Right inferior frontal gyrus implements motor inhibitory control via beta-band oscillations in humans
Figures
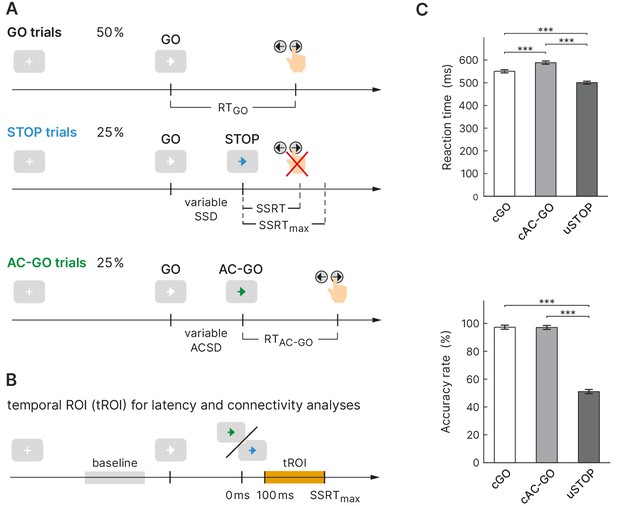
Experimental design and behavioral performance of the selective stopping task.
(A) The task comprised three conditions: a GO condition (white arrow), a STOP condition (arrow changes color to blue), and an attentional capture GO (AC-GO) condition (arrow changes color to green). The variable stop signal delay (SSD) was adapted to the participants’ performance to yield a probability of 50% of successful response inhibitions per block. SSRT, stop signal reaction time, ACSD, attentional capture signal delay (analog to SSD). (B) Temporal region of interest (orange box) for latency and connectivity analysis starts at 100 ms, where early visual processing is likely complete and ends at SSRTmax (maximal SSRT across participants, 350 ms). (C) Reaction time (RT) and accuracy rate in correct GO (cGO), correct attentional capture GO (cAC-GO), and unsuccessful stop trials (uSTOP); mean ± standard error of the mean. ***p<0.001, based on Bonferroni-corrected post hoc comparisons of a repeated-measures ANOVA, n = 59.
-
Figure 1—source data 1
Behavioral data used for the statistics in panel (C).
- https://cdn.elifesciences.org/articles/61679/elife-61679-fig1-data1-v2.csv
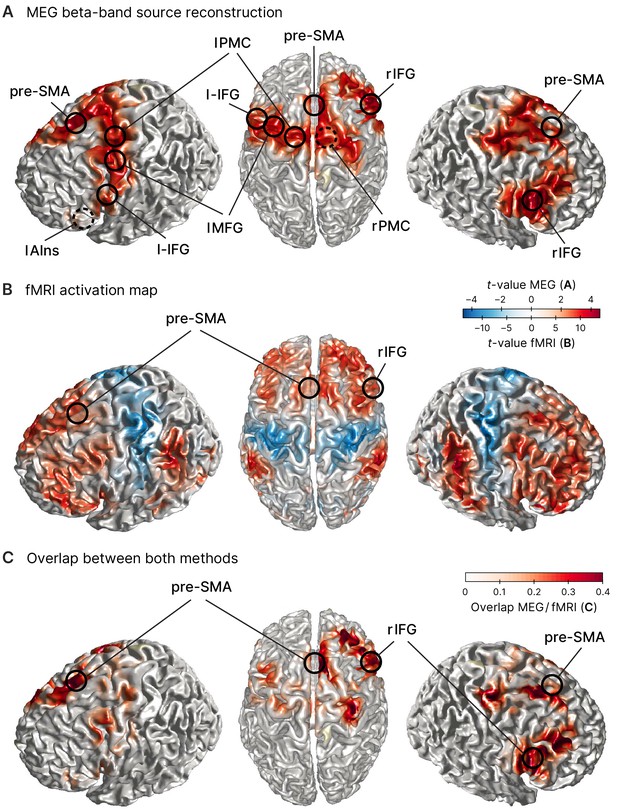
Sources involved in successful stopping.
