Evolved bacterial resistance to the chemotherapy gemcitabine modulates its efficacy in co-cultured cancer cells
Figures
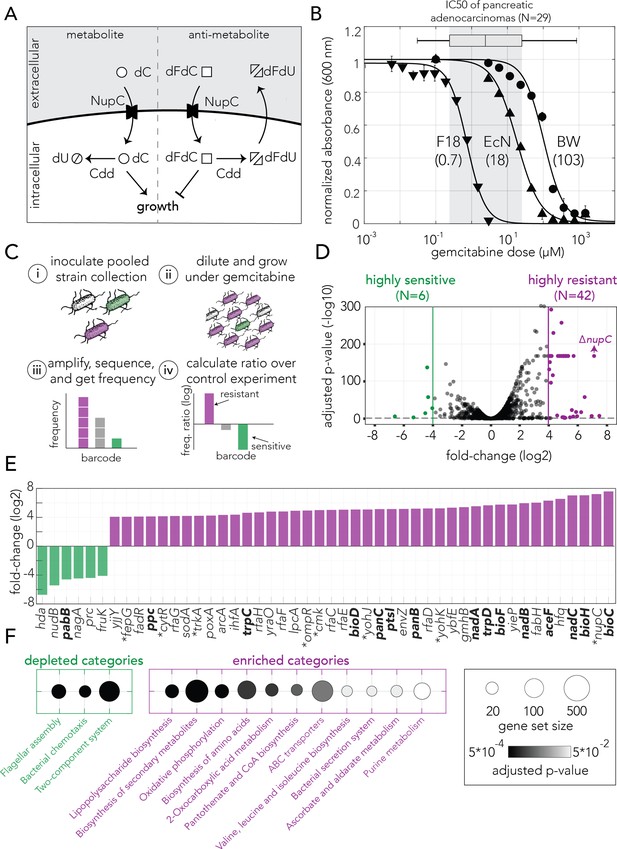
Genetic screen identifies gemcitabine sensitive and resistant loss-of-function mutations in E. coli.
(A) Gemcitabine transport and metabolism in E. coli. Similar to deoxycytidine (dC), gemcitabine (dFdC) is imported into the cell through the nucleoside permease NupC. Intracellular gemcitabine is either converted into the less toxic metabolite dFdU by the cytidine deaminase Cdd or is phosphorylated and incorporated into the DNA. (B) Gemcitabine dose–response curves for three E. coli strains (three technical replicates, error bars represent standard deviation, experiment performed once). The inferred IC50 are shown in parenthesis. Gemcitabine IC50 range of 29 pancreatic adenocarcinomas are shown as a box plot above the graph. (C) Overview of the pooled screening approach. Pooled cultures of the knockout strain collection were inoculated (i) and grown for multiple hours with or without gemcitabine (ii), DNA extracted from cells was used to amplify, sequence and calculate the frequency of barcodes that correspond to individual strains (iii), and the ratio of barcode frequencies of each strain in gemcitabine and control conditions were used to identify sensitive and resistant knockouts (iv). Experiment includes three biological replicates (independent inoculums). (D) Volcano plot of the genetic screen results. Green and purple dots represent sensitive and resistant knockout strains, respectively. (E) Highly sensitive and resistant strains. Asterisk marks the gene knockouts likely involved in gemcitabine transport and phosphorylation. Knockouts marked in bold were characterized as slow-growing strains. (F) Statistically significant enriched and depleted KEGG categories identified by the genetic screen (FDR adjusted p-value cutoff: 0.1). The marker size shows the number of genes in the category and the gray scale marks the statistical significance.
