Estimation and worldwide monitoring of the effective reproductive number of SARS-CoV-2
Figures
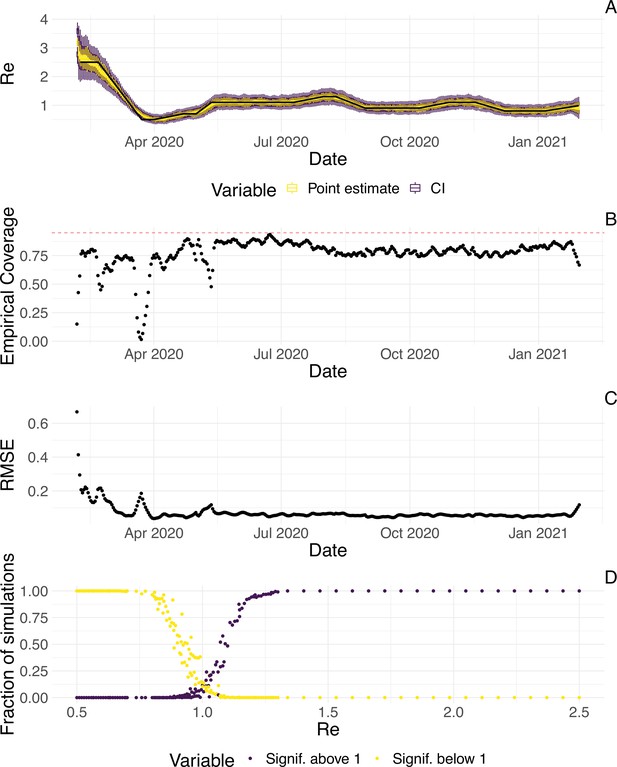
Evaluation of the pipeline on simulated data.
(A) The specified trajectory (black line) was used to stochastically simulate 100 trajectories of observed cases. From each trajectory, we estimated (yellow boxplots) and constructed a 95% confidence interval (purple boxplots of the lower/upper endpoint). (B) Fraction of simulations for which the true value was within the 95% confidence interval. The dashed red line indicates the nominal 95% coverage. (C) Root mean squared relative error for every time point. (D) Fraction of simulations for which is estimated to be significantly above or below one, depending on the true value of .
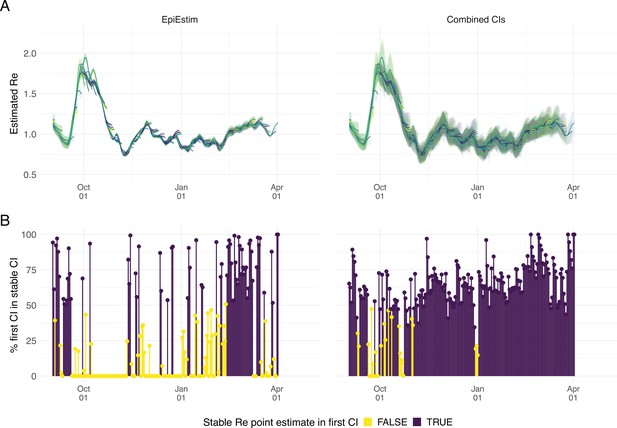
Stability of the Swiss estimates based on confirmed COVID-19 cases, upon adding additional days of observations.
(A) Line segments correspond to 3 weeks of estimates made with the same input data (e.g. data up to December 1st). The segments were assigned an arbitrary colour for ease of distinction. For each day, estimates and associated 95% confidence intervals (CIs) are overlaid, from the first possible estimate for that day up to estimates including 3 additional weeks of data. The latter, always the left end of a line segment, corresponds to the stable estimate. (B) Percentage of the first estimated CI that is contained in the stable CI based on data from 30 April 2021. This percentage was calculated as the width of the intersection of both CIs, divided by the width of the first CI. The colour indicates whether the stable estimate was contained in the first reported CI. In both rows, the left column shows uncertainty intervals from EpiEstim on the original data, and the right our improved 95% CIs. Both columns use the same pipeline, and differ only in the construction of the uncertainty intervals.
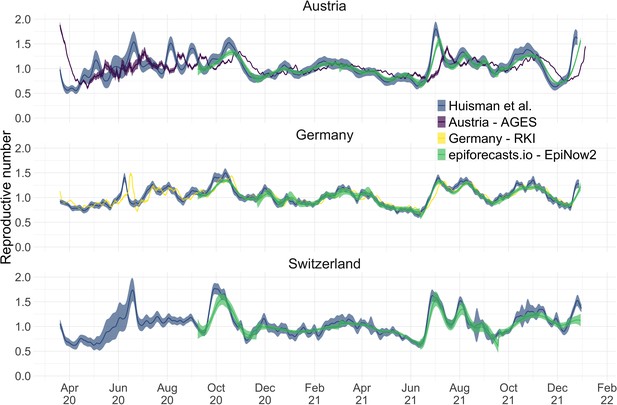
Comparison of published estimates for three countries.
Point estimates are presented with a solid line and 95% confidence intervals are presented as coloured ribbons.
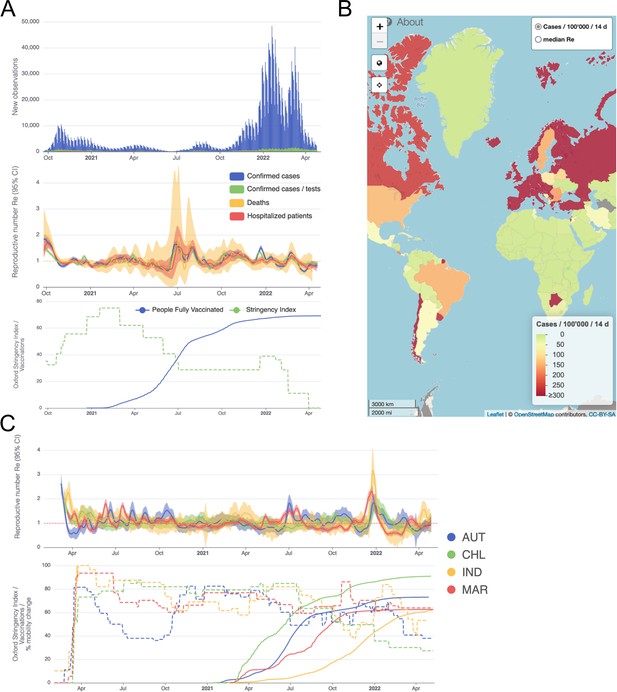
Example panels from the online dashboard.
