Systems level identification of a matrisome-associated macrophage polarisation state in multi-organ fibrosis
Figures
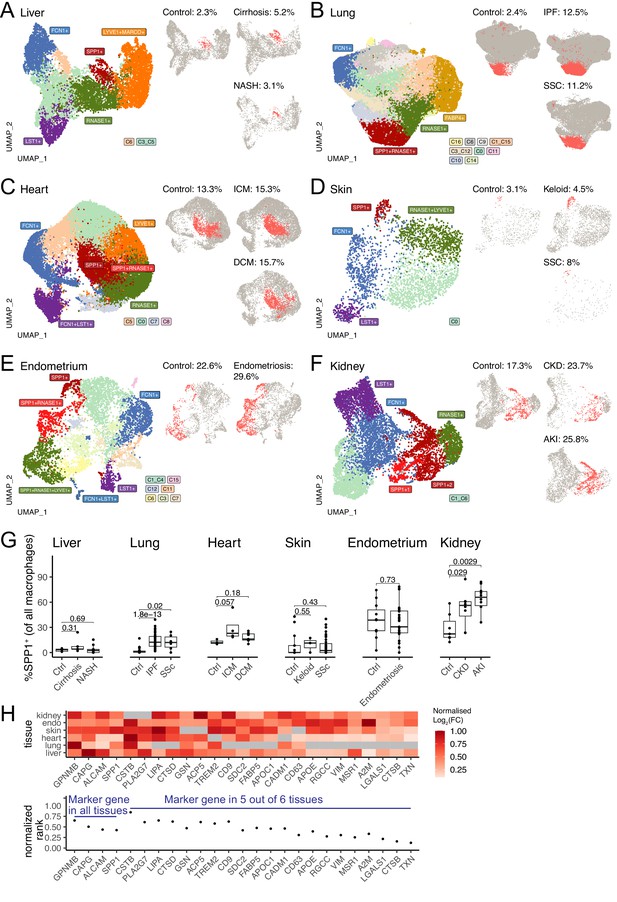
SPP1+ macrophages are increased during fibrotic disease across human tissues.
(A–F) Uniform Manifold Approximation and Projection (UMAP) dimensionality reduction of all monocytes and macrophages in single-cell RNA-sequencing (scRNA-seq) of liver (A), lung (B), heart (C), skin (D), endometrium (E), and kidney (F) from fibrotic disease patients or controls (see Table 1 for details on cell and sample numbers). Specific clusters were denoted as SPP1+ macrophages, RNASE1+/LYVE1+ homeostatic macrophages, MARCO+/FABP4+ tissue-specific macrophages, FCN1+ classical monocytes, or LST1+ non-classical monocytes based on expression of marker genes (see Figure 1—figure supplements 1–6 for details), while the remaining transitional macrophages were denoted based on their cluster ID from unsupervised clustering. SPP1+ macrophages in each tissue are stratified by disease conditions and coloured in red on separate UMAP; the proportion of SPP1+ macrophages out of all macrophages in each condition is indicated. (G) Boxplot summarising the relative proportion of SPP1+ macrophages (of all macrophages) in each subject stratified by disease status. Liver: (control) n=5, (cirrhosis) n=5, (nonalcoholic steatohepatitis [NASH]) n=9; lung: (control) n=29, (idiopathic pulmonary fibrosis [IPF]) n=40, (systemic sclerosis [SSC]) n=6; heart: (control) n=3, (ischemic cardiomyopathy [ICM]) n=4, (dilated cardiomyopathy [DCM]) n=7; skin: (control) n=13, (keloid) n=3, (SSC) n=39; endometrium: (control) n=11, (endometriosis) n=27; kidney: (control) n=7, (chronic kidney disease [CKD]) n=8, (acute kidney injury [AKI]) n=10. The Wilcoxon rank-sum test was used to evaluate the significance of the difference between groups. (H) Heatmap displaying the log2-fold-change (FC) of the top differentially expressed genes (DEGs) (x-axis) upregulated in SPP1+ macrophages compared to other macrophages across different tissues (top panel). For each gene, the log2FC was scaled to the highest value in each tissue and non-DEGs are denoted in grey. Rank-plot prioritising top DEGs conserved across tissues (bottom panel), where the x-axis shows marker genes and y-axis represents the cumulative rank of each DEG based on log2FC within each tissue.
