Larger but younger fish when growth outpaces mortality in heated ecosystem
Abstract
Ectotherms are predicted to ‘shrink’ with global warming, in line with general growth models and the temperature-size rule (TSR), both predicting smaller adult sizes with warming. However, they also predict faster juvenile growth rates and thus larger size-at-age of young organisms. Hence, the result of warming on the size-structure of a population depends on the interplay between how mortality rate, juvenile- and adult growth rates are affected by warming. Here, we use two-decade long time series of biological samples from a unique enclosed bay heated by cooling water from a nearby nuclear power plant to become 5–10 °C warmer than its reference area. We used growth-increment biochronologies (12,658 reconstructed length-at-age estimates from 2426 individuals) to quantify how >20 years of warming has affected body growth, size-at-age, and catch to quantify mortality rates and population size- and age structure of Eurasian perch (Perca fluviatilis). In the heated area, growth rates were faster for all sizes, and hence size-at-age was larger for all ages, compared to the reference area. While mortality rates were also higher (lowering mean age by 0.4 years), the faster growth rates lead to a 2 cm larger mean size in the heated area. Differences in the size-spectrum exponent (describing how the abundance declines with size) were less clear statistically. Our analyses reveal that mortality, in addition to plastic growth and size-responses, is a key factor determining the size structure of populations exposed to warming. Understanding the mechanisms by which warming affects the size- and the age structure of populations is critical for predicting the impacts of climate change on ecological functions, interactions, and dynamics.
Editor's evaluation
This work provides convincing evidence to refute a general tenet in biology, that warming induces smaller maximum body sizes in adult ectoterm individuals. Using a semi-natural experiment in an exceptional man-made ecosystem, the authors demostrate that fish in waters warmed by a nearby nuclear plant grew faster but died younger, causing little effect on the size distribution of fish in the area. This work will be of interest to ecologists and physiologists interested in the impacts of global warming on natural communities.
https://doi.org/10.7554/eLife.82996.sa0Introduction
Ectotherm species, constituting 99% of species globally (Atkinson and Sibly, 1997; Wilson, 1992), are commonly predicted to shrink in a warming world (Gardner et al., 2011; Sheridan and Bickford, 2011). However, as the size distribution of many species spans several orders of magnitude, and temperature effects on size may depend on size or age, it is important to be specific about which sizes- or life stages are predicted to shrink (usually mean or adult is meant). For instance, warming can shift size distributions without altering mean size if increases in juvenile size-at-age outweigh the decline in size-at-age in adults, which is consistent with the temperature size rule, TSR (Atkinson, 1994). Resolving how warming induces changes in population’s size distribution may thus be more instructive (Fritschie and Olden, 2016), especially for inferring warming effects on species’ ecological role, biomass production, or energy fluxes (Gårdmark and Huss, 2020; Yvon-durocher et al., 2011). This is because key processes such as metabolism, feeding, growth, and mortality scale with body size (Andersen and Link, 2020; Blanchard et al., 2017; Brown et al., 2004; Pauly, 1980; Thorson et al., 2017; Ursin, 1967). Hence, as the value of these traits at mean body size is not the same as the mean population trait value (Bernhardt et al., 2018), the size distribution within a population matters for its dynamics and for how it changes under warming.
The population size distribution can be represented as a size-spectrum, which generally is the frequency distribution of individual body sizes (Edwards et al., 2017). It is often described in terms of the size-spectrum slope (slope of individuals or biomass of a size class over the mean size of that class on a log-log scale [Edwards et al., 2017; Sheldon et al., 1973; White et al., 2007]) or simply the exponent of the power law individual size distribution (Edwards et al., 2017). The size-spectrum thus results from temperature-dependent ecological processes such as body growth, mortality, and recruitment (Blanchard et al., 2017; Heneghan et al., 2019). Despite its rich theoretical foundation (Andersen, 2019) and usefulness as an ecological indicator (Blanchard et al., 2005), few studies have evaluated warming effects on the species size-spectrum in larger-bodied species (but see Blanchard et al., 2005), and none in large scale experimental set-ups. There are numerous paths by which a species’ size-spectrum could change with warming (Heneghan et al., 2019). For instance, in line with TSR predictions, warming may lead to a smaller size-spectrum exponents (steeper slope) if the maximum size declines. However, changes in size-at-age and the relative abundances of juveniles and adults may alter this decline in the size-spectrum slope. Warming can also lead to elevated mortality (Barnett et al., 2020; Berggren et al., 2022; Biro et al., 2007; Pauly, 1980), partly because a faster pace of life with higher metabolic rates is associated with a shorter lifespan (Brown et al., 2004; Munch and Salinas, 2009) or due to direct lethal effects of extreme temperature events. This truncates the age distribution towards younger individuals (Barnett et al., 2017), which may reduce density dependence and potentially increase growth rates, thus countering the effects of mortality on the size-spectrum exponent. However, not all sizes may benefit from warming, as e.g. the optimum temperature for growth declines with size (Lindmark et al., 2022). Hence, the effect of warming on the size-spectrum depends on several interlinked processes affecting abundance-at-size and size-at-age.
Size-at-age is generally predicted to increase with warming for small individuals, but decrease for large individuals according to the mentioned TSR (Atkinson, 1994; Ohlberger, 2013). Several factors likely contribute to this pattern, such as increased allocation to reproduction (Wootton et al., 2022) and larger individuals in fish populations having optimum growth rates at lower temperatures (Lindmark et al., 2022). Empirical support in fishes for this pattern seems to be more consistent for increases in size-at-age of juveniles (Huss et al., 2019; Rindorf et al., 2008; Thresher et al., 2007) than declines in adult size-at-age (but see Baudron et al., 2014; Oke et al., 2022; Smoliński et al., 2020), for which a larger diversity in responses is observed among species (Barneche et al., 2019; e.g., Huss et al., 2019). However, most studies have been done on commercially exploited species, since long-time series are more common in such species. This may confound or interact with the effects of temperature because fishing mortality can affect density-dependent growth (van Gemert et al., 2018), but also select for slow-growing individuals and changes in maturation processes, which also influences growth trajectories (Audzijonyte et al., 2016).