(A) Swiss case incidence with evidence of weekly testing patterns (top row), estimates with associated 95% confidence intervals from four types of observation data (middle row), and timeline of stringency index and vaccination coverage (bottom row). (B) World map of incidence per 100’000 inhabitants over the last 14 days. One can also display the worldwide estimates instead. (C) Comparison of estimates across four countries (Austria, Chile, India and Morocco), with timelines of stringency indices and vaccination coverage. All panels were extracted on May 12, 2022. Dashboard url: https://ibz-shiny.ethz.ch/covid-19-re-international.
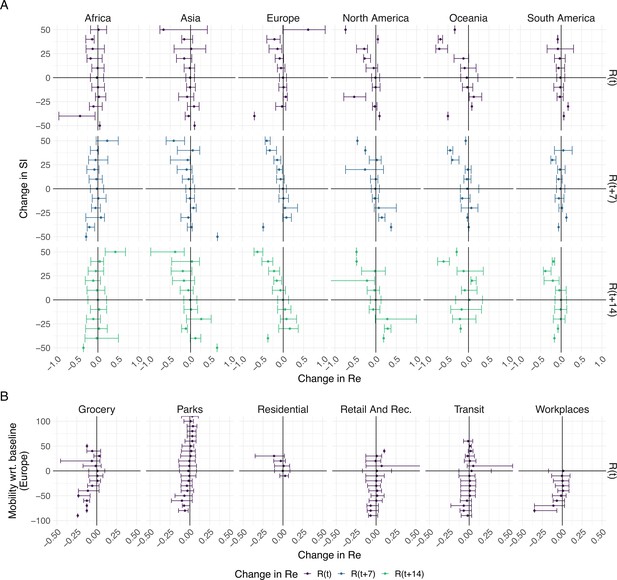
The association between the implementation or lifting of non-pharmaceutical interventions and changes in until May 2021.
(A) The change in the estimated at the same time as () or following ( and ) the implementation (above x-axis) or lifting (below x-axis) of NPIs in a given week. (B) The change in the estimated related to the change in mobility in the same week. The error bars indicate the Q1 and Q3 quartiles.
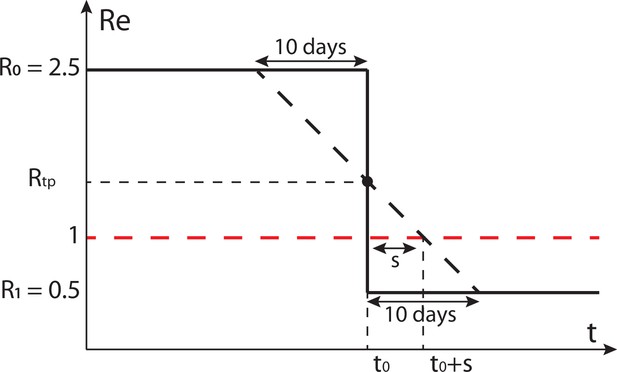
Schematic of the effect of smoothing on the ability to estimate when .
The true is indicated by the black solid line, the black dashed line shows a linear approximation of the smoothed . Instead of crossing 1 at , this line crosses 1 at .
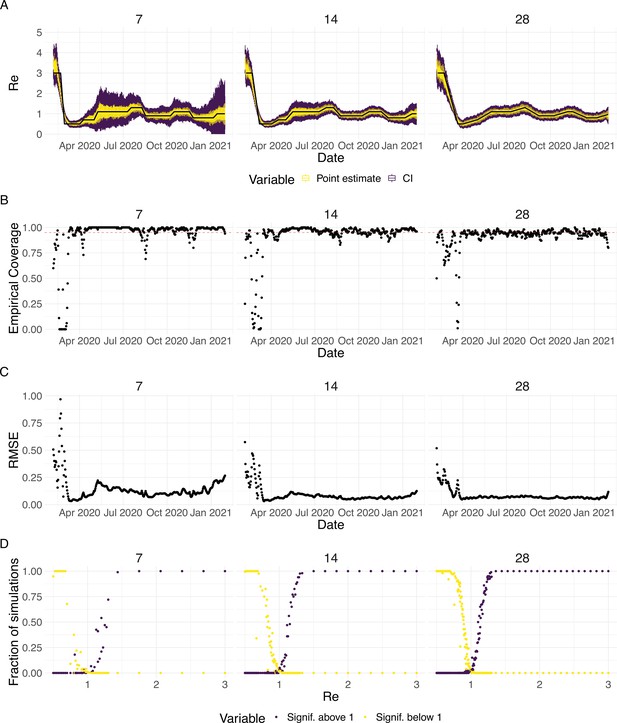
Performance of our method on simulated scenarios with differing slopes.
(A) The specified trajectory (black line; see Methods) was used to simulate a trajectory of reported cases (with Swiss case observation noise) 100 times. From each trajectory we estimated (yellow boxplots), and constructed a 95% confidence interval (purple boxplots of the lower/upper endpoint). We varied the time it took to change from one value to the next, (columns). Larger values of correspond to less abrupt changes. (B) The fraction of simulations where the true value was within the 95% confidence interval. The dashed red line indicates the nominal 95% coverage. (C) The root mean squared relative error for every time point. (D) The fraction of simulations where we estimate is significantly above or below one, depending on the true value of . We see that the method closely tracks the true in all scenarios, although the error is greater for steeper slopes. In the case of steeper changes in the overall size of the epidemic is also smaller, which explains the larger confidence intervals.
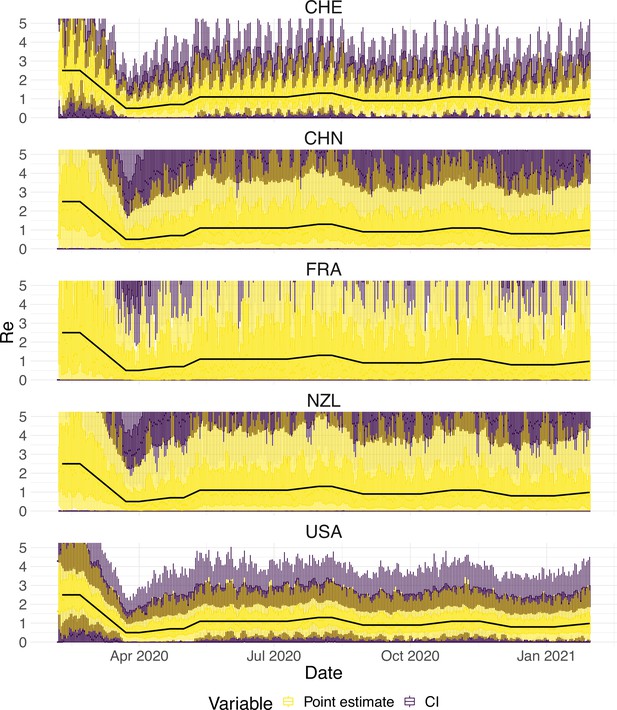
Performance of our method, modified to skip the smoothing step in the pipeline, on simulated scenarios with observation noise.