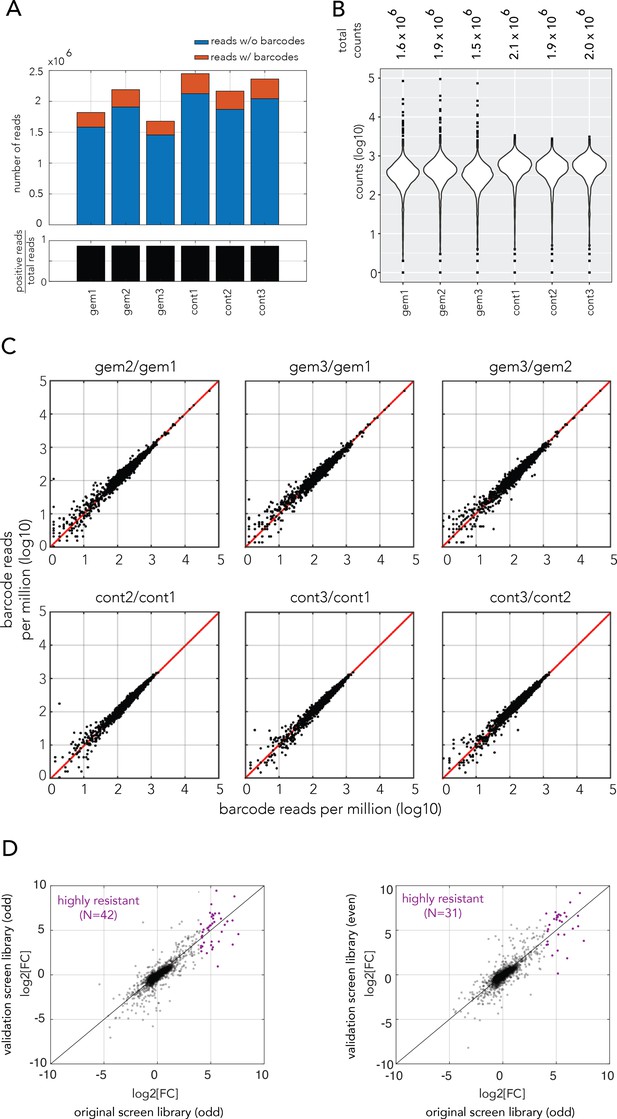
Statistics of the gemcitabine genetic screen performed with the E. coli barcoded knockout strain collection.
(A) Bar plots showing the number of reads with or without barcodes across samples (three biological replicates with gemcitabine and without drug). (B) Violin plots showing the frequency of individual barcode counts. (C) Scatter plots of barcode counts across biological replicates (D) Comparison between independent biological replicates of the genetic screen for gemcitabine resistance. Left panel: comparison between the log-fold change in strain enrichment between the original screen and a screen performed with the same strain collection (odd collection) on the different day. Right panel: comparison between the log-fold change in strain enrichment between the original screen and a screen performed with the knockout strain collection that was independently cloned (even collection). Strain marked in purple are resistant knockout strains that were identified in the original screen (Figure 1E). Eleven knockout strains that were gemcitabine resistant in the original screen were not detected at all newly used strain collection (even collection).
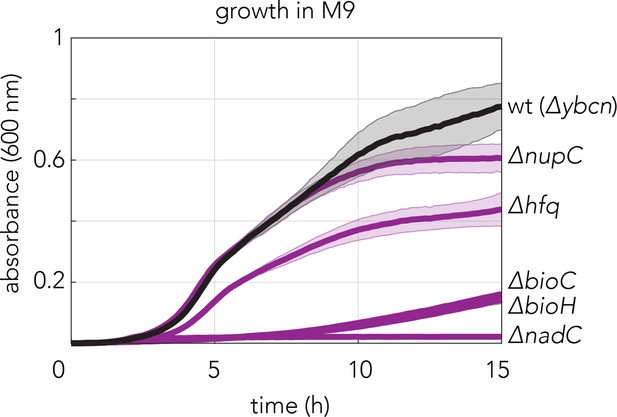
Growth of top five resistant gemcitabine knockouts indentified by the genetic screen in M9 minimal medium.
The ybcn deletion strain was used as a wild-type control since this gene deletion does not impact E. coli growth and genetic background of this strain is comparable to the other mutants used in the experiment. Background subtracted mean absorbance (600nm) of three technical replicates are plotted. Shaded areas show the standard deviation.