-
Figure 1—source data 1
Marker genes for monocyte / macrophages.
- https://cdn.elifesciences.org/articles/85530/elife-85530-fig1-data1-v2.xlsx
-
Figure 1—source data 2
Marker genes for TREM2+ macrophages.
- https://cdn.elifesciences.org/articles/85530/elife-85530-fig1-data2-v2.xlsx
-
Figure 1—source data 3
GSEA analysis of DEG between SPP1+ macrophages and other macrophages in each tissue.
- https://cdn.elifesciences.org/articles/85530/elife-85530-fig1-data3-v2.xlsx
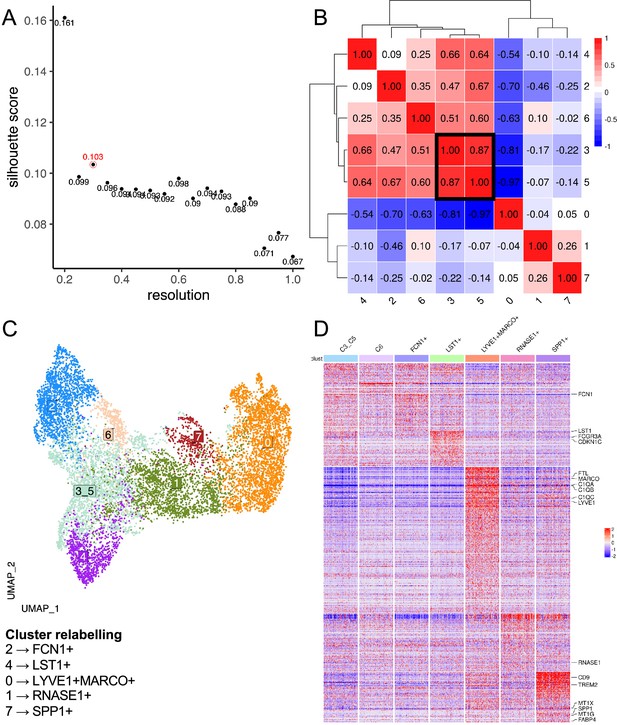
Data-driven clustering of liver macrophages.
(A) Scatterplot of Silhouette score (see Materials and methods) of single-cell unsupervised Louvain clustering results at different resolutions, which dictates the granularity of clustering. The optimal clustering is determined from a resolution ≥ 0.3 which maximises the Silhouette score, corresponding to a resolution of 0.3 with a score of 0.103 (circled in red). (B) Heatmap showing Spearman correlation between the pseudo-bulk profiles of clusters derived by using the optimal resolution. Clusters with Spearman correlation >0.6 were merged (cluster 3&5). (C) Uniform Manifold Approximation and Projection (UMAP) projection of liver macrophages, with each cell coloured by its merged cluster assignment. Specific clusters are then relabelled based on marker gene expression to give Figure 1A. (D) Heatmap displays the scaled gene expression expressions of top marker genes (average log2-fold-change>3) of each cluster across single cells. Only 200 cells are randomly sampled per cluster and shown here. Monocyte/macrophage cell-type genes and selected genes (Figure 1—source data 1 and 2) are annotated.
