Brain and Behavior: Assessing reproducibility in association studies
Scientists have always been eager to understand how complex thoughts and behaviors emerge from the intricate networks of neurons found in our brains. For instance, there appears to be a (weak) association between intelligence and total brain volume (Pietschnig et al., 2015), but also between intelligence and the dendritic size of pyramidal neurons (Goriounova et al., 2018). Yet, these relations do not provide a true insight into how individual differences in intelligence or in other behaviors emerge. Just as examining one component in a car, or weighing the whole car, will tell us relatively little about the overall performance of the vehicle, simply looking at individual neurons, or calculating the volume of a brain, will not tell the whole story about a person.
This is because brain areas and structures interact with each other and work in synergy to create and influence behavior. New techniques such as magnetic resonance imaging (MRI) have made it possible to start exploring the way a specific behavior trait is linked to the brain. In particular, many new associations between behavior and brain structure have been revealed with mass-univariate approaches, which divide the brain into small 3D units called voxels, and then map the relationship between behavior and each of these voxels using univariate statistical tests such as ANOVAs or t-tests (Ashburner and Friston, 2000; Kanai and Rees, 2011). However, it has been difficult to replicate some of the findings obtained through these methods (Figure 1).
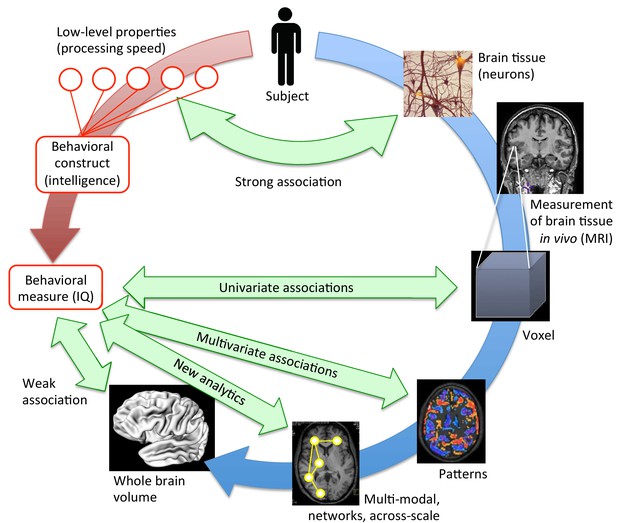
Bringing together brain structure and behavioral traits.
Several ways exist to examine how the structural properties of the brain (in blue) underpin complex behavioral traits such as intelligence (in red). At a low, neuronal level, some connections can be drawn between the characteristics of neurons and processing speed. To study more complex behavioral constructs, the brain can be examined at different levels. MRI scans provide averaged information about neural tissue throughout the brain at the scale of the millimeter (voxel; right, middle). This information can then be correlated with high-level behavioral measures using mass-univariate associations. While these structural brain -behavior associations are stronger than those obtained when looking at the whole brain volume (lower left), many have not been replicated (Kharabian Masouleh et al., 2019). However, multivariate analyses (lower right), and innovative, multi-modal analyses that work at the scale of networks (bottom), are expected to provide the strongest associations. They may hold the key to understanding how brain structure underpins psychological measures like intelligence.
Now, in eLife, Shahrzad Kharabian Masouleh, Simon Eickhoff, Felix Hoffstaedter and Sarah Genon from Research Centre Jülich and Heinrich Heine University Düsseldorf, along with the Alzheimer’s Disease Neuroimaging Initiative (ADNI), report new insights into these problems (Kharabian Masouleh et al., 2019). The team used common mass-univariate methods on two relatively large samples of 371 and 466 individuals to examine structural brain-behavior (SBB) associations with 36 psychological measures. These analyses revealed that it is difficult to pinpoint relationships between brain structures and behavioral traits, and that these relationships often occupy different locations between samples, making them hard to replicate.
What could explain and even fix this lack of reliability in SBB association studies? One issue is that psychological variables such as happiness or intelligence are complex constructs that rely on many different neuronal processes. Such diffuse measures may therefore yield unsteady correlations. In contrast, Kharabian Masouleh et al. showed that age yielded widespread and highly reproducible associations with brain structure. This may be because this ‘hard’, uncomplicated measure affects the organ consistently across different scales, from neurons to brain areas.
Another problem is that the statistical methods used in mass-univariate analyses cannot model the synergy between different parts of the brain, or that the brain may organize behaviors differently between individuals. An illustration of this limitation is that the current work failed to replicate association peaks, whereby small regions of brain tissue show important SBB associations (also discussed by Kanai, 2016). Instead, multivariate pattern recognition techniques can detect associations between behavior and structural patterns in the brain, making possible to identify groups of voxels that change together with variations in intelligence.
Nowadays, machine learning studies use these multivariate analyses. These new approaches can also independently select relevant features and take into account both interactions between brain structures and heterogeneity amongst individuals. In addition, it has become standard procedure to try to replicate results within the discovery set (by cross-validation) and in independent test samples (for example, Dwyer et al., 2018).