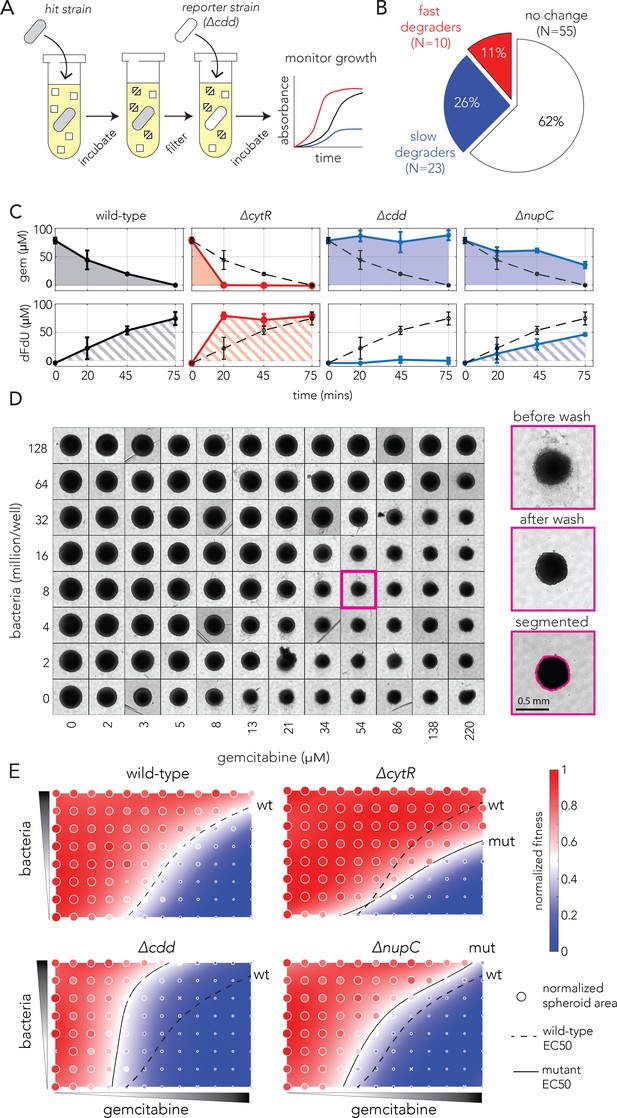
Bacterial gemcitabine resistance can oppositely affect drug degradation and impact neighboring cancer cells.
(A) The functional drug breakdown assay. Each knockout strain was inoculated in saline containing gemcitabine and incubated for 15 or 45 min. Conditioned supernatant was then filtered and mixed with fresh media before inoculating a reporter strain. Reporter strain growth was used as a proxy for changes in gemcitabine availability in the conditioned supernatant. (B) Results of the drug breakdown assay for the top 88 resistant knockouts. A third of all tested knockouts (33/88) influenced the drug degradation rate. (C) Chemical validation of the functional assay for the slowest and fastest degraders. GC-MS was used to measure gemcitabine concentration (shaded area, top panels) and its degradation product dFdU (hatched area, lower panels) in conditioned supernatant (colors as in A). The dashed black curve marks the measurements after incubation with the wild-type strain. The error bars show the standard deviation of three biological replicates. (D) Co-culture experiments shows bacterial mutations can influence gemcitabine efficacy in neighboring spheroids of cancer cells. Representative microscopy images of spheroids that were co-cultured with wild-type bacteria across multiple concentrations of drug and bacteria (large images show the same spheroid before the wash, after the wash that removed dead cell debris, and after image segmentation). (E) Bacterial mutations that modulate drug degradation can impact drug efficacy in neighboring cancer cells. Results of spheroid experiments with the slowest and fastest degraders and the inferred fitness landscapes. Each panel shows the fitness landscape calculated from spheroid size across a range of gemcitabine and bacteria concentrations (as in D). The color code shows the normalized spheroid size (ranging from the smallest spheroid to the largest one). The dashed black line marks the parameter combination (bacteria and gemcitabine concentrations) that reduce spheroid growth by 50% (EC50 front), and solid lines show the EC50 front for the knockout strains. Shifts in the EC50 front, relative to the wild-type front, indicate that co-incubation with the knockout strain during drug exposure altered the spheroid’s chemoresistance.
