Data mining methodology for response to hypertension symptomology—application to COVID-19-related pharmacovigilance
Figures
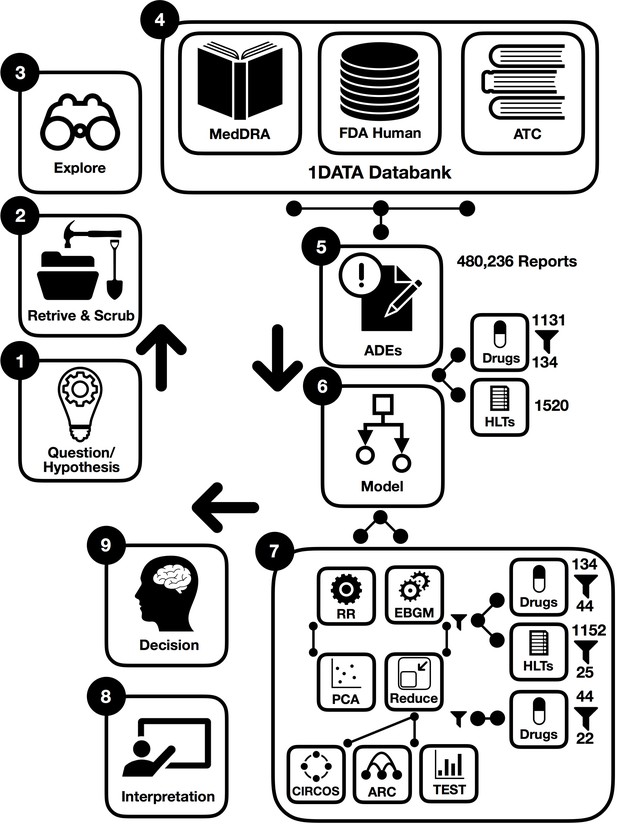
Workflow of data-driven methodology for pulmonary symptomology in hypertension using machine learning models from preprocessing and dictionary creation to storing tables in the database an analysis.
As a part of data cleaning, we were also challenged by multiple technical issues when combining drugs: (i) there were many drugs’ names that did not track a specific standard. (ii) Formulations of the same active ingredient with different generic or brand names for different routes of administration created confusion in collecting data (for instance, Revatio, Viagra, sildenafil, sildenafil citrate, APO sildenafil, sildenafil film-coated tablet, sildenafil citrate Aurobindo pharma, sildenafil Amneal Pharmaceuticals, Teva sildenafil, sildenafil Pfizer, sildenafil Greenstone, sildenafil Hormosan Filmtabletten, Revathio, sildenafil SUP, etc.). For this purpose, we combined drugs with or without salt, alcohol, etc. from different generic names and brand names.
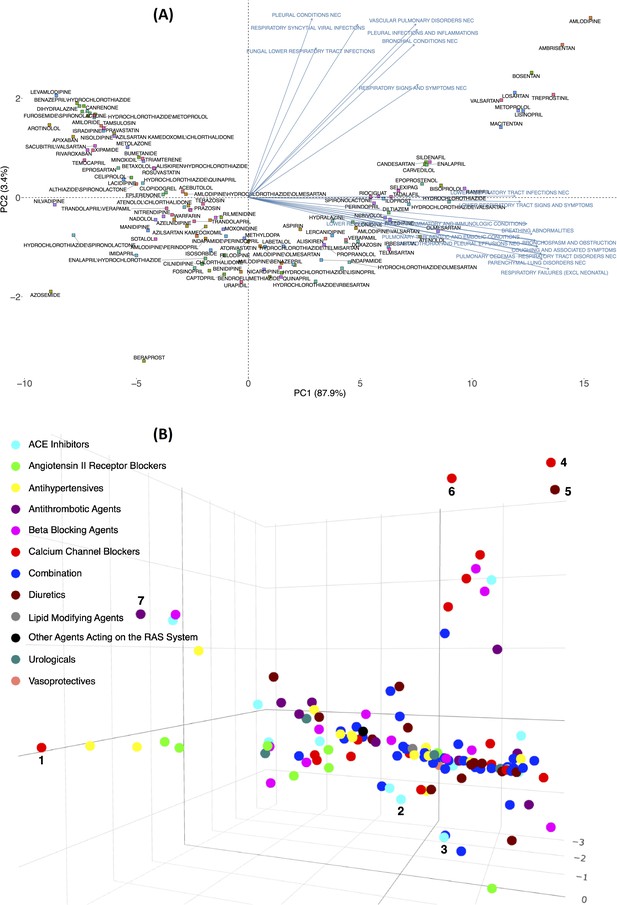
Principal component analysis of the expected count for 134 drugs (from 12 Anatomical Therapeutic Chemical [ATC] drug classes) in 2D (A) and 3D (B) spaces using the log expected value of RR, .
