Science Forum: Improving preclinical studies through replications
Abstract
The purpose of preclinical research is to inform the development of novel diagnostics or therapeutics, and the results of experiments on animal models of disease often inform the decision to conduct studies in humans. However, a substantial number of clinical trials fail, even when preclinical studies have apparently demonstrated the efficacy of a given intervention. A number of large-scale replication studies are currently trying to identify the factors that influence the robustness of preclinical research. Here, we discuss replications in the context of preclinical research trajectories, and argue that increasing validity should be a priority when selecting experiments to replicate and when performing the replication. We conclude that systematically improving three domains of validity – internal, external and translational – will result in a more efficient allocation of resources, will be more ethical, and will ultimately increase the chances of successful translation.
Introduction
Translation from preclinical research to patients is challenging for many reasons (Denayer et al., 2014; Pound and Ritskes-Hoitinga, 2018). Biological complexity and the disparity between animal models of disease and humans accounts for some failures in translation, but not all (Kimmelman and London, 2011; Mullane and Williams, 2019; van der Worp et al., 2010). Other reasons include the fact that the evidence generated in preclinical efficacy studies is often weak due to the low numbers of experimental units or entities receiving a treatment (Bonapersona et al., 2019; Carneiro et al., 2018; Howells et al., 2014). Moreover, analyses are often not reported in full, leading to the selective reporting of outcomes and the exploitation of researcher degrees of freedom (Motulsky, 2014). False positives abound in such studies, and reported effects are often inflated (Dirnagl, 2020; Kimmelman et al., 2014; Turner and Barbee, 2019). Moreover, a strong bias against the publication of non-significant results augments this problem (Sena et al., 2010) and makes meta-analytic assessment of preclinical evidence difficult (Sena et al., 2014).
An obvious way to address some of these problem would be for other research groups to reproduce and then replicate preclinical studies before starting experiments on humans. By reproduce we mean to be in principle able to repeat the original study through in depth understanding of methods, protocols, and analytical pipelines used by the original research group. By replicate we mean to actually perform a study to see if the findings of the original study still hold. This potentially involves adapting some methods, protocols, and analytical pipelines (Nosek and Errington, 2020a; Patil et al., 2016): for example, when different animal strains are used or when environmental factors are changed (Voelkl et al., 2020). Reproducibility is thus a prerequisite for engaging in replications that will increase our confidence in a finding through its wider validity.
Here, we consider the role of replications in the context of the preclinical research trajectory for a potential treatment: such a trajectory is a series of experiments designed to generate evidence that will inform any decision about testing the treatment in humans. The experiments in a preclinical research trajectory typically include exploratory studies, toxicity studies, positive and negative controls, pharmacodynamics and kinetics, and are intended to generate evidence to support an inferential claim and refute possible alternatives. They can be performed on animal models (including invertebrates, zebrafish, nonhuman primates and, quite often, rodents) or with replacement methods (such as cell cultures and organoids).
Within this framework, replications strengthen two key characteristics of preclinical experimental evidence: validity and reliability. Validity refers to the degree to which an inference is true, and reliability refers to the quality and accuracy of the data supporting an inferential claim. In this article we describe strategies for preclinical research trajectories in which replications balance reliability and validity to foster preclinical and translational research in a way that is ethical and efficient. For example, consider a researcher who hypothesizes that a disease is caused by a metabolic product. A potential drug candidate will inhibit an enzyme that is involved in the relevant metabolic process. In an exploratory study, applying the drug in a knockout mouse model of the disease reduced the metabolic product and the health condition of the animals improved. A within-lab replication confirms these initial findings. The findings are reliable as initial data and replication support the inferential claim that the drug improves health conditions. However, this does not necessarily mean that the inference is valid, particularly when extrapolated to humans: as we will outline below, the validity of such an inference can be threatened on several levels during the preclinical research trajectory.
What to replicate and how to replicate
A number of large-scale replication projects have been conducted in psychology and social sciences (Camerer et al., 2016; Camerer et al., 2018; Open Science Collaboration, 2015). Those studies were performed with healthy subjects with little to no harm anticipated through participation. Consequently, increasing the number of tested subjects was not ethically problematic or overly expensive. The same is not true for projects that involve animals, so there have been relatively few large-scale replication projects in biomedical research. Moreover, the projects that have been started – such as the Reproducibility Project: Cancer Biology, the Brazilian Reproducibility Initiative, and the Confirmatory Preclinical Studies project – all take different approaches to identifying the studies to be replicated and to performing the replications (see Table 1 and Box 1).
Overview of three large-scale replication projects in biomedical research: Reproducibility Project: Cancer Biology (RPCB); Brazilian Reproducibility Initiative (BRI); Confirmatory Preclinical Studies (CPS).
RPCB | BRI | CPS | |
---|---|---|---|
Selection of samples to be replicated | Main findings from 50 high impact citations/publications in cancer research | Replication of 60–100 experiments from research articles of Brazilian studies in different clinical areas | Two-step review process of proposals results in twelve projects |
Selection of experiment | Main finding from published studies | Experiments using five pre-defined methods | Own experiments |
Replicate own results | No | No | Yes |
Exact Protocols | Yes (consulting original authors) | No | Yes |
Blind to initial results | No | Yes | No |
Pre-registration | Pre-registered study and individual Replication Protocols | Yes | Yes |
Multi-site replication | No | Yes | Yes |
Box 1.
Three approaches to large-scale replication projects in biomedical research.