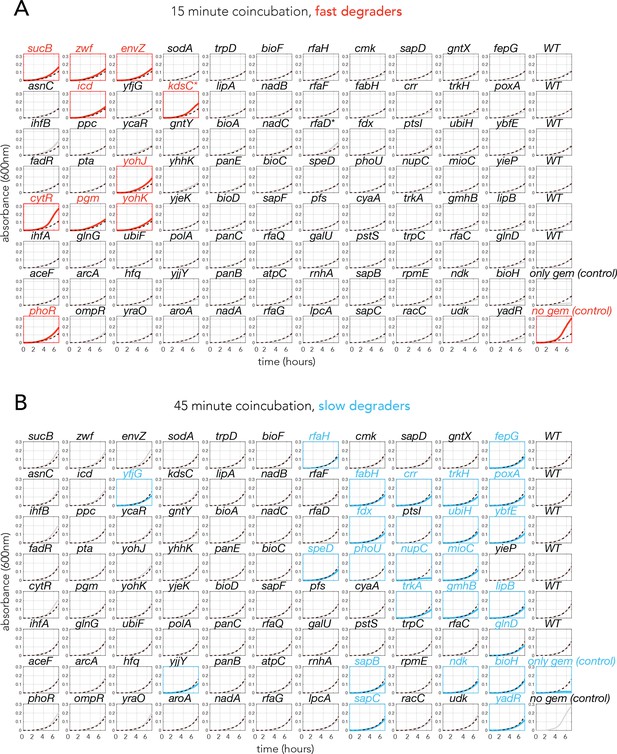
Individual growth curves of reporter strain (cdd knockout) in the functional assay to estimate gemcitabine breakdown rate of the top 88 gemcitabine resistant genetic screen hits.
The title of each graph shows the knockout strain that was incubated with gemcitabine before the reporter strain was inoculated into the filtered conditioned supernatant. (A) Mean growth curves of the reporter strain in the conditioned supernatant filtered after 15 min of co-incubation (three biological replicates) with the 88 tested knockout strains. Statistically significant fast degraders are shown with red color (one-tailed Student’s t-test with FDR-corrected p-adj<0.1). (B) Mean growth curves of the reporter strain in the conditioned supernatant filtered after 45 min of co-incubation (three biological replicates) with 88 tested knockout strains. Statistically significant slow degraders are shown with blue color (one-tailed Student’s t-test with FDR-corrected p-adj<0.1).
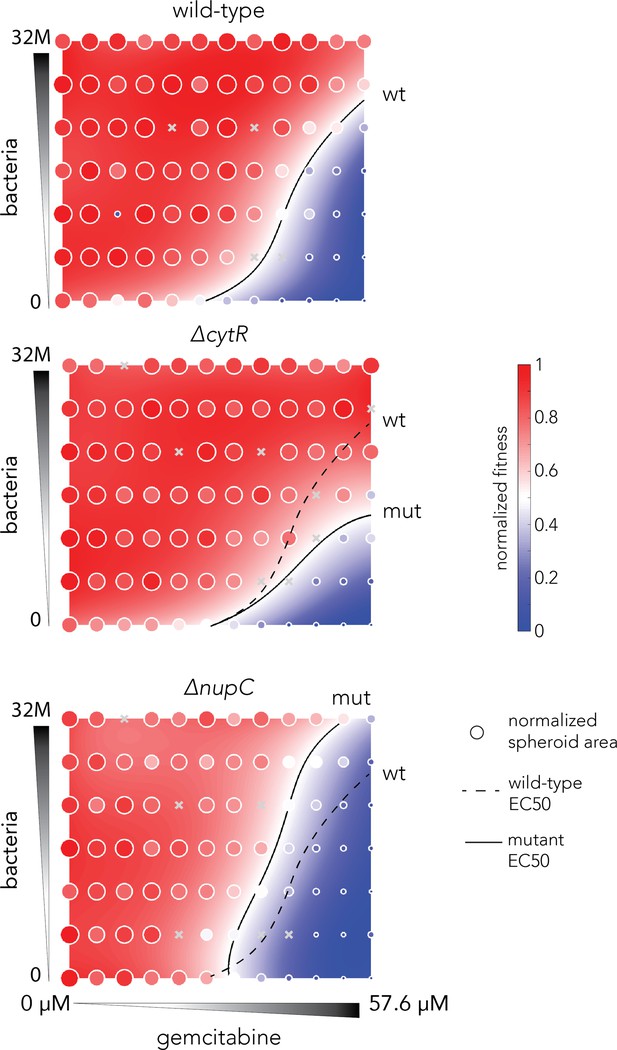
Validation of results of spheroid experiment (Figure 2E).
The fitness landscape inferred for the slowest and fastest bacterial degraders relative to the wild-type strains. Each panel shows the fitness landscape calculated from spheroid size across a range of gemcitabine (0 μM to 57.6 μM as 1.5-fold dilutions) and bacterial concentrations(0–32 million as two-fold dilutions). The color code shows the normalized spheroid size (ranging from the smallest spheroid to the largest one). The dashed black line marks the parameter combination (bacteria and gemcitabine concentrations) that reduce spheroid growth by 50% (EC50 front) and solid lines show the EC50 front for the knockout strains. Shifts in the EC50 front, relative to the wild-type front, indicate that co-incubation with the knockout strain during drug exposure altered the spheroid’s chemoresistance.