The effect of temperature on mortality rates of wild populations is often studied using among-species analyses (Pauly, 1980; Thorson et al., 2017). These relationships based on thermal gradients in space may not necessarily be the same as the effects of warming on mortality in single populations. Hence, the effects of warming on growth and size-at-age, and mortality within natural populations constitute a key knowledge gap for predicting the consequences of climate change on population size spectra.
Here, we used data from a unique, large-scale 23-year-long heating experiment of a coastal ecosystem to quantify how warming changed fish body growth, mortality, and the size structure in an unexploited population of Eurasian perch (Perca fluviatilis, ‘perch’). We compare fish from this enclosed bay exposed to temperatures approximately 5–10 °C above normal (‘heated area’) with fish from a reference area in the adjacent archipelago (Figure 1). Using hierarchical Bayesian models, we quantify differences in key individual- and population-level parameters, such as body growth, asymptotic size, mortality rates, and size spectra, between the heated and reference coastal areas.
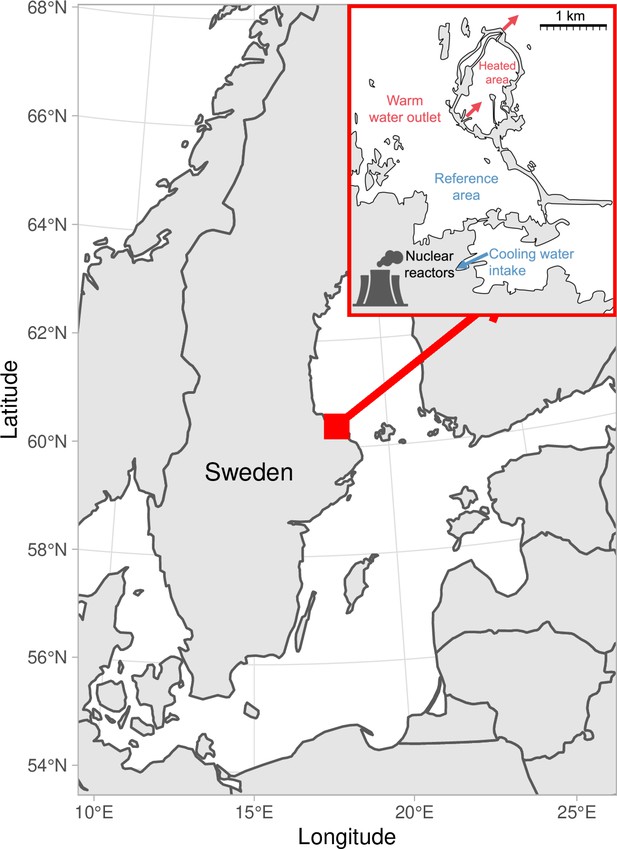
Map of the area with the unique whole-ecosystem warming experiment from which perch in this study was sampled.
Inset shows the 1 km2 enclosed coastal bay that has been artificially heated for 23 years, the adjacent reference area with natural temperatures, and locations of the cooling water intake, and where the heated water outlet from nuclear power plants enters the heated coastal basin. The arrows indicate the direction of water flow.
Results
Analysis of perch size-at-age using the von Bertalanffy growth equation (VBGE) revealed that fish cohorts (year classes) in the heated area both grew faster initially (larger size-at-age) and reached larger predicted asymptotic sizes than those in the unheated reference area (Figure 2). The model with area-specific VBGE parameters ( , , and ) had the best out-of-sample predictive accuracy (the largest expected log pointwise predictive density for a new observation; Supplementary file 1a). Models where both and were shared did not converge (Supplementary file 1a). Both the estimated values for fish asymptotic length () and growth coefficient () were larger in the heated compared to the reference area (Figure 2—figure supplement 8). We estimated that the asymptotic length of fish in the heated area was 16% larger than in the reference area (calculated as ) (, , where the point estimate is the posterior median and values in brackets correspond to the 95% credible interval). The growth coefficient was 27% larger in the heated area (, ). These differences in growth parameters lead to fish being approximately 7%–11% larger in the heated area at any age relative to the reference area (Figure 2—figure supplement 4). Due to the last three cohorts (1995–1997) having large estimates of and low (potentially due to their negative correlation and because of the young age with data far from the asymptote, Figure 2—figure supplements 3 and 5–6), we fit the same model with these cohorts omitted to evaluate the importance of those for the predicted difference between the areas. Without these, the predicted difference in size-at-age was still clear, but smaller (between 4%–7%, Figure 2—figure supplements 9–10).
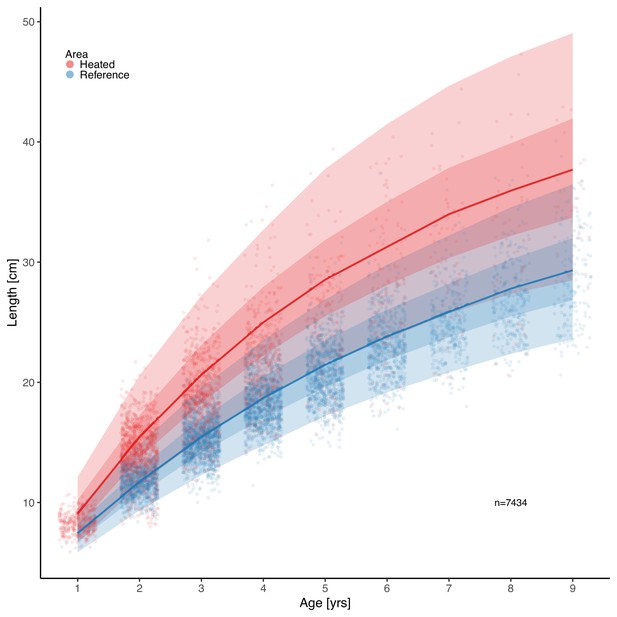
Fish grow faster and reach larger sizes in the heated enclosed bay (red) compared to the reference area (blue).