The specified trajectory (black line; see Methods) was used to simulate a trajectory of reported cases (with varying country-specific noise profiles; rows) 100 times. From each trajectory we estimated (yellow boxplots), and constructed a 95% confidence interval (purple boxplots of the lower/upper endpoint). Contrary to our normal pipeline, the observations were not smoothed prior to the deconvolution and estimation. We see that the estimates are highly variable.
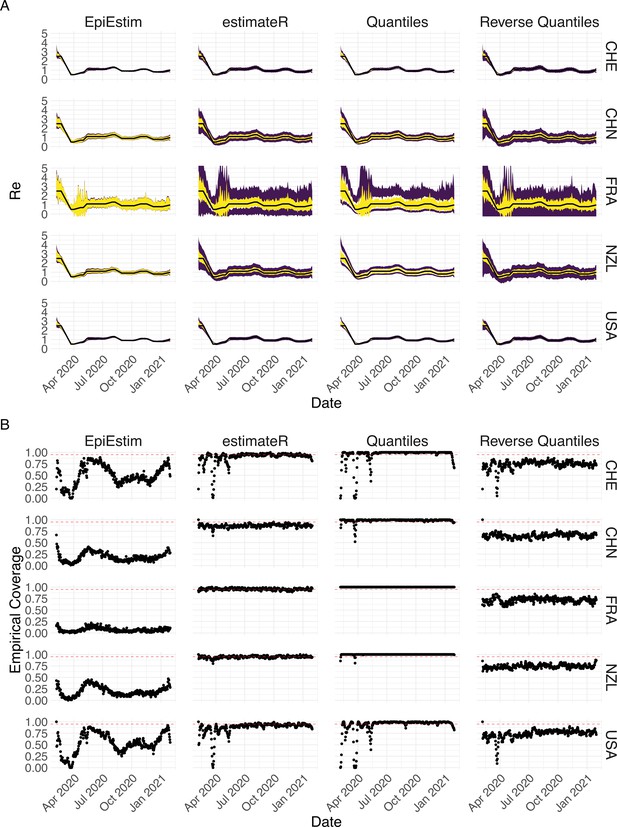
Performance of our method on simulated scenarios with observation noise.
The columns differ in the method used to construct confidence intervals: EpiEstim reports the 95% HPD of EpiEstim on the original data, estimateR refers to our method, Quantiles and Reverse Quantiles use the 5 and 95% quantiles of the estimated to construct the CIs. (A) The specified trajectory (black line; see Methods) was used to simulate a trajectory of reported cases (with varying country-specific noise profiles; rows) 100 times. From each trajectory we estimated (yellow ribbons represent the estimated mean ± sd across 100 simulations), and constructed a 95% confidence interval (purple ribbons represent the mean ± sd of the estimated lower/upper endpoint). (B) The fraction of simulations where the true value was within the 95% confidence interval. The dashed red line indicates the nominal 95% coverage.
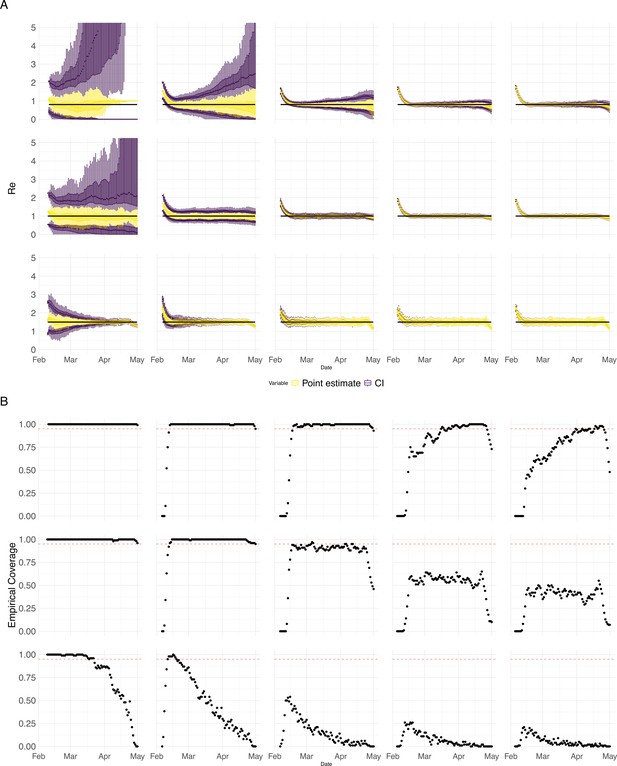
Performance of our method on simulated scenarios with varying population size, using confidence intervals from EpiEstim.
(A) We specified a constant value (black line; rows) to simulate a trajectory of reported cases (with Swiss case observation noise) 100 times. From each trajectory we estimated (yellow boxplots), and constructed a 95% confidence interval (purple boxplots of the lower/upper endpoint). The simulated scenarios had differing initial incidence of infections per day (columns). In the top row, so the epidemic is decreasing. In the middle row, , the epidemic is constant, and in the bottom row, , the epidemic is increasing. The bias at the start is due to the initialisation of the simulation. (B) The fraction of simulations where the true value was within the 95% confidence interval. The dashed red line indicates the nominal 95% coverage. We see that the EpiEstim coverage strongly declines with increased epidemic size.
