Punishment insensitivity in humans is due to failures in instrumental contingency learning
Figures
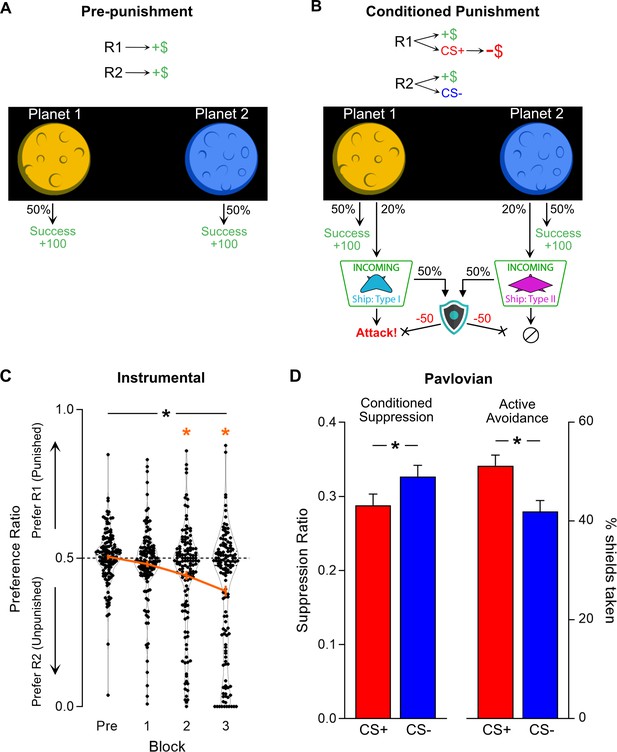
Design and aggregate behaviour in ‘Planets and Pirates’ task.
(A) During pre-punishment phase, participants could continuously click on two planets (R1 and R2 [side counterbalanced]) to earn reward (+100 points, 50% chance per response). (B) During conditioned punishment phase, additional R1→CS+ and R2→CS- contingencies were introduced (20% chance per response). CS+ precipitated attack (−20% point loss), whereas CS- had no aversive consequence. A shield button was made available on a random 50% of CS presentations; activating the shield cost 50 points but prevented any point loss from attacks. (C) Preference ratio (orange line = mean ± SEM; dots = individual preference scores) of R1:R2 clicking during pre-punishment phase (Pre) and punishment blocks (1–3). Overall, participants (n = 135) learned to avoid punishment, biasing responding away from punished R1 in favour of unpunished R2. (D) Mean ± SEM CS-elicited behaviour across punishment phase. Participants showed more response suppression (0 = complete suppression) during unshielded portions of CS+ compared CS- (left panel), and greater shield use to CS+ than CS- (right panel). * [black] p<0.05 behaviour effect; * [orange] p<0.05 vs. null ratio.
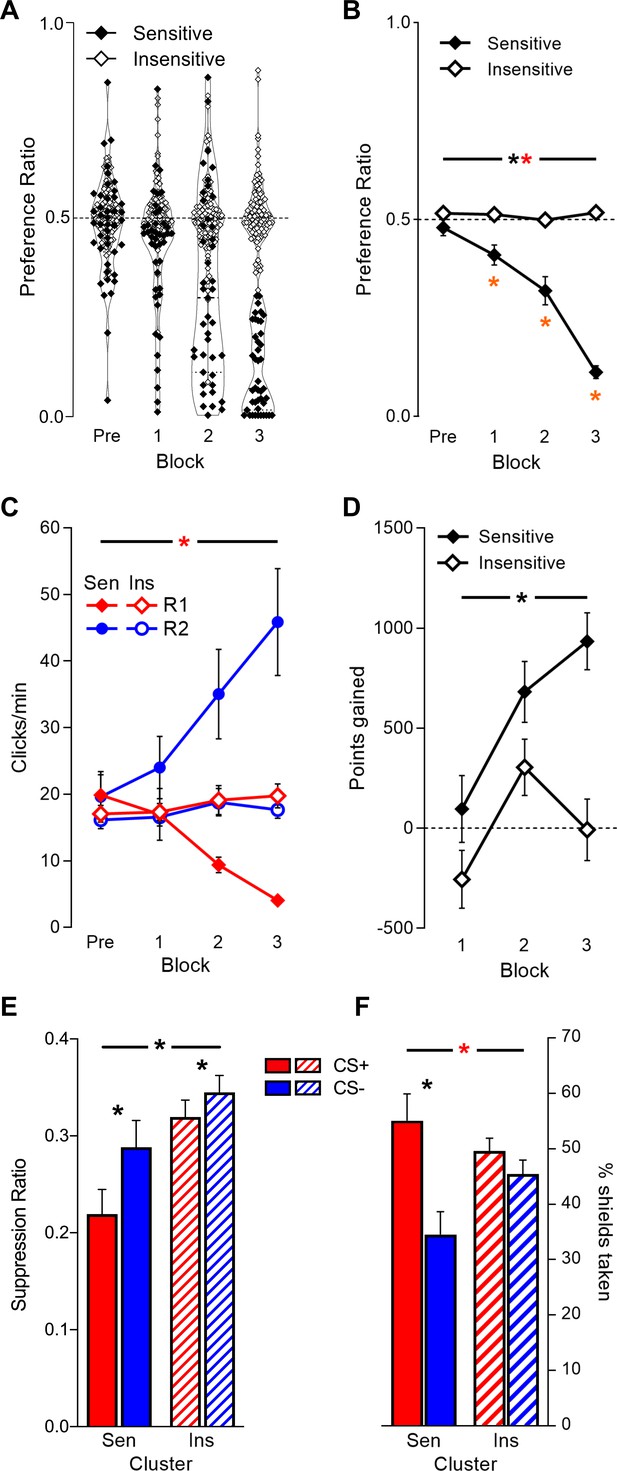
Behaviour in task by punishment sensitivity cluster.
(A) Final preference ratios (punishment avoidance) were bimodally distributed. Cluster analysis partitioned individuals into punishment-sensitive (n = 43; filled dots) and -insensitive (n = 92; unfilled dots) clusters. (B) Mean ± SEM preference ratio by cluster across pre-punishment (Pre) and punishment