Points depict individual-level length-at-age and lines show the median of the posterior draws of the global posterior predictive distribution (without group-level effects), both exponentiated, from the von Bertalanffy growth model with area-specific coefficients. The shaded areas correspond to 50% and 90% credible intervals.
In addition, we found that growth rates in the reference area were both slower and declined faster with size compared to the heated area (Figure 3). The best model for growth () had area-specific and parameters (Supplementary file 1b). Initial growth () was estimated to be 18% faster in the heated than in the reference area (, ), and the growth of fish in the heated area declines more slowly with length than in the reference area (, ). The distribution of differences of the posterior samples for α and only had 0.3% and 0.2% of the density below 0, respectively (Figure 3C and E), indicating a high probability that length-based growth rates are faster in the heated area.
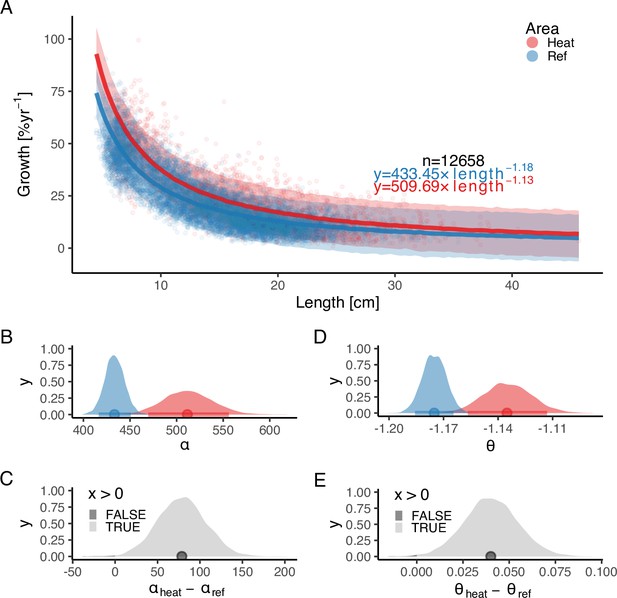
The faster growth rates in the heated area (red) compared to the reference (blue) are maintained as fish grow in size.
The points illustrate specific growth rate estimated from back-calculated length-at-age (within individuals) as a function of length, expressed as the geometric mean of the length at the start and end of the time interval. Lines show the median of the posterior draws of the global posterior predictive distribution (without group-level effects) from the allometric growth model with area-specific coefficients. The shaded areas correspond to the 90% credible interval. The equation uses mean parameter estimates. Panel (B) shows the posterior distributions for initial growth ( (red) and (blue)), and (C) the distribution of their difference. Panel (D) shows the posterior distributions for the allometric exponent ( and ), and (E) the distribution of their difference. The fill color depicts the area below 0 (0.3% and 0.2% for and , respectively).
By analyzing the decline in catch-per-unit-effort over age, we found that the instantaneous mortality rate (the rate at which log abundance declines with age) is higher in the heated area (Figure 4). was estimated as a fixed effect, as the model where only intercepts varied among years had the best out-of-sample predictive ability. The overlap with zero is 0.07% for the distribution of differences between posterior samples of and (Figure 4C). We estimated to be 0.73 [0.66,0.79] and to be 0.62 [0.58,0.67], which corresponds to annual mortality rates (calculated as ) of 52% in the heated area and 46% in the reference area.
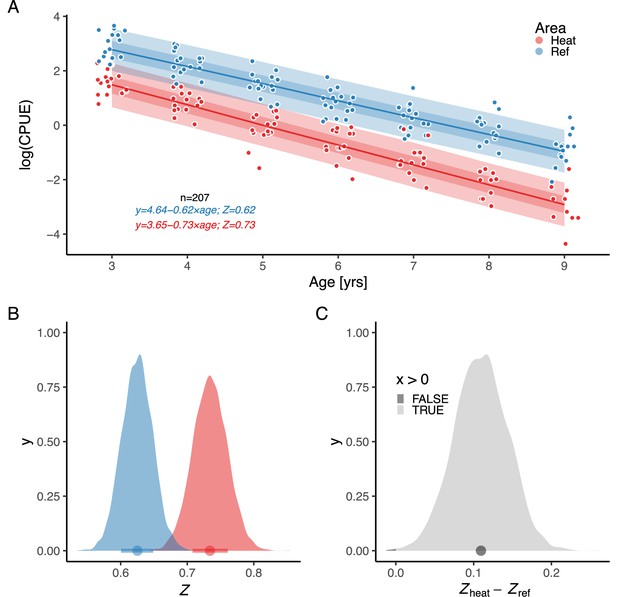
The instantaneous mortality rate () is higher in the heated area (red) than in the reference (blue).
Panel (A) shows as a function of , where the slope corresponds to . Lines show the median of the posterior draws of the global posterior predictive distribution (without group-level effects) and the shaded areas correspond to the 50% and 90% credible intervals. The equation shows mean parameter estimates. Panel (B) shows the posterior distributions for mortality rate ( and ), and (C) the distribution of their difference, where the fill color depicts the area below 0 (0.07%).
Lastly, analysis of the size- and age-structure in the two areas revealed that, despite the faster growth rates, higher mortality, and larger maximum sizes in the heated area Figure 5A, the size-spectrum exponents were largely similar Figure 5B, C. In fact, the size-spectrum exponent was only slightly larger in the heated area (Figure 5B), and their 95% confidence intervals largely overlap. However, results from the lognormal model fitted to the size- and age-distributions revealed that the average size was two centimeters longer and the average age 0.4 years younger in the heated compared to the reference area (Figure 6).