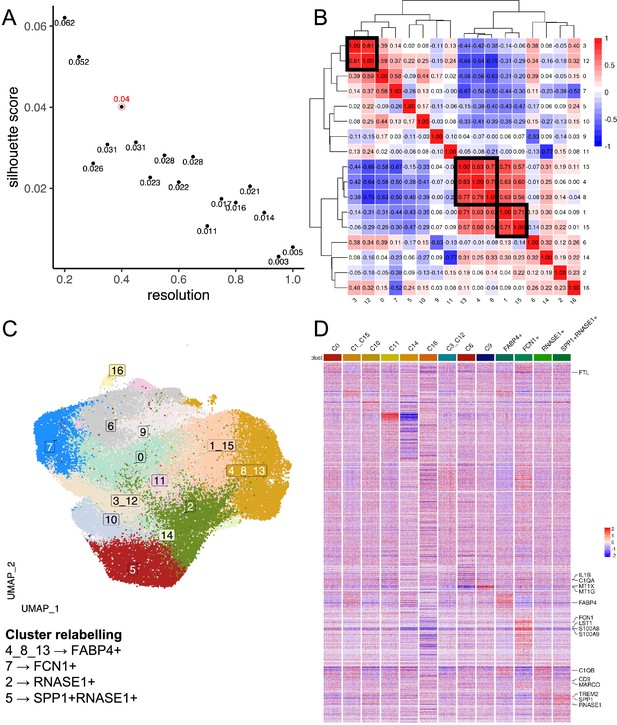
Data-driven clustering of lung macrophages.
(A) Scatterplot of Silhouette score (see Materials and methods) of single-cell unsupervised Louvain clustering results at different resolutions, which dictates the granularity of clustering. The optimal clustering is determined from a resolution ≥ 0.3 which maximises the Silhouette score, corresponding to a resolution of 0.4 with a score of 0.04 (circled in red). (B) Heatmap showing the Spearman correlation between the pseudo-bulk profiles of clusters derived by using the optimal resolution. Clusters with Spearman correlation >0.6 were merged (cluster 3&12; 13&4&8; 1&15). (C) Uniform Manifold Approximation and Projection (UMAP) projection of lung macrophages, with each cell coloured by its merged cluster assignment. Specific clusters are then relabelled based on marker gene expression to give Figure 1B. (D) Heatmap displays the scaled gene expression expressions of top marker genes (average log2-fold-change>3) of each cluster across single cells. Only 200 cells are randomly sampled per cluster and shown here. Monocyte/macrophage cell-type genes and selected genes (Figure 1—source data 1 and 2) are annotated.
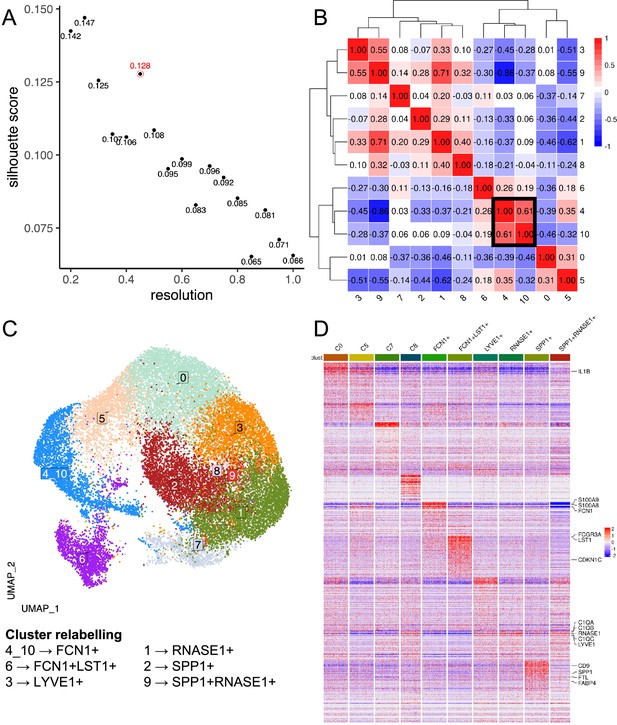
Data-driven clustering of heart macrophages.