blocks (1–3); the sensitive cluster acquired punishment avoidance, while the insensitive cluster did not. (C) Mean ± SEM planet click rates by cluster across pre-punishment and punishment blocks. Clusters exhibited similar overall click rates across task phases, but divergent response allocation. (D) Mean ± SEM point gain per punishment block; only the sensitive cluster achieved a net gain in points across punishment blocks. (E) Mean ± SEM conditioned suppression to CS+ and CS- by cluster. Both clusters showed greater response suppression to CS+ than CS-; sensitive cluster showed greater response suppression overall. (F) Mean ± SEM active avoidance (shield use) by cluster. Only sensitive cluster showed significantly greater shield use during CS+ vs. CS-. Sen = sensitive cluster; Ins = insensitive cluster * [black] p<0.05 cluster main effect; * [orange] p<0.05 vs. null ratio; * [red] p<0.05 cluster*behaviour interaction.
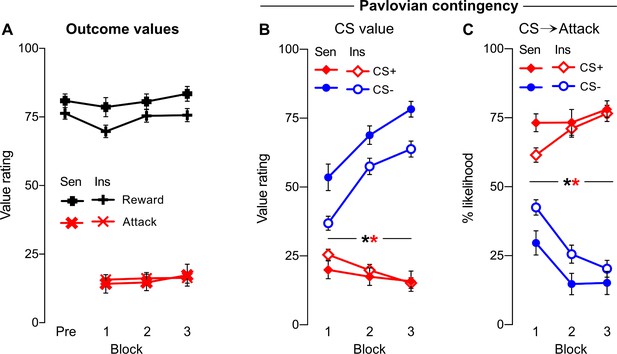
Self-reported outcome and conditioned stimulus (CS) valuations, and Pavlovian contingency knowledge.
(A) Valuation of point outcomes (reward, attack) by cluster across pre-punishment (Pre) and punishment blocks (1–3). Rewards were more highly rated by the sensitive cluster. Both clusters equally disliked attacks. (B) Valuation of CS+ and CS- by cluster across punishment blocks. CS+ was valued less than CS-; clusters only differed in their valuation of CS-. (C) Pavlovian CS→Attack inferences by cluster across punishment blocks. Attacks were attributed to CS+ over CS-; clusters only differed in attack attributions following first block of punishment. Sen = sensitive cluster; Ins = insensitive cluster * [black] p<0.05 CS main effect; * [red] p<0.05 cluster*CS interaction.
