Prediction of diabetic kidney disease risk using machine learning models: A population-based cohort study of Asian adults
Figures
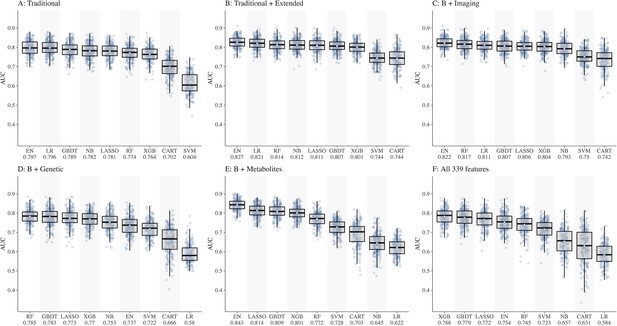
Comparison of nine machine learning models for diabetic kidney disease (DKD) incidence prediction using different sets of features (Panel A-F).
Abbreviations: CART, classification and regression tree; EN, elastic net; GBDT, gradient boosting decision tree; LASSO, least absolute shrinkage and selection operator; LR, logistic regression; NB, naïve Bayes; RF, random forest; SVM, support vector machine; XGB, extreme gradient boosting.
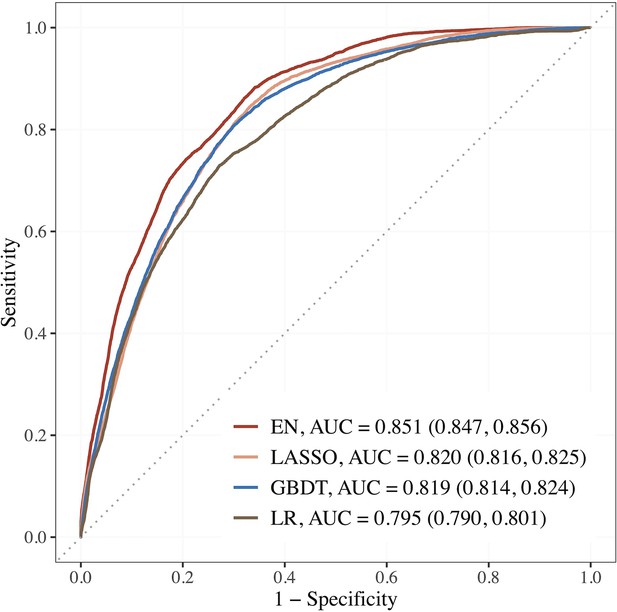
Performance of the top 3 machine learning (ML) models based on selected variables in dataset E (risk factors + blood metabolites) compared to LR using seven established features.
Abbreviations: EN, Elastic net; GBDT, gradient boosting decision tree; LASSO; least absolute shrinkage and selection operator; LR, logistic regression.

Association of the top 15 machine learning (ML)-selected predictors with incident diabetic kidney disease (DKD).
Abbreviations: LASSO, least absolute shrinkage and selection operator; GBDT, Gradient boosting decision tree.
Variables: anti-HTN Meds, anti hypertensive medications; DM, diabetes mellitus; GFR-EPI, glomerular filtration rate estimated using CKD-EPI equation; HDL, high-density lipoprotein.
Metabolites: DHA: 22:6, docosahexaenoic acid; DHAFA, Ratio of 22:6 docosahexaenoic acid to total fatty acids; IDL-CE%, Cholesterol esters to total lipids ratio in IDL; M-HDL-PL%, Phospholipids to total lipids ratio in medium HDL; M-VLDL-PL%, Phospholipids to total lipids ratio in medium VLDL; S-HDL-FC% Free cholesterol to total lipids ratio in small HDL; XL-HDL-CE%, Cholesterol esters to total lipids ratio in very large HDL; L-LDL-CE%, Cholesterol esters to total lipids ratio in large LDL; M-HDL-PL%, Phospholipids to total lipids ratio in medium HDL; IDL-C% Total cholesterol to total lipids ratio in IDL; M-HDL-PL% Phospholipids to total lipids ratio in medium HDL.
Tables
Baseline characteristics of SEED diabetic participants by incident DKD status.