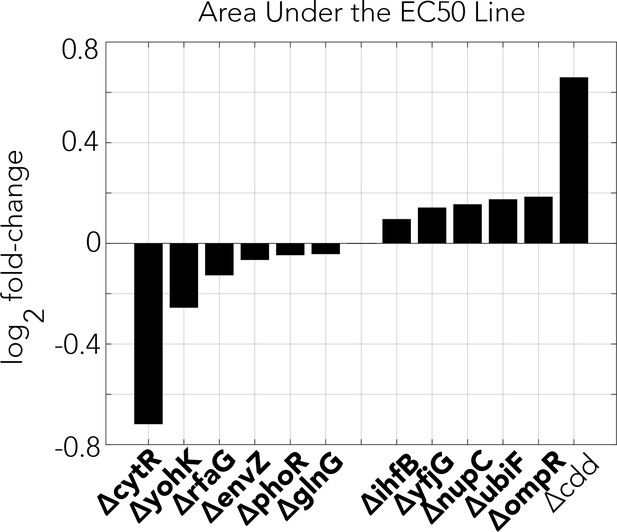
Changes in area under the EC50 front in spheroid fitness landscapes generated with co-incubation of gemcitabine and selected gemcitabine-resistant knockout strains (Figure 2E).
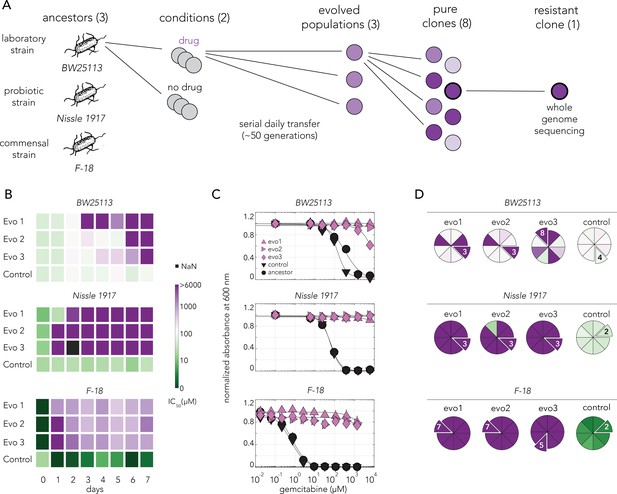
Gemcitabine selection leads to rapid evolved resistance in three E. coli strains.
(A) Overall approach for the lab evolution experiment. Three E. coli strains were evolved over 50 generations in serial transfer evolution experiment. We measured gemcitabine resistance of last day populations and eight single clones were isolated from each population. A single resistant clone was used for the whole-genome sequencing and for identifying of the underlying mechanism for drug resistance. (B) Heatmaps showing the temporal changes in gemcitabine IC50 of evolving populations in each day of the serial transfer experiment. (C) Gemcitabine dose–response curves of last day populations from lab evolution experiment. All of the gemcitabine evolved lines, but not the control evolved lines, developed a high resistance against gemcitabine. Error bars show the standard deviation of three technical replicates. (D) Pie charts showing the gemcitabine IC50 levels of screened clones. Slices represent the clone selected for whole-genome sequencing. Color scale is the same as panel (B).
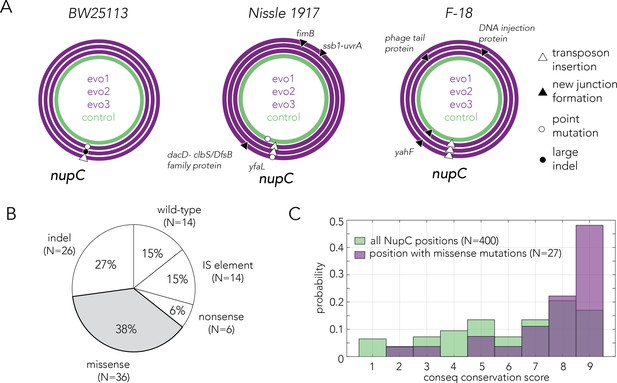
Evolved resistance converges to inactivation of the nucleoside permease NupC.