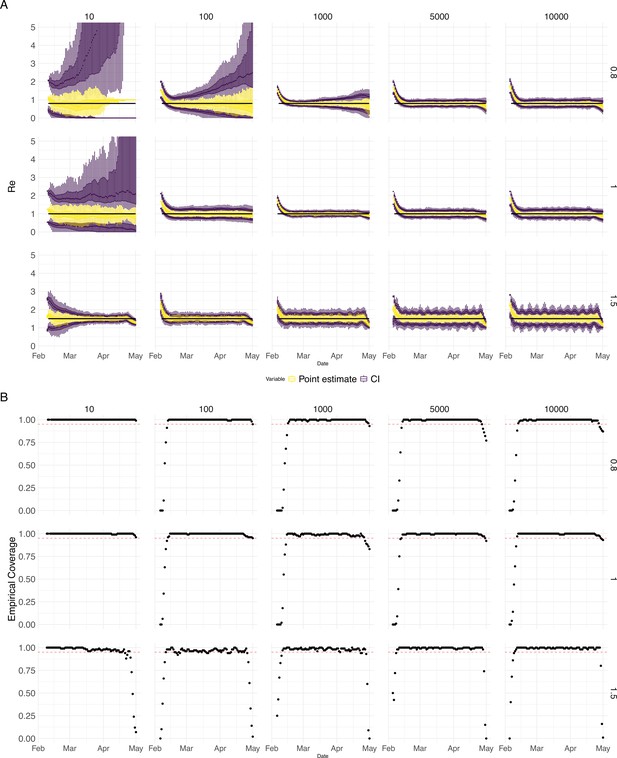
Performance of our method on simulated scenarios with varying population size, using the Union of EpiEstim and Block bootstrap 95% confidence intervals.
(A) We specified a constant value (black line; rows) to simulate a trajectory of reported cases (with Swiss case observation noise) 100 times. From each trajectory we estimated (yellow boxplots), and constructed a 95% confidence interval (purple boxplots of the lower/upper endpoint). The simulated scenarios had differing initial incidence of infections per day (columns). In the top row, so the epidemic is decreasing. In the middle row, , the epidemic is constant, and in the bottom row, , the epidemic is increasing. The bias at the start is due to the initialisation of the simulation. (B) The fraction of simulations where the true value was within the 95% confidence interval. The dashed red line indicates the nominal 95% coverage. We see that for a wide range of infection incidences, our 95% confidence interval is informative and covers the true value of .
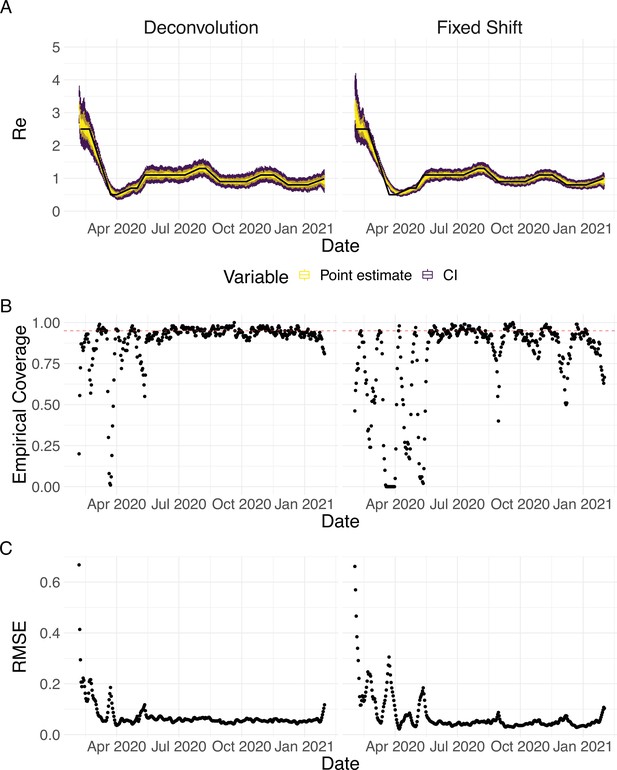
Performance of our method on simulated scenarios using a fixed shift versus the deconvolution to infer infection incidence.
The fixed shift method shifts the observations back by the mean of the delay distribution (here assumed to correspond to confirmed cases). (A) The specified trajectory (black line; see Methods) was used to simulate a trajectory of reported cases (with Swiss case observation noise) 100 times. From each trajectory we estimated (yellow boxplots), and constructed a 95% confidence interval (purple boxplots of the lower/upper endpoint). (B) The fraction of simulations where the true value was within the 95% confidence interval. The dashed red line indicates the nominal 95% coverage. The average coverage in this scenario was 0.90 with deconvolution and 0.78 with the fixed shift. (C) The root mean squared relative error for every time point. The average (cumulative) RMSE in this scenario was 0.0706 (25.4) with deconvolution and 0.0726 (26.6) with the fixed shift.
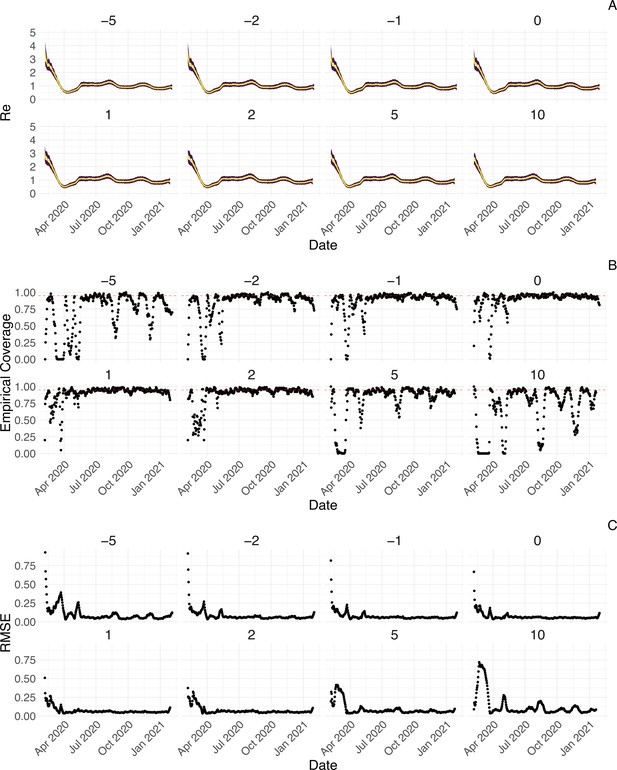
Performance of our method on simulated scenarios with misspecified delay distributions.
When estimating , we misspecified the mean of the delay distribution (5.5 for symptom-onset to case confirmation) by the numbers above the columns. (A) The specified trajectory (black line; see Methods) was used to simulate a trajectory of reported cases (with Swiss case observation noise) 100 times. From each trajectory we estimated (yellow boxplots), and a 95% confidence interval (purple boxplots of the lower/upper endpoint). (B) The fraction of simulations where the true value was within the 95% confidence interval. The dashed red line indicates the nominal 95% coverage. The average coverage in this scenario was 0.72, 0.85, 0.88, 0.90, 0.90, 0.89, 0.82, 0.69 from –5 to 10. (C) The root mean squared relative error for every time point. The average (cumulative) RMSE in this scenario was 0.0989 (36.0), 0.0807 (29.2), 0.0746 (26.9), 0.0706 (25.4), 0.0701 (25.2), 0.0726 (26.0), 0.0909 (32.3), 0.134 (47.0) from –5 to 10.