Characteristics | No DKD(n = 1203) | DKD(n = 162) | p-value | Overall(n = 1365) |
---|---|---|---|---|
Age (years) | 57.95 (8.78) | 64.63 (7.98) | <0.001 | 58.74 (8.95) |
Sex, female | 580 (48.2) | 87 (53.7) | 0.219 | 667 (48.9) |
Ethnicity | <0.001 | |||
Indians (ref) | 599 (49.8) | 49 (30.2) | 648 (47.5) | |
Malays | 310 (25.8) | 70 (43.2) | 380 (27.8) | |
Chinese | 294 (24.4) | 43 (26.5) | 337 (24.7) | |
Primary/below education (%) | 706 (58.7) | 121 (74.7) | <0.001 | 827 (60.6) |
Current smoker (%) | 173 (14.4) | 16 (9.9) | 0.15 | 189 (13.9) |
Alcohol consumption (%) | 111 (9.2) | 11 (6.8) | 0.389 | 122 (9.0) |
Hypertension (%) | 845 (70.4) | 155 (95.7) | <0.001 | 1000 (73.4) |
Diabetic retinopathy (%) | 228 (19.2) | 56 (35.4) | <0.001 | 284 (21.1) |
Cardiovascular disease (%) | 153 (12.7) | 32 (19.8) | 0.02 | 185 (13.6) |
Duration of diabetes (years) | 2.68 [0.00, 8.56] | 6.08 [1.44, 11.63] | <0.001 | 3.20 [0.00, 9.37] |
Antidiabetic medication (%) | 681 (56.6) | 122 (75.3) | <0.001 | 803 (58.8) |
Insulin use (%) | 39 (3.3) | 11 (7.1) | 0.036 | 50 (3.8) |
Body mass index (kg/m2) | 26.96 (4.62) | 27.05 (4.36) | 0.764 | 26.97 (4.59) |
Systolic blood pressure (mm Hg) | 139.42 (18.95) | 155.24 (20.01) | <0.001 | 141.29 (19.74) |
Diastolic blood pressure (mm Hg) | 78.25 (9.74) | 79.14 (10.70) | 0.278 | 78.35 (9.85) |
Random blood glucose (mmol/L) | 9.53 (4.26) | 10.44 (5.01) | 0.052 | 9.64 (4.36) |
HbA1c (%) | 7.61 (1.58) | 8.04 (1.83) | 0.003 | 7.66 (1.62) |
Blood total cholesterol (mmol/L) | 5.14 (1.14) | 4.98 (1.15) | 0.124 | 5.12 (1.15) |
Blood HDL cholesterol (mmol/L) | 1.12 (0.31) | 1.16 (0.35) | 0.178 | 1.12 (0.32) |
eGFR (mL/min/1.73 m2) | 89.98 (14.34) | 79.40 (11.69) | <0.001 | 88.72 (14.46) |
-
Values for categorical variables are presented as number (percentages); values for continuous variables are given as mean (SD) or median [IQR]. p-values are given by χ2 test or Mann–Whitney U test as appropriate for the variable.
-
DKD, diabetic kidney disease; HDL, high-density lipoprotein cholesterol; IQR, interquartile range; SD, standard deviation; SEED, Singapore Epidemiology of Eye Diseases.
Machine learning model for predicting incident CKD in literature.
Author, journal | Study cohort,country | Study populationFollow-up | CKD definition and incidence | Number of predictors | ML performance |
---|---|---|---|---|---|
Ravizza et al., 2019, Nature Medicine | EHR data from the IBM Explorys and INPC datasets, the United States | Development cohort (IBM): >500,000 adults with diabetes. Validation (INPC) = 82,912 adults with T2DM; FU = 3 y. | ICD 9/10 codes | 300 features | Based on seven prioritized features, AUC by RF = 0.833 and the Roche/IBM supervised algorithm by LR = 0.827 |
Song et al., 2020., JMIR | EHR data, the United States (2007–2017) | 14,039 adults with T2DM. FU = 1 y. | eGFR < 60 or UACR ≥30 mg/g; 34.1% | >3000 | GBM AUC = 0.83 |
Huang et al., 2020a., Diabetes | KORA cohort, Germany | 1838 adults with prediabetes and T2DM. FU = 6.5 y. | eGFR < 60 or UACR ≥30 mg/g at FU; 10.9% | 125 mets + 14 clinical factors | SVM, RF, Ada Boost Best set: Mets-SM and PC + age, TC, FPG, eGFR, UACR, AUC = 0.857 Traditional LR using 14 variables, AUC = 0.809 |
Sabanayagam et al., 2023, current study | SEED population data, Singapore | 1365 adults with diabetes. FU = 6 y. | eGFR < 60 + 25% decline in eGFR from baseline | 339 features | EN + RFE selected 15 features, AUC = 0.851 vs. 0.795 using seven features by traditional LR |
-
AUC, area under the receiver operating characteristic curve; CKD, chronic kidney disease; eGFR, estimated glomerular filtration rate; EHR, electronic health records; EN, Elastic Net; FPG, Fasting plasma glucose; FU, follow-up; GBM, Gradient Boosting Machine; ICD, International Classification of Diseases; INPC, Indiana Network for Patient Care; LR, logistic regression; ML, machine learning; RF, random forest; RFE, recursive feature selection; SEED, Singapore Epidemiology of Eye Diseases; SVM, support vector machine; T2DM, type 2 diabetes mellitus; TC, total cholesterol; UACR, urine albumin-creatinine ratio.
Additional files
-
Supplementary file 1
Description of variables in each dataset and results from supplementary analyses (Table 1a- 1g).
(Table 1a) Characteristics of the preprocessed datasets A–F. (Table 1b) List of variables used for DKD prediction. (Table 1c) Median AUC [IQR] performance of the ML models using datasets A–F. (Table 1d) Median SN%/SP% performance of the ML models using datasets A–F. (Table 1e) Source data linked to Figure 3. (Table 1f) Top ML-selected predictors for incident DKD in each of the three ethnic groups by EN and RFE. (Table 1g) Baseline characteristics of SEED diabetic participants by ethnicity (n = 1365).
- https://cdn.elifesciences.org/articles/81878/elife-81878-supp1-v2.docx
-
MDAR checklist
- https://cdn.elifesciences.org/articles/81878/elife-81878-mdarchecklist1-v2.pdf