Neuroscout, a unified platform for generalizable and reproducible fMRI research
Figures
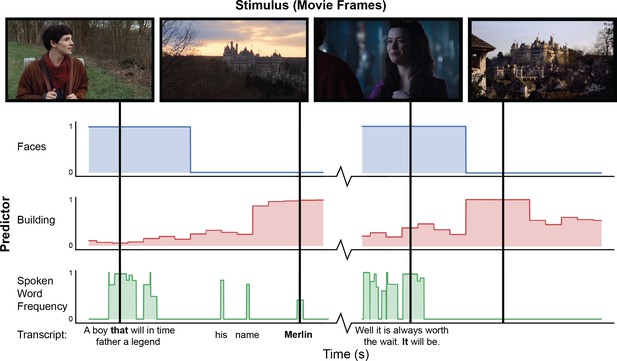
Example of automated feature extraction on stimuli from the “Merlin” dataset.
Visual features were extracted from video stimuli at a frequency of 1 Hz. ‘Faces’: we applied a well-validated cascaded convolutional network trained to detect the presence of faces (Zhang et al., 2016). ‘Building’: We used Clarifai’s General Image Recognition model to compute the probability of the presence of buildings in each frame. ‘Spoken word frequency’ codes for the lexical frequency of words in the transcript, as determined by the SubtlexUS database (Brysbaert and New, 2009). Language features are extracted using speech transcripts with precise word-by-word timing determined through forced alignment.
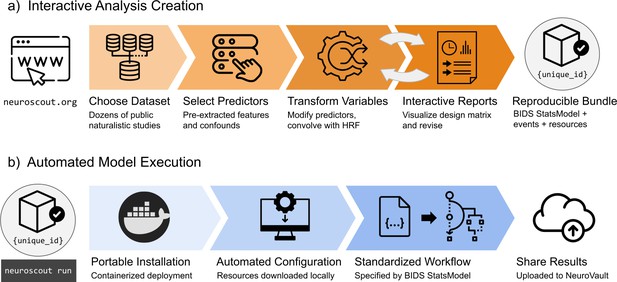
Overview schematic of analysis creation and model execution.
(a) Interactive analysis creation is made possible through an easy-to-use web application, resulting in a fully specified reproducible analysis bundle. (b) Automated model execution is achieved with little-to-no configuration through a containerized model fitting workflow. Results are automatically made available in NeuroVault, a public repository for statistical maps.
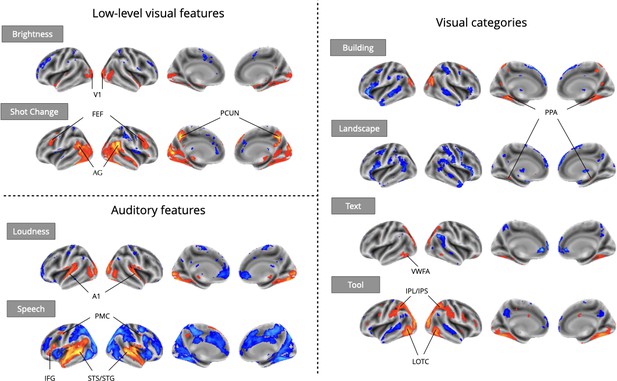
Meta-analytic statistical maps for GLM models targeting a variety of effects with strong priors from fMRI research.
Individual GLM models were fit for each effect of interest, and dataset level estimates were combined using image-based meta-analysis. Images were thresholded at Z=3.29 (P<0.001) voxel-wise. Abbreviations: V1=primary visual cortex; FEF = frontal eye fields; AG = angular gyrus; PCUN = precuneus; A1=primary auditory cortex; PMC = premotor cortex; IFG = inferior frontal gyrus; STS = superior temporal sulcus; STG = superior temporal gyrus; PPA = parahippocampal place area; VWFA = visual word-form area; IPL = inferior parietal lobule; IPS = inferior parietal sulcus; LOTC = lateral occipito-temporal cortex.
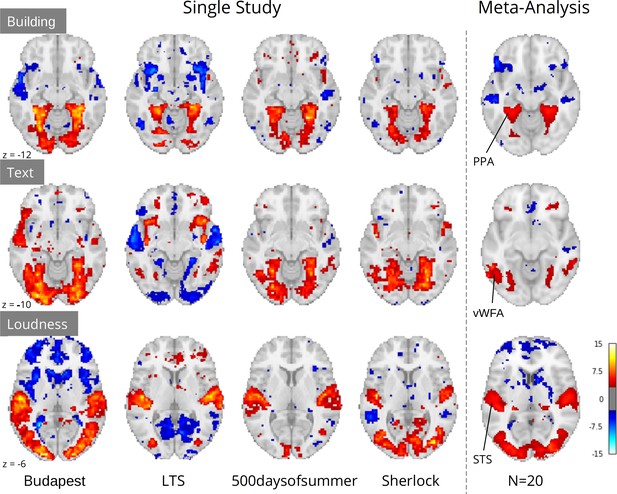
Comparison of a sample of four single study results with meta-analysis (N=20) for three features: ‘building’ and ‘text’ extracted through Clarifai visual scene detection models, and sound ‘loudness’ (root mean squared of the auditory signal).
