A curative combination cancer therapy achieves high fractional cell killing through low cross-resistance and drug additivity
Figures
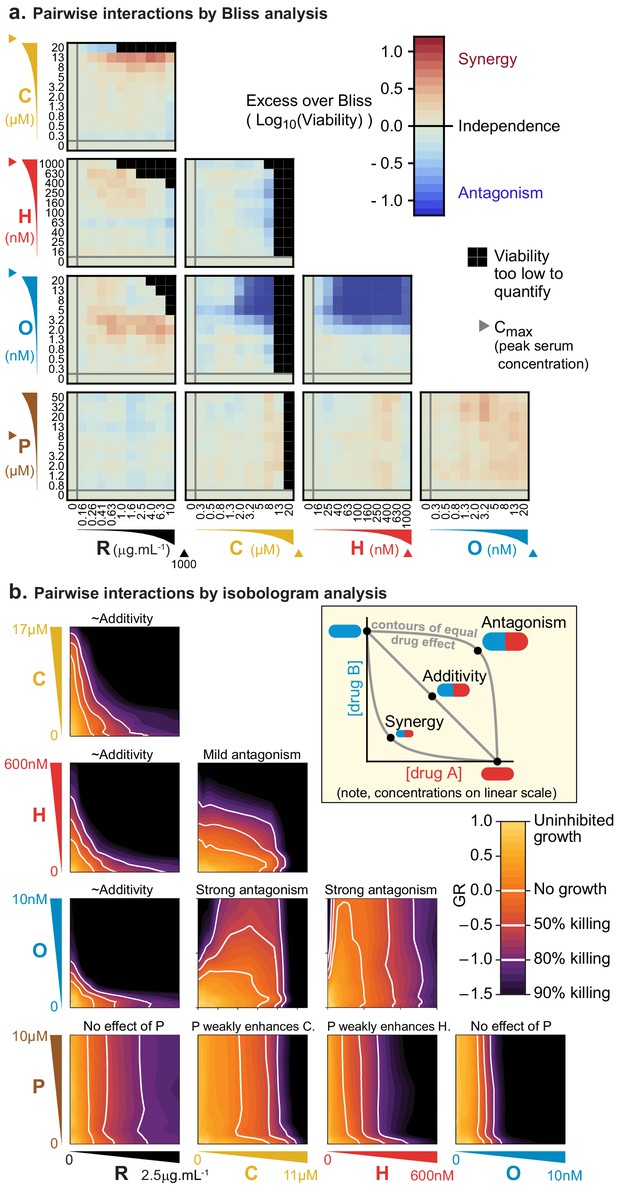
Pairs of drugs in R-CHOP exhibit little synergy, but some strong antagonism, in a Diffuse Large B-Cell Lymphoma cell line.
(a) Pfeiffer cells grown in microtiter plates were treated with drug combinations for 72 hr followed by a luminescence-based assay for cell viability. ‘Excess over Bliss’ measures the observed deviation from Bliss Independence. Triangles on axes indicate each drug’s approximate Cmax, or peak serum concentration in patients. (b) Isobologram analysis of the same experiments; luminescence relative to untreated control cells was converted into a GR metric (Hafner et al., 2016) to distinguish cytostatic from cytotoxic effects. White contours highlight thresholds equivalent to complete growth inhibition (GR = 0), and complete growth inhibition plus 50% or 80% cell killing (GR <0). Inset: principles of isobologram analysis; isoboles are contours of equal drug effect, which are straight lines in the case of ‘additivity’.
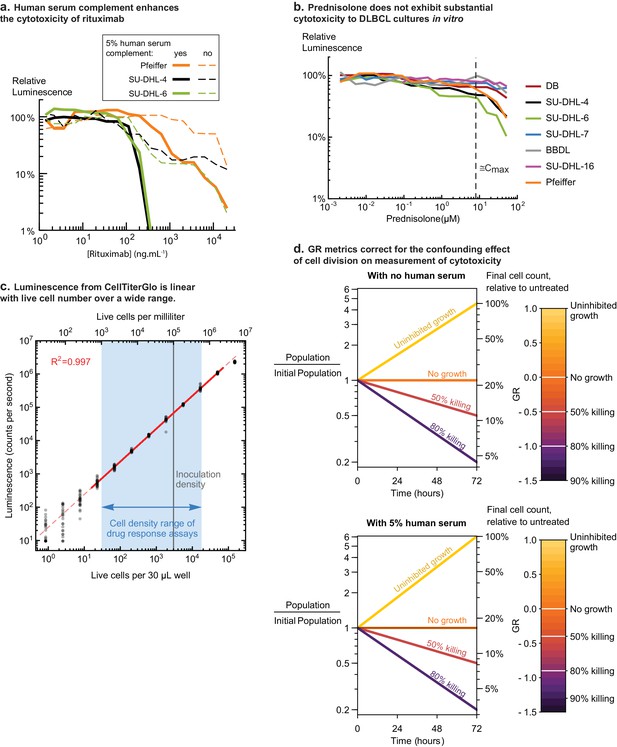
Measuring cytotoxic responses to R-CHOP drugs in DLBCL cultures.
(a) Human serum complement enhances the cytotoxicity of rituximab to DLBCL cell lines. The DLBCL cell lines Pfeiffer, SU-DHL-4, SU-DHL-6 were each seeded in 384-well plates at 105 cells/mL in complete media with or without 5% pooled human complement serum, and were treated with a range of rituximab concentrations. After 72 hr treatment, cell viability was measured by luminescent assay of ATP (CellTiter-Glo). Untreated control wells defined 100% relative luminescence. (b) Prednisolone does not exhibit substantial cytotoxicity to DLBCL cultures in vitro. DLBCL cultures were seeded in 384-well plates, treated with prednisolone for 72 hr, and relative viability was assayed by CellTiter-Glo. None of 7 cell lines demonstrated cytotoxicity at clinically relevant doses (dashed vertical line marks the estimated Cmax of 8 μM). Because untreated cultures divide ~2 to 3 times in 72 hr, reduction of luminescence to ~50% of untreated control indicates a partial reduction in proliferation rate and not net cytotoxicity. Note: SU-DHL-7 has been identified as a descendent of SU-DHL-8, which is also a DLBCL culture. (c) Luminescence from CellTiter-Glo is linear with live cell number over a wide range. Pfeiffer cells were concentrated by centrifugation and resuspended in a reduced volume of media, and the density of live cells was counted using a haemocytometer and trypan blue staining. This culture was seeded in 384-well plates with a volume of 30 μL per well of complete media and cell densities ranging from 5 × 106 down to 50 cells per mL, prepared via serial dilution. 24 replicate wells were prepared at each density. Immediately after inoculation, the plate was subjected to a luminescent assay of ATP by CellTiter-Glo. A solid red line represents the region of highly linear relationship between live cell density and luminescence (R2 = 0.997); at higher densities luminescence begins to saturate, and at lower densities significant noise is observed. A vertical gray line indicates the initial cell density used in drug response assays (Figures 1 and 2), and a blue shaded region indicates the cell density range relevant to these assays. (d) Growth Rate (GR) metrics correct for the confounding effect of cell division on cytotoxicity. Cell division during a drug treatment assay affects the relationship between cell death and cell count relative to untreated controls (Hafner et al., 2016). To correct for this effect, absolute cell counts of untreated cultures were measured before and after treatment to quantify cell proliferation (Materials and methods). Plots indicate the relation between relative cell number (measured by CellTiter-Glo) and fraction of cells killed. The ‘no human serum’ scale is relevant to pairwise interactions in CHOP (Figure 1), and ‘5% human serum’ is relevant to pairs of R and others drugs in CHOP (Figure 1) and all measurements of high-order interactions (Figure 2). Similar relationships were measured and applied for SU-DHL-4 and SU-DHL-6.
