A crowd of BashTheBug volunteers reproducibly and accurately measure the minimum inhibitory concentrations of 13 antitubercular drugs from photographs of 96-well broth microdilution plates
Figures
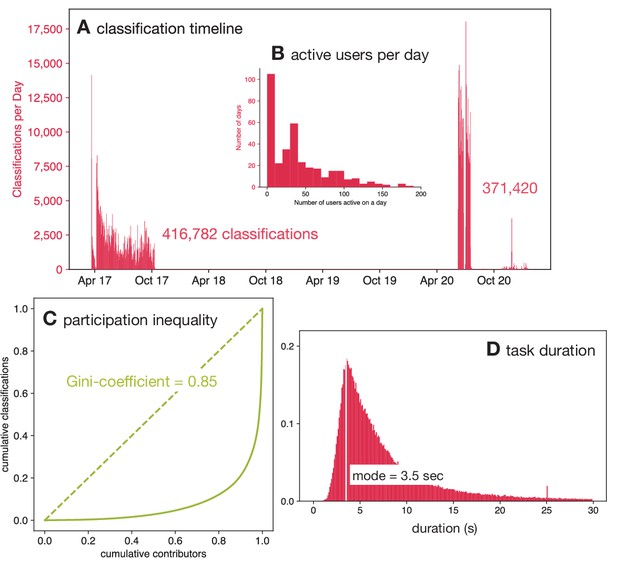
This dataset of 778,202 classifications was collected in two batches between April 2017 and Sep 2020 by 9372 volunteers.
(A) The classifications were done by the volunteers in two distinct batches; one during 2017 and a later one in 2020. Note that the higher participation during 2020 was due to the national restrictions imposed due to the SARS-Cov-2 pandemic. (B) The number of active users per day varied from zero to over 150. (C) The Lorenz curve demonstrates that there is considerable participation inequality in the project resulting in a Gini-coefficient of 0.85. (D) Volunteers spent different lengths of time classifying drug images after 14 days of incubation with a mode duration of 3.5 s.
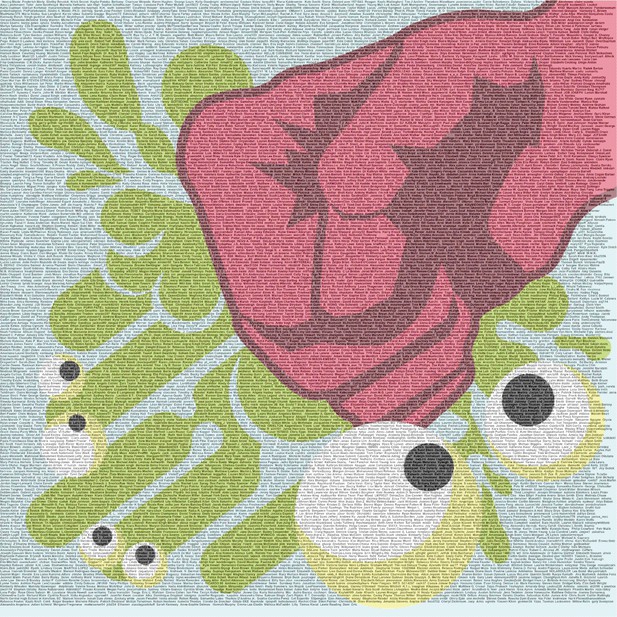
Thank you to all the volunteers who contributed one or more classifications to this manuscript.
There are the 5810 usernames of all the volunteers in this montage – volunteers who did not register or sign in are not included.
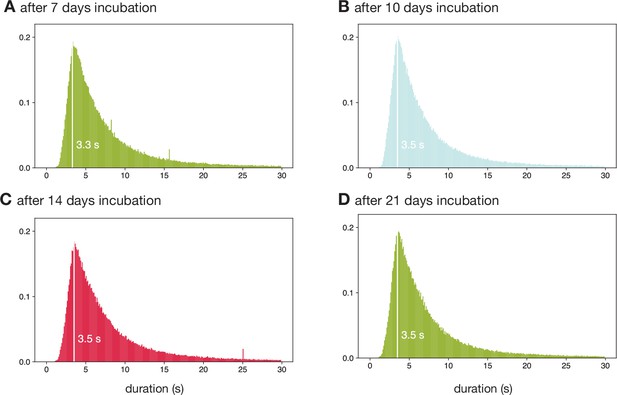
The time spent by volunteers on each classification varied with a mode of 3.5 s.
Since one would expect different amounts of bacterial growth on the microdilution plates after (A) 7, (B) 10, (C) 14 and (D) 21 days the distributions of these were examined separately. All were, however, similar indicating that this did not have a significant effect.
