During hippocampal inactivation, grid cells maintain synchrony, even when the grid pattern is lost
Abstract
The grid cell network in the medial entorhinal cortex (MEC) has been subject to thorough testing and analysis, and many theories for their formation have been suggested. To test some of these theories, we re-analyzed data from Bonnevie et al., 2013, in which the hippocampus was inactivated and grid cells were recorded in the rat MEC. We investigated whether the firing associations of grid cells depend on hippocampal inputs. Specifically, we examined temporal and spatial correlations in the firing times of simultaneously recorded grid cells before and during hippocampal inactivation. Our analysis revealed evidence of network coherence in grid cells even in the absence of hippocampal input to the MEC, both in regular grid cells and in those that became head-direction cells after hippocampal inactivation. This favors models, which suggest that phase relations between grid cells in the MEC are dependent on intrinsic connectivity within the MEC.
https://doi.org/10.7554/eLife.47147.001Introduction
The mechanism responsible for the emergence of grid cell periodicity is arguably one of the best-tested and best-established mechanisms, across neural circuits in the mammalian central nervous system, thanks to extensive testing and analysis (Hafting et al., 2005; Moser et al., 2008; Derdikman and Knierim, 2014; Rowland et al., 2016). Modeling work has suggested that either grid cells are generated intrinsically in the medial entorhinal cortex (MEC), for example by a continuous attractor network model (Burak and Fiete, 2009; Couey et al., 2013; Fuhs, 2006; Giocomo et al., 2011; Moser et al., 2014; Zilli, 2012) or alternatively have their properties form through an interaction with another region, such as the hippocampus (Dordek et al., 2016; Kropff and Treves, 2008; Stachenfeld et al., 2017). To dissociate between these possibilities, we re-analyzed data from Bonnevie et al. (2013), who inactivated hippocampal input to the MEC, and found that under this condition, the grid pattern of individual grid cells deteriorated significantly or disappeared entirely. Here we investigated whether the firing associations of grid cells depend on hippocampal inputs. Specifically, we examined correlations in the firing times of simultaneously recorded grid cells before and during hippocampal inactivation, including grid cells that acquired head directional tuning during inactivation. Our analysis yielded evidence of network coherence in grid cells even in the absence of hippocampal input to the MEC.
Results
We reanalyzed data from Bonnevie et al. (2013) in which grid cells were recorded before, during and after hippocampal inactivation (Figure 1A–C). A total of 301 well-separated cells were recorded in the MEC and parasubiculum across 40 sessions including pre-, during and post-hippocampal inactivation, with 2–18 cells recorded simultaneously per session. While the runs of pre- and post-inactivation were analyzed in their entirety, for runs during inactivation we used only the time period starting at 15 minutes or later, up to 45 minutes, during which the most data were available across all recordings. The analysis showed similar effects on grid behavior in longer trials as in the first 45 minutes; the average grid score was −0.063 ± 0.193 for the first 15–45 minutes and −0.051 ± 0.180 for the remaining minutes.
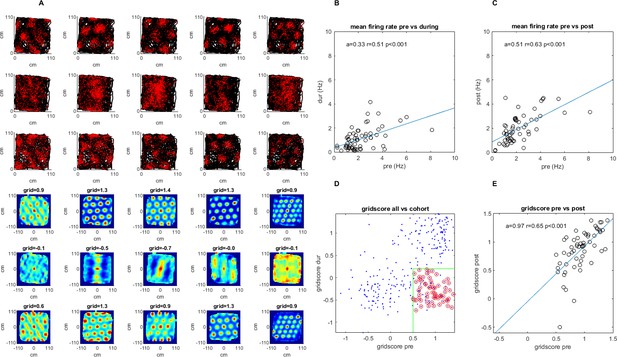
A survey of the grid cell population included in the study.
Recordings were made pre-, during, and post-injection of muscimol to the hippocampus. (A) A sample group of 5 simultaneously recorded grid cells, one cell per column. The first three plots in each column show the location of a single cell firing (red) along the rat’s trajectory (black) in a square arena pre-, during, and post-hippocampal inactivation, respectively. The last three plots in each column show the autocorrelation of the firing rate map and the grid score of that session pre-, during, and post inactivation. (B) The mean firing rate for the 63 grid cells included in the study pre- vs. during inactivation. (C) Same as (B) but for pre- vs. post-inactivation. (D) The grid score of all cells in the dataset vs. those included in the study. Red circles show the cells from the total population that were ultimately included in the study meeting the minimum and maximum grid score threshold pre- and during inactivation, respectively (green), as well as the additional criteria specified in the Materials and methods section (note that cells whose grid scores could not be calculated were set to 0). (E) Grid score pre- and post-inactivation of the cells included in the study.
