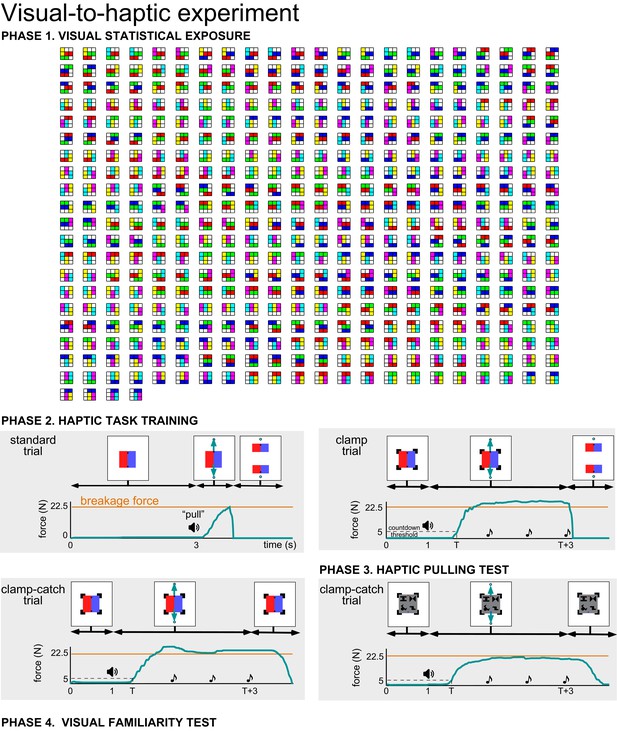
Phases of the visual statistical exposure experiment.
Phase 1. Visual statistical exposure consisted of 444 scenes (shown in a pseudorandom order), each using a combination of 3 true pairs of the inventory (see Figure 1A and Materials and methods). Each true pair is shown here as a uniquely coloured 2×1 block for illustrative purposes only and was replaced by true pair shapes in the experiment (assignment of shapes to colours was randomized across participants). The grid lines were not displayed in the experiment. Phase 2. Haptic task training consisted of two 2×1 rectangular coloured blocks (insets), which needed to be pulled apart in both the horizontal and vertical (shown) direction. On standard trials (upper left), participants were instructed to ‘pull’ (green arrows show applied forces, not movement) after the scene had been displayed for 3 s (black speaker icon), and the scene separated when the pulling force (green trace) exceeded the breakage threshold (orange line). The force was low for separating the block along their boundary and high when separating both coloured blocks into two (shown). On clamp trials (upper right) participants were asked to generate a pulling force which they thought would be just sufficient to break the scene apart. Clamps held the objects together initially. Once the participant’s pulling force exceeded a 5 N threshold (dashed line), three beeps were played at 1 s intervals, and the clamps were removed on the third beep. At that point the scene broke apart if the force exceeded the threshold, otherwise the participant had to increase their pulling force to break the scene. On clamp-catch trials (lower left) the clamps remained until the end of the trial and participants were asked to relax after the third beep. Phase 3. In the haptic pulling test (lower right), clamp-catch trials were used with scenes that were constructed using the (true) pairs of the inventory without any visible boundary between them. Phase 4. The visual familiarity test consisted of 72 trials (not shown, see Figure 1A and Materials and methods). In each trial, two scenes were displayed, one including a single true pair, the other (order counterbalanced) including a chimeric pseudo pair, and participants were asked to select the one that appeared more familiar to them. Green traces in 2 and 3 show the time course of the pulling force on representative trials for a single participant.
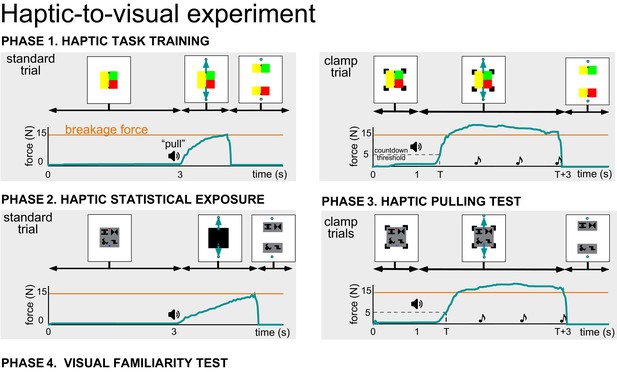
Phases of the haptic statistical exposure experiment.
Phase 1. Haptic task training consisted of different combinations of coloured blocks (2-4; see Materials and methods) and the breakage force (three levels) depended on the configuration. Standard and clamp trials were the same as in the visual statistical exposure experiment (Figure 1—figure supplement 1). Phase 2. Haptic statistical exposure consisted of standard trials with scenes consisting of the true and pseudo pairs of the inventory (see Figure 1A). Just prior to the initiation of pulling, the scene was masked and only unmasked when the pulling force exceeded the breakage threshold and the scene separated. Phase 3. Clamp trials in the haptic pulling test phase also used scenes consisting of true and pseudo pairs of the inventory. The scene was only masked after the clamps were removed if the force was insufficient to separate the scene. The mask was removed once the scene was separated. The use of masks in haptic statistical exposure and pulling test ensured that the time the scene was seen both together or apart was independent of the breakage force. Phase 4. The visual familiarity test consisted of 32 trials (not shown, see Figure 1A and Materials and methods) and was as described before (Figure 1A and Figure 1—figure supplement 1).