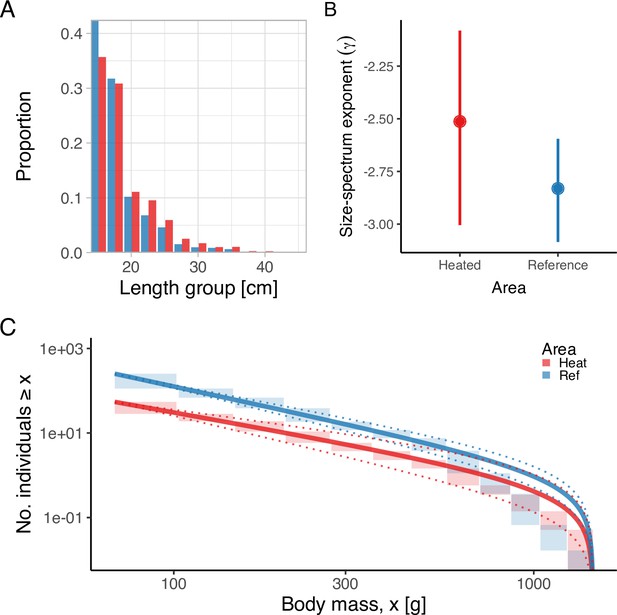
The heated area (red) has a larger proportion of large fish than the reference area (blue), illustrated both in terms of histograms of proportions at size (A) and the biomass size-spectrum (B, C), but the difference in the slope of the size spectra between the areas is not statistically clear (C).
Panel (A) illustrates histograms of length groups in the heated and reference area as proportions (for all years pooled). Panel (B) shows the estimate of the size-spectrum exponent, , where vertical lines depict the 95% confidence interval. Panel (C) shows the size distribution and MLEbins fit (red and blue solid curves for the heated and reference area, respectively) with 95% confidence intervals indicated by dotted lines. The vertical span of rectangles illustrates the possible range of the number of individuals with body mass ≥ the body mass of individuals in that bin.
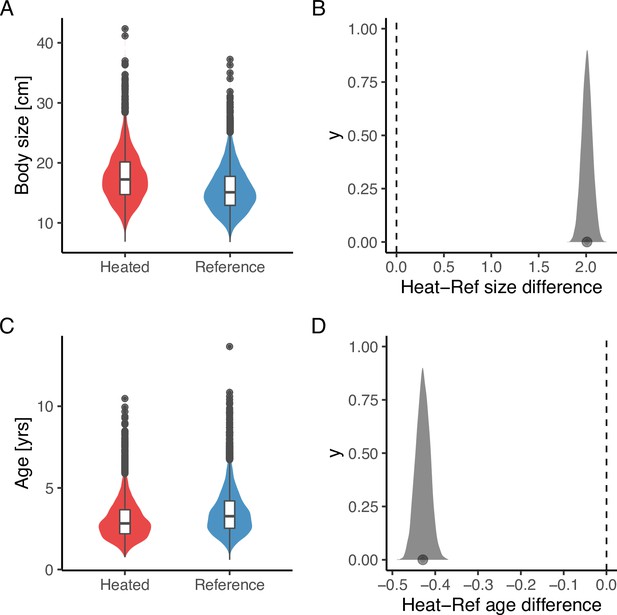
The average size is larger (A, B), but the average age (C, D) is younger in the heated area compared to the reference area.
The violin plots (A, C) are based on draws from the global posterior predictive distribution (without group-level effects) for mean size and age from the lognormal model, respectively, with the random year effect omitted, while the density plots (B, D) depict the difference between areas based on draws from the expected value of the posterior predictive distribution. Hence, the latter has a smaller variation and the difference in means is more pronounced. The average size is 2 cm larger in the heated area, and the average age is 0.4 years younger (B, D).
Discussion
Our study provides strong evidence for warming-induced differentiation in growth and mortality in a natural population of an unexploited, temperate fish species exposed to an ecosystem-scale experiment with 5–10°C above normal temperatures for more than two decades. Interestingly, these effects largely, but not completely, counteract each other when it comes to population size-structure—while the fish are younger, they are also larger on average. However, differences in the rate of decline in abundance with size are less pronounced between the areas. It is difficult to generalize these findings since it is a study of only a single species. It is, however, a unique climate change experiment, as experimental studies on fish to date are much shorter and often on scales much smaller than whole ecosystems, and long-time series of biological samples exist mainly for commercially exploited fish species (Baudron et al., 2014; Smoliński et al., 2020; Thresher et al., 2007) (in which fisheries exploitation affects size-structure both directly and indirectly by selecting for fast-growing individuals). While factors other than temperature could have contributed to the observed elevated growth and mortality, the temperature contrast is unusually large for natural systems (i.e. 5–10°C, which can be compared to the 1.35°C change in the Baltic Sea between 1982 and 2006 [Belkin, 2009]). Moreover, heating occurred at the scale of a whole ecosystem, which makes the findings highly relevant in the context of global warming.
Interestingly, our findings contrast with both broader predictions about declining mean or adult body sizes based on the GOLT hypothesis (Cheung et al., 2013; Pauly, 2021), and with intraspecific patterns such as the TSR (temperature-size rule, Atkinson, 1994). The contrasts lie in that both asymptotic size and size-at-age of mature individuals, as well as the proportion of larger individuals, were slightly larger and higher in the heated area—despite the elevated mortality rates. This result was unexpected for two reasons: optimum growth temperatures generally decline with body size within species under food satiation in experimental studies (Lindmark et al., 2022), and fish tend to mature at smaller body sizes and allocate more energy into reproduction as it gets warmer (Niu et al., 2023; Wootton et al., 2022). Both patterns have been used to explain how growth can increase for small and young fish, while large and old fish typically do not benefit from warming. Our study species is no exception to these rules (Huss et al., 2019; Karås and Thoresson, 1992; Niu et al., 2023; Sandstrom et al., 1995). This suggests that growth dynamics under food satiation may not be directly proportional to those under natural feeding conditions (Railsback, 2022). It could also mean that while temperatures is near optimum for growth in the warmest months of the year for a 15 cm individual (and above optimum for larger fish as the optimum declines with size) (Huss et al., 2019; Lindmark et al., 2022), the exposure to such high temperatures is not enough to cause strong reductions in growth and eventually size-at-age. Our results highlight that we need to focus on understanding to what extent the commonly observed increase in size-at-age for juveniles in warm environments can be maintained as they grow older.