We searched for evidence of network activity between grid cells in the absence of hippocampal input. Consequently, we examined spike time correlations and spatial firing correlations between simultaneously recorded cells whose gridness was high under normal conditions, but deteriorated during hippocampal inactivation (Figure 1D and E). To select only cells with significant gridness before inactivation and minimal gridness during inactivation, we used a minimum grid score threshold of 0.5 pre-inactivation, and a maximum of 0.2 during inactivation. Doing the same analysis with different thresholds, ranging from 0.2 to 0.9 pre-inactivation, and 0 to 0.4 during inactivation, did not change the central finding of the analysis, showing persistence of temporal correlations between cell pairs during hippocampal inactivation (Figure 3—figure supplement 5).
The mean grid score of the selected cells was 0.92 ± 0.24 pre-inactivation and −0.30 ± 0.24 during inactivation. Additionally, to ensure that the same cell was not recorded on different electrodes, we removed any cells from a single recording session whose individual spike times overlapped within a 1 millisecond window more than 5% of the time (this mostly removed cases with very large overlap that were suspected as originating from the same cell on different tetrodes). The mean spike overlap after exclusion was 0.57% ± 0.65 of the total spike strain. In total, 63 of 301 cells from 17 of 41 recording sessions met our criteria (Figure 1D), producing 107 pairs of simultaneously recorded cells, on which the results of this study are based. In our cohort, firing rates decreased by 50.0% during inactivation, and returned to 90.5% of original levels post-inactivation (pre 2.21 Hz ± 1.39, during 1.11 Hz ± 0.90, and post 2.00 Hz ±1.16). However, overall, neither the firing rate, nor the grid score seemed to correlate to temporal or spatial correlations both pre and during inactivation (Figure 4—figure supplement 1).
Temporal correlations are maintained during loss of gridness
We found that several simultaneously recorded grid cell pairs consistently maintained temporal correlations even as their gridness score deteriorated (Figure 2A and B). Compared to random shuffling (n = 1000, α = 0.01), these correlations were statistically significant; 57%, 26%, and 53% of correlations passed the shuffling significance test pre-, during, and post-inactivation, respectively. Of the statistically significant correlations, 41%, 8%, and 27% were negative for the three respective recording phases, while 16%, 19%, and 22% were positive. Temporal correlations pre- vs. during inactivation were correlated at r = 0.58 (Figure 3A, p<0.001; although also in this case the distributions were different, Wilcoxon signed-rank p<0.001), and pre- vs. post-inactivation were correlated at r = 0.86 (Figure 3A, p<0.001). The results were very similar when analyzing the period of the recordings after 45 minutes (Figure 3—figure supplement 1). The strength of the correlations, both before and during inactivation, demonstrated a slight non-significant positive dependence on the grid score (Figure 3—figure supplement 2). For comparison, the correlation coefficient of temporal correlations pre- vs. during inactivation for each cell from our cohort against each cell not from the same recording session (1854 pairs in total) was r = −0.03 (p=0.21).
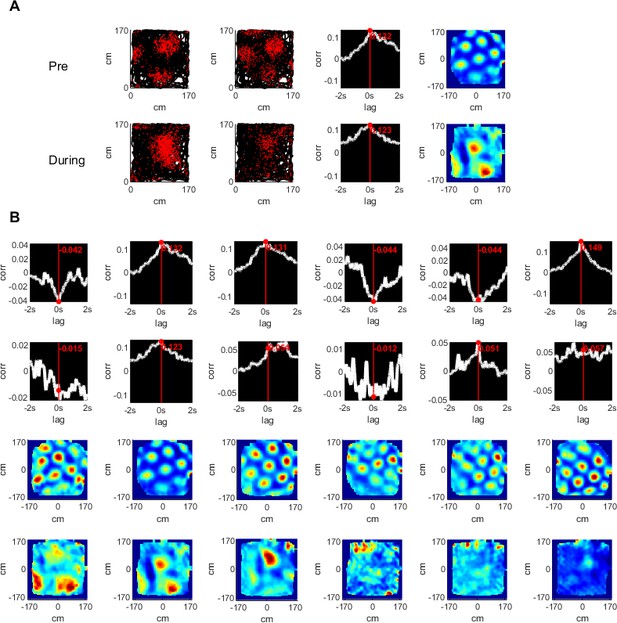
Temporal and spatial cross correlations of simultaneously recorded grid cells pre- and during hippocampal inactivation.