In Panel B, individual drugs are (significantly) separated on the extreme edges are marked by (1), amlodipine, (2) quinapril, (3) trandolapril, (4) nilvadipine, (5) azosemide, (6) azelnidipine, and (7) treprostinil. An interactive figure can be found on the 1DATA home page. Click the following URL to see the figure: https://1data.life/pages/publication/figure1B.html.
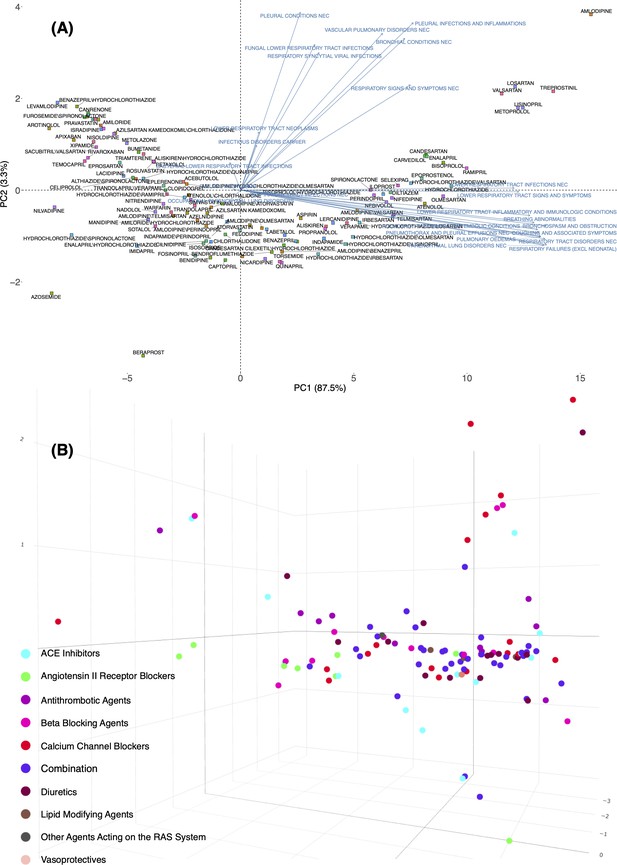
Principal component analysis of expected count for all the 117 drugs when excluding antihypertensive drugs in 2D (A) and 3D (B) space using empirical Bayes geometric mean (EBGM) for angiotensin-converting enzymes (ACEIs), angiotensin-II receptor blockers (ARBs), beta-blocking agents (BBAs), calcium channel blockers (CCBs), and TDs.
In Panel B, individual drugs are (significantly) separated on the extreme edges are marked by (1) amlodipine, (2) quinapril, (3) trandolapril, (4) nilvadipine, (5) azosemide, (6) azelnidipine, and (7) treprostinil.
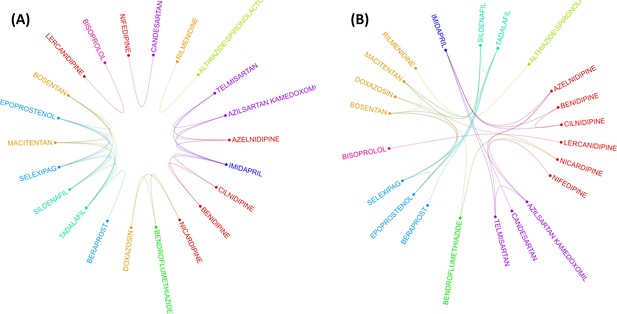
Two layouts of Circos plot for 22 hypertensive drugs selected by graphical least absolute shrinkage and selection operator (GLASSO).