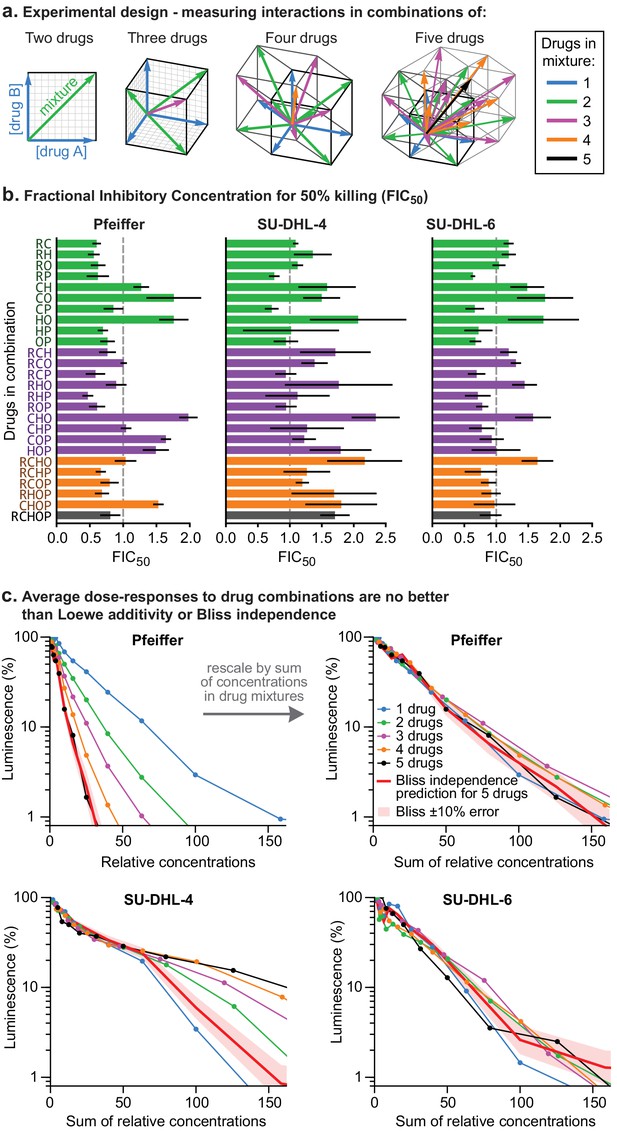
Higher order drug combinations do not exhibit synergistic cell killing.
(a) Experimental design: two or more drugs were mixed in equipotent ratios such that they similarly contributed to cytotoxicity as the dose of the mixture was increased. Dose gradients of drug mixtures span diagonal lines in multi-drug concentration space. (b) Synergy or antagonism of multidrug combinations was quantified by Fractional Inhibitory Concentrations (FIC) at the 50% killing threshold (Figure 1—figure supplement 1D). Error bars are 95% confidence intervals (n = 4 per point along dose response). (c) Average dose response functions to single drugs or mixtures of different numbers of drugs (i.e., average of single-drug responses; average of drug pair responses, etc.). Red line: expected response to R-CHOP drugs according to the Bliss Independence model; pale red area:±10% error in number of log-kills around the Bliss Independence model. Top left: Horizontal axis shows the amount of each drug present in a mixture (units are scaled to align single-agent activity; Figure 2—figure supplement 1A). Top right, bottom left, bottom right: Horizontal axis is the sum of drug concentrations.
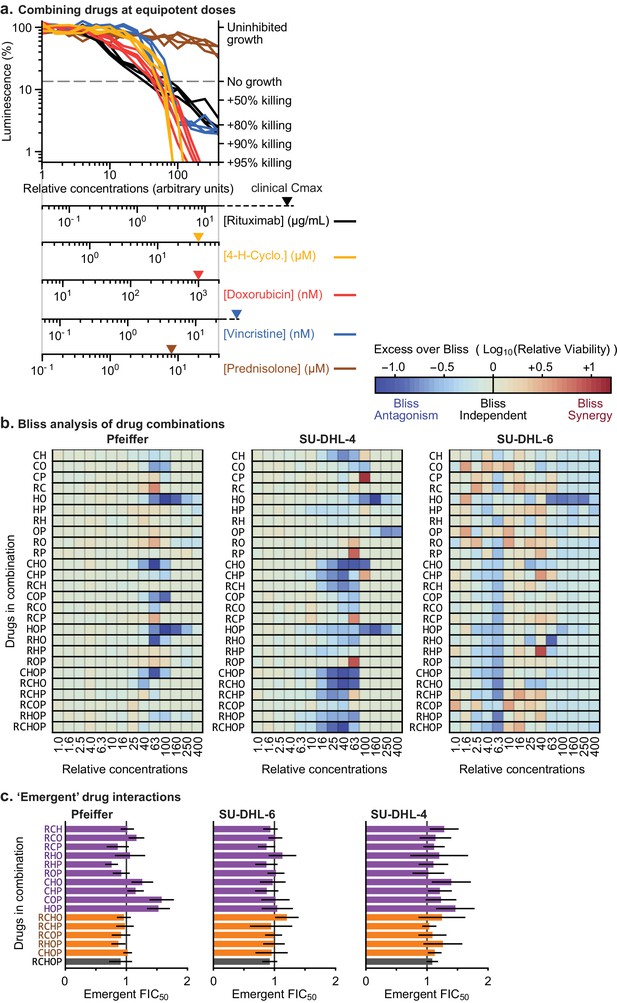
Measuring high-order interactions among R-CHOP drugs with equipotent combinations.