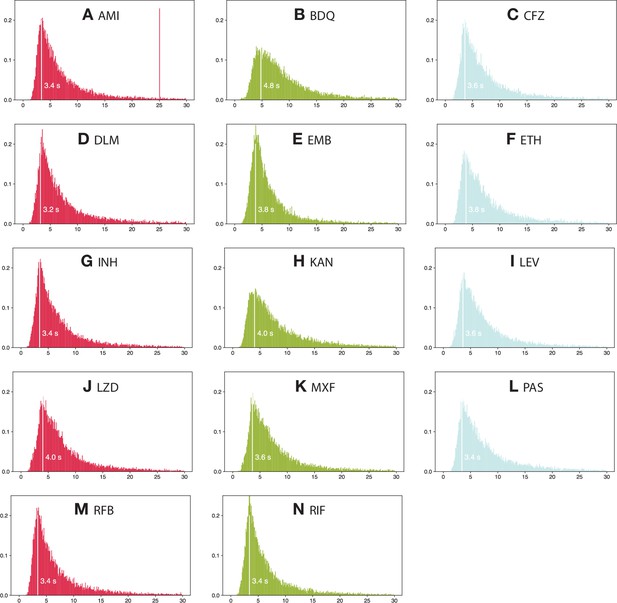
The time spent by volunteers on each classification varied depending on the drug being considered.
The mode of each distribution is labelled. The drug the volunteers spent the longest on (bedaquiline, mode 4.8 s) was also one of those with the largest number (8) of wells. As measured by its mode of 3.2 s, the volunteers spent the least time classifying delamanid.
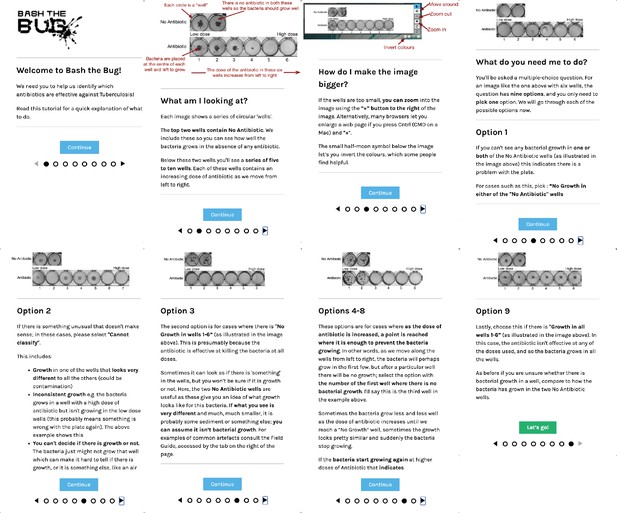
Every new user is shown this tutorial when they first join the BashTheBug Zooniverse project.
It uses example images to explain the task and then each of the options that they can choose to classify a drug image.
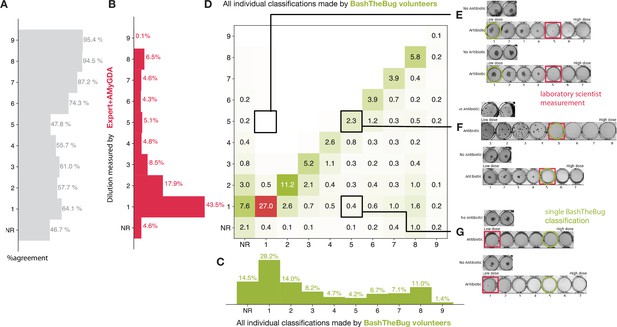
Heatmap showing how all the individual BashTheBug classifications (n=214,164) compare to the dilution measured by the laboratory scientist using the Thermo Fisher Vizion instrument after 14 days incubation (n=12,488).
(A) The probability that a single volunteer exactly agrees with the Expert +AMyGDA dataset varies with the dilution. (B) The distribution of all dilutions in the Expert +AMyGDA dataset after 14 days incubation. The differences are due to different drugs having different numbers of wells as well as the varying levels of resistance in the supplied strains. NR includes both plates that could not be read due to issues with the control wells and problems with individual drugs such as skip wells. (C) The distribution of all dilutions measured by the BashTheBug volunteers. (D) A heatmap showing the concordance between the Expert +AMyGDA dataset and the classifications made by individual BashTheBug volunteers. Only cells with gt0.1% are labelled. (E) Two example drug images where both the Expert and AMyGDA assessed the MIC as being a dilution of 5 whilst a single volunteer decided no growth could be seen in the image. (F) Two example drug images where both the laboratory scientist and a volunteer agreed that the MIC was a dilution of 5. (G) Two example drug images where the laboratory scientist decided there was no growth in any of the wells, whilst a single volunteer decided there was growth in the first four wells.