Circos plots of drugs were obtained based on the empirical Bayes geometric mean (EBGM) matrix after applying GLASSO. Edge bundling linkages for better visualization and drugs were selected by GLASSO with edge bundling. Grouped drugs based on their classes were assigned the same color based on their classes (A). Applying reverse Cuthill-McKee (RCM) reordering and edge bundling for grouping drugs based on the Anatomical Therapeutic Chemical (ATC) class and edge bundling (B).
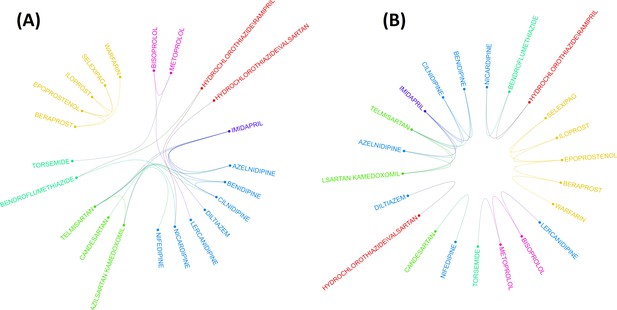
Two layouts of Circos plot for 22 hypertensive drugs selected by graphical least absolute shrinkage and selection operator (GLASSO) when excluding antihypertensives agents (AHAs).
Circos plots of drugs were obtained based on the empirical Bayes geometric mean (EBGM) matrix after applying GLASSO. Edge bundling linkages for better visualization and drugs were selected by GLASSO with edge bundling. Grouped drugs in high-level terms were assigned the same color based on their classes (A). Applying reverse Cuthill-McKee (RCM) reordering and edge bundling for grouping drugs based on the Anatomical Therapeutic Chemical (ATC) class and edge bundling (B).
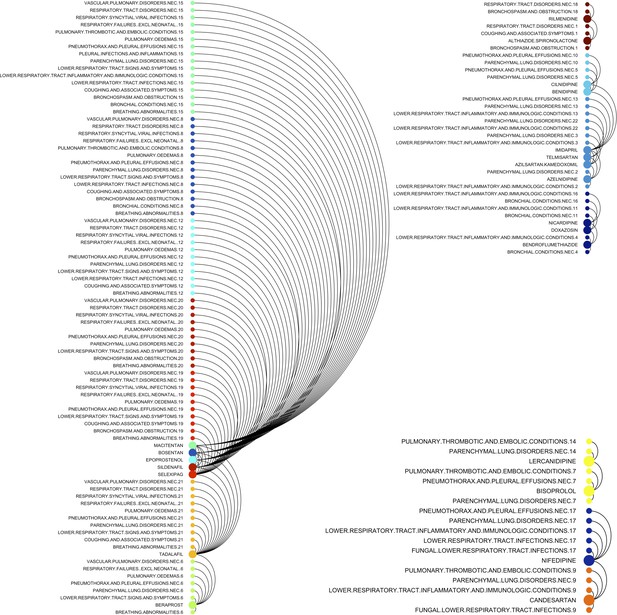
Arc diagram visualization of 22 drugs selected from graphical least absolute shrinkage and selection operator (GLASSO) and associated pulmonary adverse drug event (ADE)-drug combination.
Three inset maps as examples of highly correlated and complex interactions are shown. Large-filled circles show drugs and small circles are used for ADEs.
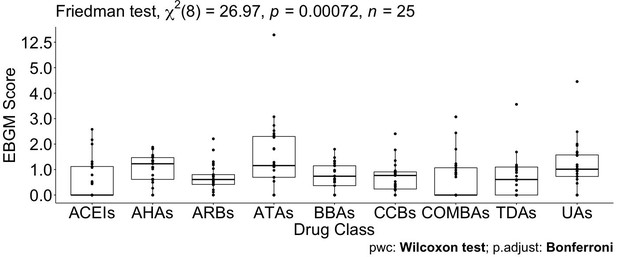
Pairwise Wilcoxon signed-rank test between different Anatomical Therapeutic Chemical (ATC) classes.
No pairwise significant comparison was found similar to Supplementary file 7. But the group comparison was highly significant, p-value = 0.00072.