Our finding that mortality rates were higher in the heated area was expected—warming leads to faster metabolic rates (faster ‘pace of life’), which in turn is associated with a shorter life span (Brown et al., 2004; McCoy and Gillooly, 2008; Munch and Salinas, 2009). Extreme temperatures, which may be more common in warmed systems under natural variability, can also be lethal if e.g., acute oxygen demands cannot be met (Sandblom et al., 2016). Warming may further increase predation mortality, as predators’ feeding rates increase in order to meet the higher demand for food (Biro et al., 2007; Pauly, 1980; Ursin, 1967). However, most evidence to date of the temperature dependence of mortality rates in natural populations stems from across-species studies (Gislason et al., 2010; Pauly, 1980; Thorson et al., 2017, but see Berggren et al., 2022; Biro et al., 2007). Across-species relationships are not necessarily determined by the same processes as within-species relationships; thus, our finding of warming-induced mortality in a heated vs control environment in two nearby con-specific populations is important.
Since a key question for understanding the implications of warming on ectotherm populations is if larger individuals in a population become rarer or smaller (Ohlberger, 2013; Ohlberger et al., 2018), within-species mortality and growth responses to warming need to be further studied. Importantly, this requires accounting also for the effects of warming on growth, and how responses in growth and mortality depend on each other. For instance, higher mortality (predation or natural, physiological mortality) can release intra-specific competition and thus increase growth. While e.g., benthic invertebrate density was not affected by the initial warming of the heated area (Sandstrom et al., 1995), warming-induced mortality may have led to higher benthic prey availability per capita for the studied perch. Conversely, altered growth and body sizes can lead to changes in size-specific mortality, such as predation or starvation, both of which are expected to change with warming (Thunell, 2023). In conclusion, individual-level patterns such as the TSR can only be used to predict changes in the population-level size structure in limited cases, as it does not concern changes in abundance-at-size via mortality. Mortality may, however, be an important driver of the observed shrinking of ectotherms (Peralta-Maraver and Rezende, 2021). Understanding the mechanisms by which the size- and age-distribution change with warming is critical for predicting how warming changes species functions and ecological roles (Audzijonyte et al., 2020; Fritschie and Olden, 2016; Gårdmark and Huss, 2020). Our findings demonstrate that a key to do this is to acknowledge temperature effects on both growth and mortality and how they interact.
Materials and methods
Data
We use size-at-age data from perch sampled annually from an artificially heated enclosed bay (‘the Biotest basin’) and its reference area, both in the western Baltic Sea (Figure 1). Heating started in 1980, the first analyzed cohort is 1981, and the first and last catch year is 1987 and 2003, respectively, to omit transient dynamics and acute responses, and to ensure we use cohorts that only experienced one of the thermal environments during its life. A grid at the outlet of the heated area (Figure 1) prevented fish larger than 10 cm from migrating between the areas (Adill et al., 2013; Huss et al., 2019), and genetic studies confirm the reproductive isolation between the two populations during this time period (Björklund et al., 2015). However, the grid was removed in 2004. Since then, fish growing up in the heated Biotest basin can easily swim out and fish caught in the reference area can no longer be assumed to be born there. Hence, we use data only up until 2003. This resulted in 12,658 length-at-age measurements from 2,426 individuals (i.e. multiple measurements per individual) from 256 net deployments.
We use data from fishing events using survey-gillnets that took place in October in the heated Biotest basin and in August in the reference area when temperatures are most comparable between the two areas (Huss et al., 2019), because temperature affects catchability in static gears. The catch was recorded by 2.5 cm length classes during 1987–2000, and into 1 cm length groups in years 2001–2003. To express lengths in a common length standard, 1 cm intervals were converted into 2.5 cm intervals. The unit of catch data is hence the number of fish caught by 2.5 cm size class per net per night (i.e. a catch-per-unit-effort (CPUE) variable). All data from fishing events with disturbance affecting the catch (e.g. seal damage, strong algal growth on the gears, clogging by drifting algae) were removed (years 1996 and 1999 from the heated area in the catch data).
Length-at-age throughout an individuals' life was reconstructed for a random or length-stratified subset of caught individuals each year (depending on which year, and in some cases, the number of fish caught). This was done using growth-increment biochronologies derived from annuli rings on the operculum bones, with control counts done on otoliths. Such analyses have become increasingly used to analyze changes in the growth and size-at-age of fishes (Essington et al., 2022; Morrongiello and Thresher, 2015). Specifically, an established power-law relationship between the distance of annual rings and fish length was used: , where is the length of the fish, the operculum radius, the intercept, and the slope of the line for the regression of log-fish length on log-operculum radius from a large reference data set for perch (Thoresson, 1996). Back-calculated length-at-age was obtained from the relationship , where is the back-calculated body length at age , is the final body length (body length at catch), is the distance from the center to the annual ring corresponding to age and for perch (Thoresson, 1996). Since perch exhibits sexual size-dimorphism, and age determination together with back-calculation of growth was not done for males in all years, we only used females for our analyses.
Statistical analysis
Request a detailed protocolThe differences in size-at-age, growth, mortality, and size structure between perch in the heated and the reference area were quantified using hierarchical linear and non-linear models fitted in a Bayesian framework. First, we describe each statistical model and then provide details of model fitting, model diagnostics, and comparison.