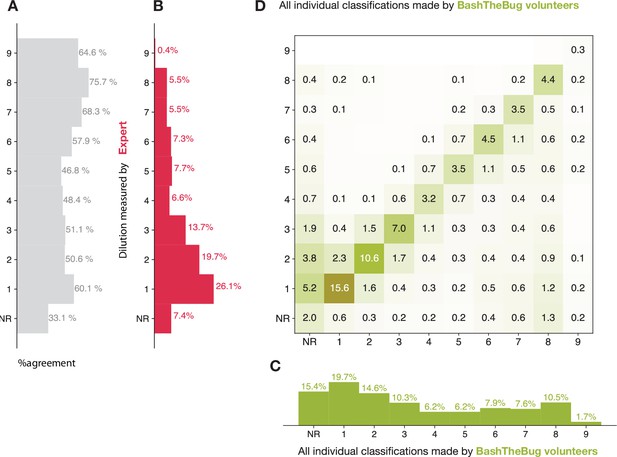
Heatmap showing how all the individual BashTheBug classifications (n=214,164) compare to the set of dilutions where the measurement made by the laboratory scientist using the Thermo Fisher Vizion instrument and a mirrored box after 14 days incubation concur (n=9402) (A).
The probability that a single volunteer exactly agrees with the Expert dataset varies with the dilution. The distribution of all MIC dilutions after 14 days incubation read by (B) laboratory scientists and (C) BashTheBug volunteers. NR includes both plates that could not be read due to issues with the control wells and problems with individual drugs such as skip wells. (D) A heatmap showing how for each set of images assessed by the laboratory scientist has having a specific dilution as the MIC, the classifications made by BashTheBug volunteers varied considerably. It is normalised so that each row sums to 100% and only cells with >0.1 % are labelled.
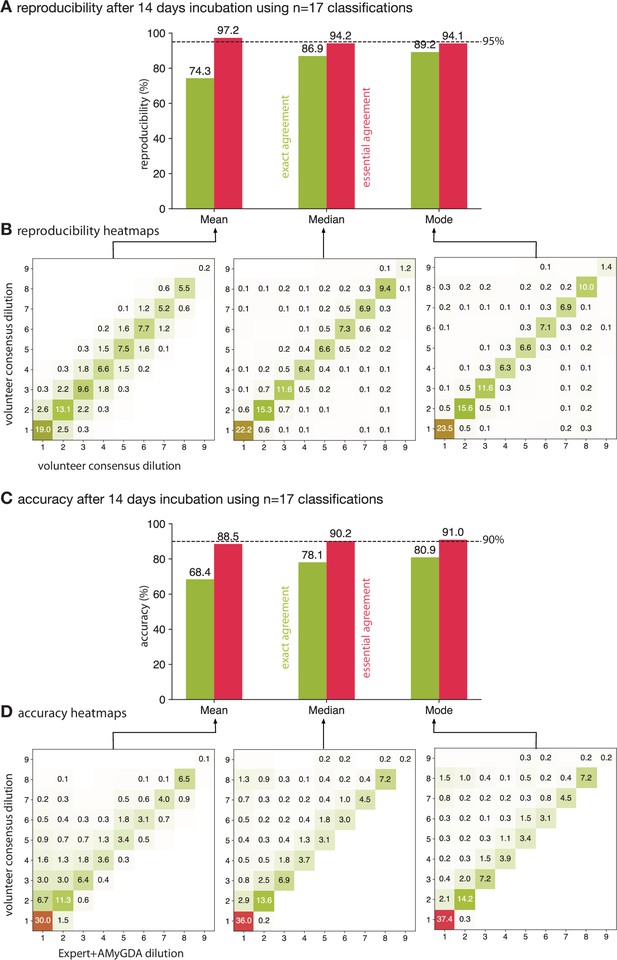
Taking the mean of 17 classifications is ≥95% reproducible whilst applying either the median or mode is ≥90% accurate.
(A) Only calculating the mean of 17 classifications achieves an essential agreement ≥95% for reproducibility International Standards Organization, 2007, followed by the median and the mode. (B) Heatmaps of the consensus formed via the mean, median or mode after 14 days incubation. Only drug images from the Expert + AMyGDA dataset are included. (C) The essential agreement between a consensus dilution formed from 17 classifications using the median or mode and the consensus Expert +AMyGDA dilution both exceed the required 90% threshold International Standards Organization, 2007. (D) The heatmaps clearly show how the volunteer consensus dilution is likely to be the same or greater than the Expert + AMyGDA consensus.