(A) Source reconstruction of MEG data in the beta band (12–32 Hz, 100–373 ms after STOP/AC-GO [attentional capture go] signal onset). Surface plots show t-values of significant clusters when contrasting successful stop (sSTOP) and correct attentional capture go (cAC-GO) trials (cluster-based permutation test, two-tailed, cluster = 0.05, n = 59). Peak voxels (local extrema) of these clusters are highlighted and labeled (MNI coordinates are shown in Table 1). Dashed circles indicate that the peak voxel is not directly visible on the surface. Anatomical regions: rIFG, right inferior frontal gyrus, pre-SMA, pre-supplementary motor area, l-IFG, left inferior frontal gyrus, lAIns, left anterior insula, lMFG, left middle frontal gyrus, lPMC, left premotor cortex, rPMC, right premotor cortex. (B) fMRI activation maps for the contrast sSTOP versus cAC-GO; map thresholded pFWE < 0.05, cluster extent k = 5 voxels, n = 76. Positive activation, that is, positive -values (red color), is obtained by the contrast sSTOP > cAC-GO, while negative activation, that is, negative -values (blue color), is obtained by the contrast sSTOP < cAC-GO. Data are taken from Sebastian et al., 2017. (C) Overlap between MEG (A) and fMRI (B) activity. To compare the activity revealed by both methods, the product of normalized -values from both methods is displayed. Peak voxels of right inferior frontal gyrus (rIFG) and pre-supplementary motor area (pre-SMA) as found in the MEG analysis are highlighted and labeled.
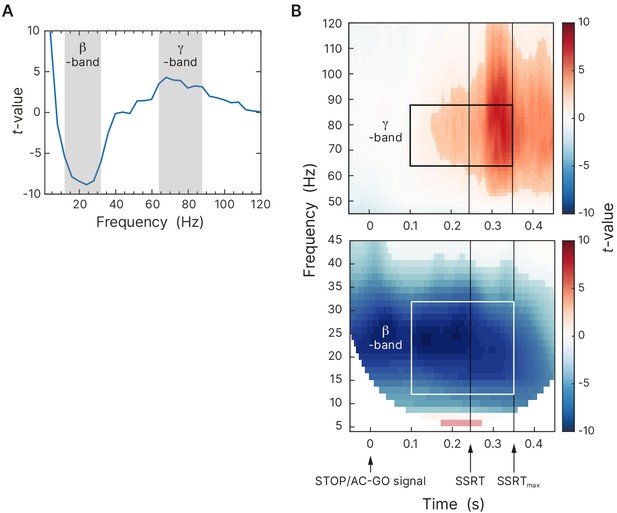
Activation versus baseline statistics at sensor level.
To identify appropriate frequency bands as beamformer parameters for source reconstruction, activation-versus-baseline statistics were performed at the sensor level for combined conditions (successful stop and correct attentional capture go). (A) Two significant frequency bands (highlighted in gray) were revealed by a clustered-based permutation statistics (two-tailed, = 0.01, n = 59) based on spectral power averaged over the temporal region of interest (tROI, 100–350 ms) and all sensors (beta band, 12.0–31.9 Hz, and gamma band, 63.8–87.7 Hz). (B) Time-frequency representations, averaged over all sensors, showed a significant broadband deactivation in the beta band extending over the whole tROI (clustered-based permutation statistics [two-tailed, = 0.05, n = 59]). Vertical solid lines represent median and maximum stop signal reaction time (SSRT). The white/black box represents the time-frequency window used for source reconstruction in the beta/gamma band.
