Fermentation: Teaming up to make kombucha
From cheese to salami, to beer or miso soup, chances are that your favorite delicacy owes its unique flavors to humble communities of microorganisms which ferment sugars into substances that preserve and improve food (Bourdichon et al., 2021). Humans have been enthusiastically brewing or pickling since the Bronze Age, yet surprisingly little is known about the intricacies of the fermentation process (Farag et al., 2019; Yang et al., 2014).
Fermenting food requires dozens if not hundreds of microbial species which work closely together, each producing substances which the others take up, use and transform into new chemicals important for other species in the community (Tamang et al., 2016). These complex interactions make it challenging to disentangle how individual actors contribute to the overall process, and to identify the ones essential for the final product. Now, in eLife, Xiaoning Huang, Yongping Xin and Ting Lu report having methodically reduced the complex microbial system which creates the tangy drink known as kombucha tea, down to a single pair of species (Huang et al., 2022).
Kombucha is created by a thriving community of yeast and bacteria which work together to ferment sugary tea. Huang et al. first focused on the features that this culture must have to produce the famous concoction. Three key characteristics emerged: both yeast and bacteria should be present; a characteristic jelly-like film or ‘pellicle’ should form at the surface; and the culture should consume sucrose while accumulating acetate, ethanol, and small amounts of sucrose constituents such as glucose. Preserving these features ensured that a core community of microbes would capture the essential metabolism of the native culture found in kombucha (Figure 1; Step 1).
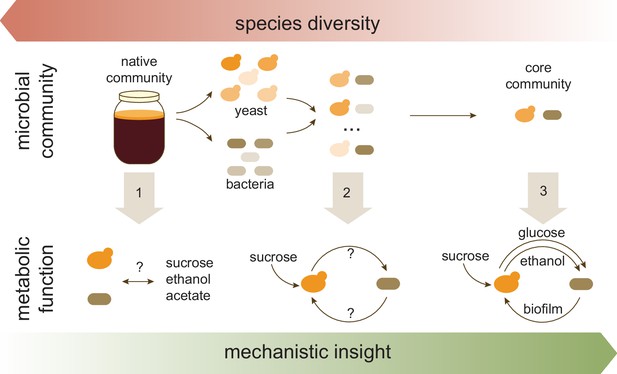
Approach used by Huang et al. to investigate the role of specific members of the microbial community found in kombucha tea.
The species richness of the kombucha microbial community was systematically reduced (top), with each step (gray arrows) improving the understanding of the metabolic function of core species in the culture (bottom). Step 1: Analyzing the native kombucha community revealed the identity and relative abundance of its various microbial members; gross metabolic changes were also recorded (such as consumption of sucrose and production of ethanol and acetate), but they were unassigned to any microorganisms. Step 2: Isolating five yeast and five bacterial species and analyzing their twenty-five pairwise combination cultures revealed that the bacteria depended on yeast degrading sucrose. Step 3: In-depth analysis of a representative yeast-bacteria pair revealed the specific interactions underlying their collaboration (cross-feeding of glucose and ethanol from yeast to bacteria, and bacterial production of biofilm which potentially protects the community).
Next, the team (who are based at the University of Illinois Urbana-Champaign and the China Agricultural University) isolated five yeast and five bacterial species, examining each of them individually or as yeast-bacteria pairs. Some bacteria completely depended on yeast to break down sucrose into glucose and into other essential molecules required for their survival (Figure 1; Step 2). Although all yeast species could survive on their own, the distinctive properties of kombucha (such as its pellicle, high acidity and acetate production) occurred only in co-cultures, indicating that bacteria did contribute to these community functions.
To understand how the community worked at an even finer scale, Huang et al. focused on a single yeast-bacteria pair which could create all three features characteristic of native kombucha. This co-culture was remarkably stable: no matter the ratio of yeast to bacteria at the start of the process, the final communities had roughly equal numbers of each species once stable. They also all produced concoctions which closely resembled traditional kombucha, with similar levels of acidity, sugars, ethanol, and acetate.
Next, these two species were individually cultured on diverse nutrient sources to closely monitor which compounds they could consume and produce (Figure 1; Step 3). The manipulation revealed that only the yeast could make glucose and ethanol; this likely involves the cells secreting an enzyme that processes sucrose into glucose, which is then available for ‘public use’ (Tran et al., 2020; Smith and Schuster, 2019). In turn, the bacteria could only create a pellicle when they consumed glucose and ethanol at the same time. This experiment helped to finally piece together how the two species interact: yeast feed and stimulate bacteria with glucose and ethanol, while bacteria wrap the community in a film that may shield it from the environment (Yin et al., 2019).
If two species alone can thrive and produce kombucha-like tea, then why does this process normally involve many more microorganisms? This taxonomic diversity may improve adaptability (Willi et al., 2006), or it may just emerge through random processes (Sloan et al., 2006); it could even be an artefact due to sampling at an inadequately large scale (Fierer and Lennon, 2011). Further studies are needed to investigate these possibilities.
The reductionist approach developed by Huang et al. allows scientists to pinpoint the core subgroups of microbes which perform the primary functions of a wider community, and to disentangle the role of individual species. This framework is useful to understand the metabolic processes responsible for the signature look, taste and smell of fermented foods. The next steps would potentially involve finetuning the method to study microbial communities which are harder to define, such as those that interact with host organisms or the wider environment.