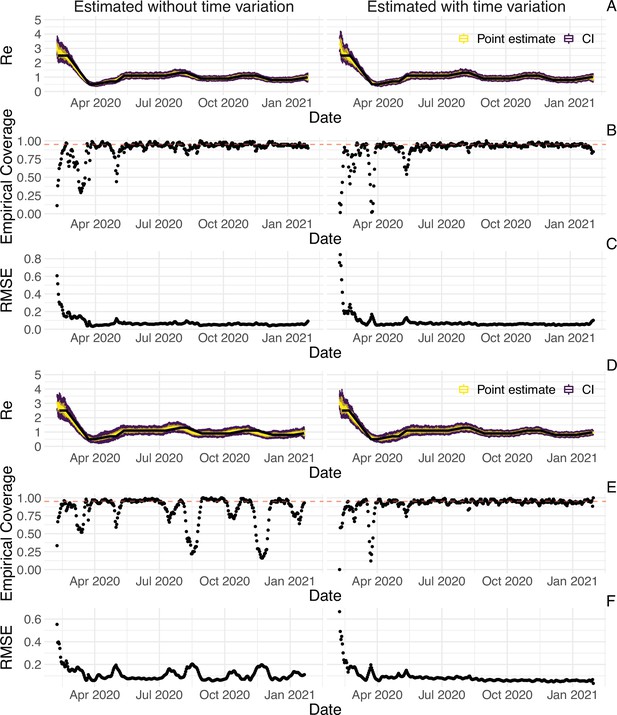
Performance of our method on simulated scenarios with time-varying delay distributions.
The observations were simulated with a time-varying delay distribution for (A,B,C) confirmed cases, or (D,E,F) deaths (see Methods), and then estimated with (right column) or without (left column) taking the time-varying distributions into account. (A, D) The specified trajectory (black line; see Methods) was used to simulate a trajectory of reported cases or deaths (with Swiss case observation noise) 100 times. From each trajectory we estimated (yellow boxplots), and a 95% confidence interval (purple boxplots of the lower/upper endpoint). (B, E) The fraction of simulations where the true value was within the 95% confidence interval. The dashed red line indicates the nominal 95% coverage. For the cumulative cases, the average coverage in this scenario was 0.89 without and 0.89 with time variation. For the deaths, the average coverage was 0.83 without and 0.92 with time variation. (C, F) The root mean squared relative error for every time point. For the cumulative cases, the average (cumulative) RMSE was 0.0728 (26.2) without and 0.0783 (28.5) with time variation. For the deaths, the average (cumulative) RMSE was 0.113 (39.5) without and 0.0861 (30.8) with time variation.
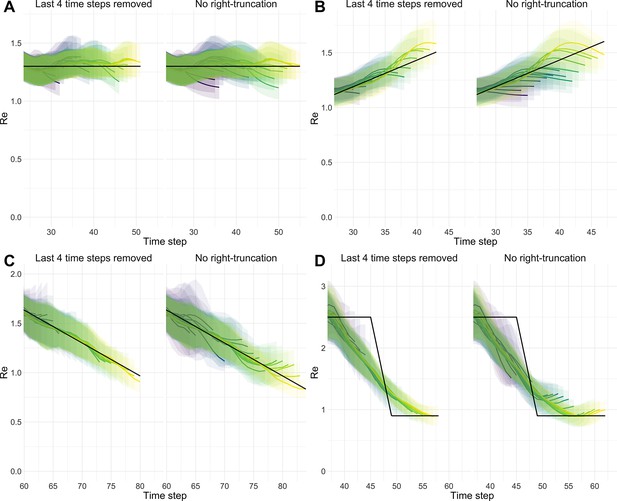
Stability of estimates at present.
We estimated through time repeatedly on 4 scenarios. With each new iteration, we added one new data point at present. Each trajectory is presented with its own colour. Purple trajectories are iterations for which the last known case data point was furthest in the past, yellow trajectories are trajectories for which the last known case data point was closest to the present. In each panel and for each trajectory, 100 simulation replicates were aggregated. The median of mean estimates are presented with lines and medians of upper and lower bounds of 95% confidence intervals are shown with translucent ribbons. For each scenario, two panels are presented. Each time the right panel correspond to raw estimates and the left panel corresponds to the same estimates with the last 4 values removed from each trajectory. (A) Stable . (B) Gradual increase in . (C) Gradual decrease in . (D) Abrupt decrease in .
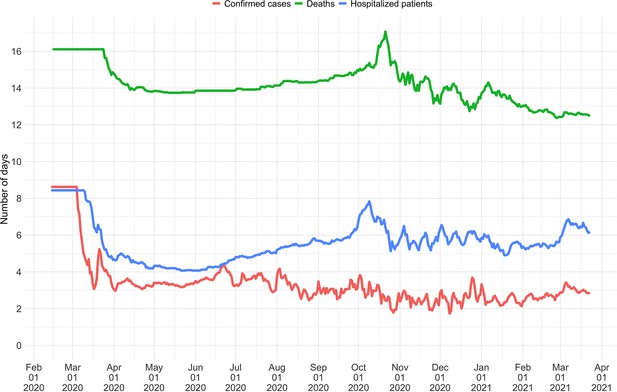
Mean delay in Switzerland between onset of symptoms and reporting.
For each date, the mean is taken over the last 300 reports with known symptom onset date, based on line list data from the FOPH. For early dates, before 300 reports are available, the mean is taken over the first 300 reports.
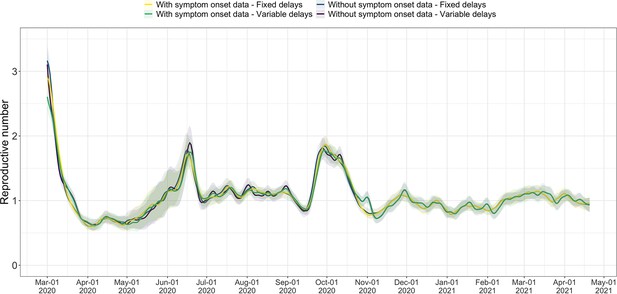
Comparison of the estimates with or without accounting for known symptom onset dates and for time-variability on reporting delays.