(a) Combining the drugs in R-CHOP at equipotent ratio. In order to measure high-order interactions in R-CHOP (Figure 2 and Figure 2—figure supplement 1B,C), it was necessary to combine agents in equipotent ratio, that is mixing them in proportions that permit each drug to contribute similarly to cytotoxicity (except for prednisolone which does not display single-agent cytotoxicity in vitro). Plotted here are dose responses to each single drug as measured in the experiments of Figure 2 for Pfeiffer (n=8; including two biological duplicate cultures, here plotting four lines per drug that are each the mean of technical duplicates). For each drug, a 400-fold dose range was selected (see axes below the plot) to align all 4 cytotoxic agents (rituximab, 4-hydroperoxy-cyclophosphamide (4-H-Cyclo.), doxorubicin, and vincristine) such that they enter the cytotoxic regime of their dose response (crossing the dashed gray line) at similar positions and that this position aligns with the clinical Cmax (peak serum concentration) of prednisolone. This alignment defines the 'relative concentration' units in Figure 2C. The concentration range also aligns the clinical Cmax (colored triangles on concentration axes) of each drug, except for rituximab which is very highly potent against Pfeiffer cells. Relative luminescence (left axis) was converted to biological effect (right axis) by measuring absolute cell count of untreated cultures before and after drug treatment (Materials and methods): in this experiment untreated cultures increased in cell count by approximately 7-fold, and therefore relative luminescence of ≈14% corresponds to no net growth or death (“no growth” on axis). A net cytotoxic effect is evidenced only by relative luminescence lower than 14% (e.g. +50% killing at ≈7% luminescence, +80% killing at ≈3% luminescence). Similar 400-fold dose windows were independently determined for SU-DHL-4 and SU-DHL-6. (b) High-order interactions of R-CHOP analyzed according to the Bliss Independence model in 3 DLBCL cell lines using data from Figure 2. The Bliss Independence model defines toxins to be ‘non-interacting’ when they confer statistically independent probabilities of cell death, thus, surviving fractions of cells are multiplied (e.g. 90% inhibition by drug A + 90% inhibition by drug B should produce 99% inhibition in combination). The ‘Excess over Bliss’ measures the observed deviation from the Bliss Independence model: in these heat maps blue indicates antagonism (less killing), and red indicates synergy (more killing). See legend above the 'SU-DHL-6' graph. The combination of all 5 drugs in R-CHOP shows neither synergy nor antagonism, being consistent with Bliss independence (bottom row of panels). (c) Emergent high-order interactions of R-CHOP analyzed using fractional inhibitory concentrations at 50% killing threshold (FIC50) in 3 DLBCL cell lines. Emergent FIC quantifies any deviation in the potency of 3, 4, or 5-way combinations from the assumption of additivity between known lower-order interactions (Cokol et al., 2017). FIC is the sum of each drug’s concentration in that mix as a fraction of their single-agent dose producing the same effect: ; and Emergent FIC (e.g. for three drugs ABC: FICABC / average(FICAB, FICAC, FICBC). Error bars are 95% confidence intervals (n=8 per dose point for single-drug dose responses, n=4 per dose point for multi-drug dose responses).
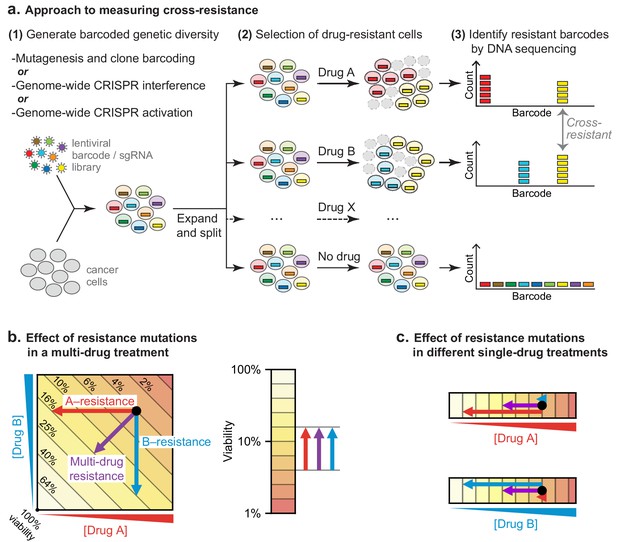
Strategy for measuring cross-resistance between drugs.
(a) Cells were mutagenized and barcoded using one of three approaches: (i) random mutagenesis and clone tracing, (ii) knockdown by CRISPRi or (iii) overexpression by CRISPRa. 106 mutagenized clones or genome-wide CRISPRi/a libraries were expanded and split into replicate cultures, treated with single drugs, and DNA barcodes/sgRNAs abundance was measured by DNA sequencing. The resistance of cells to drug treatment was scored based on the degree of barcode enrichment, and cross-resistance was determined by significant enrichment in two or more drug treatments. (b) Schematic showing importance of selecting for resistance to single drugs not cocktails. Arrows: resistance is analogous to lower drug concentration and moves cells to different coordinates; cross-resistance (purple arrow) has same net effect as more penetrant single-drug resistance mutations (red, blue arrows). (c) By selecting mutations on single drugs the magnitude of the effect on each drug is known.
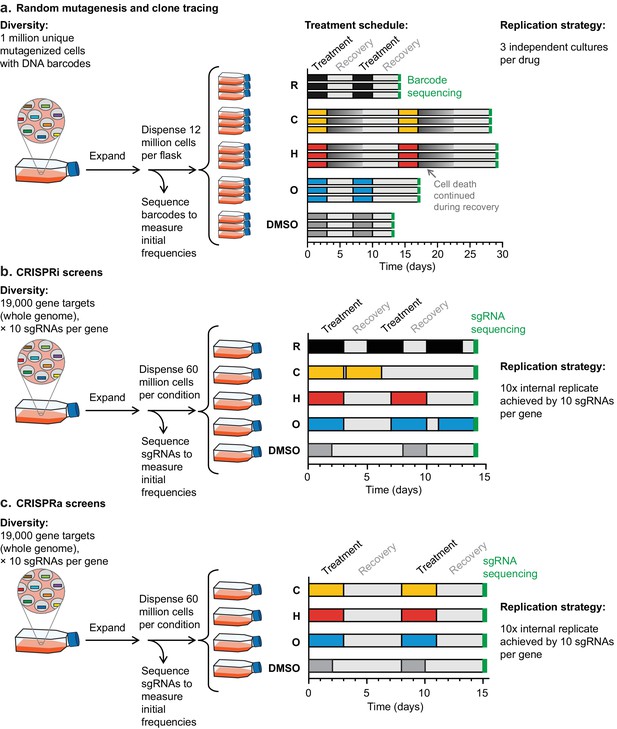
Dosing schedule and replication strategy for all three approaches taken to isolate cells resistant to single cytotoxic drugs in the R-CHOP combination.
(a) Experimental design for measuring drug resistance in DNA-barcoded Pfeiffer clones. 1 million unique mutagenized Pfeiffer cells individually tagged with DNA barcodes were expanded. Replicate flasks of cells (three per drug) were treated with 2 pulses of drug according to the dosing regimen depicted. Recovery was slowest after treatment by C or H, which induced sustained cell death for days after medium was exchanged and drug removed. The abundance of each clone was determined in the pre-treatment population and in each drug-treated replicate sample by high-throughput sequencing. (b) Experimental design for measuring drug resistance in a whole-genome collection of knockdown mutants (CRISPRi). Pfeiffer CRISPRi cells transduced with a genome-wide library of sgRNAs were treated with two-three 72 hr pulses of drug according to the dosing regimen depicted. The treatment schedule was adjusted in order to achieve 7–9 fewer population doublings than the DMSO control. The CRISPRi library contains 10 sgRNAs per gene which serve as internal replicates for each data point. (c) Experimental design for measuring drug resistance in a whole-genome collection of overexpression mutants (CRISPRa). K562 CRISPRa cells transduced with a genome-wide library of sgRNAs were treated with two 72 hr pulses of drug according to the dosing regimen depicted. The treatment schedule was adjusted in order to achieve 9–10 fewer population doublings than the DMSO control. The CRISPRa library contains 10 sgRNAs per gene which serve as internal replicates for each data point.