To describe individual growth throughout life, we fit the von Bertalanffy growth equation (VBGE) (Beverton and Holt, 1957; von Bertalanffy, 1938) on a scale, describing length as a function of age to evaluate differences in size-at-age and asymptotic size: , where is the length-at-age (years), is the asymptotic size, is the Brody growth coefficient () and is the age when the average length was zero. Here and henceforth, log refers to natural logarithms. We used only age and size at catch, i.e. not back-calculated length-at-age. This was to have a simpler model and not have to account for parameters varying within individuals as well as cohorts, as mean sample size per individual was only ~5. We let parameters vary among cohorts rather than year of catch, because individuals within cohorts share similar environmental conditions and density dependence (Morrongiello and Thresher, 2015). Eight models in total were fitted with area dummy-coded, with different combinations of shared and area-specific parameters. We evaluated if models with area-specific parameters led to better fit and quantified the differences in area-specific parameters (indexed by subscripts and ). The model with all area-specific parameters can be written as:
where log lengths are distributed to account for extreme observations, , , and represent the degrees of freedom, mean, and the scale parameter, respectively. and are dummy variables such that and if it is the reference area, and vice versa for the heated area. The multivariate normal distribution in Equation 3 is the prior for the cohort-varying parameters , , , and (for cohorts …,1997) (note that cohorts extend further back in time than the catch data), with hyper-parameters , , , describing the population means and a covariance matrix with the between-cohort variation along the diagonal. We did not model a correlation between the parameters, hence off-diagonals are 0. The other seven models include some or all parameters as parameters common for the two areas, e.g., substituting and with . To aid the convergence of this non-linear model, we used informative priors chosen after visualizing draws from prior predictive distributions (Wesner and Pomeranz, 2021) using probable parameter values (Figure 2—figure supplement 7; Figure 3—figure supplement 3). We used the same prior distribution for each parameter class for both areas to not introduce any other sources of differences in parameter estimates between areas. We used the following priors for the VBGE model: , , , and . parameters, , , , were given a prior.
We also compared how body growth scales with body size (in contrast to length vs age). This is because size-at-age reflects lifetime growth history rather than current growth and may thus be large because growth was fast early in life, not because current growth rates are fast (Lorenzen, 2016). We therefore fit allometric growth models describing how specific growth rate scales with length: , where , the annual specific growth between year and , is defined as: and is the geometric mean length: . Here we use back-calculated length-at-age, resulting in multiple observations per individual. As with the VBGE model, we dummy-coded area to compare models with different combinations of common and shared parameters. We assumed growth rates were distributed, and the full model can be written as:
We assumed only varied across individuals within cohorts and compared two models: one with common for the heated and reference area, and one with an area-specific . We used the following priors: , and . , and were all given a prior.
We estimated total mortality by fitting linear models to the natural log of catch (CPUE) as a function of age (catch curve regression), under the assumption that in a closed population, the exponential decline can be described as , where is the population at time , is the initial population size and is the instantaneous mortality rate. This equation can be rewritten as a linear equation: , where is a catch at age , if a catch is assumed proportional to the number of fish (i.e. ). Hence, the negative of the age slope is the mortality rate, . To get catch-at-age data, we constructed area-specific age-length keys using the sub-sample of the total (female) catch that was age-determined. Age length keys describe the age proportions of each length category (i.e. a matrix with length category as rows, and ages as columns). The age composition is then estimated for the total catch based on the ‘probability’ of fish in each length category being a certain age. Due to the smallest and youngest fish not being representatively caught with the gillnet, the catch is dome-shaped over size and age. We therefore followed the practice of selecting only ages on the descending right limb (Dunn et al., 2002; Figure 4—figure supplement 1). We fit this model with and without an -interaction, and the former can be written as:
where and are the intercepts for the reference and heated areas, respectively, is the age slope for the reference area and is the difference between the age slope in the reference area and in the heated area. All parameters vary by cohort (for cohort ). We use the default brms priors for these models, i.e., flat priors for the regression coefficients (Bürkner, 2017) and . and were given a prior.
Lastly, we quantified differences in the average age and size distributions between the areas. We estimate the biomass size-spectrum exponent directly, using the likelihood approach for binned data, i.e., the MLEbin method in the R package sizeSpectra (Edwards, 2020; Edwards et al., 2020; Edwards et al., 2017). This method explicitly accounts for uncertainty in body masses within size classes (bins) in the data and has been shown to be less biased than regression-based methods or the likelihood method based on bin midpoints (Edwards et al., 2020; Edwards et al., 2017). We pooled all years to ensure negative relationships between biomass and size in the size classes (as the sign of the relationship varied between years). We also fitted lognormal models as data are positive and tailed to length- and age-resolved catch data. Here, we assume that the catchability with respect to size does not differ between the areas, and, therefore, use the entire catch (Figure 6—figure supplement 1). In contrast to the catch curve regression, we do not need to filter representatively caught size or age classes. The lognormal models fitted to age or size (denoted ) model can be written as:
where is the intercept for the reference area and is the intercept for the heated area. These intercepts vary by year (for years ). We use flat priors for the regression coefficients, and was given a prior, and compared models with and without random slopes.
All analyses were done using R (R Development Core Team, 2020) version 4.0.2 with R Studio (2021.09.1). The packages within the tidyverse (Wickham et al., 2019) collection was used to process and visualize data. Models were fit using the R package brms (Bürkner, 2018). For the non-linear von Bertalanffy growth equation and the allometric growth model, we used informative priors to facilitate convergence. These were chosen by defining vague priors, and then progressively tightening these until convergence was achieved (Bürkner, 2017; Gesmann and Morris, 2020). We used prior predictive checks to ensure the priors were suitable (vague enough to include also unlikely predictions, but informative enough to ensure convergence), and the final prior predictive checks are shown in Figure 2—figure supplement 1 and Figure 3—figure supplement 1. We also explored priors vs posteriors to evaluate the influence of our informative priors visually (Figure 2—figure supplement 7; Figure 3—figure supplement 3). For the linear models (catch curve and mean size), which do not require the same procedure to achieve convergence typically, we used the default priors from brms as written above. We used three chains and 4000 iterations in total per chain. Models were compared by evaluating their expected predictive accuracy (expected log pointwise predictive density) using leave-one-out cross-validation (LOO-CV) (Vehtari et al., 2017) while ensuring Pareto values <0.7, in the R package loo (Vehtari et al., 2020). Results of the model comparison can be found in the Supplementary file 1. We used bayesplot (Gabry et al., 2019) and tidybayes (Kay, 2019) to process and visualize model diagnostics and posteriors. Model convergence and the fit were assessed by ensuring potential scale reduction factors () were less than 1.1, suggesting all three chains converged to a common distribution (Gelman et al., 2003), and by visually inspecting trace plots, residuals QQ-plots, and with posterior predictive checks (Figures 2—4, Figure 6—figure supplement 2).