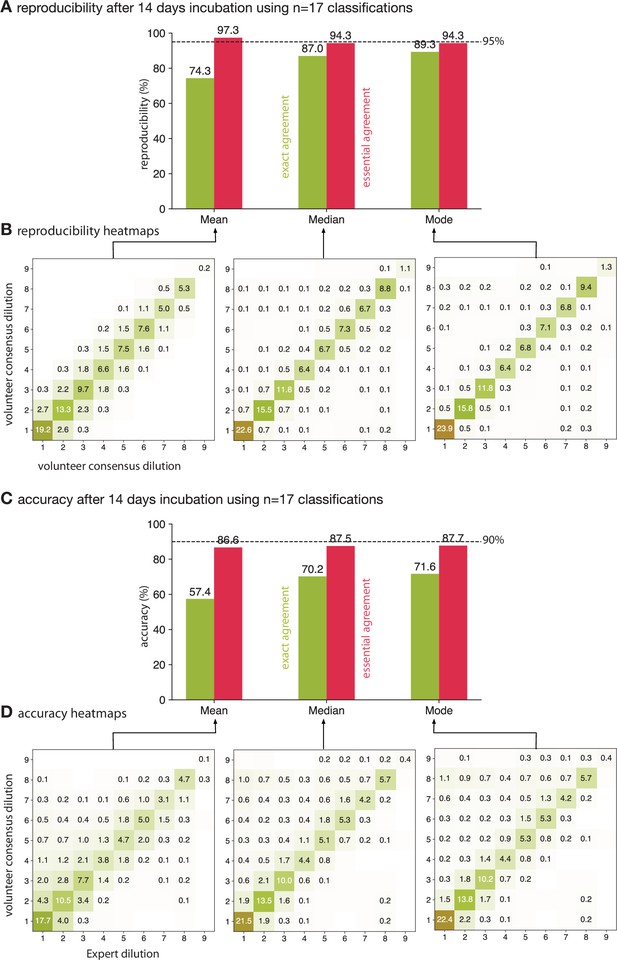
Taking the mean of 17 classifications is ≥95% reproducible whilst none of the methods reach have an essential agreement for accuracy of ≥90% when using the Expert dataset.
(A) Only calculating the mean of 17 classifications achieves an essential agreement ≥95% for reproducibility International Standards Organization, 2007, followed by the median and then the mode. There is no specified threshold for exact agreement; the trend is reversed with the mode performing best, followed by the median and then the mean. (B) Heatmaps of the consensus formed via the mean, median, or mode after 14 days incubation. Each consensus dilution is a different selection, with replacement, of the original classifications. Drug images from the larger Expert dataset are included. (C) The essential agreement between a consensus dilution formed from 17 classifications using the median or mode and the consensus Expert dilution is ≥ 90%, which is the required threshold International Standards Organization, 2007. (D) The heatmaps clearly show how the volunteer consensus dilution is likely to be the same or greater than the Expert consensus.
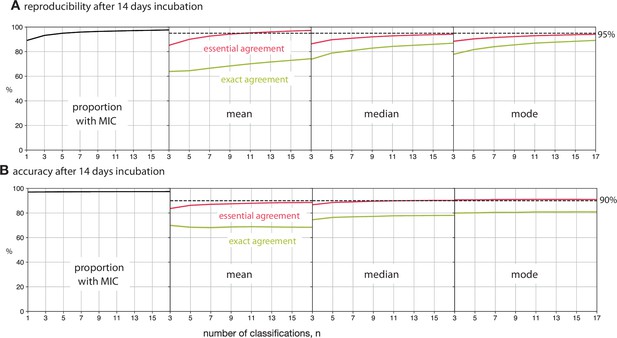
Reducing the number of classifications, , used to build the consensus dilution decreases the reproducibility and accuracy of the consensus measurement.
(A) The consensus dilution becomes less reproducible as the number of classifications is reduced, as measured by both the exact and essential agreements. (B) Likewise, the consensus dilution becomes less accurate as the number of classifications is decreased, however the highest level of exact agreement using the mean is obtained when and the mode, and to a lesser extent the median, are relatively insensitive to the number of classifications. These data are all with respect to the Expert +AMyGDA dataset.
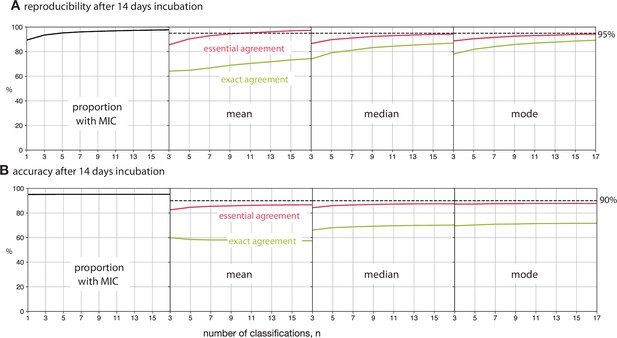
Reducing the number of classifications, , used to build the consensus dilution decreases the reproducibility and accuracy of the consensus measurement.
(A) The consensus dilution becomes less reproducible as the number of classifications is reduced, as measured by both the exact and essential agreements. (B) Likewise, the consensus dilution becomes less accurate as the number of classifications is decreased, however the highest level of exact agreement using the mean is obtained when and the mode, and to a lesser extent the median, are relatively insensitive to the number of classifications. These data are all with respect to the Expert dataset.