Using more data will also protect against irreproducibility and improve generalizability. Small samples are prone to chance findings, but large datasets help to reduce noise and sampling variance while also capturing more heterogeneity (Schnack and Kahn, 2016). Finally, recommendations point towards making as much information as possible public, for instance by publishing null findings and sharing raw data (as done by, for example, the UK Biobank). If this is not possible, the results of the analyses – the statistical brain maps – should be released so they can be used in meta-analyses, for example.
Voxel-based measures work at the scale of the millimeter and therefore ignore the details of the many neurons present in the voxels. On the other hand, it is now possible to zoom in on cortical layers using 3T MRI (Ferguson et al., 2018). This could be a first step towards examining the living brain with a resolution normally only accessible through post mortem research.
Further improvements could come from going beyond measuring volumes, for instance by starting to assess connectivity at different scales (Scholtens and van den Heuvel, 2018), by employing spectroscopic measures, or by combining the two. Yet, more rigorous innovations may still be necessary: to finally understand rich and multifaceted concepts, such as the emergence of intelligence, scientists will need to design equally complex approaches to analyze the brain in a more clever way.
References
-
Machine learning approaches for clinical psychology and psychiatryAnnual Review of Clinical Psychology 14:91–118.https://doi.org/10.1146/annurev-clinpsy-032816-045037
-
The structural basis of inter-individual differences in human behaviour and cognitionNature Reviews Neuroscience 12:231–242.https://doi.org/10.1038/nrn3000
-
Meta-analysis of associations between human brain volume and intelligence differences: how strong are they and what do they mean?Neuroscience & Biobehavioral Reviews 57:411–432.https://doi.org/10.1016/j.neubiorev.2015.09.017
-
Multimodal connectomics in psychiatry: bridging scales from micro to macroBiological Psychiatry: Cognitive Neuroscience and Neuroimaging 3:767–776.https://doi.org/10.1016/j.bpsc.2018.03.017
Article and author information
Author details
Publication history
Copyright
© 2019, Schnack
This article is distributed under the terms of the Creative Commons Attribution License, which permits unrestricted use and redistribution provided that the original author and source are credited.
Metrics
-
- 5,434
- views
-
- 242
- downloads
-
- 12
- citations
Views, downloads and citations are aggregated across all versions of this paper published by eLife.
Download links
Downloads (link to download the article as PDF)
Open citations (links to open the citations from this article in various online reference manager services)
Cite this article (links to download the citations from this article in formats compatible with various reference manager tools)
Further reading
-
- Computational and Systems Biology
- Neuroscience
Accumulating evidence to make decisions is a core cognitive function. Previous studies have tended to estimate accumulation using either neural or behavioral data alone. Here, we develop a unified framework for modeling stimulus-driven behavior and multi-neuron activity simultaneously. We applied our method to choices and neural recordings from three rat brain regions—the posterior parietal cortex (PPC), the frontal orienting fields (FOF), and the anterior-dorsal striatum (ADS)—while subjects performed a pulse-based accumulation task. Each region was best described by a distinct accumulation model, which all differed from the model that best described the animal’s choices. FOF activity was consistent with an accumulator where early evidence was favored while the ADS reflected near perfect accumulation. Neural responses within an accumulation framework unveiled a distinct association between each brain region and choice. Choices were better predicted from all regions using a comprehensive, accumulation-based framework and different brain regions were found to differentially reflect choice-related accumulation signals: FOF and ADS both reflected choice but ADS showed more instances of decision vacillation. Previous studies relating neural data to behaviorally inferred accumulation dynamics have implicitly assumed that individual brain regions reflect the whole-animal level accumulator. Our results suggest that different brain regions represent accumulated evidence in dramatically different ways and that accumulation at the whole-animal level may be constructed from a variety of neural-level accumulators.
-
- Genetics and Genomics
- Neuroscience
The central complex (CX) plays a key role in many higher-order functions of the insect brain including navigation and activity regulation. Genetic tools for manipulating individual cell types, and knowledge of what neurotransmitters and neuromodulators they express, will be required to gain mechanistic understanding of how these functions are implemented. We generated and characterized split-GAL4 driver lines that express in individual or small subsets of about half of CX cell types. We surveyed neuropeptide and neuropeptide receptor expression in the central brain using fluorescent in situ hybridization. About half of the neuropeptides we examined were expressed in only a few cells, while the rest were expressed in dozens to hundreds of cells. Neuropeptide receptors were expressed more broadly and at lower levels. Using our GAL4 drivers to mark individual cell types, we found that 51 of the 85 CX cell types we examined expressed at least one neuropeptide and 21 expressed multiple neuropeptides. Surprisingly, all co-expressed a small molecule neurotransmitter. Finally, we used our driver lines to identify CX cell types whose activation affects sleep, and identified other central brain cell types that link the circadian clock to the CX. The well-characterized genetic tools and information on neuropeptide and neurotransmitter expression we provide should enhance studies of the CX.