The comparison is based on time series of confirmed cases in Switzerland, from line list data provided by the FOPH. Both the inclusion of known symptom onset dates and of the time-variability of reporting delay distributions have an effect on the Re estimates, in particular for early estimates in this case. The fraction of cases with known symptom onset date has drastically reduced since November 2020, hence the overlap in curves with and without symptom onset data for later dates. For each trajectory the point estimate is shown with a line, and the translucent ribbon indicates the 95% confidence interval.
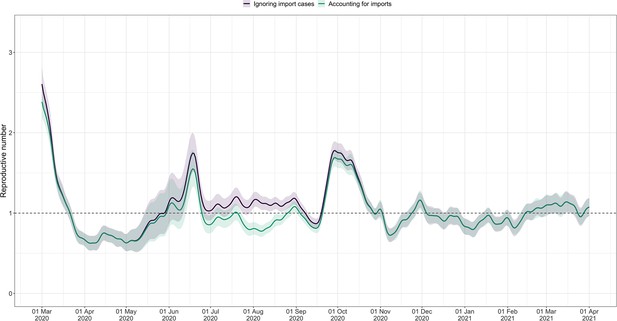
Effective reproductive number estimates with or without accounting for known imports.
The comparison is based on time series of confirmed cases in Switzerland, from line list data provided by the FOPH. The analysis ignoring imports is unbiased if the number of imports equals the number of exports. Since the analysis accounting for imports is not accounting for exports, the results are a lower limit for the effective reproductive number. Very few imported cases were reported since November 2020, hence the complete overlap in the curves after that date. For each trajectory the point estimate is shown with a line, and the translucent ribbon indicates the 95% confidence interval.
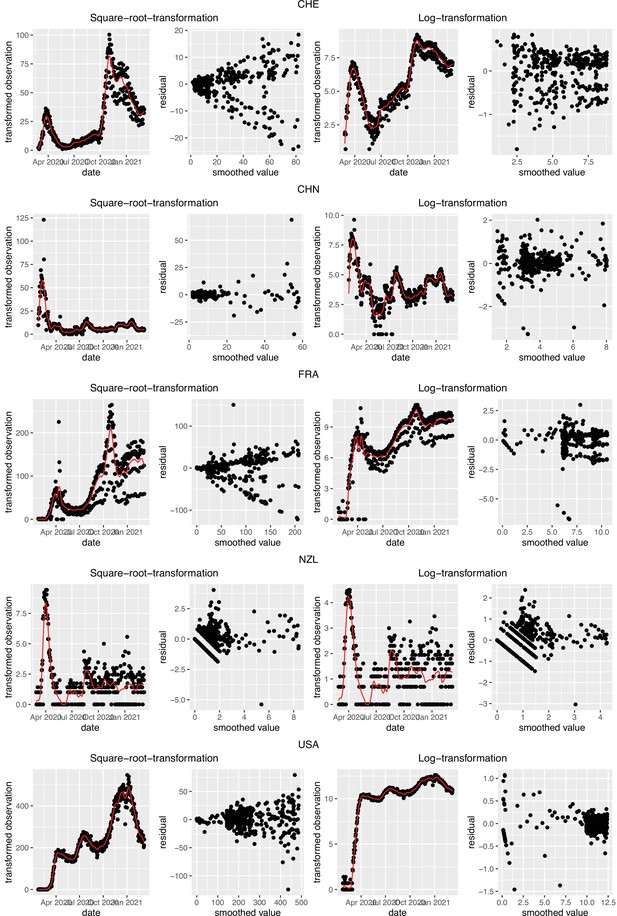
Square root and log transformations to stabilise the variance of residuals.
Each row corresponds to the results of observations from Switzerland (CHE), China (CHN), France (FRA), New Zealand (NZL) and the United States (USA), respectively. The first and last two plots correspond to the result of square root transformation and log transformation, respectively.
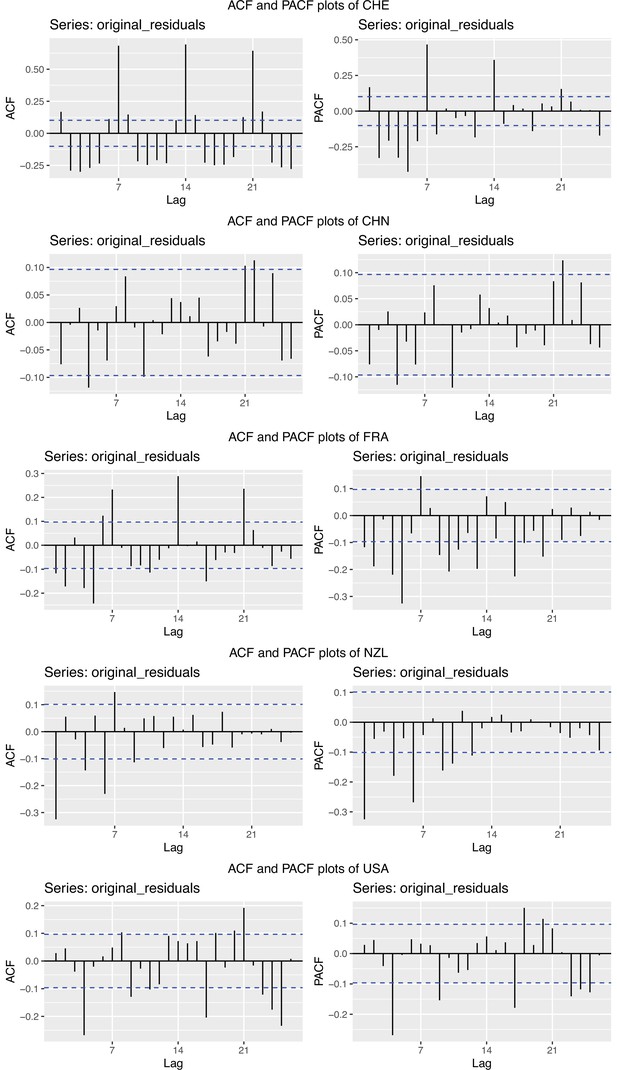
Autocorrelation function (ACF) and partial autocorrelation function (PACF) plots of the observations from five different countries.
In each row, the two plots are the ACF and PACF plots of the observations from Switzerland (CHE), China (CHN), France (FRA), New Zealand (NZL) and the United States (USA), respectively.