(A) An example of a pair of simultaneously recorded grid cells (columns 1, 2); the location of the cell firing (red) plotted over the rat’s trajectory (black) in a square arena. Columns 3 and 4 show the temporal and spatial cross correlation of the firing rate maps of the cells, respectively. Rows show the same analysis pre- and during inactivation. (B) The temporal and spatial cross correlations of cell pairs of an entire group of simultaneously recorded grid cells (one pair per column). Rows 1, 2 show temporal correlations pre- and during inactivation; rows 3, 4 show the same for spatial cross correlations.
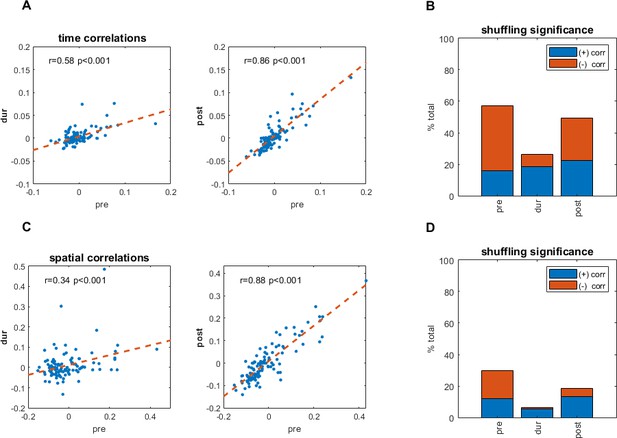
Temporal and spatial correlations pre- and during inactivation, for simultaneously recorded cell pairs.
(A) Temporal cross correlations pre- and during inactivation, and pre- and post-inactivation, with correlation value (r), and corresponding p-value. (B) Proportions of significant temporal correlations, according to shuffling measures, pre-, during, and post-inactivation, including the sign (positive, negative) of the correlation value. (C) Same as (A) but for spatial correlations of the firing pattern in the arena at [0,0]. (D) Same as (B) but for spatial correlations of the firing pattern in the arena at [0,0].
Spatial correlations are maintained during loss of gridness
To examine whether short-range spatial correlations were maintained also during hippocampal inactivation, we compared the correlation coefficient of the 2D firing rate maps at the same position (x,y = 0,0). Overall, spatial correlations did not persist as consistently as temporal correlations during hippocampal inactivation; however, some degree of persistence was present between simultaneously recorded cell pairs. Spatial correlations were correlated to each other pre-inactivation vs. during inactivation at r = 0.34 (Figure 3C, p<0.001), while pre- vs. post-spatial correlations were correlated at r = 0.88 (Figure 3C, p<0.001). For a control comparison, the correlation coefficient of spatial correlations pre- vs. during inactivation for each cell from our cohort correlated against each other cell not from the same recording session, was lower (r = 0.10, p<0.001). The shuffling significance test (n = 1000, α = 0.01) found that 30%, 7%, and 22% of spatial correlations were significant pre-, during, and post-inactivation, respectively. Of the correlations, 18%, 1%, and 6% were negatively significant for all three recording phases, respectively, while 12%, 6%, and 13% were positive (Figure 3B,D). We note though that the amount of significant cell pairs was significantly lower during inactivation (χ2 = 12.2, p<0.001). The angular direction of the nearest peak in the spatial correlations was not maintained (Figure 3—figure supplement 6: while pre-inactivation vs. post-inactivation difference in angle was very non-uniform (Rayleigh non-uniformity test, p<0.001), the difference in angle between pre-inactivation and during inactivation did not diverge from uniformity (Rayleigh non-uniformity test, p=0.974), however when performing a time-windowed analysis of spatial correlations (see Materials and methods), at least in the 1 s range there were comparable correlations between spatial patterns before and during inactivation to those after inactivation (Figure 3—figure supplement 4). Although the statistical significance was lower overall for spatial correlations than for temporal correlations, the results for spatial correlations were consistent with those for temporal correlations. Additionally, plotting temporal and spatial correlations against each other demonstrated a clear linear relationship; the correlations of temporal to spatial correlations were r = 0.90 (p<0.001), r = 0.72 (p<0.001), and r = 0.90 (p<0.001) for pre-, during, and post-inactivation, respectively (Figure 3—figure supplement 3).