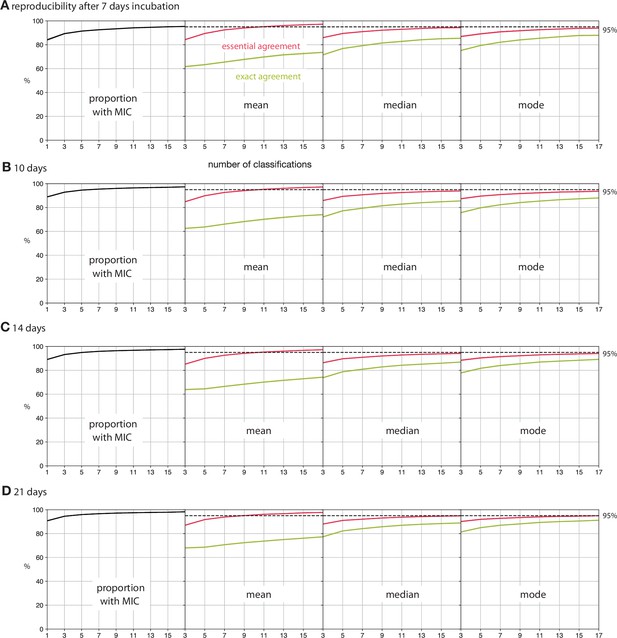
Altering the number of days incubation does not markedly affect the observed trends in reproducibility.
Shown are results for the Expert +AMyGDA dataset after (A) 7, (B) 10, (C) 14 and (D) 21 days of incubation. A previous study (Rancoita et al., 2018) showed that optimal results were achieved after 14 days incubation.
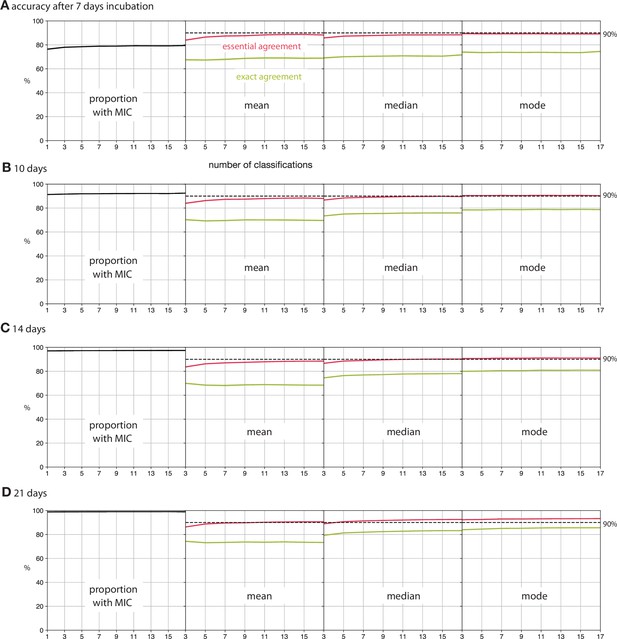
Altering the number of days incubation does not markedly affect the observed trends in accuracy.
Shown are results for the Expert +AMyGDA dataset after (A) 7, (B) 10, (C) 14 and (D) 21 days of incubation. A previous study (Rancoita et al., 2018) showed that optimal results were achieved after 14 days incubation.
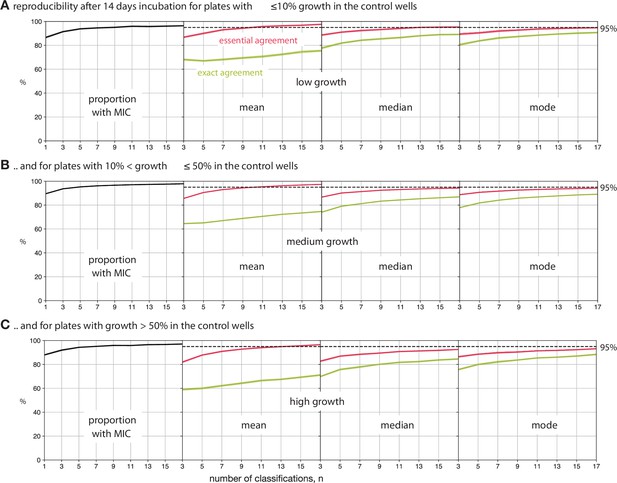
Segmenting the drug images by the mean amount of growth in the positive control wells (Figure 6—figure supplement 3) does not markedly affect the reproducibility of the three consensus methods.
The plates are split into those with (A) low (≤ 10 %) growth, (B) medium (10 < growth ≤) growth and (C) high (> 50 %) growth. The drug images from the Expert +AMyGDA dataset were used and the proportion with MIC is the proportion of consensus readings that are a definite numerical minimum inhibitory concentration.