The Reproducibility Project: Cancer Biology (RPCB) started with the aim of reproducing selected findings from 50 high-impact articles published between 2010 and 2012 in the field of cancer biology (Errington et al., 2014; Morrison, 2014). The plan was to publish a peer-reviewed Registered Report that outlined the protocols for each attempted reproduction – based on information contained in the original paper and, if necessary, additional information obtained from the original authors – before any experiments were performed (Nosek and Errington, 2020b). The experiments were to be conducted by commercial contract research organizations and academic core facilities from the Science Exchange network, and the results were to be published in a separate peer-reviewed Replication Study. The researchers performing the experiments were not blinded with regard to the original results. In the end, due to various problems, only 29 Registered Reports and 18 Replication Studies were published, and the overall conclusions of the project are currently being written up. The aim of the Brazilian Reproducibility Initiative is to assess the reproducibility of biomedical science published by researchers based in Brazil (Amaral et al., 2019; Neves et al., 2020). The studies selected had to use one of five experimental techniques, including behavioural and wet lab methods, on certain widely-used model organisms. The BRI researchers assume that protocols will never be reproduced exactly so they employ a ‘naturalistic approach’ in which the teams repeating the experiments can supplement the published protocols based on their best judgement and experience. Moreover, three teams will attempt to repeat each study selected, and will preregister their protocols before starting experiments. Furthermore, the researchers performing the experiments will be blinded to the identity of the original authors and the results of the paper. Recently, the Federal Ministry of Education and Research in Germany invited research groups to apply for funding to attempt to confirm promising results from their own preclinical studies (BMBF-DLR, 2018). After being screened and reviewed by a panel of international experts, 12 groups have received funding under the CPS (Confirmatory Preclinical Studies) project: one condition of the project is that the groups funded have to collaborate with other groups (of their choosing) in a multi-centre approach with a view to harmonising protocols across sites. Again the groups will have to pre-register their protocols: however, as the researchers are repeating their own experiments, they will not be blinded to the original results.
Based on the results from the study being replicated and the stage in the preclinical research trajectory, the question is: what additional evidence is needed to ultimately decide to start trials in human subjects? Throughout a sequence of preclinical experiments, validity and reliability have to be adapted at each stage, and criteria are set so that we know whether to continue, to revise, or even completely break off the experiments (Figure 1). How, for example, could the previous experiments be improved? How many animals should be tested? Are additional controls needed? Should additional labs be involved? At all stages the aim should be to increase the validity of replications and test the reliability of the initial finding (Dirnagl, 2020; Kimmelman et al., 2014; Piper et al., 2019).
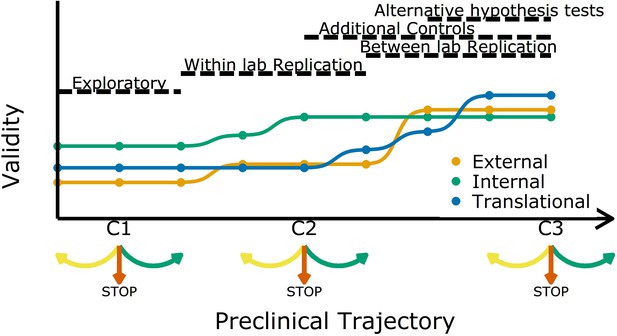
Increasing different forms of validity (internal, external and translational) during a preclinical research trajectory.
This schematic shows that internal validity (green line) is higher than external validity (orange) and translational validity (blue) at the start of a preclinical research trajectory (left), and that evidence from different types of experiments can increase different types of validity. For example, evidence from exploratory studies can increase internal and external validity, and evidence from between-lab replications can increase translational validity. C1, C2 and C3 are decision points where researchers can decide to refine the current experiment (yellow arrow), stop the trajectory (red arrow), or proceed to the next experiment (green arrow).
Researchers usually start off with a study in exploratory mode. As not all details and confounders in such a study can be known upfront, the reliability and validity are potentially not fully optimized. However, even at these early stages researchers should implement strategies to mitigate risks of bias (Figure 1). After an initial phase, the question is: should we continue to test a particular claim? The criteria used to answer this question should be lenient: standard p-value criteria are potentially too strict and identifying the range of possible effect sizes is a viable goal in early phases. Particularly in fields with a low prevalence of true hypotheses, this will prevent discarding promising treatments too early (Albers and Lakens, 2018; Lakens, 2014). More stringent criteria should be applied in later experiments. Standard p-values will then identify true effects in high powered replications, which will reduce Type I errors and increase the predictive value of a set of experiments.
As we move along the research trajectory it also becomes helpful to think in terms of three types of validity: internal validity, external validity, and translation validity (Figure 1). To illustrate this, we return to the example of a disease caused by a metabolic product. An experiment in which the inhibition of a metabolic pathway leads to an improvement in animal health is internally valid if the measured effect (improved health) is caused by the experimental manipulation (administration of the inhibitor). Such an experiment is also externally valid if the effect is observed in other animal models and/or can be replicated in other labs. And if the effect is also observed in humans it is translationally valid. As we shall discuss, different strategies are needed to improve these three types of validity.
Internal validity
Poor study design and the lack of control for biases are major contributors towards low internal validity (Bailoo et al., 2014; Pound and Ritskes-Hoitinga, 2018; Würbel, 2017). To increase internal validity, controlling for selection and detection bias is essential. For this, methods of randomization and blinding need to be clearly specified, and inclusion and exclusion criteria need to be defined, before data are collected (Würbel, 2017). Replications can be leveraged to improve this further. Imagine, for example, that an exploratory study has found that a physiological factor (such as animal weight) influences the primary outcome variable: in the next round of experiments, animals could be randomized and stratified by weight. Particularly for replications, all these choices (along with detailed methods and analysis plans) should be communicated before conducting the experiment, ideally via pre-registration at a platform such as http://www.animalstudyregistry.org or http://www.preclinicaltrials.eu.
Methods and analyses also need to be reported transparently and completely (Vollert et al., 2020; Percie du Sert et al., 2020). However, completely reported experiments can still have low internal validity because following a reporting guideline will not safeguard against suboptimal experimental design: that is, experiments need high internal validity and complete reporting to prevent research waste and fruitless animal testing (Macleod et al., 2014).
The three replication projects described in Box 1 all aim at high internal validity through exact specifications of replication protocols. Within the RPCB, for example, protocols were pre-registered, peer reviewed and published before replication experiments were performed. However, in some cases where results have been difficult to interpret, exact pre-specification of replication experiments has limited the possibility of performing the experiments in another, potentially improved way (eLife, 2017). The BRI describes a more naturalistic approach where each participating lab will fill the gaps in papers as best as they can without consulting primary authors. Nevertheless, protocols will still be preregistered and undergo a round of internal peer review among collaborating labs. In summary, high levels of methodological rigour are necessary to ensure high internal validity and to make replications meaningful.
External validity
For the assessment of external validity, research findings from one setting need to generalise to other settings (Pound and Ritskes-Hoitinga, 2018). One way to increase external validity is to conduct replications at multiple sites, emulating an approach already applied in clinical trials (Dechartres et al., 2011; Friedman et al., 2015). Multi-centre studies (or between-lab replications at a single centre) can index known and unknown differences that define boundary conditions for investigated effects (Glasgow et al., 2006; Pound and Ritskes-Hoitinga, 2018). Regarding generalizability, external validity can often be improved by including aged or comorbid animals, and by performing multimodal studies with animals of different sex and/or varying strains. This systematically introduced heterogeneity strengthens external validity and explores the extent to which standardization is introducing unwanted idiosyncrasies that may limit external validity and prevent successful replication (Richter et al., 2010; Voelkl et al., 2018). Additionally negative and positive control groups that are added to replications can further foster external validity (Kafkafi et al., 2018).