Data availability
All raw data and R code to clean and reproduce the results reported in this paper are available on GitHub (copy archived at Lindmark, 2023) and have been deposited on Zenodo.
References
-
ReportBiologisk recipientkontroll vid forsmarks kärnkraftverk (in Swedish)Öregrund.
-
Size-based theory for fisheries adviceICES Journal of Marine Science 77:2445–2455.https://doi.org/10.1093/icesjms/fsaa157
-
Temperature and organism size—A biological law for ectotherms?Advances in Ecological Research 25:1–58.https://doi.org/10.1016/S0065-2504(08)60212-3
-
Why are organisms usually bigger in colder environments? Making sense of a life history puzzleTrends in Ecology & Evolution 12:235–239.https://doi.org/10.1016/s0169-5347(97)01058-6
-
Fish body sizes change with temperature but not all species shrink with warmingNature Ecology & Evolution 4:809–814.https://doi.org/10.1038/s41559-020-1171-0
-
Warming increases the cost of growth in a model vertebrateFunctional Ecology 33:1256–1266.https://doi.org/10.1111/1365-2435.13348
-
Old-growth fishes become scarce under fishingCurrent Biology 27:2843–2848.https://doi.org/10.1016/j.cub.2017.07.069
-
Warming temperatures and smaller body sizes: synchronous changes in growth of North Sea fishesGlobal Change Biology 20:1023–1031.https://doi.org/10.1111/gcb.12514
-
Rapid warming of large marine ecosystemsProgress in Oceanography 81:207–213.https://doi.org/10.1016/j.pocean.2009.04.011
-
Warmer water increases early body growth of Northern pike ( esox lucius ), but mortality has larger impact on decreasing body sizesCanadian Journal of Fisheries and Aquatic Sciences 79:771–781.https://doi.org/10.1139/cjfas-2020-0386
-
Nonlinear averaging of thermal experience predicts population growth rates in a thermally variable environmentProceedings. Biological Sciences 285:20181076.https://doi.org/10.1098/rspb.2018.1076
-
On the dynamics of exploited fish populationsFishery Investigations London Series 19:2106-4.https://doi.org/10.1007/978-94-011-2106-4
-
Do climate and fishing influence size-based indicators of Celtic Sea fish community structure?ICES Journal of Marine Science 62:405–411.https://doi.org/10.1016/j.icesjms.2005.01.006
-
From bacteria to whales: using functional size spectra to model marine ecosystemsTrends in Ecology & Evolution 32:174–186.https://doi.org/10.1016/j.tree.2016.12.003
-
Brms: an R package for bayesian multilevel models using stanJournal of Statistical Software 80:i01.https://doi.org/10.18637/jss.v080.i01
-
Shrinking of fishes exacerbates impacts of global ocean changes on marine ecosystemsNature Climate Change 3:254–258.https://doi.org/10.1038/nclimate1691
-
Testing and recommending methods for fitting size spectra to dataMethods in Ecology and Evolution 8:57–67.https://doi.org/10.1111/2041-210X.12641
-
Accounting for the bin structure of data removes bias when fitting size spectraMarine Ecology Progress Series 636:19–33.https://doi.org/10.3354/meps13230
-
Fitting growth models to otolith increments to reveal time-varying growthCanadian Journal of Fisheries and Aquatic Sciences 79:159–167.https://doi.org/10.1139/cjfas-2021-0046
-
Visualization in Bayesian workflowJournal of the Royal Statistical Society Series A 182:389–402.https://doi.org/10.1111/rssa.12378
-
Individual variation and interactions explain food web responses to global warmingPhilosophical Transactions of the Royal Society of London. Series B, Biological Sciences 375:20190449.https://doi.org/10.1098/rstb.2019.0449
-
Declining body size: a third universal response to warming?Trends in Ecology & Evolution 26:285–291.https://doi.org/10.1016/j.tree.2011.03.005
-
Size, growth, temperature and the natural mortality of marine fishFish and Fisheries 11:149–158.https://doi.org/10.1111/j.1467-2979.2009.00350.x
-
Climate change impacts on marine ecosystems through the lens of the size spectrumEmerging Topics in Life Sciences 3:233–243.https://doi.org/10.1042/ETLS20190042
-
An application of a bioenergetics model to Eurasian perch (Perca fluviatilis L)Journal of Fish Biology 41:217–230.https://doi.org/10.1111/j.1095-8649.1992.tb02652.x
-
Optimum growth temperature declines with body size within fish speciesGlobal Change Biology 28:2259–2271.https://doi.org/10.1111/gcb.16067
-
Predicting natural mortality rates of plants and animalsEcology Letters 11:710–716.https://doi.org/10.1111/j.1461-0248.2008.01190.x
-
Demographic changes in Chinook salmon across the Northeast Pacific OceanFish and Fisheries 19:533–546.https://doi.org/10.1111/faf.12272
-
Warming leads to opposite patterns in weight-at-age for young versus old age classes of Bering sea walleye pollockCanadian Journal of Fisheries and Aquatic Sciences 79:1655–1666.https://doi.org/10.1139/cjfas-2021-0315
-
On the interrelationships between natural mortality, growth parameters, and mean environmental temperature in 175 fish stocksICES Journal of Marine Science 39:175–192.https://doi.org/10.1093/icesjms/39.2.175
-
The gill-oxygen limitation theory (GOLT) and its criticsScience Advances 7:eabc6050.https://doi.org/10.1126/sciadv.abc6050
-
Heat tolerance in ectotherms scales predictably with body sizeNature Climate Change 11:58–63.https://doi.org/10.1038/s41558-020-00938-y
-
What we don't know about the effects of temperature on salmonid growthTransactions of the American Fisheries Society 151:3–12.https://doi.org/10.1002/tafs.10338
-
SoftwareR: A language and environment for statistical computingR Foundation for Statistical Computing, Vienna, Austria.