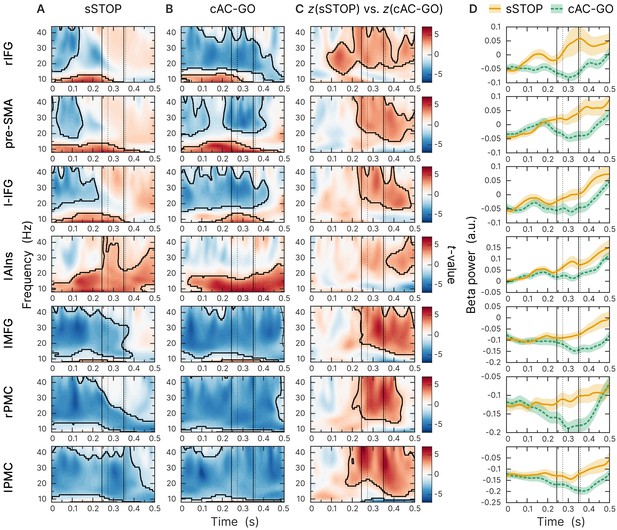
Beta-band time-frequency representations of virtual channels placed at sources identified.
First and second solid lines indicate stop signal reaction time (SSRT) and SSRTmax; first and second dotted lines indicate 10% and 50% percentiles of RTAC-GO for selected AC-GO trials with RTAC-GO > SSRT (RTAC-GO is the duration between AC-GO signal and button press, see Figure 1A). Time axis locked to STOP and AC-GO signal (0 s). All plots show the results of a cluster-based permutation test, two-tailed, cluster = 0.05, n = 59, significant clusters are outlined. (A) Task-versus-baseline power for successful stop (sSTOP) trials. (B) Task-versus-baseline power for correct attentional capture go (cAC-GO trials). (C) Contrast of z-transformed sSTOP versus cAC-GO trials. (D) Averaged beta-band power (12–32 Hz). Orange curve (solid line): sSTOP trials, green curve (dashed line): cAC-GO trials, bounded lines: standard error of the mean.
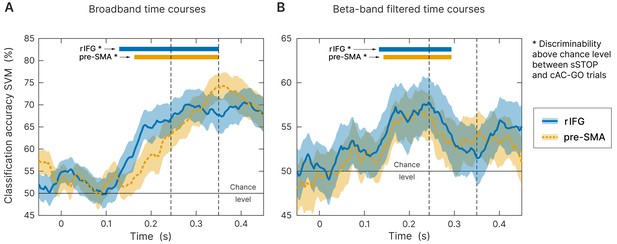
Time-resolved support vector machine (SVM) analysis.
Both trial types (successful stop [sSTOP] and correct attentional capture go [cAC-GO]) were robustly classified above chance within the temporal region of interest (tROI) (100–350 ms) across subjects at right inferior frontal gyrus (rIFG) (blue curve, solid line) and pre-supplementary motor area (pre-SMA) (orange curve, dashed line). As input, time-embedded vectors of 14 consecutive samples were used, corresponding with one beta cycle at the center frequency of 22 Hz (see 'Materials and methods'). Significance for above chance-level accuracy was tested between 100 and 350 ms. Significant classifications within this range are highlighted by colored bars above the curves and onsets are indicated by arrows. Bounded lines: 95% confidence intervals of the mean, n = 59. First and second dashed lines indicate stop signal reaction time (SSRT) and SSRTmax. (A) Broadband time course data as input for the SVM. While above-chance level onset for rIFG was at 129 ms (p<0.0001), pre-SMA was above chance at 163 ms (p<0.0001). (B) Same analysis applied on beta-band filtered data (12–32 Hz). While above-chance level onset for rIFG was at 132 ms (p<0.0001), pre-SMA was above chance at 142 ms (p<0.0001).
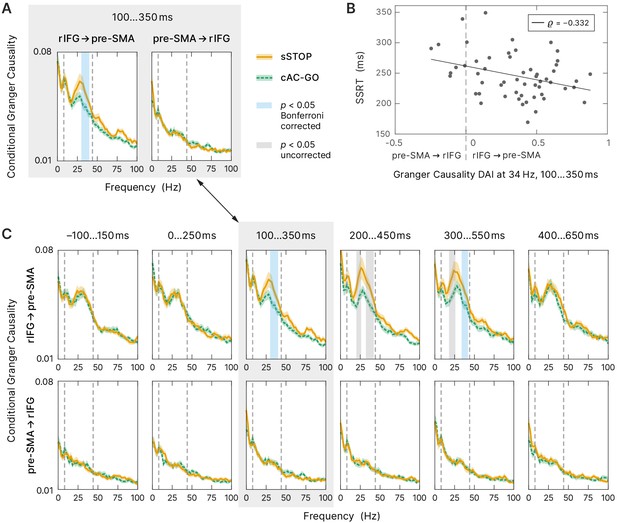
Connectivity analysis.