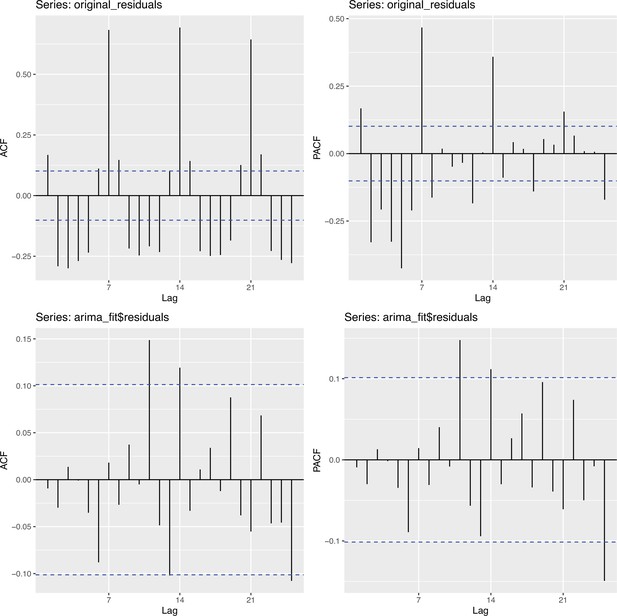
Autocorrelation function (ACF) and partial autocorrelation function (PACF) plots of observations from Switzerland and the fitted ARIMA model.
The two plots on the upper row are the ACF and PACF plots of the observations from Switzerland. The two plots on the lower row are the ACF and PACF plots of the residuals of the fitted ARIMA model.
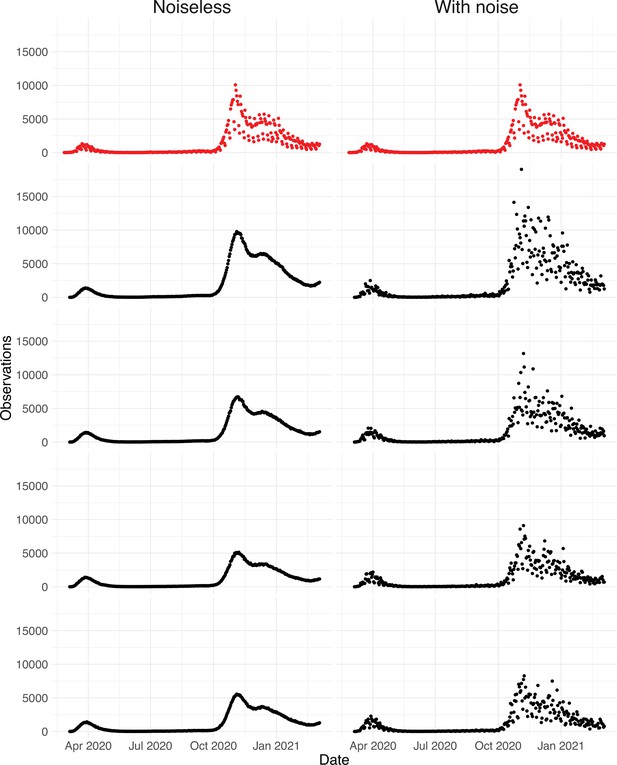
Simulated observations with and without noise.
The upper row shows the real observations from Switzerland (twice the same). The other four rows show simulated observations, the left column shows simulations without the noise term ( in Section 4.7), and the right the simulated observations with the noise term ( in Section 4.7).
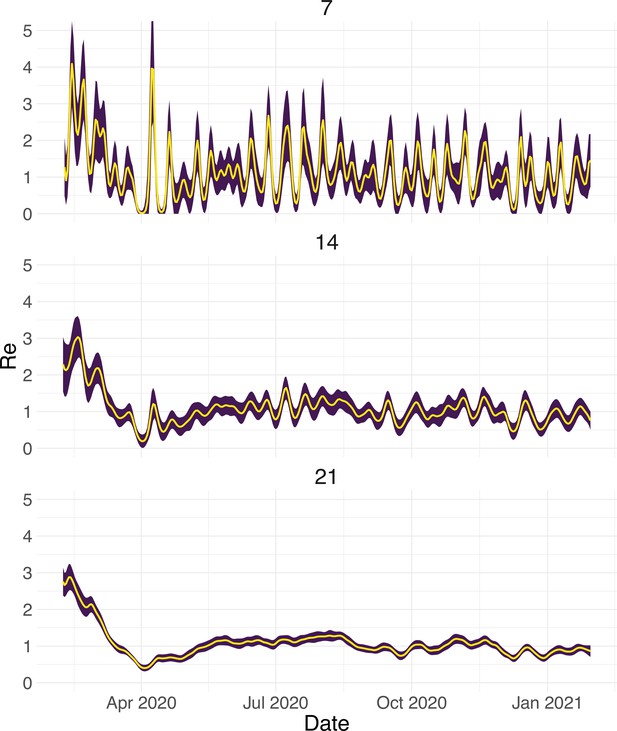
Estimated with different smoothing windows.
For each trajectory the point estimate is shown with a yellow line, and the purple ribbon indicates the 95% confidence interval.
Tables
Investigating the relation between the date of ‘lockdown’ and the date when the estimated based on case reports dropped below 1.
Based on news reports, we report when a country implemented stay-at-home orders (a ‘lockdown’). The column ‘’ indicates when the point estimate first dropped below 1. The column ‘CI includes 1’ details the corresponding time interval where the 95% confidence interval included 1. Of the investigated countries that implemented a nationwide lockdown, four (Denmark, Germany, the Netherlands, Slovenia) had 95% confidence intervals that included 1 or were below before a nationwide lockdown was implemented. The column ‘Time until ’ indicates the number of days between the lockdown and the date that the point estimate dropped below 1.
Country | Lockdown | Re <1 | CI includes 1 | Time until Re <1 |
---|---|---|---|---|
Austria | 16–30 | 20–30 | [20-03, 20-03] | 4 days |
Belgium | 18–30 | 30–30 | [25-03, 03-04] | 12 days |
Denmark | 18–30 | ≤10–03 | [≤10–03, 20–06] | –8 days |
Finland | 16–30 | 01-Feb | [29-03, 30-04] | 17 days |
France | 17–30 | 27–30 | [23-03, 07-04] | 10 days |
Germany | 22–30 | 18–30 | [17-03, 19-03] | –4 days |
Ireland | 27–30 | 01–100 | [04–04, 15–04] | 12 days |
Italy | 01–300 | 18–30 | [17-03, 19-03] | 8 days |
Netherlands | 23–30 | 01-Jan | [22-03, 10-04] | 13 days |
Norway | 14–30 | 21–30 | [17-03, 19-03] | 7 days |
Poland | 25–30 | 01-Jan | [31-03, 17-04] | 8 days |
Portugal | 16–30 | 28–30 | [23-03, 15-04] | 12 days |
Romania | 24–30 | 01-Jun | [31-03, 29-04] | 13 days |
Russian Federation | 30–30 | 01-Apr | [01–05, 08–05] | 35 days |
Slovenia | 20–30 | 23–30 | [≤13–03, 26–03] | 3 days |
Spain | 14–30 | 26–30 | [25-03, 26-03] | 12 days |
Sweden | 01-Jan | [06–03,≥03-05-2021] | ||
Switzerland | 17–30 | 22–30 | [20-03, 22-03] | 5 days |
Turkey | 21–30 | 01-Aug | [01–04, 13–04] | 18 days |
United Kingdom | 24–30 | 30–30 | [28-03, 20-04] | 6 days |
Gamma distributions used in the pipeline: serial interval, incubation period, and the delay distributions assumed for each observation type.