Grid-turned head direction cells maintain temporal but not spatial correlations during inactivation
In the original Bonnevie et al. (2013) study, it was reported that a subset of the grid cells became head direction cells during hippocampal inactivation. Cells within our cohort showed a bi-modal distribution of Rayleigh head-direction tuning scores during inactivation (Figure 4C). This enabled selecting the cells that became head-direction cells, defined as having a Rayleigh score smaller than 0.4 pre-, and greater than 0.4 during inactivation (15 of the 63 cells in our cohort, from 3 of the 17 recording sessions in two rats; 37 pairs out of the 107 pairs) and those which did not, defined as staying below 0.4 both pre- and during inactivation (39 of the 63 cells in our cohort, from 13 of the 17 recording sessions in six rats; 46 pairs out of the 107 pairs) (Figure 4C,D and E). Figure 4A depicts an example of these grid-turned head direction cells from the same recording session. Examining these two clusters separately, looking at temporal correlations pre- and during inactivation, non-head direction pairs were more correlated at r = 0.71, than the head direction pairs at r = 0.52, though both were correlations were significant (p<0.001; Figure 4D). For spatial correlations, pre-inactivation compared to during inactivation, had a correlation coefficient of r = 0.46 (p<0.001) in the non-head directional cluster, and r = 0.22 in the head direction cluster, though this latter correlation was not significant with p=0.19 (Figure 4D). In conclusion, while spatial correlations were probably less persistent in grid-turned head direction cells, temporal correlations persisted similarly in both regular and grid-turned head direction cells, during hippocampal inactivation. There did seem to be a bias in the tuning angle for the grid turned head direction cells whose average was 37.0° ± 3.5 during inactivation, which was also seen in the rest of the cells in the population, whose Rayleigh score was also above the same threshold of 0.4 pre- and during inactivation. Their averages were 26.5° ± 6.1, and 38.7° ± 4.5 respectively (Figure 4E and F). This bias is consistent with the fact that all the grid-turned head direction cells were recorded in the same room (room 3), as were the majority of the rest of the cells (Figure 4—figure supplement 2).
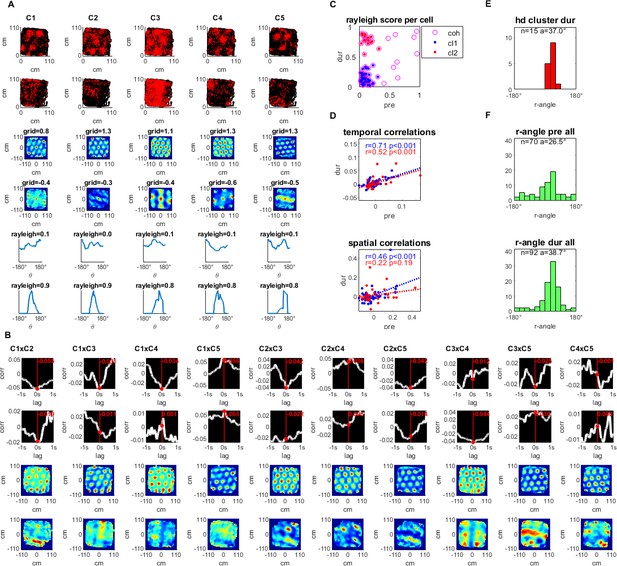
Simultaneously recorded grid cells that became head directional during hippocampal inactivation.