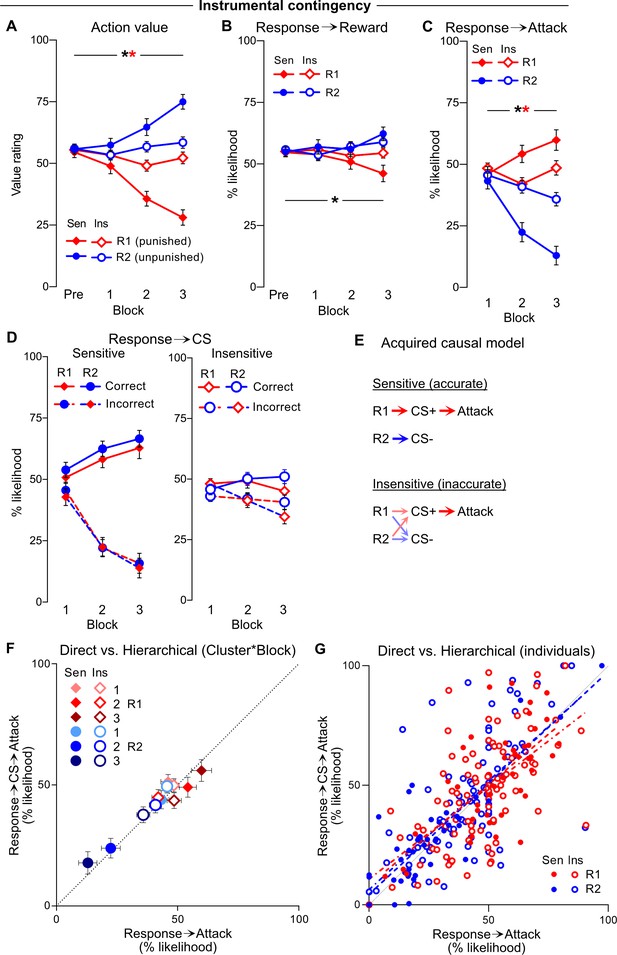
Instrumental valuations and contingency knowledge.
(A) Mean ± SEM valuation of planets (R1, R2) by cluster across pre-punishment (Pre) and punishment blocks (1–3). Unpunished R2 was gradually valued more than punished R1, particularly by sensitive cluster. (B) Mean ± SEM instrumental Response→Reward inferences by cluster. Rewards were spuriously attributed to R2 more than R1; this did not interact with cluster. (C) Mean ± SEM instrumental Response→Attack inferences. Attacks were attributed to R1 over R2, particularly by sensitive cluster. (D) Mean ± SEM instrumental Response→CS inferences (Left panel: sensitive cluster; Right panel: insensitive cluster) according to correct (R1→CS+, R2→CS-) vs. incorrect (R1→CS-, R2→CS+) inferences. Clusters attributed CSs to their respective responses, particularly by sensitive cluster. (E) Putative causal model acquired by clusters across punishment phase. Sensitive individuals acquired accurate Response→CS and CS→Attack contingency knowledge. Insensitive individuals acquired accurate CS→Attack knowledge, but failed to acquire accurate Response→CS knowledge. (F) Mean ± SEM direct, self-reported Response→Attack inferences vs. estimate computed from hierarchical Response→CS→Attack inferences per response (R1, R2), cluster (Sen, Ins) and punishment block (1–3). Black dotted line represents perfect correspondence between direct and hierarchical inferences. (G) Direct, self-reported Response→Attack inferences vs. estimate computed from hierarchical Response→CS→Attack inferences per subject (averaged across punishment). Black dotted line represents perfect correspondence between direct and hierarchical inferences. Dashed line represents lines of best fit for sensitive cluster (per response); dotted-dashed line represents line of best fit line for insensitive cluster (per response). Sen = sensitive cluster; Ins = insensitive cluster * [black] p<0.05 response main effect; * [red] p<0.05 cluster*response interaction.