References
-
The forgotten role of food culturesFEMS Microbiology Letters 368:fnab085.https://doi.org/10.1093/femsle/fnab085
-
The generation and maintenance of diversity in microbial communitiesAmerican Journal of Botany 98:439–448.https://doi.org/10.3732/ajb.1000498
-
Quantifying the roles of immigration and chance in shaping prokaryote community structureEnvironmental Microbiology 8:732–740.https://doi.org/10.1111/j.1462-2920.2005.00956.x
-
Public goods and cheating in microbesCurrent Biology 29:R442–R447.https://doi.org/10.1016/j.cub.2019.03.001
-
Review: Diversity of microorganisms in global fermented foods and beveragesFrontiers in Microbiology 7:377.https://doi.org/10.3389/fmicb.2016.00377
-
Limits to the adaptive potential of small populationsAnnual Review of Ecology, Evolution, and Systematics 37:433–458.https://doi.org/10.1146/annurev.ecolsys.37.091305.110145
-
Proteomics evidence for kefir dairy in early Bronze Age ChinaJournal of Archaeological Science 45:178–186.https://doi.org/10.1016/j.jas.2014.02.005
-
Biofilms: the microbial “protective clothing” in extreme environmentsInternational Journal of Molecular Sciences 20:E3423.https://doi.org/10.3390/ijms20143423
Article and author information
Author details
Publication history
Copyright
© 2022, Ponomarova
This article is distributed under the terms of the Creative Commons Attribution License, which permits unrestricted use and redistribution provided that the original author and source are credited.
Metrics
-
- 2,448
- views
-
- 175
- downloads
-
- 0
- citations
Views, downloads and citations are aggregated across all versions of this paper published by eLife.
Download links
Downloads (link to download the article as PDF)
Open citations (links to open the citations from this article in various online reference manager services)
Cite this article (links to download the citations from this article in formats compatible with various reference manager tools)
Further reading
-
- Computational and Systems Biology
- Genetics and Genomics
Untranslated regions (UTRs) contain crucial regulatory elements for RNA stability, translation and localization, so their integrity is indispensable for gene expression. Approximately 3.7% of genetic variants associated with diseases occur in UTRs, yet a comprehensive understanding of UTR variant functions remains limited due to inefficient experimental and computational assessment methods. To systematically evaluate the effects of UTR variants on RNA stability, we established a massively parallel reporter assay on 6555 UTR variants reported in human disease databases. We examined the RNA degradation patterns mediated by the UTR library in two cell lines, and then applied LASSO regression to model the influential regulators of RNA stability. We found that UA dinucleotides and UA-rich motifs are the most prominent destabilizing element. Gain of UA dinucleotide outlined mutant UTRs with reduced stability. Studies on endogenous transcripts indicate that high UA-dinucleotide ratios in UTRs promote RNA degradation. Conversely, elevated GC content and protein binding on UA dinucleotides protect high-UA RNA from degradation. Further analysis reveals polarized roles of UA-dinucleotide-binding proteins in RNA protection and degradation. Furthermore, the UA-dinucleotide ratio of both UTRs is a common characteristic of genes in innate immune response pathways, implying a coordinated stability regulation through UTRs at the transcriptomic level. We also demonstrate that stability-altering UTRs are associated with changes in biobank-based health indices, underscoring the importance of precise UTR regulation for wellness. Our study highlights the importance of RNA stability regulation through UTR primary sequences, paving the way for further exploration of their implications in gene networks and precision medicine.
-
- Computational and Systems Biology
- Medicine
Excessive consumption of sucrose, in the form of sugar-sweetened beverages, has been implicated in the pathogenesis of metabolic dysfunction‐associated fatty liver disease (MAFLD) and other related metabolic syndromes. The c-Jun N-terminal kinase (JNK) pathway plays a crucial role in response to dietary stressors, and it was demonstrated that the inhibition of the JNK pathway could potentially be used in the treatment of MAFLD. However, the intricate mechanisms underlying these interventions remain incompletely understood given their multifaceted effects across multiple tissues. In this study, we challenged rats with sucrose-sweetened water and investigated the potential effects of JNK inhibition by employing network analysis based on the transcriptome profiling obtained from hepatic and extrahepatic tissues, including visceral white adipose tissue, skeletal muscle, and brain. Our data demonstrate that JNK inhibition by JNK-IN-5A effectively reduces the circulating triglyceride accumulation and inflammation in rats subjected to sucrose consumption. Coexpression analysis and genome-scale metabolic modeling reveal that sucrose overconsumption primarily induces transcriptional dysfunction related to fatty acid and oxidative metabolism in the liver and adipose tissues, which are largely rectified after JNK inhibition at a clinically relevant dose. Skeletal muscle exhibited minimal transcriptional changes to sucrose overconsumption but underwent substantial metabolic adaptation following the JNK inhibition. Overall, our data provides novel insights into the molecular basis by which JNK inhibition exerts its metabolic effect in the metabolically active tissues. Furthermore, our findings underpin the critical role of extrahepatic metabolism in the development of diet-induced steatosis, offering valuable guidance for future studies focused on JNK-targeting for effective treatment of MAFLD.