Again, the three replication projects described in Box 1 take different approaches. The BRI and CPS projects take multi-centre approaches, whereas the RPCB does not, which may result in lower external validity. However, multi-centre studies have their own limitations and shortcomings as they come with an organizational overhead that includes decisions on which parts of experiments should be standardised and safeguarding adherence to the agreed protocols throughout a study (Maysami et al., 2016). This can be challenging, given different infrastructure and/or resources across laboratories with different budget or resource constraints.
Difference in equipment can be one reason for variation in performing a certain intervention (e.g. surgery). Moreover, if the centres are in different countries, ethics boards and local regulations will most likely differ, complicating and potentially delaying ethics approval (Hunniford et al., 2019; Llovera et al., 2015; Maysami et al., 2016). For example, regulations for analgesic regimes differ between jurisdictions, so that it is difficult to follow the same protocol across sites. Nevertheless, multi-centre studies are characterised by high quality standards with cross validation of results, larger sample size, lower risk of bias as compared to single-centre studies, and higher completeness of reporting (Hunniford et al., 2019). This strongly suggests that a multi-centre approach will be an important component in enabling decisions about clinical trial initiation (Prohaska and Etkin, 2010).
Translational validity
Translational validity is used here as an umbrella term for factors that putatively contribute to the translation from animal models to humans. In particular, it pertains to how well measurements and animal models represent a certain disease and its underlying pathomechanisms in humans, as it is common for only a limited number of disease characteristics to present in animal models. In models of Alzheimer’s disease, for example, the focus on familial early onset genes in mouse models has potentially led to translational failures as the majority of diagnoses of Alzheimer’s disease in humans are classified as sporadic late onset form (Mullane and Williams, 2019; Sasaguri et al., 2017). Translational validity thus reflects whether measured parameters in animal models are diagnostic for human conditions and consequently, to what extent the observed outcomes will predict outcomes in humans (Denayer et al., 2014; Mullane and Williams, 2019).
Ideally, auxiliary measures are collected alongside the primary outcome variable of the initial study. While such secondary outcomes might not be recorded in early experiments, replications are ideally suited to including these additional measures. Clinical biomarkers that are diagnostic for a disease in humans can provide information on the translational potential if collected also in replications in animal models (Metselaar and Lammers, 2020; Volk et al., 2015). In this context, if an imaging method like MRI is used in the diagnosis of humans, the same method applied in animals can reveal whether physiological parameters and disease location are comparable. This can be combined with experiments that gather converging and discriminant evidence to identify mechanistic underpinnings of an intervention to increase translational validity and identify limitations and boundary conditions. For example, studies of pharmacodynamics and kinetics in preclinical models support in-depth understanding of physiological processes and allow comparison with human pharmacological processes (Salvadori et al., 2019; Tuntland et al., 2014).
Replication studies can also be performed in a more complex animal model. In cancer research, for example, the initial study might be performed in an animal model with a subcutaneous tumour, while the replication could be conducted in a more advanced tumour model, in which the development of an organ-specific tumour microenvironment more closely mimics the clinical reality (Guerin et al., 2020). The decision on which additional information will be helpful should be based on an exchange between preclinical researchers and clinicians.
Therefore, translational validity needs to be considered at each stage during preclinical research.
Ethical conduct of replications
To optimize evidence from experiments on animals it is necessary to balance the different types of validity and reliability of experiments and replications. Early on, internal validity needs to be established with high priority. As knowledge about the animal model and disease mechanisms increases, external validity needs to be strengthened through within-lab replications and, for core results, to multiple centres. Systematic heterogeneity (additional strains, similar animal models, different sexes) will further strengthen external validity, and such heterogeneity should be introduced at the early stages of the work if this is feasible.
Replications at such later stages should also include secondary outcomes that directly link to clinically relevant parameters. However, even in high-validity experiments, reliability can be low when the number of experimental units is not sufficient to detect existing effects. For replications at this stage, reliability should be increased by increasing sample sizes or refining measurement procedures. As true preclinical effect sizes are frequently small and associated with considerable variance between experimental units, increased numbers of experimental units are needed to obtain reliable results (Bonapersona et al., 2020; Carneiro et al., 2018). According to the 3R principles (Russell and Burch, 1959), the number of animals tested needs to reflect the current stage in the preclinical trajectory (Sneddon et al., 2017; Strech and Dirnagl, 2019).
There is, however, no consensus yet on how to balance ethical and statistical power considerations in replications in animal experiments. Standard approaches where power calculations are based on the point estimate of the initial study will often yield too small animal numbers (Albers and Lakens, 2018; Piper et al., 2019). This potentially inflates false negatives, running the risk of missing important effects and wrongfully failing a replication. Alternatives like safeguard power analysis (Perugini et al., 2014), sceptical p-value (Held, 2020), or adjusting for uncertainty (Anderson and Maxwell, 2017) have been proposed mainly for psychological experiments with human subjects. These approaches will often yield high enough sample sizes to ensure sufficient power for replications. Due to ethical and resource constraints, preclinical replications are seldom able to test such high numbers, which may be one reason why such approaches have not yet been implemented widely in preclinical research design. Here, we see clear room for improvement and research opportunities.
Regarding the number of experimental units, the RPCB aimed at achieving at least 80% statistical power, based on the effect size measured in the original study. However, the 'winner's curse' means that published effect sizes (p<0.05) tend to be larger due to random sample variability. So basing the design of a replication on the effect size reported in the original study could result in the replication being underpowered (Colquhoun, 2014).
The BRI team calculated sample sizes to achieve a statistical power of 95% to detect the original effect in each of the three replications (which will be conducted in different labs). Through this, the BRI team tried to compensate for a possible inflation of the original results due to publication bias and winner’s curse. Furthermore, they planned to include additional positive and/or negative controls to ensure interpretation of the outcomes (Neves and Amaral, 2020). The different approaches taken by RPCB and BRI also confirm that there is, as yet, no consensus on how to calculate animal numbers towards an ethical conduct of replications.