Distribution | Mean (days) | SD (days) | Reference |
---|---|---|---|
Serial interval | 4.8 | 2.3 | Nishiura et al., 2020 |
Infection to onset of symptoms | 5.3 | 3.2 | Linton et al., 2020 |
Onset of symptoms to case confirmation | 5.5 | 3.8 | Bi et al., 2020 |
Onset of symptoms to hospital admission | 5.1 | 4.2 | Pellis et al., 2021 |
Onset of symptoms to death | 15.0 | 6.9 | Linton et al., 2020 |
The effect of smoothing on the ability to estimate when .
These values were calculated using Equation 11.
R0 | R1 | (per day) | Delays (days) | |
---|---|---|---|---|
6.0 | 0.0 | 3.0 | –0.30 | 6.7 |
3.0 | 0.0 | 1.5 | –0.15 | 3.3 |
3.5 | 0.5 | 2.0 | –0.15 | 6.7 |
2.5 | 0.5 | 1.5 | –0.10 | 5.0 |
3.3 | 0.9 | 2.1 | –0.12 | 9.2 |
1.8 | 0.8 | 1.3 | –0.05 | 6.0 |
Residuals and their corresponding days of the week.
Day of the week | Mon | Tue | Wed | Thu | Fri | Sat | Sun |
---|---|---|---|---|---|---|---|
Residuals | e1 | e2 | e3 | e4 | e5 | e6 | |
e7 | e8 | e9 | e10 | e11 | e12 | e13 | |
e14 | e15 | e16 | e17 | e18 | e19 | e20 | |
e21 | e22 | e23 | e24 | e25 | e26 | e27 | |
e28 | e29 | e30 |
Investigating the relation between the date of ‘lockdown’ and the date that the estimated dropped below 1.
The first three columns contain the same information as the first four columns of the main text Table 1. The last two columns are analogous to the third (‘ based on confirmed cases’) but are based on estimates for hospitalisations and deaths respectively. For each of these observation types, we used our method to determine when the estimate first dropped below 1, and for which dates the corresponding 95% confidence interval contained 1. Further, we used news reports to determine when a country implemented stay-at-home orders (a ‘lockdown’). Based on our estimates for confirmed cases, Denmark, Germany, the Netherlands, and Slovenia had 95% confidence intervals that included or were below one before a nationwide lockdown was implemented. For estimates based on COVID-19 deaths, there are also four: Denmark, the Netherlands, Poland, and the United Kingdom. See Appendix 2 for smoothing related caveats.
Country | Lockdown | based on Confirmed cases | based on Deaths | based on Hospitalisations |
---|---|---|---|---|
Austria | 16–03 | 20–03 [20-03, 20-03] | ||
Belgium | 18–03 | 30–03 [25-03, 03-04] | 26–03 [24-03, 26-03] | 25–03 [24-03, 25-03] |
Denmark | 18–03 | 10–03 [10-03, 20-06] | 22–03 [18-03, 07-01] | |
Finland | 16–03 | 02–04 [29-03, 30-04] | 07–04 [25-03, 11-04] | |
France | 17–03 | 27–03 [23-03, 07-04] | 24–03 [22-03, 26-03] | 27–03 [25-03, 26-03] |
Germany | 22–03 | 18–03 [17-03, 19-03] | 31–03 [23-03, 04-04] | |
Ireland | 27–03 | 08–04 [04–04, 15–04] | 05–04 [31-03, 09-04] | 06–04 [06–04, 26–04] |
Italy | 10–03 | 18–03 [17-03, 19-03] | 14–03 [01–03, 29–05] | |
Netherlands | 23–03 | 05–04 [22-03, 10-04] | 22–03 [19-03, 02-04] | 26–03 [24-03, 26-03] |
Norway | 14–03 | 21–03 [17-03, 24-03] | 25–03 [18-03, 08-04] | |
Poland | 25–03 | 02–04 [31-03, 17-04] | 09–04 [24-03, 01-12] | |
Portugal | 16–03 | 28–03 [23-03, 15-04] | 28–03 [21-03, 12-04] | |
Romania | 24–03 | 06–04 [31-03, 29-04] | 17–04 [25-03, 28-04] | |
Russian Federation | 30–03 | 04–05 [01–05, 08–05] | 18–05 [14-05, 12-12] | |
Slovenia | 20–03 | 23–03 [13-03, 26-03] | 26–03 [20–03, ≥03-05-2021] | |
Spain | 14–03 | 26–03 [25-03, 26-03] | ||
Sweden | 01–04 [06–03,≥03-05-2021] | 05–04 [13–03,≥03-05-2021] | ||
Switzerland | 17–03 | 22–03 [20-03, 22-03] | 21–03 [18-03, 23-03] | 18–03 [16-03, 18-03] |
Turkey | 21–03 | 08–04 [01–04, 13–04] | 04–04 [31-03, 06-04] | |
United Kingdom | 24–03 | 30–03 [28-03, 20-04] | 25–03 [24-03, 25-03] | 29–03 [27-03, 29-03] |
News and public resources used to determine when a country implemented the first non-pharmaceutical interventions, and a nationwide lockdown.
Additional files
-
Supplementary file 1
A table summarising the sources of incidence data.
- https://cdn.elifesciences.org/articles/71345/elife-71345-supp1-v2.xlsx
-
Supplementary file 2
A table comparing the structure and features of different methods to estimate Re.
- https://cdn.elifesciences.org/articles/71345/elife-71345-supp2-v2.xlsx
-
Transparent reporting form
- https://cdn.elifesciences.org/articles/71345/elife-71345-transrepform1-v2.pdf