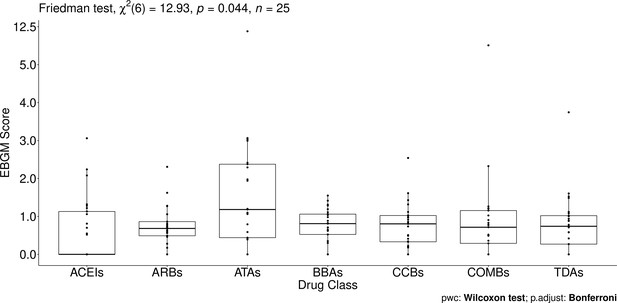
Pairwise Wilcoxon signed-rank test between different Anatomical Therapeutic Chemical (ATC) classes.
No pairwise significant comparison was found similar to Supplementary file 7. But the group comparison was very significant, p-value = 0.044.
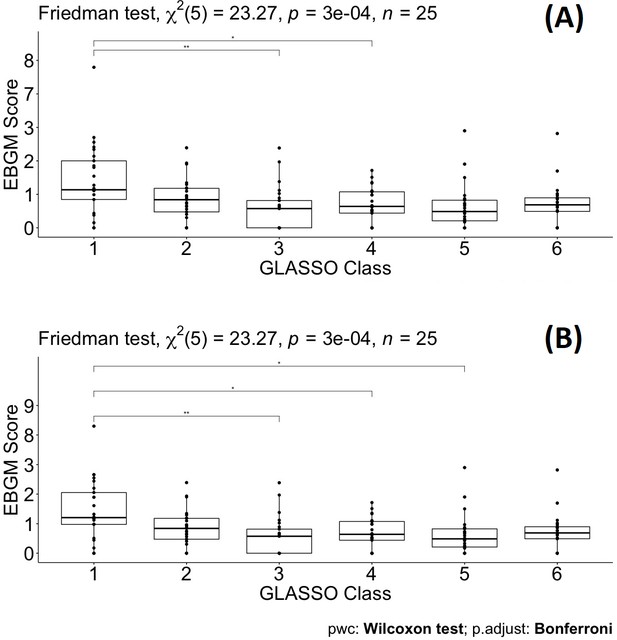
Pairwise Wilcoxon signed-rank test between different classes defined by graphical least absolute shrinkage and selection operator (GLASSO) (A) and pairwise Wilcoxon signed-rank test between different classes defined by GLASSO excluding tadalafil (B).
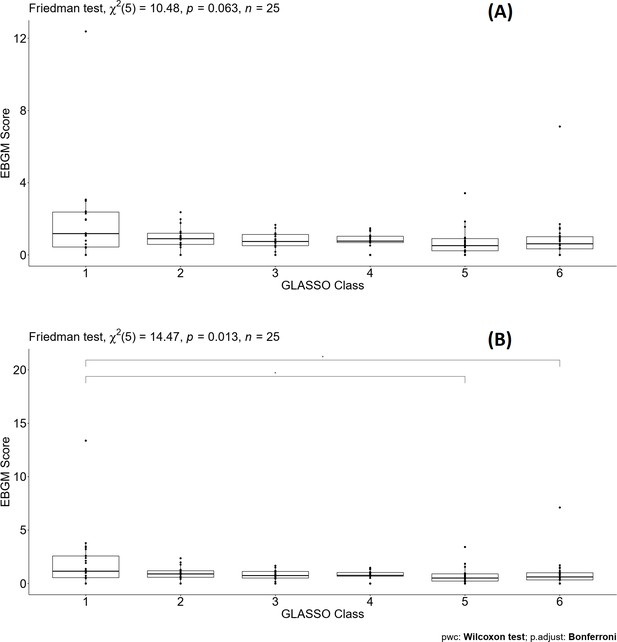
Pairwise Wilcoxon signed-rank test for different classes defined by graphical least absolute shrinkage and selection operator (GLASSO) (A) and the same test for different classes defined by GLASSO excluding warfarin (B) similar to Supplementary file 8.
Tables
Drug class after applying first the two filtering rules to obtain 44 drugs and then the elimination process from the penalized regression graphical least absolute shrinkage and selection operator (GLASSO) to obtain 22 drugs.