(A) Spectral-resolved conditional Granger causality (cGC) between right inferior frontal gyrus (rIFG) and pre-supplementary motor area (pre-SMA). Orange curve (solid line): cGC for successful stop (sSTOP) trials, green curve (dashed line): cGC for correct attentional capture go (cAC-GO) trials, bounded lines: standard error of the mean. A cluster-based permutation test was used to identify significant differences between both conditions. Significant differences (p<0.05) are highlighted in blue if Bonferroni corrected, that is, the two links tested, and in gray if uncorrected. The frequency range tested (8–44 Hz) is indicated by vertical dashed lines. n = 58 for the link rIFG to pre-SMA, n = 57 for pre-SMA to rIFG (subjects with average cGC below bias level in both conditions were excluded). (B) Stop-signal reaction time (SSRT) correlated with directed influence asymmetry index (DAI) for rIFG and pre-SMA cGC values (sSTOP trials, cGC at 34 Hz in the temporal region of interest [tROI], 100–350 ms). Positive DAI corresponds to links from rIFG to pre-SMA, while negative DAI corresponds to links from pre-SMA to rIFG. The negative correlation indicates that participants with higher cGC from rIFG to pre-SMA are the better inhibitors (faster SSRT). n = 55 with average cGC reliably above bias level (note that only sSTOP trials has been used here). (C) Temporal evolution of spectral-resolved cGC was analyzed post hoc between rIFG to pre-SMA (top) and pre-SMA to rIFG (bottom). A sliding window was shifted with 100 ms steps around the tROI. cGC from rIFG to pre-SMA (but not in the opposite direction) was significantly higher for sSTOP compared to cAC-GO trials in the tROI itself and in a later time window tested. Here, we corrected for testing six time windows and for testing in two directions (rIFG to pre-SMA and vice versa). Same formatting as in (A). Spectra of the tROI, highlighted with a gray-colored box, are also shown in (A).
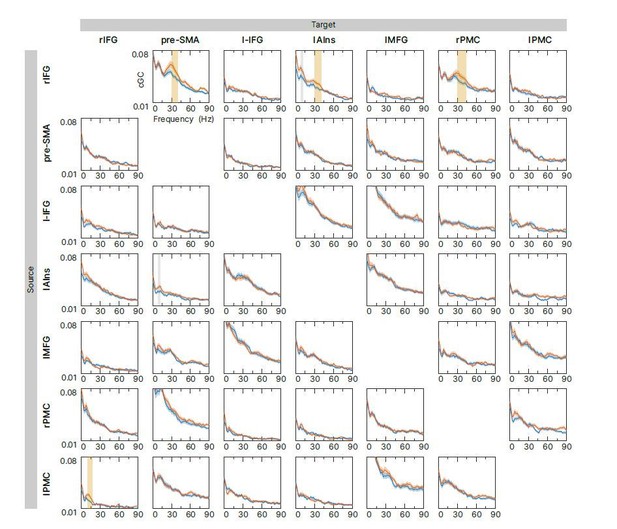
Exploratory connectivity analysis during the tROI.
Spectral-resolved conditional Granger causality (cGC) between all source target pairs (links) was analyzed. Blue curve: cGC for sSTOP trials, green curve: cGC for cAC-GO trials, bounded lines: standard error of the mean. There were no significant differences between both conditions in the frequency range of 8-44Hz when correcting for all 42 links. However, significant differences were found if correction for multiple comparisons was reduced to six links (highlighted in yellow). Uncorrected significance is highlighted in grey.