-
Growth, temperature, and density relationships of North Sea cod (Gadus morhua)Canadian Journal of Fisheries and Aquatic Sciences 65:456–470.https://doi.org/10.1139/f07-150
-
Effects of temperature on life history variables in perchJournal of Fish Biology 47:652–670.https://doi.org/10.1111/j.1095-8649.1995.tb01932.x
-
The production of particles in the surface waters of the ocean with particular reference to the Sargasso SeaLimnology and Oceanography 18:719–733.https://doi.org/10.4319/lo.1973.18.5.0719
-
Shrinking body size as an ecological response to climate changeNature Climate Change 1:401–406.https://doi.org/10.1038/nclimate1259
-
BookMetoder För Övervakning Av Kustfiskbestånd in SwedishKustlaboratoriet, Fiskeriverket.
-
Predicting life history parameters for all fishes worldwideEcological Applications 27:2262–2276.https://doi.org/10.1002/eap.1606
-
ThesisFish life histories in a warming climate: a mechanistic basis of change and a community contextSwedish University of Agricultural Sciences, Uppsala, Sweden.https://doi.org/10.54612/a.61rases4mb
-
A mathematical model of some aspects of fish growth, respiration, and mortalityJournal of the Fisheries Research Board of Canada 24:2355–2453.https://doi.org/10.1139/f67-190
-
Implications of late-in-life density-dependent growth for fishery size-at-entry leading to maximum sustainable yieldICES Journal of Marine Science 75:1296–1305.https://doi.org/10.1093/icesjms/fsx236
-
Practical Bayesian model evaluation using leave-one-out cross-validation and WAICStatistics and Computing 27:1413–1432.https://doi.org/10.1007/s11222-016-9696-4
-
A quantitative theory of organic growth (inquiries on growth lawsHuman Biology 10:181–213.
-
Relationships between body size and abundance in ecologyTrends in Ecology & Evolution 22:323–330.https://doi.org/10.1016/j.tree.2007.03.007
-
Welcome to the tidyverseJournal of Open Source Software 4:1686.https://doi.org/10.21105/joss.01686
-
Smaller adult fish size in warmer water is not explained by elevated metabolismEcology Letters 25:1177–1188.https://doi.org/10.1111/ele.13989
Article and author information
Author details
Funding
No external funding was received for this work.
Acknowledgements
We thank all staff involved in data collection, Jens Olsson and Göran Sundblad for discussions, Christine Stawitz and an anonymous reviewer for feedback that greatly improved the manuscript, and Forsmark Kraftgrupp AB for making data publicly available. This study was supported by SLU Quantitative Fish and Fisheries Ecology.
Copyright
© 2023, Lindmark et al.
This article is distributed under the terms of the Creative Commons Attribution License, which permits unrestricted use and redistribution provided that the original author and source are credited.
Metrics
-
- 951
- views
-
- 122
- downloads
-
- 8
- citations
Views, downloads and citations are aggregated across all versions of this paper published by eLife.
Download links
Downloads (link to download the article as PDF)
Open citations (links to open the citations from this article in various online reference manager services)
Cite this article (links to download the citations from this article in formats compatible with various reference manager tools)
Further reading
-
- Ecology
Bumblebees (Bombus terrestris) have been shown to engage in string-pulling behavior to access rewards. The objective of this study was to elucidate whether bumblebees display means-end comprehension in a string-pulling task. We presented bumblebees with two options: one where a string was connected to an artificial flower containing a reward and the other presenting an interrupted string. Bumblebees displayed a consistent preference for pulling connected strings over interrupted ones after training with a stepwise pulling technique. When exposed to novel string colors, bees continued to exhibit a bias towards pulling the connected string. This suggests that bumblebees engage in featural generalization of the visual display of the string connected to the flower in this task. If the view of the string connected to the flower was restricted during the training phase, the proportion of bumblebees choosing the connected strings significantly decreased. Similarly, when the bumblebees were confronted with coiled connected strings during the testing phase, they failed to identify and reject the interrupted strings. This finding underscores the significance of visual consistency in enabling the bumblebees to perform the task successfully. Our results suggest that bumblebees’ ability to distinguish between continuous strings and interrupted strings relies on a combination of image matching and associative learning, rather than means-end understanding. These insights contribute to a deeper understanding of the cognitive processes employed by bumblebees when tackling complex spatial tasks.
-
- Ecology
During collective vigilance, it is commonly assumed that individual animals compromise their feeding time to be vigilant against predators, benefiting the entire group. One notable issue with this assumption concerns the unclear nature of predator ‘detection’, particularly in terms of vision. It remains uncertain how a vigilant individual utilizes its high-acuity vision (such as the fovea) to detect a predator cue and subsequently guide individual and collective escape responses. Using fine-scale motion-capture technologies, we tracked the head and body orientations of pigeons (hence reconstructed their visual fields and foveal projections) foraging in a flock during simulated predator attacks. Pigeons used their fovea to inspect predator cues. Earlier foveation on a predator cue was linked to preceding behaviors related to vigilance and feeding, such as head-up or down positions, head-scanning, and food-pecking. Moreover, earlier foveation predicted earlier evasion flights at both the individual and collective levels. However, we also found that relatively long delay between their foveation and escape responses in individuals obscured the relationship between these two responses. While our results largely support the existing assumptions about vigilance, they also underscore the importance of considering vision and addressing the disparity between detection and escape responses in future research.