Summary and recommendations
The goal of a preclinical research trajectory is to enable the decision to engage in clinical studies. In a simplified scheme, decision options include to discontinue experiments because of futility (hypothesis apparently not true or effects not biologically significant), to gather more evidence to resolve ambiguity (with increased validity and reliability), or to engage in a clinical study (when enough evidence is collected). Most studies in preclinical research, however, are targeted at initial findings that are often exploratory (Howells et al., 2014). Systematic replication efforts that are decision-enabling are rare in academic preclinical research and have only recently begun to be conducted (Kimmelman et al., 2014). For the decision to finally engage in a clinical trial, a systematic review of all experiments is needed. In such a review, evidence should be judged on the validity and reliability criteria discussed here. Ideally, this will form the basis for informative investigator brochures that are currently lacking such decision enabling information (Wieschowski et al., 2018).
Our proposed framework is of course not applicable in all cases. If prior knowledge about a mechanism is already available and models are established, internal validity may already be high from the onset. Moreover, our simplified proposal may not generalise across all fields, or to academic and industry settings alike. Nonetheless, replications (and ideally every preclinical experiment) need to be framed in terms of validity and reliability. This is even more pressing as replications constitute an important foundation for successful translation. To enable the establishment of preclinical research trajectories, we see a need for action for funders and researchers.
The surprising lack of systematic replication in preclinical research also stems from lack of funding opportunities. Contrary to clinical trials, across-lab multi-centre replications are rare in preclinical research. Funders should thus design specific calls aimed at replications in the broad sense described here. Funding schemes should take the structure of preclinical research trajectories into account and may specifically be tailored towards the different experimental stages.
For replications, researchers need to specify how validity is improved by the replication compared to an initial study. Detailing how internal, external and translational validity increase in replications will emphasise the new evidence that is generated beyond the initial study and provide an ethical justification for the replication. Sample-size calculations should consider how reliability and validity are balanced against each other and define clear criteria for decisions to advance to the next stage in the trajectory. This will require a deviation from standard one-size-fits-all sample-size calculations. Researchers need guidance on how to adjust sample-size calculations and decision criteria, starting from an initial exploratory study that will serve as a proof of concept and gradually moving towards decision-enabling studies that will define whether a clinical trial is warranted.
The scientific endeavour is not limited to a single study and simple null-hypothesis testing. Even though the prevailing statistical test framework may suggest this, researchers are operating in a larger framework where evidence is accumulated over several levels with several competing alternative hypotheses (Platt, 1964). Replications are an important building block, where research priorities are transparently updated according to current knowledge. This includes proper reporting at all stages and registration of research at critical stages to avoid biases (Strech and Dirnagl, 2019). Currently, the literature on preclinical research and associated decisions is scarce (see, for example, van der Staay et al., 2010). Research on successful – and also on less successful – research lines will inform about best practices and yield important insights how biases potentially distort evidence collection (Kiwanuka et al., 2018).
In conclusion, systematically improving scientific validity in replications will improve trustworthiness and usefulness of preclinical studies and thus allow for a responsible conduct of animal experiments.
Data availability
No data was generated.
References
-
When power analyses based on pilot data are biased: inaccurate effect size estimators and follow-up biasJournal of Experimental Social Psychology 74:187–195.https://doi.org/10.1016/j.jesp.2017.09.004
-
Addressing the "Replication Crisis": Using original studies to design replication studies with appropriate statistical powerMultivariate Behavioral Research 52:305–324.https://doi.org/10.1080/00273171.2017.1289361
-
WebsiteConfirmatory preclinical studies (Förderung von konfirmatorischen präklinischen studien)German Federal Ministry of Education and Research. Accessed January 14, 2021.
-
P.201 reduction by prior animal informed research (RePAIR): a power solution to animal experimentationEuropean Neuropsychopharmacology 31:S19–S20.https://doi.org/10.1016/j.euroneuro.2019.12.027
-
An investigation of the false discovery rate and the misinterpretation of p-valuesRoyal Society Open Science 1:140216.https://doi.org/10.1098/rsos.140216
-
Animal models in translational medicine: validation and predictionNew Horizons in Translational Medicine 2:5–11.https://doi.org/10.1016/j.nhtm.2014.08.001
-
BookResolving the tension between exploration and confirmation in preclinical biomedical researchIn: Bespalov A, Michel M. C, Steckler T, editors. Good Research Practice in Non-Clinical Pharmacology and Biomedicine. Springer International Publishing. pp. 71–79.https://doi.org/10.1007/978-3-030-33656-1
-
BookMulticenter TrialsIn: Friedman L. M, Furberg C. D, DeMets D. L, Reboussin D. M, Granger C. B, editors. Fundamentals of Clinical Trials. Springer. pp. 501–518.https://doi.org/10.1007/978-1-4419-1586-3
-
External validity: we need to do moreAnnals of Behavioral Medicine 31:105–108.https://doi.org/10.1207/s15324796abm3102_1
-
A new standard for the analysis and design of replication studiesJournal of the Royal Statistical Society: Series A 183:431–448.https://doi.org/10.1111/rssa.12493
-
Bringing rigour to translational medicineNature Reviews Neurology 10:37–43.https://doi.org/10.1038/nrneurol.2013.232
-
Reproducibility and replicability of rodent phenotyping in preclinical studiesNeuroscience & Biobehavioral Reviews 87:218–232.https://doi.org/10.1016/j.neubiorev.2018.01.003
-
The case for introducing pre-registered confirmatory pharmacological pre-clinical studiesJournal of Cerebral Blood Flow & Metabolism 38:749–754.https://doi.org/10.1177/0271678X18760109
-
Performing high-powered studies efficiently with sequential analysesEuropean Journal of Social Psychology 44:701–710.https://doi.org/10.1002/ejsp.2023
-
Results of a preclinical randomized controlled multicenter trial (pRCT): Anti-CD49d treatment for acute brain ischemiaScience Translational Medicine 7:299ra121.https://doi.org/10.1126/scitranslmed.aaa9853
-
A cross-laboratory preclinical study on the effectiveness of interleukin-1 receptor antagonist in strokeJournal of Cerebral Blood Flow & Metabolism 36:596–605.https://doi.org/10.1177/0271678X15606714
-
Challenges in nanomedicine clinical translationDrug Delivery and Translational Research 10:721–725.https://doi.org/10.1007/s13346-020-00740-5
-
Common misconceptions about data analysis and statisticsJournal of Pharmacology and Experimental Therapeutics 351:200–205.https://doi.org/10.1124/jpet.114.219170
-
Preclinical models of Alzheimer's disease: Relevance and translational validityCurrent Protocols in Pharmacology 84:e57.https://doi.org/10.1002/cpph.57
-
What should researchers expect when they replicate studies? A statistical view of replicability in psychological sciencePerspectives on Psychological Science 11:539–544.https://doi.org/10.1177/1745691616646366
-
Safeguard power as a protection against imprecise power estimatesPerspectives on Psychological Science 9:319–332.https://doi.org/10.1177/1745691614528519
-
External validity and translation from research to implementationGenerations 34:59–65.