(A) Circa plots showing the mutations identified by whole-genome sequencing in pure clones isolated from independently evolved populations. Mutations in the coding region of the nupC gene were observed across all gemcitabine-evolved clones but not in the no-drug control evolved clones. Since the F-18 genome is not fully assembled, relative positions on the F-18 circa plot are not real genomic positions. (B) Pie chart showing the frequency of various mutation types identified by sequencing the nupC gene across 96 spontaneous gemcitabine resistant mutants in the BW25113 strain. (C) A comparison of the evolutionary conservation of missense mutation positions relative to the conservation of all positions in the nupC gene. The positions of the missense mutations are statistically biased towards the conserved positions (p-value = 1.95 * 10–4 in Wilcoxon rank-sum test).
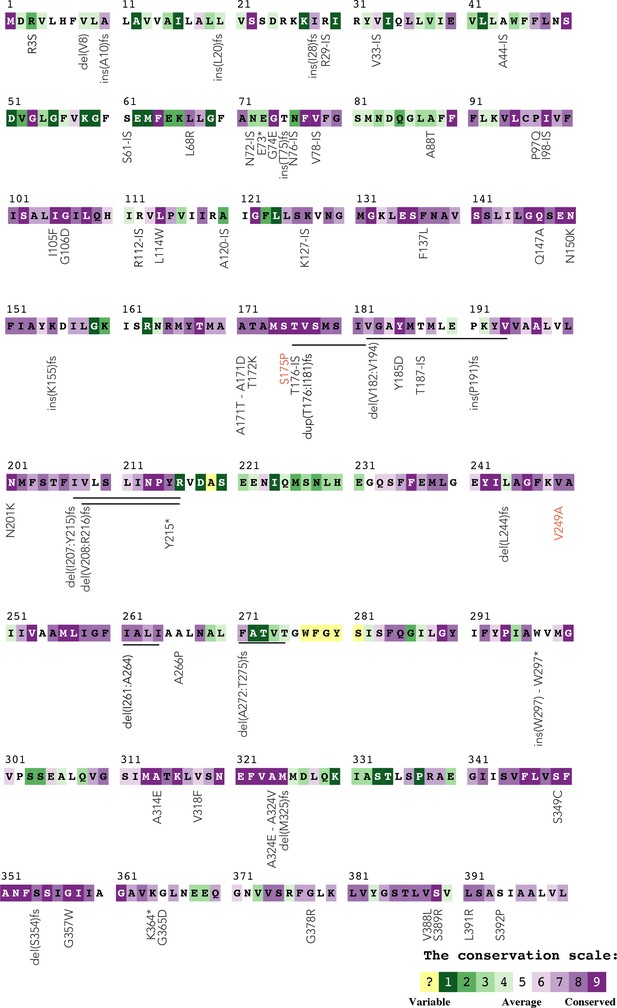
Annotation of nupC mutations by evolutionary conservation.
The color code shows the conservation level inferred for the NupC protein with the ConSurf server. Mutations shown in black are found in spontaneous mutants isolated from agar plates containing high concentrations of gemcitabine. Mutations shown in red are missense mutations found in the gemcitabine-evolved strains. del: deletion; ins: insertion; fs: frameshift-causing mutation; IS: insertion element (transposon insertion).
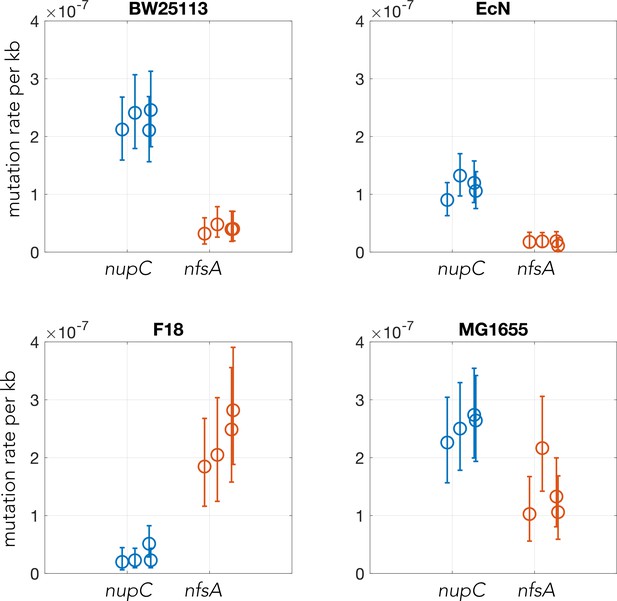
Plots showing the calculated mutation rates for the loci nupC and nfsA in four E. coli strains using Luria–Delbrück fluctuation experiments.