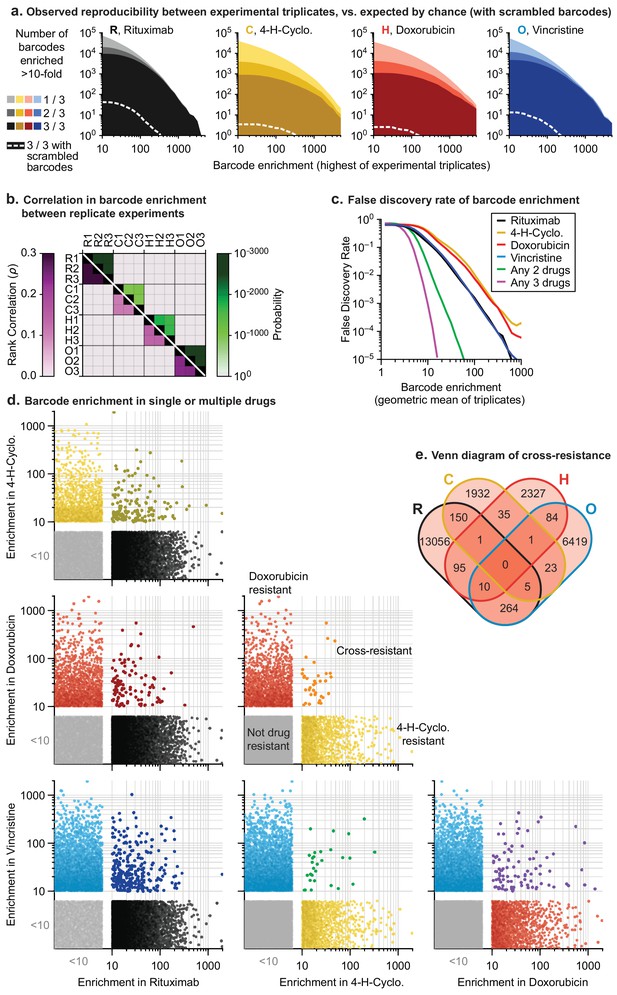
In mutagenized clones single-drug resistance is common but multi-drug resistance is rare.
(a) Reproducibility of DNA barcode enrichment among triplicate drug treatments. Horizontal axis is the highest value for each barcode’s enrichment scores in any replicate. Vertical axis is the number of barcodes for which 1, 2, or three triplicates had enrichment ≥10. Dashed white line: error model indicating repeated enrichment expected by random chance; see Materials and methods. (b) Matrix of Spearman rank correlation in enrichment scores between drug treatments (ρ in pink scale; one-sided p-value in green scale). (c) False discovery rate of barcode enrichment, per magnitude of enrichment (geometric mean of triplicates), was computed by comparing observed barcode enrichment to an error model of scrambled barcodes (1010 triplicates simulated by scrambling actual data). At geometric mean enrichment = 10, false discovery rate for 2-drug and 3-drug resistance is 2.5% and 0.1%, respectively. (d) Scatterplots of barcode enrichment scores (geometric mean of biological triplicates for each drug) for each pair of two drugs in RCHO. Each dot represents a single barcode. Enrichment scores < 10 are deemed not significant. (e) Venn diagram of the number of barcodes exhibiting resistance (geometric mean enrichment ≥10) to single or multiple drugs.
-
Figure 4—source data 1
Barcode counts for all clone tracing experiments.
- https://cdn.elifesciences.org/articles/50036/elife-50036-fig4-data1-v2.txt
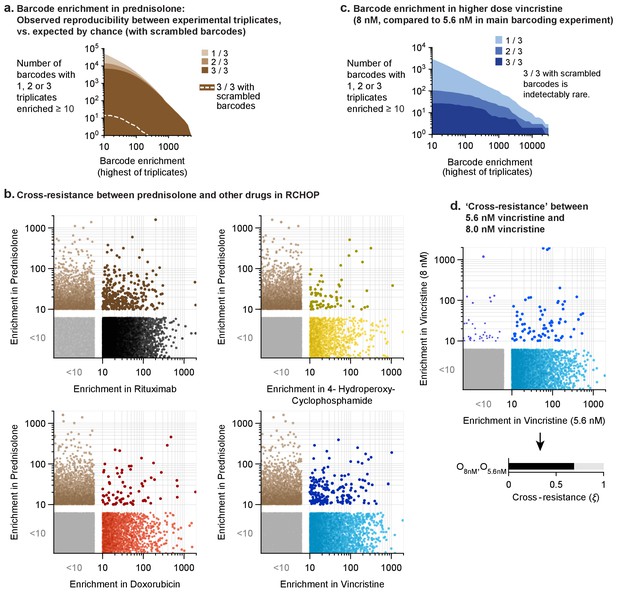
Prednisolone-resistant clones show low cross-resistance to other drugs in R-CHOP, and repeats of vincristine show high cross-resistance.
(a) Enriched barcodes in the cultures treated with prednisolone show a high degree of reproducibility between three replicate drug selections. As prednisolone did not show single-agent cytotoxicity but only partially inhibited growth (Figure 1—figure supplement 1B), barcoded Pfeiffer cultures were exposed to 20 days of continuous treatment with 20 μM prednisolone (Materials and methods). The number of barcodes reproducibly enriched by prednisolone treatment was far in excess (>300 fold) of the number of barcodes expected by random chance (error model based on scrambled barcode identities; Materials and methods). (b) Scatter plots displaying enrichment scores in prednisolone and other drugs in R-CHOP. Enrichment scores of individual barcodes were determined by the geometric mean enrichment of triplicates, and were deemed significant at a score above 10 (based on false discovery rate of double-resistant clones). (c) A set of biological triplicate barcoding experiments were conducted at a higher dose of vincristine (8 nM) than was used in the main barcoding experiments (5.6 nM vincristine). The higher dose imposed far stronger selection: the population required ≈2 weeks to recover following each of two 72 hr treatment cycles, and fewer barcodes exhibited significant enrichment. (d) Analysis of ‘cross-resistance’ between the two doses of vincristine. The majority of barcodes (≈70%) enriched in 8 nM vincristine were also enriched in 5.6 nM vincristine (the opposite cannot be true because fewer barcodes in total were enriched at 8 nM). This corresponds to a cross-resistance parameter of ξ = 0.69, a value that indicates close to maximally overlapping resistance.
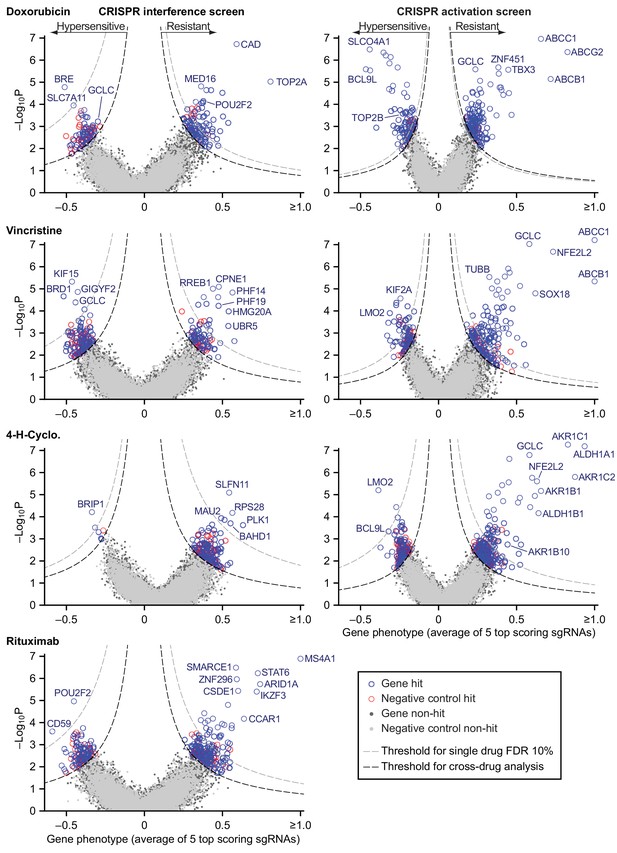
Identification of mechanisms of single drug resistance by genome-wide CRISPRi and CRISPRa screening.