(A) A sample group of 5 simultaneously recorded grid cells, one cell per column. The first two plots in each column show the location of a single cell firing (red) along the rat’s trajectory (black) in a square arena pre- and during hippocampal inactivation. Next, two plots show the autocorrelation of the firing rate map and the associated grid score pre- and during inactivation. The last two plots in the column show the firing rate by head direction with an associated Rayleigh score, pre- and during inactivation. (B) Temporal and spatial cross correlations for each cell pair of the group, pre- and during inactivation by column. (C) Rayleigh scores pre- and during inactivation for all cells in the cohort (magenta circles) clustered by low head directionality (Rayleigh score <0.4 pre- and during inactivation, blue) and high head directionality (Rayleigh score <0.4 pre- and >0.4 during inactivation, red) (D) Temporal and spatial correlations (at 0,0) pre- and during inactivation grouped by head directionality clusters defined in (C), with the trendline slope (a) correlation coefficient (r), and corresponding p-value (p). (E) Histogram of Rayleigh angles for the cells in the HD cluster (red cluster in panels C and D) during inactivation (angles pre-inactivation are not shown since these cells had low Rayleigh scores for that period). (F) Rayleigh angles for all cells in population with Rayleigh score >0.4 pre-, and during inactivation.
Discussion
This study reanalyzed data from Bonnevie et al. (2013), in which hippocampal input to the MEC was inactivated. The aim was to examine possible evidence of local grid cell coordination. We found that despite the disappearance of the grid pattern of these cells during hippocampal inactivation, temporal correlations between grid cells remained, at least partially, as did local spatial correlations, although to a lesser, yet still statistically significant degree. A time-windowed version of the spatial correlations suggests a window of about 1 s in which spatial structure remained.
First, these findings assert that hippocampal input does not completely account for spatially and temporally correlated activity between grid cells. Second, the slow decay of the correlations at a timescale in the order of magnitude of 1 s indicates that this effect is not due solely to direct synaptic connectivity, but to recurrent network activity of dense networks in behavioral timescales. Last, some grid cells became head-direction cells during hippocampal inactivation, and their spatial correlations were less dominant; nonetheless, temporal correlations persisted. Taken together, this paper is adding another piece of evidence to a line of results that support continuous attractor dynamics (Burak and Fiete, 2009; Couey et al., 2013; Fuhs, 2006; Giocomo et al., 2011; Moser et al., 2014; Zilli, 2012; Yoon et al., 2013; Heys et al., 2014; Gu et al., 2018; Trettel et al., 2019; Gardner et al., 2019) while being less supportive of feedforward models creating grid cells through summation of information from the hippocampus (Dordek et al., 2016; Kropff and Treves, 2008; Stachenfeld et al., 2017). We cannot preclude, though, the possibility that grid cells are formed through a different feedforward process, not involving the hippocampus, or that they are generated through a recurrent loop involving information from both the hippocampus and the entorhinal cortex (Donato et al., 2017).
A network model for grid cell firing pattern at least strongly predicts, if not implicitly requires, a significant level of synchronicity in temporal firing between two connected grid cells (Moser et al., 2014). The attractor manifold model for grid pattern generation predicts that even when the network is deprived of spatial input, in this case from the hippocampus, the activity pattern that is maintained (though no longer anchored to physical space) would cause nearby cells in the manifold to fire with high correlation, and more distant cells not to fire (Burak and Fiete, 2009; Dunn et al., 2014; Fuhs, 2006; Tocker et al., 2015). This aligns with our observations of both correlated and anticorrelated activity during inactivation.
In addition to the evidence of network coordination, we found that the distinct subset of cells that became head direction-tuned during inactivation, maintained temporal but not spatial correlations during hippocampal inactivation. Interestingly, the head-direction selectivity of these cells was very biased (Figure 4E). The large overlap in Rayleigh angle between these cells enabled the temporal correlations to remain significant despite the transformation to head-direction cells. This subset of grid-turned head direction cells is likely defined by strong input from the retrosplenial cortex, or the pre- or para- subiculum, which also project into the MEC, and which dominate these cells’ spatial tuning and firing time synchronization in the absence of input from the hippocampus (Clark and Taube, 2012). Because the grid-turned head-direction cells originated from recordings that did not contain grid cells that did not turn into head-direction cells we did not observe direct evidence of a temporal correlation between grid cells that became head-direction cells and those that did not. Notably, both groups were similar in their temporal correlation values before and during inactivation, regardless of their differences in spatial correlations. This suggests that despite receiving additional spatial input, the grid cells that became head-direction cells were part of the grid-cell network.