-
Dissecting the pharmacodynamics and pharmacokinetics of MSCs to overcome limitations in their clinical translationMolecular Therapy - Methods & Clinical Development 14:1–15.https://doi.org/10.1016/j.omtm.2019.05.004
-
APP mouse models for Alzheimer’s disease preclinical studiesThe EMBO Journal 36:2473–2487.https://doi.org/10.15252/embj.201797397
-
Systematic reviews and meta-analysis of preclinical studies: why perform them and how to appraise them criticallyJournal of Cerebral Blood Flow & Metabolism 34:737–742.https://doi.org/10.1038/jcbfm.2014.28
-
Considering aspects of the 3Rs principles within experimental animal biologyThe Journal of Experimental Biology 220:3007–3016.https://doi.org/10.1242/jeb.147058
-
3Rs missing: animal research without scientific value is unethicalBMJ Open Science 3:bmjos-2018-000048.https://doi.org/10.1136/bmjos-2018-000048
-
The standardization–generalization dilemma: A way outGenes, Brain, and Behavior 9:849–855.https://doi.org/10.1111/j.1601-183X.2010.00628.x
-
Reproducibility of animal research in light of biological variationNature Reviews Neuroscience 21:384–393.https://doi.org/10.1038/s41583-020-0313-3
-
Key elements for nourishing the translational research environmentScience Translational Medicine 7:282cm2.https://doi.org/10.1126/scitranslmed.aaa2049
Decision letter
-
Peter RodgersSenior and Reviewing Editor; eLife, United Kingdom
-
Catherine WinchesterReviewer; Beatson Institute, United Kingdom
-
Hanno WuerbelReviewer; University of Bern, Switzerland
In the interests of transparency, eLife publishes the most substantive revision requests and the accompanying author responses.
Thank you for submitting your article "Improving the predictiveness and ethics of preclinical studies through replications" to eLife for consideration as a Feature Article. Your article has been reviewed by three peer reviewers, and the evaluation has been overseen by the eLife Features Editor (Peter Rodgers). The following individuals involved in review of your submission have agreed to reveal their identity: Catherine Winchester (Reviewer #1); Hanno Wuerbel (Reviewer #2).
The reviewers and editors have discussed the reviews and we have drafted this decision letter to help you prepare a revised submission.
Summary
This paper presents an informal framework to guide preclinical research programmes, with the aim to systematically and gradually improve the reliability and validity of the research findings. According to the authors, such a procedure would not only increase chances for translational success but also lead to a more efficient allocation of resources and ethical use of animals. While the overall message is clear – most experiments are part of larger research programmes, and experimental designs need to be adjusted to the specific questions asked at any stage of a research programme – the authors need to be more precise in their definition/use of terms like replication, and to provide more details on how their proposals would work in practice.
Essential revisions
1) Definition of replication
The definition of "replication" used in the article seems almost redundant with the term "experiment". For example, the authors state: "Here, we define replications as experiments that aim to generate evidence that supports a previously made inferential claim (Nosek and Errington, 2020a)".
First, I am not sure this is equivalent to definition given in Nosek and Errington, 2020a: "Replication is a study for which any outcome would be considered diagnostic evidence about a claim from prior research". This should be clarified.
However, even if so, I am still not convinced it would be a useful definition of "replication". Nosek and Errington conclude: "Theories make predictions; replications test those predictions. Outcomes from replications are fodder for refining, altering, or extending theory to generate new predictions. Replication is a central part of the iterative maturing cycle of description, prediction, and explanation."
This is in fact a concise description of the "scientific method". Thus, Wikipedia (sorry about that….) describes the "scientific method" as: "It [the scientific method] involves formulating hypotheses, via induction, based on such observations; experimental and measurement-based testing of deductions drawn from the hypotheses; and refinement (or elimination) of the hypotheses based on the experimental findings."
I don't think it makes sense to replace well established terms such as "scientific method" or "experiment" by "replication", which for most scientists has a much more specific meaning. The authors should clarify this point and clearly state how they distinguish their term "replication" from the term "experiment" (every experiment is a test of a claim from prior research and therefore fits Nosek and Errington's definition of replication) and how they distinguish their framework from the "scientific method" in general.
This becomes even more explicit in the following paragraph, where they state: "Besides within and between laboratory replications, experiments in such series include initial exploratory studies, toxicity studies, positive and negative controls, pharmacodynamics and kinetics that all aim to generate evidence to support an inferential claim and refute possible alternatives". Thus, according to the authors, all of these experiments (toxicity studies, pharmacodynamics, etc.) are nothing but replications – this is stretching the term replication extremely far, and I am not convinced that readers will follow them. And it would not be necessary; they could present the exact same framework by using conventional terms.
Related to this, please explain the difference/relationship between replication and reproducibility.
2) The section "What to replicate?"
The description of the three projects in the section is too long, and needs to be better integrated into the article.
Note from the Editor: since this point is largely an editorial matter, dealing with it can be deferred until later.
3) The section "How to replicate?"
The stepwise procedure presented in the text and in Figure 1 is not well developed. The trade-offs between the different dimensions of validity are not presented in any detail, and the ethical and scientific implications of different decisions at different stages in a research programme are not discussed. In particular:
– "At the start, reliability and validity are potentially low due to biased assessment of outcomes, unblinded conduct of experiments, undisclosed researcher degrees of freedom, low sample sizes, etc.". This reads like a recommendation: start your research line with quick and dirty studies and only refine methods once you have discovered something interesting. I am sure that this is not what the authors wanted to say but it is how it may come across. The fact that these problems are prevalent throughout past (and current) preclinical research does not justify them. Even at early, exploratory stages of a research programme, scientific rigour is essential for both scientific and ethical reasons. Therefore, I do not agree with the authors that internal validity may be low initially and should only be improved in the course of a research programme. Studies may be smaller (using smaller sample sizes) initially, but there is no excuse for ignoring any other measure against risks of bias (randomization, blinding, complete reporting of all measured outcome variables, etc.) as these are simply part of good research practice!