Volcano plots of gene phenotype and p-value for CRISPRi (left) and CRISPRa (right) screens of single R-CHOP drugs. Phenotype of 1 is full resistance, 0 is parental sensitivity,<0 is hypersensitivity. The coordinate of each gene was determined by the average phenotype of the five most active sgRNAs targeting that gene and -log10 of the p-value (Mann-Whitney test of phenotypes for all targeting sgRNAs compared to 4000 non-targeting controls). Negative control genes were generated by randomly grouping sets of non-targeting sgRNAs. Gray dashed line: threshold for 10% FDR for single-drug resistance, or hypersensitivity. Black dashed line: threshold for cross-resistance set to yield less than one double-resistant negative control gene out of all possible drug pairs (equal to multi-drug resistance FDR 4% for CRISPRi and 2% for CRISPRa). Labeled genes are a partial list of top scoring hits.
-
Figure 5—source data 1
sgRNA counts for all CRISPR screens.
- https://cdn.elifesciences.org/articles/50036/elife-50036-fig5-data1-v2.xlsx
-
Figure 5—source data 2
sgRNA phenotype scores for all CRISPR screens.
- https://cdn.elifesciences.org/articles/50036/elife-50036-fig5-data2-v2.xlsx
-
Figure 5—source data 3
Gene scores for all CRISPR screens.
- https://cdn.elifesciences.org/articles/50036/elife-50036-fig5-data3-v2.xlsx
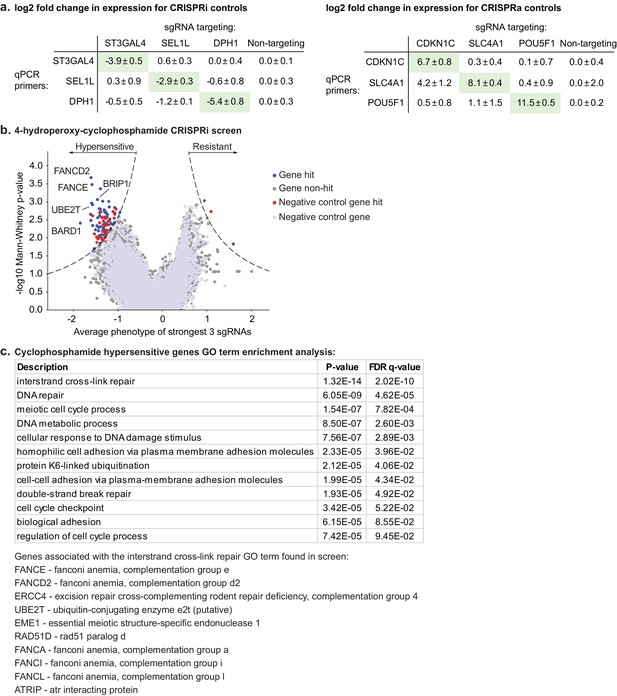
CRISPRi/a cell lines strongly alter gene expression of targeted genes and additional cyclophosphamide CRISPRi screen identifies hypersensitive hits in the DNA interstrand crosslink pathway.
(a) CRISPRi targeting of 3 control genes results in knockdown in Pfeiffer cells (left) and CRISPRa targeting of 3 control genes results in overexpression in K562 cells (left). On-target effect is strong (green shaded boxes) whereas off-target and/or indirect effects are either weak or undetectable. Cell lines expressing either the CRISPRi or CRISPRa system were transduced with sgRNAs targeting control genes. The expression of control genes was measured in those cell lines by RT-qPCR using gene-specific primers and was compared to a non-targeting control sgRNA (Materials and methods). (b) Volcano plot of gene phenotype and enrichment p-value for the CRISPRi screen of 4- hydroperoxy-cyclophosphamide performed at a lower concentration (as compared to Figure 5). The position of each gene was determined by the average phenotype of the three most active sgRNAs (out of a library of 5 sgRNAs per gene) and -log10 of the p-value determined using the Mann-Whitney test of all sgRNAs targeting that gene compared to non-targeting controls. For genes with multiple transcription start sites (TSSs) targeted, sgRNAs were grouped by TSS and the TSS with the lowest p-value was used for display and downstream analysis. Negative control genes were generated by randomly grouping sets of 5 non-targeting controls which were subsequently analyzed as true genes. Dashed lines represent a cutoff ≥7 as calculated from: (gene phenotype z-score)×(-log10(p-value)) and where the z-score is calculated from the standard deviation of the set of negative control genes. (c) Table containing the top hits from a gene ontology analysis performed on the CRISPRi hypersensitive hits. A hypersensitivity score was calculated for each gene by multiplying the two metrics determined in the screen (score = -log10(p-value) × gene phenotype). Gene ontology analysis was performed on the list of genes ranked by hypersensitivity score using GOrilla (Eden et al., 2009). Reported p-values are the enrichment p-values computed according to the GOrilla algorithm and the 'FDR q-values' represent the correction of the p-values for multiple testing hypothesis. A list of all genes belonging to the GO term 'interstrand cross-link repair' and which were found as hypersensitive in the CRISPRi cyclophosphamide screen is reported below the table.
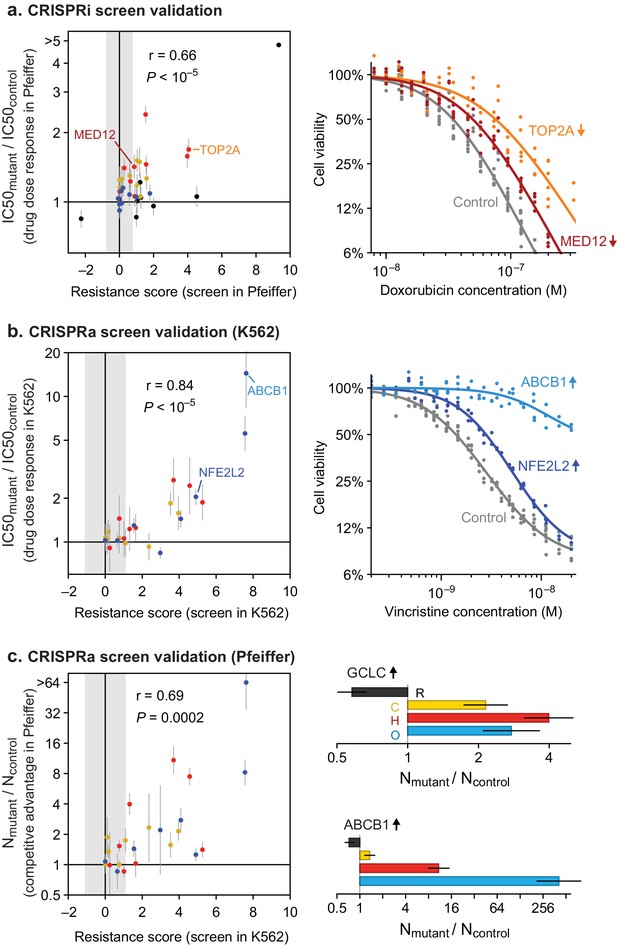
Validation of CRISPRi and CRISPRa screen results by individual drug sensitivity measurements.