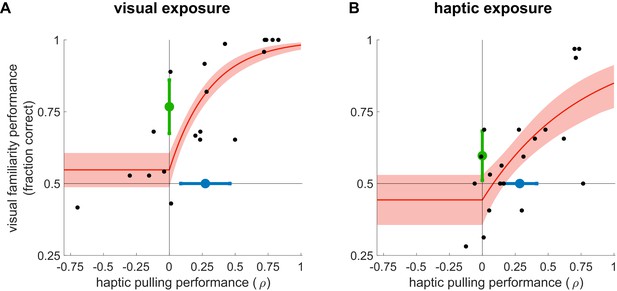
Learning from exposure to visual (A) and haptic statistics (B).
Performance on the visual familiarity test against haptic pulling performance for individual participants (black dots) with rectified exponential-binomial fit (red ±95% confidence limits, see Materials and methods). Visual familiarity performance was measured by the fraction of correct responses (selecting true over pseudo pairs). Haptic pulling performance was quantified as the correlation coefficient (ρ) between the true breakage force and participants’ pulling force across test scenes. Average performance (mean ±95% confidence intervals) across participants in the two tasks is shown by coloured error bars (familiarity: green, pulling: blue). Vertical and horizontal lines show chance performance for visual familiarity and haptic pulling performance, respectively. Note that in the first experiment (A), the performance of two participants was identically high in both tasks, and thus their data points overlap in the top right corner of the plot.
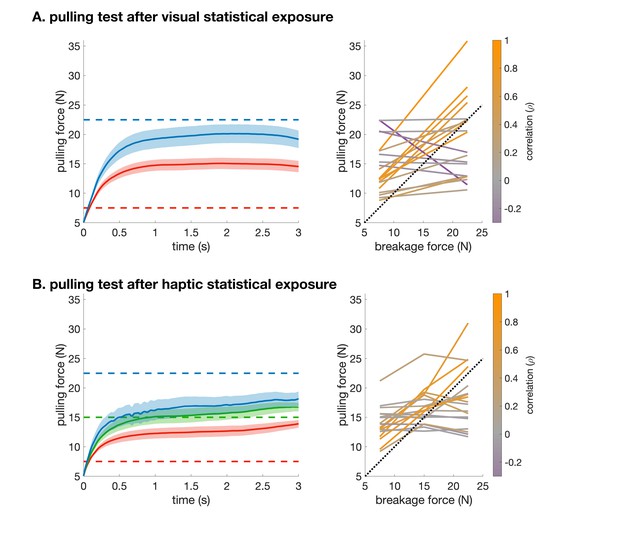
Pulling performance in the visual (A) and haptic (B) statistical exposure experiment.
(A) Left: Force traces from the start of pulling (5 N) on clamp-catch trials in the haptic pulling test of the visual statistical exposure experiment. Data shows mean ± s.e.m. across participants for trials in which the breakage force was high (blue) or low (red). Dashed lines show the corresponding breakage forces. Right: Average pulling force (at 3 s) vs. breakage force (two levels) for each participant colour coded by their correlation (across all trials). Dotted line shows identity. The average pulling force difference between the two levels was 9.3 N ± 3.3 N (s.e.m.). (B) as A for the clamp trials in the haptic pulling test of the haptic statistical exposure experiment, in which there were three levels of breakage force. The average pulling force difference between the low and medium breakage force levels was 5.4 N ± 1.2 N (s.e.m.), and between the medium and high breakage force levels was 2.8 N ± 1.7 N (s.e.m.). Raw data necessary to generate this figure was only saved for 18 participants.
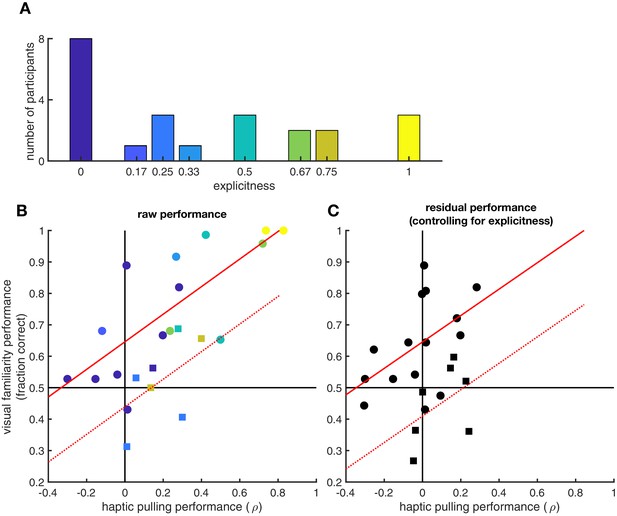
Effects of explicit knowledge on generalisation.
Participants’ explicit sense of knowledge was quantified as the proportion of true pairs they correctly identified (out of 6 in the visual statistical exposure and out of 4 in the haptic statistical exposure experiment, resulting in nine possible unique levels in total, out of which eight were realized) during a debriefing session following the experiment (N = 23 participants). (A) Histogram of explicitness across participants (average = 0.37). (B) Visual and haptic performance as in Figure 2, pooled across the two experiments for those participants who were debriefed (circles: visual statistical exposure, squares: haptic statistical exposure experiment). Colours show explicitness for each participant as in panel A. Red lines show linear regression assuming same slope but allowing for different average performances in the two experiments (solid: visual statistical exposure, dotted: haptic statistical exposure experiment): R = 0.84 (95% CI: 0.65–0.93), p=6.2⋅10−7. (C) Residual visual and haptic performance after controlling for explicitness (symbols as in panel B). In each experiment, both haptic and visual performance were regressed against explicitness. Residual performances in each modality were then computed by subtracting the performances predicted based on explicitness from the actual performances. Red lines show linear regression as in panel B: R = 0.69 (95% CI: 0.38–0.86), p=3.1⋅10−4.
Additional files
-
Transparent reporting form
- https://doi.org/10.7554/eLife.43942.008