– This brings me to another point: I am not convinced that exploratory research should be conducted under highly restricted conditions. In fact, including variation of conditions (e.g. genotype, environment, measurement) right from the beginning may be much more productive a strategy for generating valuable hypotheses (see e.g. Voelkl et al., 2020). Furthermore, designing larger and more complex confirmatory or replication studies (covering a larger inference space) may reduce the need for additional studies to assess convergent and discriminant validity and external validity. Thus, there is a trade-off between more but simpler vs. fewer but more complex studies that is not at all represented in the framework presented here (and in Figure 1).
– "At this stage, criteria to decide whether to conduct additional experiments and replications should be lenient". Here it seems that the authors do make a difference between "experiments" and "replications", but as discussed above, it is unclear on what grounds.
– The definition of "translational validity" needs attention. They state that it covers "construct validity" and "predictive validity", but not "concurrent validity" and "content validity", which are the four common dimensions of test validity. Thus, it is unclear whether they actually mean "test validity" (but consider concurrent validity and content validity less important) or whether they consider "translational validity" to be different from "test validity". Importantly, however, "construct validity" is made up by "convergent validity" and "discriminant validity". This means that assessing "construct validity" requires tests of convergent and discriminant validity that cannot – according to common terminology – be considered as replications as they explicitly test and compare measures of different constructs that should or should not be related.
– "In studies with low internal validity that stand at the beginning of a new research line, e.g. in an exploratory phase, low numbers of animals may be acceptable." Again, I don't think low internal validity is ever acceptable, certainly not if animals are involved. The number of animals always needs to be justified by the specific question to be answered – that in exploratory research often low numbers of animals are used does not necessarily mean that this is acceptable.
– The recommendations in this section are rather vague. For example, the authors write: "For replications, researchers need to specify how validity is improved by the replication compared to the initial study." Yet, after this general demand, no further details or suggestions are given how this can be achieved. Similarly, they write "Researchers need guidance on how to adjust sample size calculations..." but no guidance is offered here nor are any hints given where to find such guidance (references might be useful) on what principles this guidance should be based etc.
– Please comment on how much evidence (how many replication studies/ different approaches exploring the same question) is needed? And who should collate this body of evidence – researchers, clinicians, funders, a committee?
https://doi.org/10.7554/eLife.62101.sa1Author response
Essential revisions
1) Definition of replication
The definition of "replication" used in the article seems almost redundant with the term "experiment". For example, the authors state: "Here, we define replications as experiments that aim to generate evidence that supports a previously made inferential claim (Nosek and Errington, 2020a)".
First, I am not sure this is equivalent to definition given in Nosek and Errington, 2020a: "Replication is a study for which any outcome would be considered diagnostic evidence about a claim from prior research". This should be clarified.
However, even if so, I am still not convinced it would be a useful definition of "replication". Nosek and Errington conclude: "Theories make predictions; replications test those predictions. Outcomes from replications are fodder for refining, altering, or extending theory to generate new predictions. Replication is a central part of the iterative maturing cycle of description, prediction, and explanation."
This is in fact a concise description of the "scientific method". Thus, Wikipedia (sorry about that….) describes the "scientific method" as: "It [the scientific method] involves formulating hypotheses, via induction, based on such observations; experimental and measurement-based testing of deductions drawn from the hypotheses; and refinement (or elimination) of the hypotheses based on the experimental findings."
I don't think it makes sense to replace well established terms such as "scientific method" or "experiment" by "replication", which for most scientists has a much more specific meaning. The authors should clarify this point and clearly state how they distinguish their term "replication" from the term "experiment" (every experiment is a test of a claim from prior research and therefore fits Nosek and Errington's definition of replication) and how they distinguish their framework from the "scientific method" in general.
This becomes even more explicit in the following paragraph, where they state: "Besides within and between laboratory replications, experiments in such series include initial exploratory studies, toxicity studies, positive and negative controls, pharmacodynamics and kinetics that all aim to generate evidence to support an inferential claim and refute possible alternatives". Thus, according to the authors, all of these experiments (toxicity studies, pharmacodynamics, etc.) are nothing but replications – this is stretching the term replication extremely far, and I am not convinced that readers will follow them. And it would not be necessary; they could present the exact same framework by using conventional terms.
Related to this, please explain the difference/relationship between replication and reproducibility.
We agree that our definition was not exactly matching the Nosek and Errington definition. We now clarify that replications are not solely about supporting evidence but works in both ways also to refute a claim. Starting from the admittedly very broad Nosek and Errington definition, we now specify that a replication is indeed based on a specific previous experiment. We thus rewrote the passage mentioned above to clarify this important issue. We now distinguish between experiments that test the same claim but with different design and approach and replications that are closely modelled after an initial experiment, but are changed to improve validity and reliability.
“Here, we define replications as experiments that are based on previous studies that aim to distinguish false from true claims (Nosek and Errington, 2020a). For this, previous experiments need to be reproducible with all methods and analytical pipelines unambiguously described. Reproducibility is thus a necessary prerequisite to engage in a contrastable replication (Patil et al., 2016; Plesser, 2018). Replications can deviate from previous experimental protocols by e.g. introducing different animal strains or changing environmental factors. Introducing such systematic heterogeneity between studies potentially strengthens generated evidence about inferential claims (Voelkl et al., 2020). “
With regard to the scientific method, we now emphasise that even tough preclinical research is of course based on a scientific method we now emphasise distinct features (measuring human conditions entirely in model systems) that sets it apart from others disciplines.
“With its goal to closely model human disease conditions, preclinical research differs from other scientific disciplines as there is a biological gap between experimentally studied models and patients as the ultimate beneficent. This affects the role of replications as they should not only confirm previous results but ideally also increase predictive power for the human case to enable successful translation.”
2) The section "What to replicate?"
The description of the three projects in the section is too long, and needs to be better integrated into the article.
Note from the Editor: since this point is largely an editorial matter, dealing with it can be deferred until later.
We make a suggestion how to shorten this paragraph. We reduced the number of words from 1027 to 807. We look forward to discussing this paragraph further if necessary.