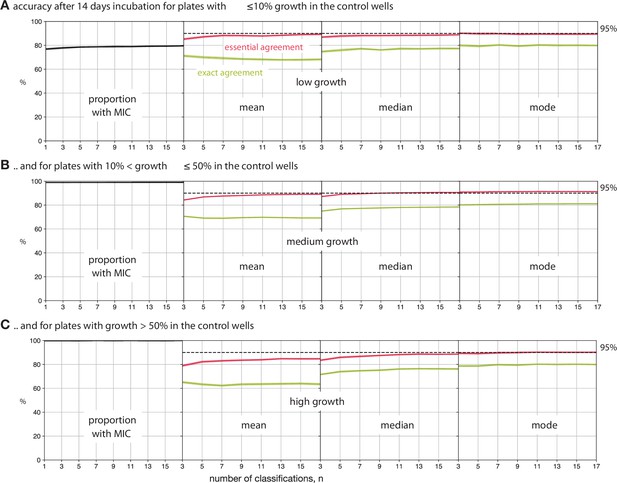
Segmenting the drug images by the mean amount of growth in the positive control wells (Figure 6—figure supplement 3) does not markedly affect the accuracy of the three consensus methods.
The plates are split into those with (A) low (≤ 10% %) growth, (B) medium (10 < growth ≤ 50 %) growth and (C) high (> 50 %) growth. The drug images from the Expert +AMyGDA dataset were used and the proportion with MIC is the proportion of consensus readings that are a definite numerical minimum inhibitory concentration.
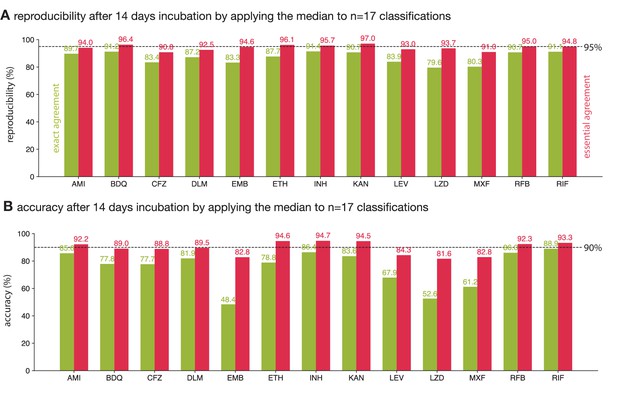
The reproducibility and accuracy of the consensus MICs varies by drug.
Consensus MICs were arrived at by taking the median of 17 classifications after 14 days incubation. The essential and exact agreements are drawn as red and green bars, respectively. For the former the minimum thresholds required are 95% and 90% for the reproducibility and accuracy, respectively (International Standards Organization, 2007). See (Figure 5—figure supplement 1) for the other consensus methods.
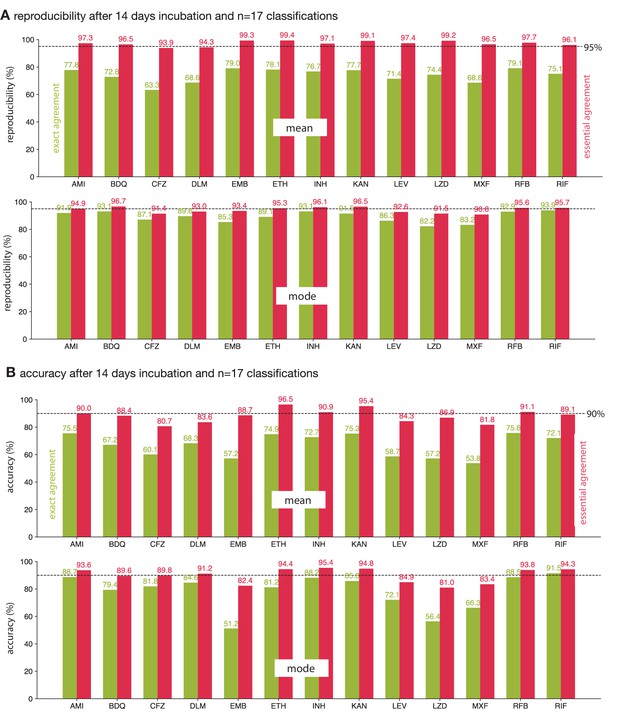
The reproducibility and accuracy after 14 days incubation of the 13 antibiotics on the UKMYC5 plate.
A total of 17 classifications were used for each measurement and either the mean or mode was used to obtain a consensus reading of the (A) reproducibility and (B) accuracy. The essential agreement is drawn in red and the required thresholds are 95% and 90% for the reproducibility and accuracy, respectively (International Standards Organization, 2007). The exact agreement is drawn in green and no threshold is defined. The drug abbreviations are defined in (Figure 6—figure supplement 1). The dataset used was Expert +AMyGDA.