Several other experiments have investigated grid cell activity when spatial input was curtailed, specifically following removal of visual input. In their original paper that described grid cells, Hafting et al. (2005) observed rat grid cells in darkness, and found that the grid pattern did not deteriorate. More recently, two studies, by Pérez-Escobar et al. (2016) and Chen et al. (2016) that examined mouse grid cells in darkness reported that the grid pattern deteriorated without visual input. Furthermore, both studies found that significant temporal correlations were maintained during impaired spatial input to the entorhinal cortex, in accordance with our findings. Similarly, two new studies have demonstrated that grid cell temporal correlations remain during sleep (Trettel et al., 2019; Gardner et al., 2019).
The steady synchronicity in our study suggests an underlying network structure in the MEC that is responsible for grid cell formation. This corroborates the idea of an attractor manifold involved in grid cell formation.
Materials and methods
The following sections describe the acquisition of the original data from Bonnevie et al. (2013), and the analytical methods we applied to the data. All code was written in MATLAB (V. 2018b). The code was uploaded to GitHub at https://github.com/derdikman/Almog-et-al.-Matlab-code (Derdikman, 2019; copy archived at https://github.com/elifesciences-publications/Almog-et-al.-Matlab-code). The data files, in Matlab format, are available through Dryad at https://doi.org/10.5061/dryad.bk3j9kd6d.
Input data
Request a detailed protocolBriefly, the original experiment by Bonnevie et al. (2013) was performed with eight male, 3–5 month old Long-Evans rats, with water available ad libitum. The rats were kept on a 12 hour light, 12 hour dark schedule and tested in the dark phase. Rats were implanted with a microdrive connected to four tetrodes of twisted 17 μm platinum-iridium wire; one bundle was implanted in the MEC in all rats, anteroposterior 0.4–0.5 mm in front of the transverse sinus, mediolateral 4.5–4.6 mm from the midline, and dorsoventral 1.4–1.8 mm below the dura. Tetrodes were angled 10° in the sagittal plane. For hippocampal inactivation, cannulae were implanted at a 30° angle in the posterior direction towards the dorsal hippocampus; 0.24–0.30 μl of the GABAA receptor agonist muscimol (5-aminomethyl-3-hydroxyisoxazole) diluted in PBS was used to inactivate the hippocampus.
Rats were run in an open-field 100 cm, 150 cm, or 170 cm square arena, size depending on which of the three recording rooms were used (rooms 3, 9 and 10), polarized by a single white que card in an otherwise black environment for a 20 minute period, after which muscimol was infused. Subsequently, the firing rate of all principal cells recorded in the dorsal CA1 region decreased rapidly,~2.2 mm posterior and lateral to the infusion site (82 cells, all of which were place cells), with firing rates dropping to 1% of the baseline rate in 79% of the recorded cells within 20 minutes. Inactivation of the hippocampus had only minimal impact on the behavior of the rats. Rats then ran for an average of 160 minutes in the open field. After 6–24 hours, the rats were run for 20 minutes to check for cell recovery and grid stability.
Quantifying gridness and head direction selectivity
Request a detailed protocolFor this analysis, we were interested in specifically examining grid cells whose spatial firing pattern was significantly degraded. To quantify this, we used the generally accepted measure of grid score, which essentially measures the extent the cell’s firing pattern repeats itself at 60o intervals on a two-dimensional (2D) plane (how hexagonal the firing pattern is). The procedure undertaken to achieve this calculation is as follows (based on the procedure described in Sargolini et al., 2006; Tocker et al., 2015):
The arenas were divided into 50x50 equally sized square bins. First, a 2D map of neuron spiking was generated by creating a matrix where the index [i, j] represents the location in the arena, and the value represents the number of spikes in that location. The equivalent matrix for time spent at each location was also created. These two matrices were divided by each other element-wise, creating a matrix of firing rates at each location bin.
Next, a 2D normalized spatial autocorrelation was performed on the rate map matrix accounting for non-existent values in the rate map, as described in Tocker et al. (2015). Firing fields were identified using a method that treated the smoothed (2D Gaussian smoothing with σ = 2) autocorrelation matrix as an image, and identified distinct regions bounded by a given pixel value of x in all eight directions whose external values are all less than x. Typical grid cell autocorrelations have at least six firing fields, at approximately 60° intervals (Hafting et al., 2005). Typical grid cell activity was manifested as equidistant firing fields at 60° intervals from each other.