Drug class | # Reports(Total 612,733) | # Drugs after initial filtering(total 134) | # Drugs correspond to ≥2 ADEs in HLT codes when EB05 > 1 (total 44) | Drugs using GLASSO (total 22) |
---|---|---|---|---|
ACEIs | 69,327 | 13 | 3 | 1 |
ARBs | 87,415 | 8 | 5 | 3 |
Other RAS agents | 3,471 | 1 | 0 | 0 |
Other antihypertensive | 120,425 | 14 | 7 | 4 |
Antithrombotic agents | 67,767 | 10 | 7 | 3 |
Beta blocking agents | 74,574 | 13 | 3 | 1 |
Calcium channel blockers | 86,399 | 18 | 10 | 6 |
Diuretics | 29,394 | 14 | 3 | 1 |
Lipid modifying agents | 2,634 | 4 | 0 | 0 |
Urologicals | 18,186 | 4 | 2 | 2 |
Vasoprotectives | 909 | 1 | 0 | 0 |
Combinations | 52,232 | 34 | 4 | 1 |
The number of pulmonary adverse drug events (ADEs) when relative reporting ratio (RR) larger than two or the fifth quantile of empirical Bayes geometric mean (EBGM), EB05, larger than two after graphical least absolute shrinkage and selection operator (GLASSO) filtering process implemented in Table 1.
Drug | # Pulmonary ADEs | Order by EBGM | # Pulmonary ADEs | Order by RR |
---|---|---|---|---|
Macitentan | 16 | 1 | 10 | 2 |
Bosentan | 14 | 2 | 5 | 11 |
Epoprostenol | 11 | 4 | 9 | 4 |
Selexipag | 10 | 5 | 10 | 2 |
Sildenafil | 10 | 6 | 7 | 6 |
Tadalafil | 10 | 7 | 3 | 44 |
Beraprost | 7 | 10 | 13 | 1 |
Nifedipine | 5 | 13 | 5 | 11 |
Candesartan | 4 | 16 | 3 | 34 |
Althiazide/Spironolactone | 3 | 20 | 4 | 18 |
Bisoprolol | 3 | 21 | #N/A | #N/A |
Imidapril | 3 | 24 | 5 | 11 |
Azelnidipine | 2 | 30 | 4 | 23 |
Azilsartan Kamedoxomil | 2 | 31 | 3 | 32 |
Bendroflumethiazide | 2 | 32 | 3 | 33 |
Benidipine | 2 | 33 | 5 | 11 |
Cilnidipine | 2 | 34 | 5 | 11 |
Doxazosin | 2 | 36 | 3 | 36 |
Lercanidipine | 2 | 39 | 1 | 90 |
Nicardipine | 2 | 40 | 5 | 11 |
Rilmenidine | 2 | 42 | #N/A | #N/A |
Telmisartan | 2 | 43 | 4 | 30 |
Comparative analysis of each drug and associated pulmonary adverse drug events (ADEs) based on the new classification from different graphical least absolute shrinkage and selection operator (GLASSO) (GL) Clusters.
Drug | Drug class | ADEs for EB05 > 1 (n) * | GL Cluster |
---|---|---|---|
Macitentan | AHAs | 1–15,17 (16) | 1 |
Bosentan | AHAs | 1,2,4–15 (14) | 1 |
Epoprostenol | ATAs | 1,2,4–9,11,12,15 (11) | 1 |
Selexipag | ATAs | 2,4–12 (10) | 1 |
Sildenafil | UAs | 1,2,4–12 (10) | 1 |
Tadalafil | UAs | 1,2,4–12 (10) | 1 |
Beraprost | ATAs | 1,2,5–9 (7) | 1 |
Nifedipine | CCBs | 1–3,15,16 (5) | 2 |
Candesartan | ARBs | 1,3,14,16 (4) | 2 |
Althiazide\Spironolactone | COMBs | 4,10,11 (3) | 3 |
Rilmenidine | AHAs | 4,10 (2) | 3 |
Bisoprolol | BBAs | 1,2,14 (3) | 4 |
Lercanidipine | CCBs | 1,14 (2) | 4 |
Imidapril | ACEs | 1–3 (3) | 5 |
Azelnidipine | CCBs | 1,3 (2) | 5 |
Azilsartan Kamedoxomil | ARBs | 1,3 (2) | 5 |
Benidipine | CCBs | 1,2 (2) | 5 |
Cilnidipine | CCBs | 1,2 (2) | 5 |
Telmisartan | ARBs | 1,3 (2) | 5 |
Bendroflumethiazide | TDAs | 3,13 (2) | 6 |
Doxazosin | AHAs | 3,13 (2) | 6 |
Nicardipine | CCBs | 3,13 (2) | 6 |
-
*Below ADEs can be found corresponding to each drug:
