Artificial selection methods from evolutionary computing show promise for directed evolution of microbes
Figures
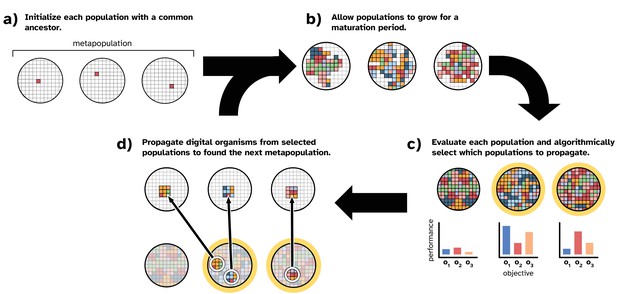
Overview of our model of directed microbial evolution.
In (a), we found each of populations with a single digital organism. In this figure, the metapopulation comprises three populations. Next (b), each population undergoes a maturation period during which digital organisms compete for space, reproduce, mutate, and evolve. After maturation, (c) we evaluate each population based on one or more population-level characteristics, and we select populations (repeat selections allowed) to partition into ‘offspring’ populations. In this figure, we show populations being evaluated on three objectives (o1, o2, and o3). In this work, population-level objectives include the ability to compute different mathematical expressions (see Table 1). We see this as analogous to a microbial population’s ability to produce different biomolecules or to metabolize different resources. After evaluation, populations are chosen algorithmically using one of the selection protocols described in ‘Methods.’
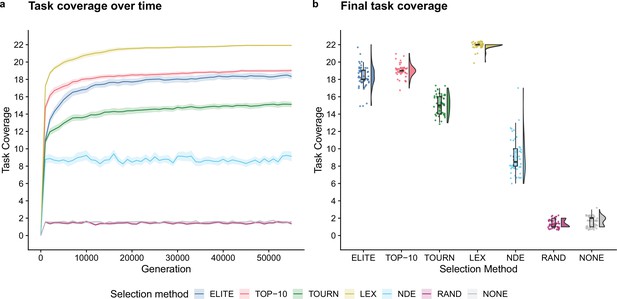
Task coverage of the best program (per replicate) evolved in an evolutionary computing context over time (a) and at the end of the experiment (b).
Selection scheme abbreviations are as follows: TOURN, tournament; LEX, lexicase; NDE, non-dominated elite; RAND, random; NONE, no selection. In panel (a), each line gives the mean value across 50 replicates, and the shading around each mean gives a bootstrapped 95% confidence interval. Differences in final task coverage among treatments were statistically significant (Kruskal–Wallis, ).
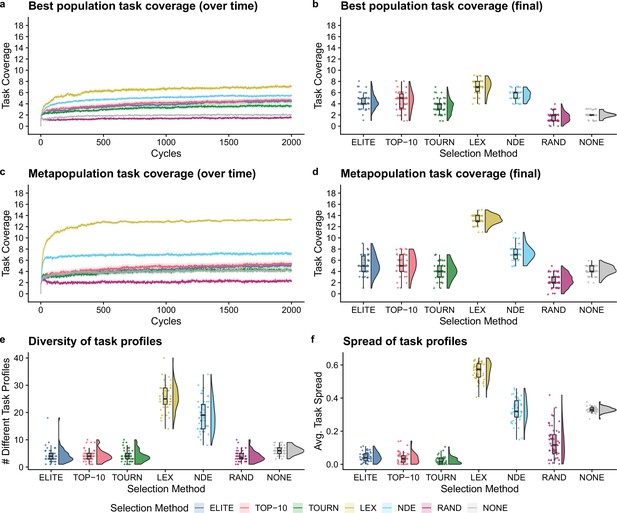
Digital directed evolution results.
For panels (a) and (c), each line gives the mean value across 50 replicates, and the shading around each mean gives a bootstrapped 95% confidence interval. At the end of the experiment, differences among treatments were statistically significant for each of (b) best population task coverage, (d) metapopulation task coverage, (e) task profile diversity, and (f) task profile spread (Kruskal–Wallis, ).
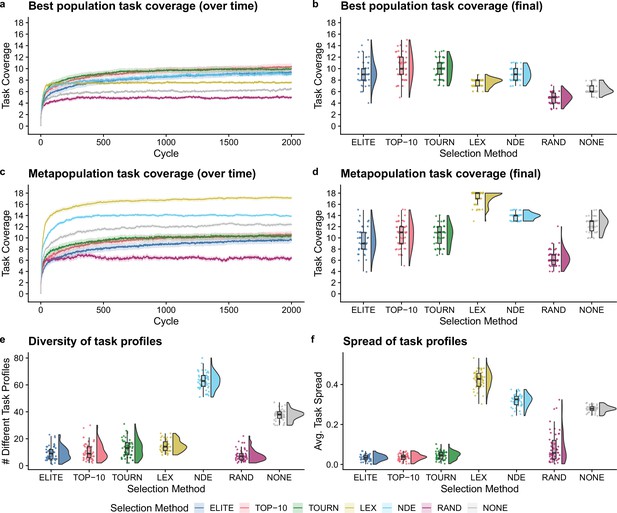
Digital directed evolution results when organism survival is tied to population-level functions.
For panels (a) and (c), each line gives the mean value across 50 replicates, and the shading around each mean gives a bootstrapped 95% confidence interval. At the end of the experiment, differences among treatments were statistically significant for each of (b) best population task coverage, (d) metapopulation task coverage, (e) task profile diversity, and (f) task profile spread (Kruskal-Wallis, ).
Tables
Computational functions that conferred individual-level or population-level benefits.
The particular functions were chosen to be used in our model based on those used in the Avida system (Bryson et al., 2021). In all experiments, we included two versions of ECHO (each for different input values), resulting in 22 possible functions that organisms could perform. In general, functions that confer population-level benefits are more complex (i.e., require more instructions to perform) than functions designated to confer individual-level benefits.
Function | # Inputs | Benefit |
---|---|---|
ECHO | 1 | Individual |
NAND | 2 | Individual |
NOT | 1 | Population |
ORNOT | 2 | Population |
AND | 2 | Population |
OR | 2 | Population |
ANDNOT | 2 | Population |
NOR | 2 | Population |
XOR | 2 | Population |
EQU | 2 | Population |
1 | Individual | |
1 | Population | |
1 | Population | |
2 | Population | |
2 | Population | |
2 | Population | |
2 | Population | |
2 | Population | |
2 | Population | |
2 | Population | |
2 | Population |