Errorbars show the 95% confidence intervals (data from ten technical replicates for each biological replicate). Each experiment was performed with four biological replicates/single colonies.
Tables
Bacteria used in this study.
Strain | Source | Remarks |
---|---|---|
E. coli BW25113 | Walhout Lab, University of Massachusetts Chan Medical School, MA, USA | |
E. coli F-18 | McCormick Lab, University of Massachusetts Chan Medical School, MA, USA | |
E. coli MG1655 | Brewster Lab, University of Massachusetts Chan Medical School, MA, USA | |
E. coli Nissle 1917 | ArdeyPharm GmbH, (Pharma Zentrale GmbH), Germany | |
E. coli KEIO knockout collection | Dharmacon (GE Life Sciences) | |
E. coli Barcoded knockout collection (barcoded library) | Hirotada Mori, Nara Institute of Science and Technology, Japan | Parent Strain: BW38028 |
BW25113 ∆pyrD::tGFP-chlr-barcode ∆cdd::kanr | Barcoded library, KEIO Collection | Double knockout was generated by P1 transduction |
BW25113 ∆pyrD::tGFP-chlr-barcode ∆cytR::kanr | Barcoded library, KEIO Collection | Double knockout was generated by P1 transduction |
BW25113 ∆pyrD::tGFP-chlr-barcode ∆envZ::kanr | Barcoded library, KEIO Collection | Double knockout was generated by P1 transduction |
BW25113 ∆pyrD::tGFP-chlr-barcode ∆glnG::kanr | Barcoded library, KEIO Collection | Double knockout was generated by P1 transduction |
BW25113 ∆pyrD::tGFP-chlr-barcode ∆ihfB::kanr | Barcoded library, KEIO Collection | Double knockout was generated by P1 transduction |
BW25113 ∆pyrD::tGFP-chlr-barcode ∆nupC::kanr | Barcoded library, KEIO Collection | Double knockout was generated by P1 transduction |
BW25113 ∆pyrD::tGFP-chlr-barcode ∆ompR::kanr | Barcoded library, KEIO Collection | Double knockout was generated by P1 transduction |
BW25113 ∆pyrD::tGFP-chlr-barcode ∆phoR::kanr | Barcoded library, KEIO Collection | Double knockout was generated by P1 transduction |
BW25113 ∆pyrD::tGFP-chlr-barcode ∆rfaG::kanr | Barcoded library, KEIO Collection | Double knockout was generated by P1 transduction |
BW25113 ∆pyrD::tGFP-chlr-barcode ∆ubiF::kanr | Barcoded library, KEIO Collection | Double knockout was generated by P1 transduction |
BW25113 ∆pyrD::tGFP-chlr-barcode ∆yfjG::kanr | Barcoded library, KEIO Collection | Double knockout was generated by P1 transduction |
BW25113 ∆pyrD::tGFP-chlr-barcode ∆yohK::kanr | Barcoded library, KEIO Collection | Double knockout was generated by P1 transduction |
Additional files
-
Supplementary file 1
Primary and validation screen results.
- https://cdn.elifesciences.org/articles/83140/elife-83140-supp1-v2.xlsx
-
Supplementary file 2
Enriched and depleted pathways in the genetic screen using two databases: KEGG: yellow; GO: green, p-adj<0.1.
- https://cdn.elifesciences.org/articles/83140/elife-83140-supp2-v2.xlsx
-
Supplementary file 3
Summary of the mutations detected with the BreSeq tool in evolved strains.
- https://cdn.elifesciences.org/articles/83140/elife-83140-supp3-v2.xlsx
-
Supplementary file 4
Mutation rates (per kb) for the nupC and nfsA genes determined by Luria–Delbrück fluctuation experiments.
- https://cdn.elifesciences.org/articles/83140/elife-83140-supp4-v2.xlsx
-
Supplementary file 5
List of mutations and their annotations detected in gemcitabine resistant BW25113 colonies.
- https://cdn.elifesciences.org/articles/83140/elife-83140-supp5-v2.xlsx
-
MDAR checklist
- https://cdn.elifesciences.org/articles/83140/elife-83140-mdarchecklist1-v2.docx