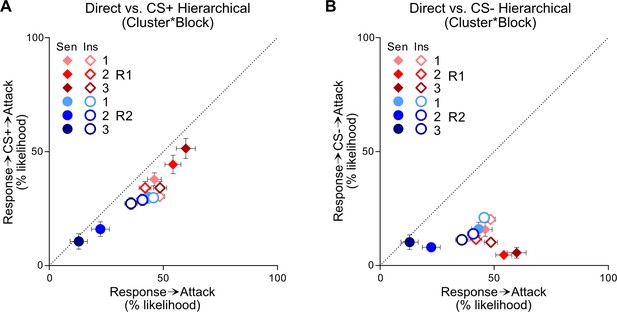
Relationship between self-reported Response→Attack inferences and estimate computed from hierarchical Response→CS→Attack inferences.
(A) Mean ± SEM direct Response→Attack inferences vs. estimate computed from hierarchical Response→CS+→Attack inferences per response (R1, R2), cluster (Sen, Ins) and punishment block (1–3). Black dotted line represents perfect correspondence between direct and hierarchical inferences; slight underprediction is observed without accounting for CS- contingencies. (B) Mean ± SEM direct Response→Attack inferences vs. estimate computed from hierarchical Response→CS-→Attack inferences per response (R1, R2), cluster (Sen, Ins) and punishment block (1–3). Black dotted line represents perfect correspondence between direct and hierarchical inferences; substantial underprediction is observed without accounting for CS+ contingencies.
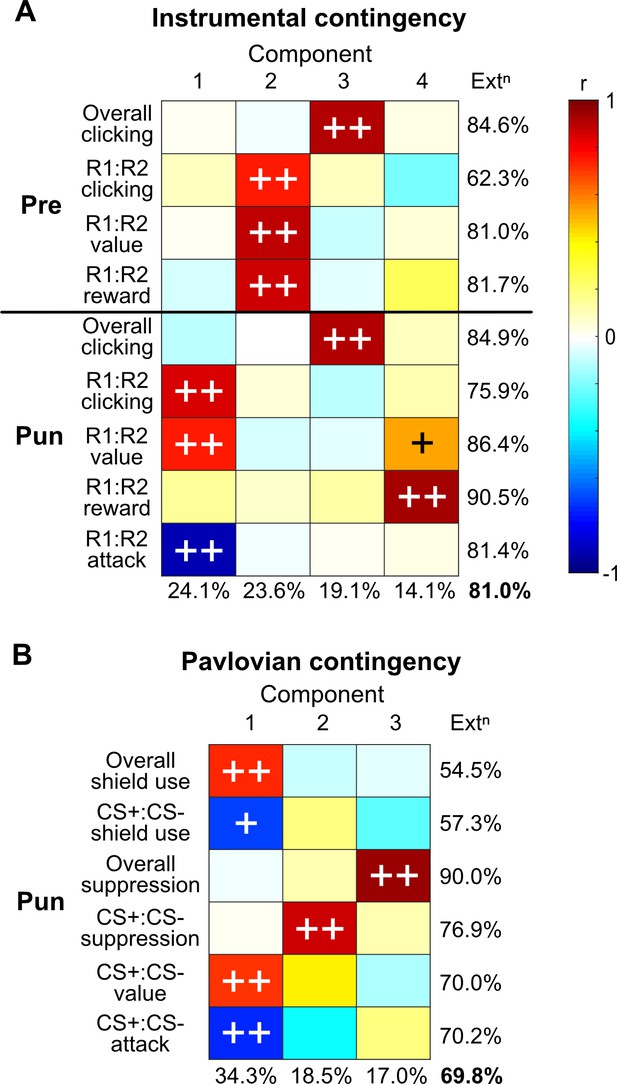
Alignments in behaviour, valuations, and contingency knowledge.
(A) Principal component analysis of instrumental behaviour, valuations, and contingency knowledge across pre-punishment (Pre) and punishment (Pun) phases. (B) Principal component analysis of conditioned stimulus (CS)-related (Pavlovian) behaviour, valuations, and contingency knowledge across punishment (Pun) phase. Extn = overall extraction; ++ = >0.707 loading (>50% variance accounted for by component); + = >0.5 loading (>25% variance accounted for by component).