Images were thresholded at Z=3.29 (p<0.001) voxel-wise. Regions with a priori association with each predictor are highlighted: PPA, parahippocampal place area; VWFA, visual word form area; STS, superior temporal sulcus. Datasets: Budapest, Learning Temporal Structure (LTS), 500daysofsummer task from Naturalistic Neuroimaging Database, and Sherlock.
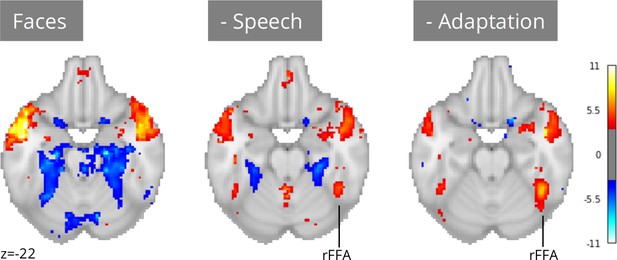
Meta-analysis of face perception with iterative addition of covariates.
Left; Only including binary predictors coding for the presence of faces on screen did not reveal activity in the right fusiform face area (rFFA). Middle; Controlling for speech removed spurious activations and revealed rFFA association with face presentation. Right; Controlling for temporal adaptation to face identity in addition to speech further strengthened the association between rFFA and face presentation. N=17 datasets; images were thresholded at Z=3.29 (p<0.001) voxel-wise.
Tables
Neuroscout datasets included in the validation analyses.
Subj is the number of unique subjects. Scan Time is the mean scan time per subject (in minutes). AV = Audio-Visual; AN = Audio Narrative.
Name | Subj | DOI/URI | Scan time | Modality | Description |
---|---|---|---|---|---|
Study Forrest (Hanke et al., 2014) | 13 | 10.18112/openneuro.ds000113.v1.3.0 | 120 | AV | Slightly abridged German version of the movie: ‘Forrest Gump’ |
Life (Nastase et al., 2018) | 19 | datasets.datalad.org/?dir=/labs/haxby/life | 62.8 | AV | Four segments of the Life nature documentary |
Raiders (Haxby et al., 2011) | 11 | datasets.datalad.org/?dir=/labs/haxby/raiders | 113.3 | AV | Full movie: ‘Raiders of the Lost Ark’ |
Learning Temporal Structure (LTS) (Aly et al., 2018) | 30 | 10.18112/openneuro.ds001545.v1.1.1 | 20.1 | AV | Three clips from the movie ‘Grand Budapest Hotel’, presented six times each. Some clips were scrambled. |
Sherlock (Chen et al., 2017) | 16 | 10.18112/openneuro.ds001132.v1.0.0 | 23.7 | AV | The first half of the first episode from ‘Sherlock’ TV series. |
SherlockMerlin (Zadbood et al., 2017) | 18 | Temporarily unavailable | 25.1 | AV | Full episode from ‘Merlin’ TV series. Only used Merlin task to avoid analyzing the Sherlock task twice. |
Schematic Narrative (Baldassano et al., 2018) | 31 | 10.18112/openneuro.ds001510.v2.0.2 | 50.4 | AV/AN | 16 three-minute clips, including audiovisual clips and narration. |
ParanoiaStory (Finn et al., 2018) | 22 | 10.18112/openneuro.ds001338.v1.0.0 | 21.8 | AN | Audio narrative designed to elicit individual variation in suspicion/paranoia. |
Budapest (Visconti et al., 2020) | 25 | 10.18112/openneuro.ds003017.v1.0.3 | 50.9 | AV | The majority of the movie ‘Grand Budapest Hotel’, presented in intact order |
Naturalistic Neuroimaging Database (NNDb) (Aliko et al., 2020) | 86 | 10.18112/openneuro.ds002837.v2.0.0 | 112.03 | AV | Movie watching of 10 full-length movies |
Narratives (Nastase et al., 2021) | 328 | 10.18112/openneuro.ds002345.v1.1.4 | 32.5 | AN | Passive listening of 16 audio narratives (two tasks were not analyzed due to preprocessing error) |
Extractor name, feature name, and description for all Neuroscout features used in the validation analyses.
Extractor | Feature | Description |
---|---|---|
Brightness | brightness | Average luminosity across all pixels in each video frame. |
Clarifai | building, landscape, text, tool | Indicators of the probability that an object belonging to each of these categories is present in the video frame. |
FaceNet | any_faces, log_mean_time_cum | For each video frame, any_faces indicates the probability that the image displays at least one face. log_mean_time_cum indicates the cumulative time (in seconds) a given face has been on screen up since the beginning of the movie. If multiple faces are present, their cumulative time on screen is averaged. |
Google Video Intelligence | shot_change | Binary indicator coding for shot changes. |
FAVE/Rev | speech | Binary indicator coding for the presence of speech in the audio signal, inferred from word onsets/offsets information from force-aligned speech transcripts. |
RMS | rms | Root mean square (RMS) energy of the audio signal. |
Lexical norms | Log10WF, concreteness, phonlev, numsylls, numphones, duration, text_length | Logarithm of SubtlexUS lexical frequency, concreteness rating, phonological Levenshtein distance, number of syllables, number of phones, average auditory duration and number of characters for each word in the speech transcript. These metrics are extracted from lexical databases available through pliers. |