(A) Scatterplot of Silhouette score (see Materials and methods) of single-cell unsupervised Louvain clustering results at different resolutions, which dictates the granularity of clustering. The optimal clustering is determined from a resolution ≥ 0.3 which maximises the Silhouette score, corresponding to a resolution of 0.45 with a score of 0.128 (circled in red). (B) Heatmap showing the Spearman correlation between the pseudo-bulk profiles of clusters derived by using the optimal resolution. Clusters with Spearman correlation >0.6 were merged (cluster 4&10). (C) Uniform Manifold Approximation and Projection (UMAP) projection of heart macrophages, with each cell coloured by its merged cluster assignment. Specific clusters are then relabelled based on marker gene expression to give Figure 1C. (D) Heatmap displays the scaled gene expression expressions of top marker genes (average log2-fold-change>3) of each cluster across single cells. Only 200 cells are randomly sampled per cluster and shown here. Monocyte/macrophage cell-type genes and selected genes (Figure 1—source data 1 and 2) are annotated.

Data-driven clustering of skin macrophages.
(A) Scatterplot of Silhouette score (see Materials and methods) of single-cell unsupervised Louvain clustering results at different resolutions, which dictates the granularity of clustering. The optimal clustering is determined from a resolution ≥ 0.3 which maximises the Silhouette score, corresponding to a resolution of 0.3 with a score of 0.117 (circled in red). (B) Heatmap showing the Spearman correlation between the pseudo-bulk profiles of clusters derived by using the optimal resolution. (C) Uniform Manifold Approximation and Projection (UMAP) projection of skin macrophages, with each cell coloured by its cluster assignment. Specific clusters are then relabelled based on marker gene expression to give Figure 1D. (D) Heatmap displays the scaled gene expression expressions of top marker genes (average log2-fold-change>3) of each cluster across single cells. Only 200 cells are randomly sampled per cluster and shown here. Monocyte/macrophage cell-type genes and selected genes (Figure 1—source data 1 and 2) are annotated.
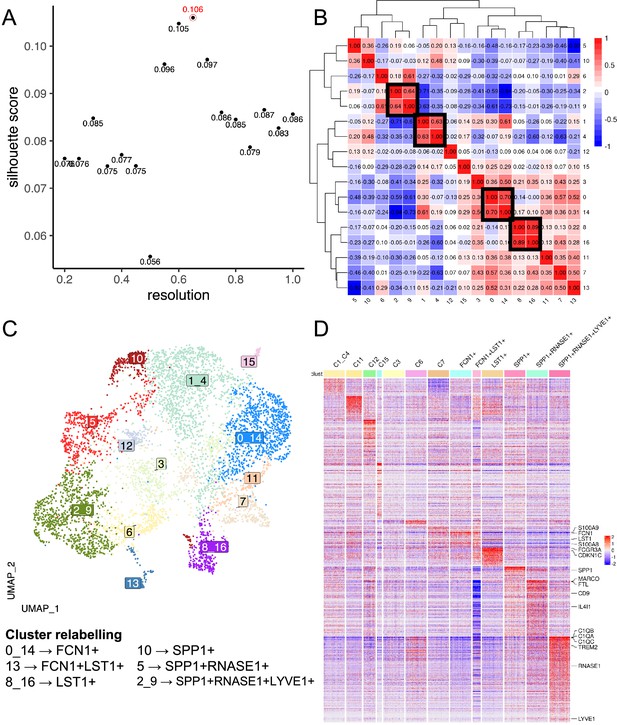
Data-driven clustering of endometrium macrophages.