(a) Gene knockdown by CRISPRi produces changes in drug sensitivity (IC50) that are correlated with resistance phenotypes from the genome-wide CRISPRi screen (Pearson correlation r = 0.66, p<10–5). Drug dose responses were measured in Pfeiffer CRISPRi cells bearing single sgRNAs, for each of 9 knockdown screen hits, or control non-targeting sgRNA. Error bars are 95% confidence intervals in IC50 (determined from curve fit; n = 6). Point color indicates drug: black, rituximab; yellow, 4-hydroperoxy-cyclophosphamide; red, doxorubicin; blue, vincristine. Gray region: threshold in resistance score that was used to identify screen hits. Right: example dose response measurements for control sgRNAs, or sgRNAs inducing TOP2A and MED12 knockdown and consequent doxorubicin resistance. (b) Gene overexpression by CRISPRa produces changes in drug sensitivity (IC50) that are correlated with resistance phenotypes from the genome-wide CRISPRa screen (r = 0.84, p<10–5). Drug dose responses (n = 4) were measured in K562 CRISPRa cells bearing sgRNAs for each of 8 overexpression screen hits, or control non-targeting sgRNA. Right: example dose response measurements for control sgRNAs, or sgRNAs inducing overexpression of ABCB1 and NFE2L2 and consequent vincristine resistance. (c) Gene overexpression by CRISPRa in DLBCL cells (Pfeiffer) produces drug resistance that is correlated with resistance scores from the CRISPRa screen in K562 cells (r = 0.69, p=0.0002). Pfeiffer CRISPRa cells bearing targeted sgRNAs were mixed at 1:1 ratio with cells bearing non-targeting sgRNA, the co-culture was subjected to two 72 hr drug treatment and recovery periods, and changes in the ratio of mutant to control cells was measured by qPCR of sgRNAs. Error bars are 95% confidence intervals (n = 3). Right: Change in ratio of cells bearing sgRNA that induces GCLC overexpression (or ABCB1 overexpression) versus cells bearing control sgRNA, after drug treatment.
-
Figure 6—source data 1
Data obtained in the CRISPR screen validation experiments.
- https://cdn.elifesciences.org/articles/50036/elife-50036-fig6-data1-v2.xlsx
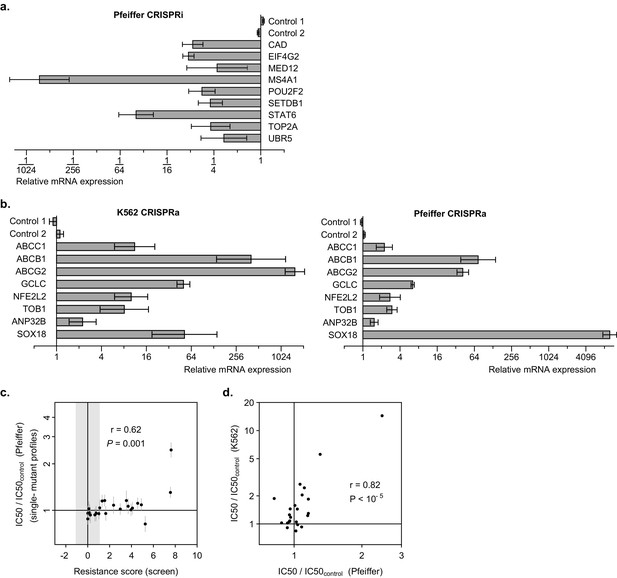
Changing transcript abundance with CRISPRi and CRISPRa.
(a–b) Quantification of changes in gene expression in validation CRISPRi and CRISPRa cell lines and (c–d) Gene overexpression by CRISPRa produces changes in drug resistance that are correlated across K562 and Pfeiffer DLBCL cells, but are weaker in Pfeiffer. (a) Measurement of changes in mRNA levels by RT-qPCR in Pfeiffer CRISPRi cells transduced with individual sgRNAs for each of 9 knockdown screen hits. Controls 1 and 2 represent cell lines expressing non-targeting sgRNAs and the average of the two cell lines was used for normalization. Error bars are 95% confidence intervals (n = 3 technical repeats). (b) same as (a) except for K562 CRISPRa cell lines (left) and Pfeiffer CRISPRa cell lines (right). (c) Drug dose response measurements in Pfeiffer CRISPRa cells transduced with individual sgRNAs, for each of 8 overexpression screen hits, show changes in IC50 that are correlated with resistance phenotypes measured in the genome-wide CRISPRa screen in K562, but the magnitude of effects was weak in Pfeiffer. Error bars are 95% confidence intervals in IC50 (determined from curve fit; n = 3 biological repeats). Gray region: threshold in resistance score that was used to identify screen hits. (d) For the same screen hits in (c), gene overexpression by CRISPRa produces changes in IC50 in Pfeiffer DLBCL cells that are correlated but weaker than changes in IC50 measured in K562, where CRISPRa produces stronger induction of gene expression.
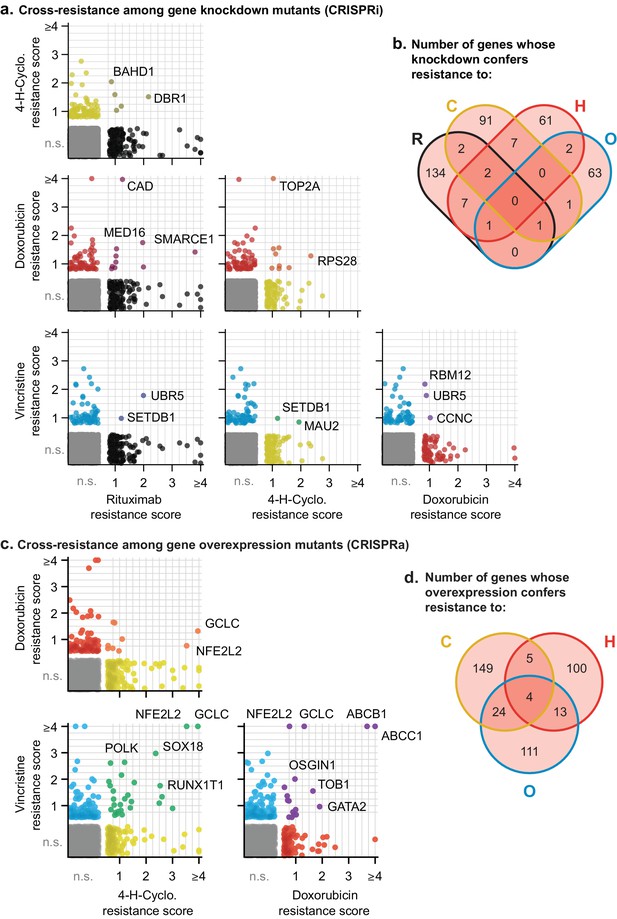
Cross-resistance analysis of the CRISPRi and CRISPRa screens reveals a small number of multi-drug resistance mechanisms.