3) The section "How to replicate?"
The stepwise procedure presented in the text and in Figure 1 is not well developed. The trade-offs between the different dimensions of validity are not presented in any detail, and the ethical and scientific implications of different decisions at different stages in a research programme are not discussed. In particular:
– "At the start, reliability and validity are potentially low due to biased assessment of outcomes, unblinded conduct of experiments, undisclosed researcher degrees of freedom, low sample sizes, etc.". This reads like a recommendation: start your research line with quick and dirty studies and only refine methods once you have discovered something interesting. I am sure that this is not what the authors wanted to say but it is how it may come across. The fact that these problems are prevalent throughout past (and current) preclinical research does not justify them. Even at early, exploratory stages of a research programme, scientific rigour is essential for both scientific and ethical reasons. Therefore, I do not agree with the authors that internal validity may be low initially and should only be improved in the course of a research programme. Studies may be smaller (using smaller sample sizes) initially, but there is no excuse for ignoring any other measure against risks of bias (randomization, blinding, complete reporting of all measured outcome variables, etc.) as these are simply part of good research practice!
We fully agree with the reviewers here and admit that we introduced some ambiguity here that we now clarify. In particular, we stress that internal validity should be already high in early stages. We also adjusted Figure 1 to indicate that internal validity should be already considered at early stages.
“At the start, researchers usually start off with an explorative study. As not all details and confounders in such a study can be known upfront reliability and validity are potentially not fully optimized. However, even at these early stages researchers should implement e.g. strategies to mitigate risks of bias (Figure 1).”
– This brings me to another point: I am not convinced that exploratory research should be conducted under highly restricted conditions. In fact, including variation of conditions (e.g. genotype, environment, measurement) right from the beginning may be much more productive a strategy for generating valuable hypotheses (see e.g. Voelkl et al., 2020). Furthermore, designing larger and more complex confirmatory or replication studies (covering a larger inference space) may reduce the need for additional studies to assess convergent and discriminant validity and external validity. Thus, there is a trade-off between more but simpler vs. fewer but more complex studies that is not at all represented in the framework presented here (and in Figure 1).
We agree with the reviewers that the standardisation fallacy is an important topic, particularly to be considered in preclinical research. As our focus is on the role of replications, we now extend our description on within and between laboratory replications to consider heterogenization. We emphasise this at various locations now. We further point readers to the mentioned paper by Voelkl et al., 2020 as a reference for a more general discussion. At this stage, we feel that introducing also a discussion on how to start a preclinical research process and negotiate simpler vs more complex studies would stray too far from our core message. To accommodate this important thought nonetheless we now state:
“Systematic heterogeneity (additional strains, similar animal models, different sex) will further strengthen external validity. If feasible heterogenization should be introduced at early stages already.”
– "At this stage, criteria to decide whether to conduct additional experiments and replications should be lenient". Here it seems that the authors do make a difference between "experiments" and "replications", but as discussed above, it is unclear on what grounds.
We adjusted the Introduction (see above) to make the distinction between a replication and additional experiments in preclinical research clear.
– The definition of "translational validity" needs attention. They state that it covers "construct validity" and "predictive validity", but not "concurrent validity" and "content validity", which are the four common dimensions of test validity. Thus, it is unclear whether they actually mean "test validity" (but consider concurrent validity and content validity less important) or whether they consider "translational validity" to be different from "test validity". Importantly, however, "construct validity" is made up by "convergent validity" and "discriminant validity". This means that assessing "construct validity" requires tests of convergent and discriminant validity that cannot – according to common terminology – be considered as replications as they explicitly test and compare measures of different constructs that should or should not be related.
We rewrote the paragraph on translational validity to address these points. Importantly, we removed all references to other types of validity than translational validity. The types of validity cited are derived mainly from psychological test theory. They have specific meanings in this context and it is (after lengthy discussion amongst the authors) not clear whether they relate to preclinical research in the same way they relate to psychological test theory. Even in psychology, construct validity is still a hotly debated topic where Meehl/Cronbach accounts clash with Messick for example. As this paper is addressing preclinical researchers who will most likely not be familiar with these terms and their theoretical framing, we removed the terms. We retained the original implications though. That is, translational validity is about the appropriateness of the disease model on a mechanistic level and how well it predicts the human condition. We also introduced as suggested by the reviewers converging and discriminant evidence without however resorting to calling this a type of validity. We clarify also that experiments generating converging and discriminant evidence are separate from replications.
“The term translational validity is used here as an umbrella term for factors that putatively contribute to translational success. It pertains to how well measurements and animal models represent a certain disease and its underlying pathomechanisms in humans. To assess this, complementary experiments evaluate the bounds of a model to discriminate it from other very similar diseases and collect converging evidence from different approaches. Often only parts of a disease are present in animal models. In models of neurodegenerative diseases like Alzheimer’s disease (AD) (Sasaguri et al., 2017), the focus on familial early onset genes in mouse models has potentially led to translational failures as the majority of human AD diagnoses are classified as sporadic late onset form (Mullane and Williams, 2019). Translational validity thus reflects whether measured parameters in animal models are diagnostic for human conditions and consequently, to what extent the observed outcomes will predict outcomes in humans (Denayer et al., 2014; Mullane and Williams, 2019).”
– "In studies with low internal validity that stand at the beginning of a new research line, e.g. in an exploratory phase, low numbers of animals may be acceptable." Again, I don't think low internal validity is ever acceptable, certainly not if animals are involved. The number of animals always needs to be justified by the specific question to be answered – that in exploratory research often low numbers of animals are used does not necessarily mean that this is acceptable.
– The recommendations in this section are rather vague. For example, the authors write: "For replications, researchers need to specify how validity is improved by the replication compared to the initial study." Yet, after this general demand, no further details or suggestions are given how this can be achieved. Similarly, they write "Researchers need guidance on how to adjust sample size calculations..." but no guidance is offered here nor are any hints given where to find such guidance (references might be useful) on what principles this guidance should be based etc.
Here, we want to point out that the number of animals is in our view not so much related to validity as to reliability. We now address this point in more detail (the reviewers note themselves above: “Studies may be smaller (using smaller sample sizes) initially.”).