The final step in calculating the grid score was to create a ring around the center of the smoothed autocorrelation (2D Gaussian smoothing with σ = 2), with an inner radius small enough to contain the innermost firing field, and the outer radius large enough to contain the outermost edge of the sixth closest field. Next, the ring was rotated 60° and correlated to the original (using a normalized correlation, accounting for empty matrix values as described above). This value was then subtracted by the value of the ring correlated at a 30° rotation. Since both correlations have values in the range of [−1,1], the range of grid scores is [−2, 2]. Cells whose autocorrelation did not create six distinct firing fields for calculating the annulus using the above method were set to a default grid score of 0.
A Rayleigh score from 0 to 1 was used to quantify head directionality of cells, similar to that described in Tocker et al. (2015).
Cell pair correlations
Request a detailed protocolTo quantify temporal correlations between cells, we calculated the Pearson correlation of their spike trains (lag = 0 ms). For spatial correlations, a 2D Pearson correlation of the rate maps (see Quantifying gridness) was performed and compared at [0,0]. Spatial cross correlations were done following the Pearson moment formula accounting for missing values in the rate map as described in Tocker et al. (2015). Smoothing was done on the spike trains prior to both temporal and spatial correlations using a moving average window of 25 ms. Varying the smoothing windows from 1 ms to 1000 ms had little or no impact on the correlation results.
Shuffling to measure significance
Request a detailed protocolTo measure the statistical significance of the correlations, we employed a shuffling method in which spike train times were shifted cyclically n times by total_spike_train_time/n to create pseudo random spike trains (n = 1000 unless stated otherwise) and their correlations recalculated. Correlations were considered significant if they were in the 99th percentile when compared to the shuffled correlations.
Time-windowed spatial correlation analysis
Request a detailed protocolIn order to measure spatial correlations at a smaller time-scale during inactivation, we analyzed spike to spike locations relative to the first cell in a cell pair within a given time window. For a given cell pair, each spike was treated as the origin in two dimensional space [0,0], and a 2D binned rate map (see above) consisting of all spikes in a given time window [±1s; ±2s; ±3s; ±5s or ±10s], was constructed. This collection of rate maps for a given cell pair was aggregated into a single rate map by adding up the values at a given bin location in the rate map. This procedure was done for each recording session [pre- during post-inactivation]. These cell pair maps were then spatially correlated against each other by session [pre- vs. during inactivation; pre- vs. post-inactivation], where [pre- vs. post-inactivation] represented the control in which we expected to see stable maps for cell pairs between pre -and post- and therefore high correlations.
Data availability
Original data for this study have been taken from Bonnevie et al. (2013). The link to the mat files is now available through Dryad at https://doi.org/10.5061/dryad.bk3j9kd6d. Analyses were performed in Matlab, and code was uploaded to GitHub: https://github.com/derdikman/Almog-et-al.-Matlab-code (copy archived at https://github.com/elifesciences-publications/Almog-et-al.-Matlab-code).
-
Dryad Digital RepositoryData from: During hippocampal inactivation, grid cells maintain synchrony, even when the grid pattern is lost.https://doi.org/10.5061/dryad.bk3j9kd6d
References
-
Grid cells require excitatory drive from the HippocampusNature Neuroscience 16:309–317.https://doi.org/10.1038/nn.3311
-
Accurate path integration in continuous attractor network models of grid cellsPLOS Computational Biology 5:e1000291.https://doi.org/10.1371/journal.pcbi.1000291
-
Recurrent inhibitory circuitry as a mechanism for grid formationNature Neuroscience 16:318–324.https://doi.org/10.1038/nn.3310
-
Correlations and functional connections in a population of grid cellsPLOS Computational Biology 11:e1004052.https://doi.org/10.1371/journal.pcbi.1004052
-
A spin glass model of path integration in rat medial entorhinal cortexJournal of Neuroscience 26:4266–4276.https://doi.org/10.1523/JNEUROSCI.4353-05.2006
-
Correlation structure of grid cells is preserved during sleepNature Neuroscience 22:598–608.https://doi.org/10.1038/s41593-019-0360-0
-
Place cells, grid cells, and the brain's spatial representation systemAnnual Review of Neuroscience 31:69–89.https://doi.org/10.1146/annurev.neuro.31.061307.090723
-
Network mechanisms of grid cellsPhilosophical Transactions of the Royal Society B: Biological Sciences 369:20120511.https://doi.org/10.1098/rstb.2012.0511
-
Ten years of grid cellsAnnual Review of Neuroscience 39:19–40.https://doi.org/10.1146/annurev-neuro-070815-013824
-
The hippocampus as a predictive mapNature Neuroscience 20:1643–1653.https://doi.org/10.1038/nn.4650
-
Specific evidence of low-dimensional continuous attractor dynamics in grid cellsNature Neuroscience 16:1077–1084.https://doi.org/10.1038/nn.3450
-
Models of grid cell spatial firing published 2005-2011Frontiers in Neural Circuits 6:16.https://doi.org/10.3389/fncir.2012.00016
Article and author information
Author details
Funding
Israel Science Foundation (955/13,2344/16,2655/18)
- Dori Derdikman
Rappaport Institute
- Dori Derdikman
Allen and Jewel Prince Center for Neurodegenerative Disorders of the Brain
- Dori Derdikman
Adelis Foundation (Technion-Weizmann collaboration grant)
- Dori Derdikman
The funders had no role in study design, data collection and interpretation, or the decision to submit the work for publication.