(a) Scatter plots of resistance scores obtained in CRISPRi screens for each pair of drugs in RCHO; each dot represents a gene. Resistance scores were calculated from the product of the gene phenotype and the significance of the enrichment (-log10P). n.s., not significant; genes significant in one drug treatment but not in another are displayed against the left or bottom axis. Labeled genes are a partial list of top scoring hits. (b) Venn diagram of the number of genes whose knockdown confers resistance to one or multiple drugs in RCHO. (c) Scatter plots of resistance scores obtained in CRISPRa screens for each pair of drugs in CHO. Data were analyzed and displayed as in (a). (d) Venn diagram of the number of genes whose overexpression confers resistance to one or multiple drugs in CHO.
-
Figure 7—source data 1
Gamma growth scores for triple-resistant genes identified in CRISPRi screens.
This data was extracted from Figure 5—source data 3.
- https://cdn.elifesciences.org/articles/50036/elife-50036-fig7-data1-v2.xlsx
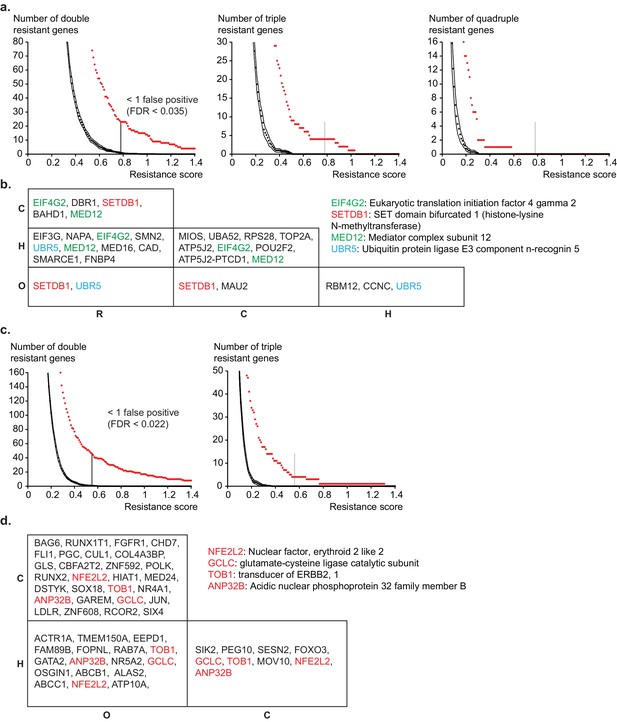
Determination of a cutoff threshold for cross-resistance analysis and identity of all cross-resistant genes for CRISPRi and CRISPRa screens.
(a) Plots of the total number of double-, triple- and quadruple-resistant genes as a function of the resistance score determined in the CRISPRi screens. Whole genome sets of negative control genes were simulated by randomly grouping sets of non-targeting sgRNAs. The resistance score for each negative control gene was then calculated for each drug CRISPRi screen. Black dots represent the average number of negative control genes (over a total of 10 simulated sets) that score as cross-resistant as a function of the resistance score. Black lines represent 90% confidence interval lines. Red dots represent the number of true cross-resistant genes. The threshold for cross-resistance analysis was set at a score that yielded less than an average of one double-resistant negative control gene. The false positive rate (FDR) was calculated by dividing the average number of double-resistant negative control genes by the number of true double-resistant genes. (b) Cross-resistant genes identified in the CRISPRi screens for RCHO. Genes that are triple-resistant are highlighted in colored font. (c) Plots of the total number of double- and triple-resistant genes as a function of the resistance score determined in the CRISPRa screens. Data were analyzed and displayed as for (a). (d) Cross-resistant genes identified in the CRISPRa screens for CHO. Genes that are triple-resistant are highlighted in red.
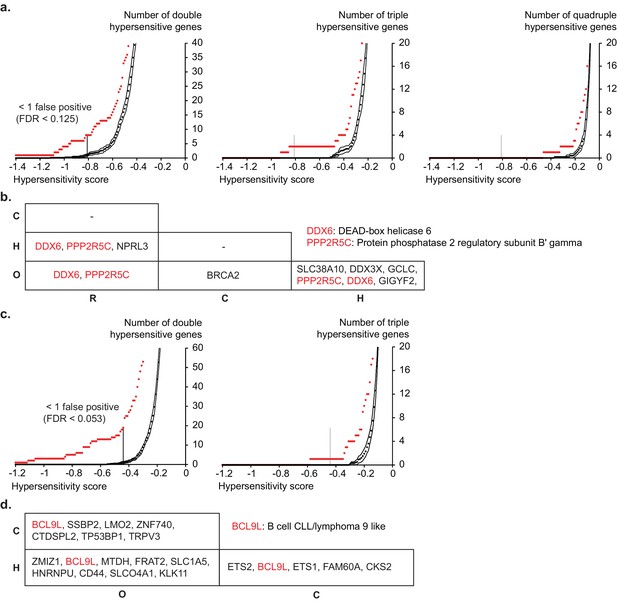
Determination of a cutoff threshold for cross-hypersensitivity analysis and identity of all cross-hypersensitive genes for CRISPRi and CRISPRa screens.
(a) Plots of the total number of double-, triple- and quadruple-hypersensitive genes as a function of the hypersensitivity score determined in the CRISPRi screens. Whole genome sets of negative control genes were simulated by randomly grouping sets of non-targeting sgRNAs. The hypersensitivity score for each negative control gene was then calculated for each drug CRISPRi screen. Black dots represent the average number of negative control genes (over a total of 10 simulated sets) that score as cross-hypersensitive as a function of the hypersensitivity score. Black lines represent 90% confidence interval lines. Red dots represent the number of true cross-hypersensitive genes. The threshold for cross-hypersensitivity analysis was set at a score that yielded less than an average of one double-hypersensitive negative control gene. The false positive rate (FDR) was calculated by dividing the average number of double-hypersensitive negative control genes by the number of true double-hypersensitive genes. (b) Cross-hypersensitive genes identified in the CRISPRi screen for RCHO. Genes that are triple-hypersensitive are highlighted in red. (c) Plots of the total number of double- and triple-hypersensitive genes as a function of the hypersensitivity score determined in the CRISPRa screens. Data were analyzed and displayed as for (a). (d) Cross-hypersensitive genes identified in the CRISPRa screen for CHO. Genes that are triple-hypersensitive are highlighted in red.
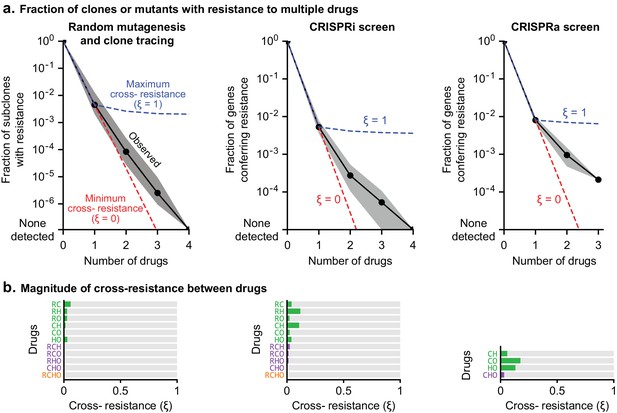
Cross-resistance between drugs in R-CHOP is close to its theoretical minimum.