(A) Scatterplot of Silhouette score (see Materials and methods) of single-cell unsupervised Louvain clustering results at different resolutions, which dictates the granularity of clustering. The optimal clustering is determined from a resolution ≥ 0.3 which maximises the Silhouette score, corresponding to a resolution of 0.65 with a score of 0.106 (circled in red). (B) Heatmap showing the Spearman correlation between the pseudo-bulk profiles of clusters derived by using the optimal resolution. Clusters with Spearman correlation >0.6 were merged (cluster 2&9; 1&4; 0&14; 8&16). (C) Uniform Manifold Approximation and Projection (UMAP) projection of endometrium macrophages, with each cell coloured by its merged cluster assignment. Specific clusters are then relabelled based on marker gene expression to give Figure 1E. (D) Heatmap displays the scaled gene expression expressions of top marker genes (average log2-fold-change>3) of each cluster across single cells. Only 200 cells are randomly sampled per cluster and shown here. Monocyte/macrophage cell-type genes and selected genes (Figure 1—source data 1 and 2) are annotated.
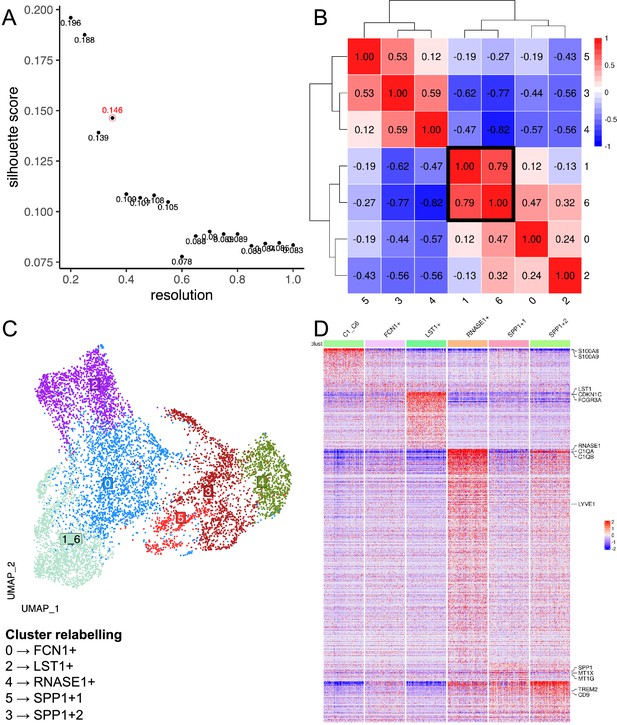
Data-driven clustering of kidney macrophages.
(A) Scatterplot of Silhouette score (see Materials and methods) of single-cell unsupervised Louvain clustering results at different resolutions, which dictates the granularity of clustering. The optimal clustering is determined from a resolution ≥ 0.3 which maximises the Silhouette score, corresponding to a resolution of 0.35 with a score of 0.146 (circled in red). (B) Heatmap showing Spearman correlation between the pseudo-bulk profiles of clusters derived by using the optimal resolution. Clusters with Spearman correlation >0.6 were merged (cluster 1&6). (C) Uniform Manifold Approximation and Projection (UMAP) projection of kidney macrophages, with each cell coloured by its merged cluster assignment. Specific clusters are then relabelled based on marker gene expression to give Figure 1F. (D) Heatmap displays the scaled gene expression expressions of top marker genes (average log2-fold-change>3) of each cluster across single cells. Only 200 cells are randomly sampled per cluster and shown here. Monocyte/macrophage cell-type genes and selected genes (Figure 1—source data 1 and 2) are annotated.