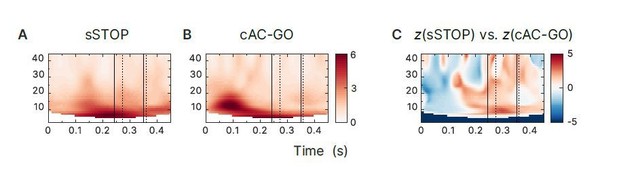
Time-frequency representations of evoked responses in rIFG.
First and second solid line indicate SSRT and SSRTmax, first and second dotted line indicate 10% and 50%=percentiles of RTAC-GO for selected AC-GO trials with RTAC-GO > SSRT (RTAC-GO is the duration between AC-GO signal and button press). Time axis locked to STOP and AC-GO signal (Os). (A) To obtain evoked responses, successful stop (sSTOP) trials were averaged (for baseline correction, the mean of a 500ms long baseline segment that ended 100ms before the Go signal was used, no low-pass filter applied) and then transformed to TRFs. (B) Same as in (A), but for correct attentional capture go trials (cAC-GO). (C) Contrast of sSTOP versus cAC-GO trials cluster-based permutation test, tow-tailed, αcluster = 0.05, n=59, no significant clusters found
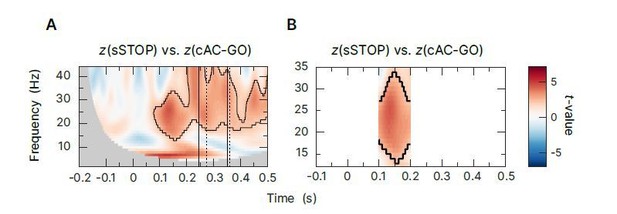
Time-frequency statistics focused on early β-band cluster in rIFG.
Contrast of z-transformed sSTOP versus cAC-GO trials (cluster-based permutation test, two-tailed, αcluster = 0.05, n=59, significant cluster is outlined). (A) Tested between -200-500ms and 2-44Hz as in the analysis showed in Figure in the revised manuscript. (B) Statistics performed in a smaller time window (100-200ms) and only between 12-35Hz. Thus, we tried to exclude that the early significant cluster revealed by the original analysis is just spilling over from the larger and later β-rebound that would survive permutation-based statistics more easily than a smaller, separate cluster. And indeed, although we specifically tested around the outline of the early cluster in rIFG, it was still significant and not a spill-over from other clusters.
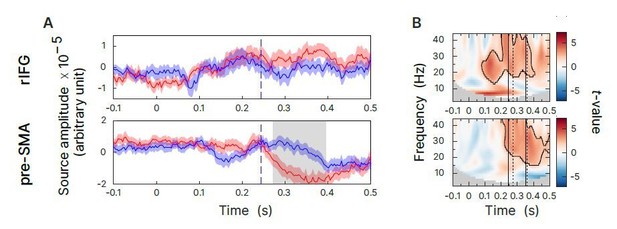
Additional analysis of evoked responses and very low frequency oscillations.
(A) Statistical comparison of evoked responses, sSTOP red curve, cAC-GO blue curve. A cluster-based permutation test was performed between 0 and 500 ms. Significant difference are highlighted by grey boxes. (B) Oscillator analysis including very low frequencies (2-44Hz). Here, the contrast of z-transformed sSTOP versus cCA-GO trials is show (Cluster-based permutations test, two-tailed, αcluster = 0.5, n=59, significant clusters are outlined).
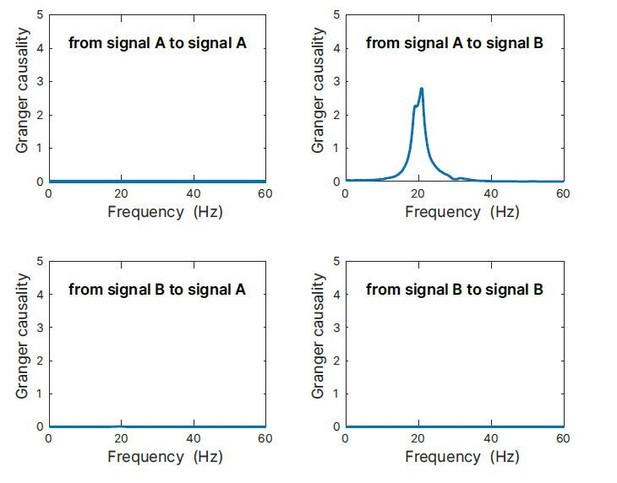
Granger causality simulation.