“However, even in high-validity experiments, reliability can be low when the number of experimental units is not sufficient to detect existing effects. For replications at this stage, reliability should be increased by increasing sample sizes or refining measurement procedures. As true preclinical effect sizes are frequently small and associated with considerable variance between experimental units, increased numbers of experimental units are needed to obtain reliable results (Bonapersona et al., 2020; Carneiro et al., 2018). “
We further provide concrete examples how to increase internal validity, external validity and translational validity.
Guidance on sample size calculations in replications is scarce and a theme that we are currently researching ourselves. We nonetheless now point to recent approaches that were developed mainly for psychology and thus may not transfer easily.
“Under consideration of a reduction of animals according to the 3R principles (Russell and Burch, 1959), the number of animals tested needs to reflect the current stage in the preclinical trajectory (Sneddon et al., 2017; Strech and Dirnagl, 2019). For replications, this estimation involves consideration of effect sizes from previous studies. A power calculation based on the point estimate of the initial study will often yield too small animal numbers (Albers and Lakens, 2018; Piper et al., 2019). This potentially inflates false negatives, running the risk of missing important effects and wrongfully failing a replication. Alternatives like safeguard power analysis (Perugini et al., 2014), sceptical p-value (Held, 2020), or adjusting for uncertainty (Anderson and Maxwell, 2017) have been proposed mainly for psychological experiments with human subjects. Sample sizes in animal experiments are, however, much lower than estimated by the above methods due to ethical concerns and resource constraints. This may be one reason why such approaches have not yet been implemented widely in preclinical research.“
– Please comment on how much evidence (how many replication studies/ different approaches exploring the same question) is needed? And who should collate this body of evidence – researchers, clinicians, funders, a committee?
This is indeed an interesting and pressing question. Researchers from the emerging field of meta-analysis in preclinical research will be well suited to collate such evidence for example in systematic reviews. Such analyses could in turn form the basis for investigator brochures that are required for early clinical trials. We outline this as one scenario and admit that there may be different routes how to arrive at the conclusion that there is enough data to start a clinical trial.
“Systematic replication efforts that are decision-enabling are rare in academic preclinical research and have only recently begun to be conducted (Kimmelman et al., 2014). For the decision to finally engage in a clinical trial a systematic review of all experiments is needed. In such a review, evidence should be judged on the validity and reliability criteria discussed here. Ideally, this will form the basis for informative investigator brochures that are currently lacking such decision enabling information (Wieschowski et al., 2018).”
https://doi.org/10.7554/eLife.62101.sa2Article and author information
Author details
Funding
Bundesministerium für Bildung und Forschung (01KC1901A)
- Natascha Ingrid Drude
- Lorena Martinez Gamboa
The funders had no role in study design, data collection and interpretation, or the decision to submit the work for publication.
Publication history
- Received:
- Accepted:
- Accepted Manuscript published:
- Version of Record published:
Copyright
© 2021, Drude et al.
This article is distributed under the terms of the Creative Commons Attribution License, which permits unrestricted use and redistribution provided that the original author and source are credited.
Metrics
-
- 3,260
- views
-
- 318
- downloads
-
- 38
- citations
Views, downloads and citations are aggregated across all versions of this paper published by eLife.
Download links
Downloads (link to download the article as PDF)
Open citations (links to open the citations from this article in various online reference manager services)
Cite this article (links to download the citations from this article in formats compatible with various reference manager tools)
Further reading
-
- Cancer Biology
- Medicine
A doctoral-level internship program was developed at the University of North Carolina at Chapel Hill with the intent to create customizable experiential learning opportunities for biomedical trainees to support career exploration, preparation, and transition into their postgraduate professional roles. We report the outcomes of this program over a 5-year period. During that 5-year period, 123 internships took place at over 70 partner sites, representing at least 20 academic, for-profit, and non-profit career paths in the life sciences. A major goal of the program was to enhance trainees’ skill development and expertise in careers of interest. The benefits of the internship program for interns, host/employer, and supervisor/principal investigator were assessed using a mixed-methods approach, including surveys with closed- and open-ended responses as well as focus group interviews. Balancing stakeholder interests is key to creating a sustainable program with widespread support; hence, the level of support from internship hosts and faculty members were the key metrics analyzed throughout. We hypothesized that once a successful internship program was implemented, faculty culture might shift to be more accepting of internships; indeed, the data quantifying faculty attitudes support this. Furthermore, host motivation and performance expectations of interns were compared with results achieved, and this data revealed both expected and surprising benefits to hosts. Data suggests a myriad of benefits for each stakeholder group, and themes are cataloged and discussed. Program outcomes, evaluation data, policies, resources, and best practices developed through the implementation of this program are shared to provide resources that facilitate the creation of similar internship programs at other institutions. Program development was initially spurred by National Institutes of Health pilot funding, thereafter, successfully transitioning from a grant-supported model, to an institutionally supported funding model to achieve long-term programmatic sustainability.
-
- Computational and Systems Biology
- Medicine
Excessive consumption of sucrose, in the form of sugar-sweetened beverages, has been implicated in the pathogenesis of metabolic dysfunction‐associated fatty liver disease (MAFLD) and other related metabolic syndromes. The c-Jun N-terminal kinase (JNK) pathway plays a crucial role in response to dietary stressors, and it was demonstrated that the inhibition of the JNK pathway could potentially be used in the treatment of MAFLD. However, the intricate mechanisms underlying these interventions remain incompletely understood given their multifaceted effects across multiple tissues. In this study, we challenged rats with sucrose-sweetened water and investigated the potential effects of JNK inhibition by employing network analysis based on the transcriptome profiling obtained from hepatic and extrahepatic tissues, including visceral white adipose tissue, skeletal muscle, and brain. Our data demonstrate that JNK inhibition by JNK-IN-5A effectively reduces the circulating triglyceride accumulation and inflammation in rats subjected to sucrose consumption. Coexpression analysis and genome-scale metabolic modeling reveal that sucrose overconsumption primarily induces transcriptional dysfunction related to fatty acid and oxidative metabolism in the liver and adipose tissues, which are largely rectified after JNK inhibition at a clinically relevant dose. Skeletal muscle exhibited minimal transcriptional changes to sucrose overconsumption but underwent substantial metabolic adaptation following the JNK inhibition. Overall, our data provides novel insights into the molecular basis by which JNK inhibition exerts its metabolic effect in the metabolically active tissues. Furthermore, our findings underpin the critical role of extrahepatic metabolism in the development of diet-induced steatosis, offering valuable guidance for future studies focused on JNK-targeting for effective treatment of MAFLD.