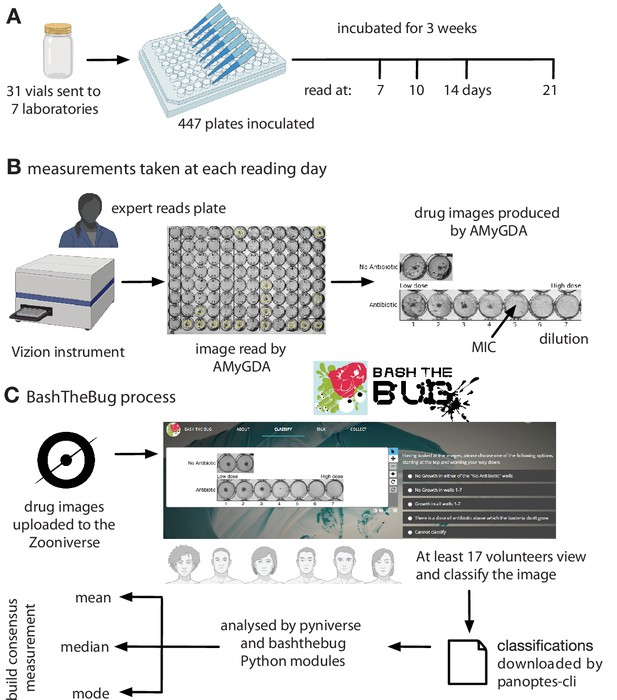
Each UKMYC5 plate was read by an Expert, by some software (AMyGDA) and by at least 17 citizen scientist volunteers via the BashTheBug project.
(A) 447 UKMYC5 plates were prepared and read after 7, 10, 14 and 21 days incubation. (B) The minimum inhibitory concentrations (MIC) for the 14 drugs on each plate were read by an by Expert, using a Vizion instrument. The Vizion also took a photograph which was subsequently analysed by AMyGDA – this software then composited 14 drug images from each photograph, each containing an image of the two positive control wells. To allow data from different drugs to be aggregated, all MICs were converted to dilutions. (C) All drug images were then uploaded to the Zooniverse platform before being shown to volunteers through their web browser. Images were retired once they had been classified by 17 different volunteers. Classification data were downloaded and processed using two Python modules (pyniverse +bashthebug) before consensus measurements being built using different methods.
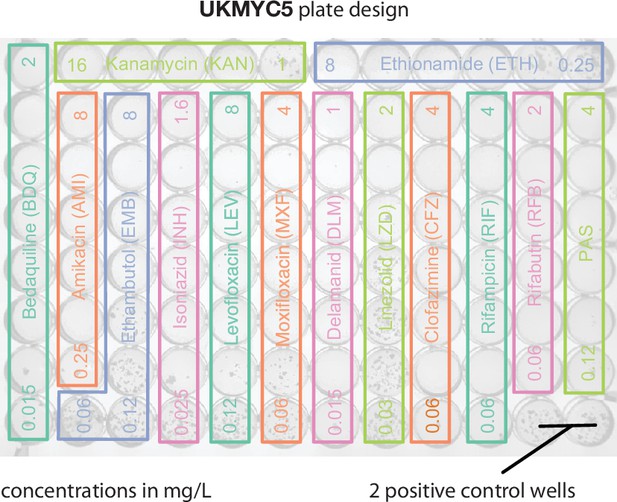
The UKMYC5 plate contains 14 different anti-TB drugs.
A previous study (Rancoita et al., 2018) showed that para-aminosalicylic acid (PAS) performed poorly and it has been removed from the subsequent UKMYC6 plate design. We have therefore excluded this drug from all analyses. Each drug was contained in 5, 6, 7, or 8 wells with each well having double the concentration of drug as the one before. The concentration of the first and last well in each drug series is labelled (mg/L). Two wells contain no drug and are therefore positive control wells.
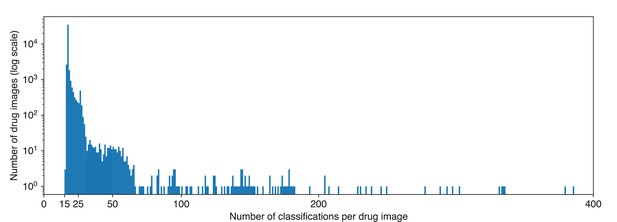
Although the retirement limit within the Zooniverse platform was set to 17, over 1800 images received more classifications than this and a small number were only classified 15 or 16 times.