Simulation results of an auto-regressive model of a coupled system, where process A is oscillating with 20Hz and process B with 30 Hz, and where A is driving B with a delay of 16.67 ms. When analysing spectral Granger causality, we would expect no GC from A→A, B→A, and B→B. Only A→B should reveal a 20 Hz component. Indeed, this is what we obtained as results by our simulation. Thus, it is well possible to detect Granger causality of signal with a period (50 ms) that is considerably larger than the coupling delay (16.67 ms).
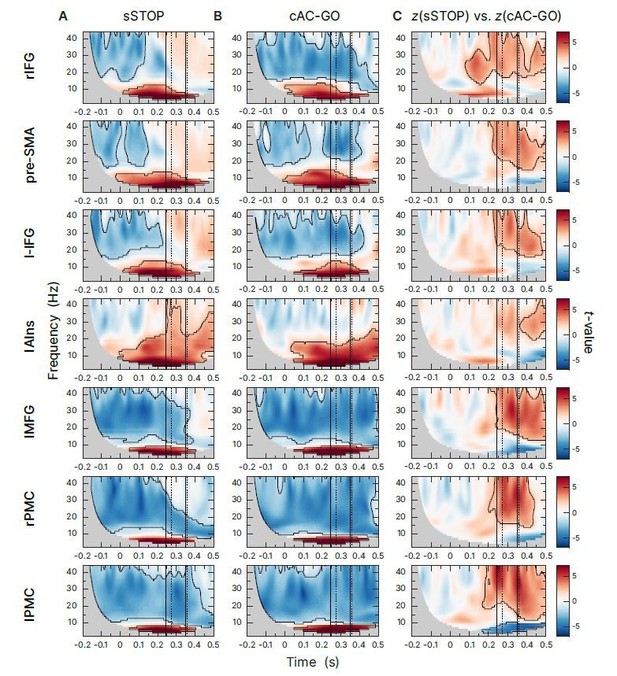
Β-band time-frequency representations of virtual channels at sources identified.
First and second solid line indicate SSRT and SSRTmax, first and second dotted line indicate 10% and 50%-percentiles of RTAC-GO for selected AC-GO trials with RTAC-GO > SSRT (RT AC-GO is the duration between AC-GO signal and button, see Figure 1 of the manuscript). Time axis locked to STOP and AC-GO signal (0s). All plots shows the results of a cluster-based permutation test, two-tailed, αcluster = 0.05, n=59, significant cluster are outlined. (A) Task-versus-baseline power for successful stop (sSTOP) trials. (B) Task-versus-baseline power for correct attentional capture go (cAC-GO trials). (C) Contrast of z-transformed successful stop (sSTOP) versus correct attentional capture go (cAC-GO) trials.
Tables
MEG source reconstruction in the beta band.
MNI coordinates obtained as peak voxels when contrasting successful stop (sSTOP) versus correct attentional capture GO (cAC-GO) trials.
Coordinates | ||||
---|---|---|---|---|
Region (label) | -Value | |||
Inferior frontal gyrus BA44/45 R (rIFG) | 50 | 20 | 20 | 3.654 |
Inferior frontal gyrus BA44/45 L (l-IFG) | –50 | 10 | 20 | 2.820 |
Anterior insula/left IFG (lAIns) | –40 | 30 | 0 | 2.894 |
Pre-supplementary motor area (pre-SMA) | 0 | 20 | 60 | 3.878 |
Middle frontal gyrus (lMFG) | –40 | 0 | 50 | 3.360 |
Premotor cortex BA6 R (rPMC) | 10 | –10 | 50 | 4.015 |
Premotor cortex BA6 L (lPMC) | –20 | –10 | 60 | 3.571 |
Fornix | 0 | 0 | 10 | 3.740 |