(a) Fraction of clones or genetic perturbations resistant to one or more drugs in RCHO. Gray shading spans the range for different sets of drugs (e.g. six different pairs), and black points mark the average on a log scale. Dashed blue line: average frequency of Multi-Drug Resistance (MDR) if resistance is maximally overlapping (maximal overlap is the minimum of constituent single drug MDR frequencies; cross resistance parameter ξ = 1). Dashed red line: average frequency of MDR as the product of single-drug MDR frequencies with ξ = 0. (b) Strength of cross-resistance (ξ) for different sets of drugs in RCHO, as determined from data summarized in (a).
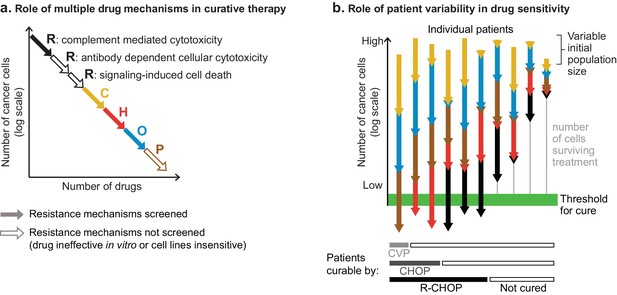
The role of multiple drug mechanisms in increasing the probability of cure by combination therapy.
(a) Conceptual schematic of the role of multiple drug mechanisms, each subject to different mechanisms of resistance, in the eradication of drug resistant clones and cure of a patient’s cancer. When drug cross-resistance is low (among cancer cells in one patient), the ‘log-kills’ achieved by each drug mechanism add up. (b) Conceptual schematic of the role of patient-to-patient variability in drug sensitivity. The number of cancer cells that survive n drugs is , where P0 is initial population, and fi is fraction of cells that survive drug i (on log-scale this is Log(P0) + ΣiLog(fi); note Log(fi) ≤ 0). The effect of combination therapy in each patient depends on initial tumor burden and the magnitude of effect of each constituent drug (f1, f2, etc), which varies across patients. Whether a patient is cured depends on the number of cancer cells surviving all drugs (vertical gray line), which can be zero in some patients and large in others, even if drugs are always additive and lack cross-resistance. Consistent with the clinical history of DLBCL, increasing the number of individually efficacious and non-cross-resistant drugs in combination therapies is expected to increase the fraction of patients cured; although an added drug could fail to improve efficacy if it lacks individual efficacy or is cross-resistant with drugs already given. It remains unresolved whether chemotherapy must eradicate every cancer cell.
Tables
Reagent type (species) or resource | Designation | Source or reference | Identifiers | Additional information |
---|---|---|---|---|
Cell line (Homo-sapiens) | Pfeiffer | ATCC (Cat# CRL-2632) | RRID:CVCL_3326 | Diffuse Large B-Cell Lymphoma |
Cell line (Homo-sapiens) | SU-DHL-4 | ATCC (Cat# CRL-2957) | RRID:CVCL_0539 | Diffuse Large B-Cell Lymphoma |
Cell line (Homo-sapiens) | SU-DHL-6 | ATCC (Cat# CRL-2959) | RRID:CVCL_2206 | Diffuse Large B-Cell Lymphoma |
Biological sample (Homo-sapiens) | Pooled human complement serum | Innovative Research | IPLA-CSER | |
Peptide, recombinant protein | Rituximab | Dana Farber Cancer Institute | 8 mg/mL in the clinical formulation + 10% Glycerol | |
Chemical compound, drug | 4-hydroperoxy-cyclophosphamide (4-H-Cyclo.) | Niomech | D-18864 | Pre-activated form of cyclophosphamide |
Chemical compound, drug | Doxorubicin | Selleck Chemicals | S1208 | |
Chemical compound, drug | Vincristine | Selleck Chemicals | S1241 | |
Chemical compound, drug | Prednisolone | Selleck Chemicals | S1737 | Pre-activated form of prednisone |
Commercial assay or kit | CellTiter-Glo | Promega | G7573 | Luminescent cell viability assay |
Chemical compound, drug | N-ethyl-N-nitrosourea (ENU) | Sigma Aldrich | N3385 | Mutation-inducing agent |
Recombinant DNA reagent | ClonTracer Barcoding library | Addgene (Cat# 67267) | RRID:Addgene_67267 | (Bhang et al., 2015) |
Software, algorithm | clonTracer_analyze v1.0 | (Bhang et al., 2015) | Script for analysis of barcode composition | |
Cell line (Homo-sapiens) | HEK293T | ATCC (Cat# CRL-3216) | RRID:CVCL_0063 | For lentivirus production |
Recombinant DNA reagent | psPAX2 | Addgene (Cat# 12260) | RRID:Addgene_12260 | Lentiviral packaging plasmid |
Recombinant DNA reagent | pCMV-VSV-G | Addgene (Cat# 8454) | RRID:Addgene_8454 | VSV-G envelope expressing plasmid for lentivirus production |
Cell line (Homo-sapiens) | Pfeiffer CRISPRi | This paper | See Materials and methods, Section ‘Generation of dCas9-expressing cell lines’ | |
Recombinant DNA reagent | pMH0001 | Addgene (Cat# 85969) | RRID:Addgene_85969 | Lentiviral construct for expression of dCas9-BFP-KRAB |
Cell line (Homo-sapiens) | K562 | ATCC (Cat# CCL-243) | RRID:CVCL_0004 | Chronic myeloid leukemia (CML) cell line |
Cell line (Homo-sapiens) | K562 CRISPRa | This paper | See Materials and methods, Section ‘Generation of dCas9-expressing cell lines’ | |
Recombinant DNA reagent | pHRdSV40-dCas9-10xGCN4_v4-P2A-BFP | Addgene (Cat# 60903) | RRID:Addgene_60903 | Lentiviral construct for expression of dCas9-SunTag |
Recombinant DNA reagent | pHRdSV40-scFv-GCN4-sfGFP-VP64-GB1-NLS | Addgene (Cat# 60904) | RRID:Addgene_60904 | Lentiviral construct for expression of scFv-sfGFP-VP64 |
Recombinant DNA reagent | pU6-sgRNA EF1Alpha-puro-T2A-BFP | Addgene (Cat# 60955) | RRID:Addgene_60955 | Lentiviral construct for expression of sgRNAs |
Recombinant DNA reagent | hCRISPRi_v2 | Addgene (Cat#83969 and 83970) | RRID:Addgene_83969 | Genome-wide human library of sgRNAs for CRISPRi |
Recombinant DNA reagent | hCRISPRa_v2 | Addgene (Cat# 83978 and 83979) | RRID:Addgene_83978 | Genome-wide human library of sgRNAs for CRISPRa |
Software, algorithm | ScreenProcessing pipeline | (Horlbeck et al., 2016) | https://github.com/mhorlbeck/ScreenProcessing | |
Cell line (Homo-sapiens) | Pfeiffer CRISPRa | This paper | See Materials and methods, Section ‘Validation of CRISPR screens’ |
Additional files
-
Supplementary file 1
List of oligonucleotides used in this study and sequence of sgRNA protospacers used in individual CRISPRi/a cell line construction.
- https://cdn.elifesciences.org/articles/50036/elife-50036-supp1-v2.xlsx
-
Transparent reporting form
- https://cdn.elifesciences.org/articles/50036/elife-50036-transrepform-v2.pdf