-
1. Parenchymal lung disorders NEC.
-
2. Pneumothorax and pleural effusions NEC.
-
3. Lower respiratory tract inflammatory and immunologic conditions.
-
4. Respiratory tract disorders NEC.
-
5. Breathing abnormalities.
-
6. Lower respiratory tract signs and symptoms.
-
7. Pulmonary oedemas.
-
8. Respiratory failures (Excl Neonatal).
-
9. Vascular pulmonary disorders NEC.
-
10. Bronchospasm and obstruction.
-
11. Coughing and associated symptoms.
-
12. Respiratory syncytial viral infections.
-
13. Bronchial conditions NEC.
-
14. Pulmonary thrombotic and embolic conditions.
-
15. Lower respiratory tract infections NEC.
-
16. Fungal lower respiratory tract infections.
-
17. Pleural infections and inflammations.
The Friedman test for drugs in Anatomical Therapeutic Chemical (ATC) class and graphical least absolute shrinkage and selection operator (GLASSO) class.
ATC class | p-value (44 drugs) | p-value (22 drugs) | GL Cluster | The p-value for 22 drugs |
---|---|---|---|---|
ACEIs | 0.271 | – | 1 | <0.001 (0.199, when excluding tadalafil) |
ARBs | <0.001 | <0.001 | 2 | 0.110 |
AHAs | <0.001 | <0.001 | 3 | 0.884 |
ATAs | <0.001 | <0.001 | 4 | 0.346 |
BBAs | 0.0232 | – | 5 | 0.127 |
CCBs | 0.001 | 0.001 | 6 | 0.0522 |
COMBs | 0.236 | – | ||
TDAs | 0.0329 | – | ||
UAs | 0.127 | 0.127 |
-
The p-value for statistical significance is <0.05.
Additional files
-
Supplementary file 1
30 pulmonary ADEs.
- https://cdn.elifesciences.org/articles/70734/elife-70734-supp1-v2.docx
-
Supplementary file 2
Contribution of Pulmonary ADEs in 2D and 3D PCAs.
- https://cdn.elifesciences.org/articles/70734/elife-70734-supp2-v2.docx
-
Supplementary file 3
Frequency of pulmonary ADEs when RR larger than two or the 5th quantile of EBGM, EB05, large than two.
- https://cdn.elifesciences.org/articles/70734/elife-70734-supp3-v2.docx
-
Supplementary file 4
Description of arc diagram visualization.
- https://cdn.elifesciences.org/articles/70734/elife-70734-supp4-v2.docx
-
Supplementary file 5
Comparative analysis of drug and associated pulmonary ADEs in different GLASSO Clusters.
- https://cdn.elifesciences.org/articles/70734/elife-70734-supp5-v2.docx
-
Supplementary file 6
Friedman test for drugs in ATC class and GLASSO class.
- https://cdn.elifesciences.org/articles/70734/elife-70734-supp6-v2.docx
-
Supplementary file 7
A. Multiple comparisons of different ATC classes together with the adjusted p-value using the rigorous paired Wilcoxon signed-rank test with Bonferroni correction.
B. Multiple comparisons of different ATC classes excluding AHAs and UAs.
- https://cdn.elifesciences.org/articles/70734/elife-70734-supp7-v2.docx
-
Supplementary file 8
A. Multiple comparisons of drugs from GL Clusters and multiple comparisons of drugs from GL clusters excluding Tadalafil.
B. Multiple comparisons of drugs from GLASSO clusters and multiple comparisons of drugs from GLASSO clusters excluding Warfarin.
- https://cdn.elifesciences.org/articles/70734/elife-70734-supp8-v2.docx
-
Supplementary file 9
Dose distribution related to tadalafil and sildenafil and ADE.
- https://cdn.elifesciences.org/articles/70734/elife-70734-supp9-v2.docx
-
Transparent reporting form
- https://cdn.elifesciences.org/articles/70734/elife-70734-transrepform1-v2.pdf
-
Source data 1
This folder contains the data for R programs in the Source Code files.
- https://cdn.elifesciences.org/articles/70734/elife-70734-supp10-v2.zip
-
Source code 1
This folder contains R codes for the manuscript: Data-Driven Methodology COVID19 Related Pharmacovigilance.
- https://cdn.elifesciences.org/articles/70734/elife-70734-supp11-v2.zip