Acknowledgements
We thank Cindy Cohen for proofreading. We thank Chen Elbak and Irina Reiter for help with experiment administration. We thank members of the Derdikman lab for fruitful discussions.
Version history
- Received: March 26, 2019
- Accepted: October 3, 2019
- Version of Record published: October 17, 2019 (version 1)
Copyright
© 2019, Almog et al.
This article is distributed under the terms of the Creative Commons Attribution License, which permits unrestricted use and redistribution provided that the original author and source are credited.
Metrics
-
- 2,068
- views
-
- 279
- downloads
-
- 12
- citations
Views, downloads and citations are aggregated across all versions of this paper published by eLife.
Download links
Downloads (link to download the article as PDF)
Open citations (links to open the citations from this article in various online reference manager services)
Cite this article (links to download the citations from this article in formats compatible with various reference manager tools)
Further reading
-
- Cell Biology
- Neuroscience
One of the most extensively studied members of the Ras superfamily of small GTPases, Rac1 is an intracellular signal transducer that remodels actin and phosphorylation signaling networks. Previous studies have shown that Rac1-mediated signaling is associated with hippocampal-dependent working memory and longer-term forms of learning and memory and that Rac1 can modulate forms of both pre- and postsynaptic plasticity. How these different cognitive functions and forms of plasticity mediated by Rac1 are linked, however, is unclear. Here, we show that spatial working memory in mice is selectively impaired following the expression of a genetically encoded Rac1 inhibitor at presynaptic terminals, while longer-term cognitive processes are affected by Rac1 inhibition at postsynaptic sites. To investigate the regulatory mechanisms of this presynaptic process, we leveraged new advances in mass spectrometry to identify the proteomic and post-translational landscape of presynaptic Rac1 signaling. We identified serine/threonine kinases and phosphorylated cytoskeletal signaling and synaptic vesicle proteins enriched with active Rac1. The phosphorylated sites in these proteins are at positions likely to have regulatory effects on synaptic vesicles. Consistent with this, we also report changes in the distribution and morphology of synaptic vesicles and in postsynaptic ultrastructure following presynaptic Rac1 inhibition. Overall, this study reveals a previously unrecognized presynaptic role of Rac1 signaling in cognitive processes and provides insights into its potential regulatory mechanisms.
-
- Neuroscience
Daily experiences often involve the processing of multiple sequences, yet storing them challenges the limited capacity of working memory (WM). To achieve efficient memory storage, relational structures shared by sequences would be leveraged to reorganize and compress information. Here, participants memorized a sequence of items with different colors and spatial locations and later reproduced the full color and location sequences one after another. Crucially, we manipulated the consistency between location and color sequence trajectories. First, sequences with consistent trajectories demonstrate improved memory performance and a trajectory correlation between reproduced color and location sequences. Second, sequences with consistent trajectories show neural reactivation of common trajectories, and display spontaneous replay of color sequences when recalling locations. Finally, neural reactivation correlates with WM behavior. Our findings suggest that a shared common structure is leveraged for the storage of multiple sequences through compressed encoding and neural replay, together facilitating efficient information organization in WM.