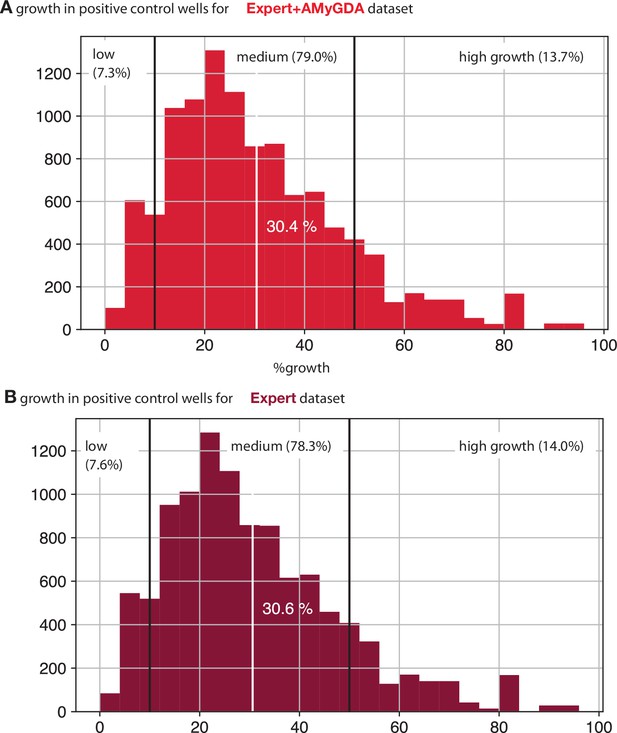
The Expert +AMyGDA consensus dataset has the same distribution of bacterial growth in the positive control wells as the Expert dataset after 14 days incubation.
(A) The distribution of the mean positive control well growth, as measured by AMyGDA, for the Expert +AMyGDA dataset. The dataset is arbitrarily split into three categories: low (<10%), medium (10 ≤ growth < 50 %) and high (≥ 50 %) growth. The proportions of the dataset in each category are labelled. (B) The distribution of the mean positive control well growth, as measured by AMyGDA, for the Expert dataset. There are around twice as many plates in this dataset (Supplementary file 1c).
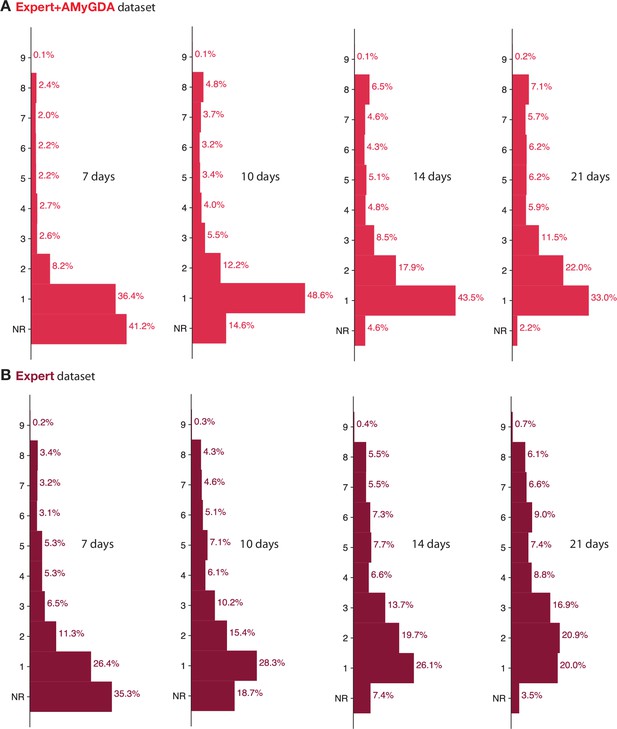
The Expert +AMyGDA dataset has a greater proportion of drug images with low dilutions compared to the Expert dataset.
The growth of the bacteria is also evident as the number of days the sample was incubated for is increased.
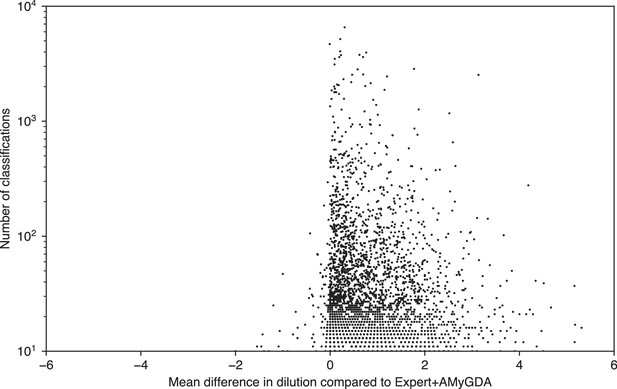
The average bias per volunteer decreases with experience.
The average bias per volunteer, as defined by the difference between a volunteer’s reading and that from the Expert +AMyGDA dataset, is plotted against the total number of classifications done by each volunteer. Only volunteers who have done 10 or more classifications are plotted.
Additional files
-
MDAR checklist
- https://cdn.elifesciences.org/articles/75046/elife-75046-mdarchecklist1-v3.pdf
-
Supplementary file 1
A supplementary file containing a tables (a-i) is available online.
The majority of the tables in the supplemental file can also be reproduced using the accompanying jupyter notebook at https://github.com/fowler-lab/bashthebug-consensus-dataset; Fowler Lab, 2022.
- https://cdn.elifesciences.org/articles/75046/elife-75046-supp1-v3.tex