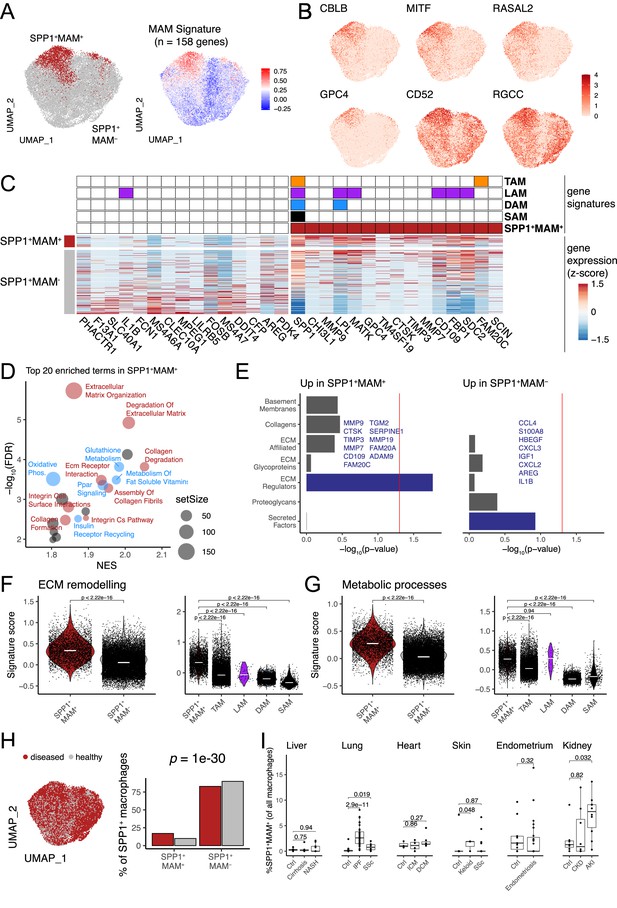
Identification of a matrisome-associated macrophage (MAM) state within SPP1+ macrophages.
(A) Uniform Manifold Approximation and Projection (UMAP) dimensionality reduction of all SPP1+ macrophages merged from the liver, lung, heart, skin, endometrium, and kidney tissues following data integration (see Materials and methods). Unsupervised clustering of SPP1+ macrophages identified a MAM subpopulation (red), denoted as SPP1+MAM+ (left panel; Figure 2—figure supplement 1). Each macrophage is coloured by the expression level of SPP1+MAM+ signature genes (n=158 genes; right panel). (B) UMAP of SPP1+ macrophages with each cell coloured by the expression of top markers for SPP1+MAM+ macrophages as predicted by COMET (see Materials and methods and Figure 2—figure supplement 2). (C) Heatmap showing the single-cell gene expression levels of top 15 differentially expressed genes (DEGs), based on log2-fold-change, between SPP1+MAM+ and SPP1+MAM- macrophages. Markers of SPP1+MAM+, tumour-associated macrophages (TAMs) (Mulder et al., 2021), lipid-associated macrophages (LAMs) (Jaitin et al., 2019), disease-associated microglia (DAM) (Keren-Shaul et al., 2017; Silvin et al., 2022), and scar-associated macrophages (SAMs) (Ramachandran et al., 2019) are indicated in the heatmap. (D) Scatterplot summarising the results of gene set enrichment analysis (GSEA) of differential expression between SPP1+MAM+ and SPP1+MAM- macrophages. For each significant pathway (FDR <0.05), x-axis indicates the normalised enrichment score (NES) and y-axis the significance of the enrichment; the size of each dot is proportional to the number of genes in the gene set. Metabolism-related pathways are coloured in blue, extracellular matrix (ECM) remodelling in red, and remaining terms in grey. (E) Bar plots summarising pathway enrichment of DEG upregulated in SPP1+MAM+ (left panel) or in SPP1+MAM- macrophages (right panel) using a matrisome-specific database (Naba et al., 2012). p-Values are calculated using the hypergeometric test and red line indicates a p-value of 0.05. Overlapping genes in the top-enriched pathways are displayed. (F, G) Violin plots showing the transcriptomic signature scores (y-axis) of SPP1+MAM+ ECM remodelling gene set (n=18 genes) (F), and SPP1+MAM+ metabolic processes gene set (n=27 genes) (G) in SPP1+MAM+ and SPP1+MAM- macrophages (left), and in other macrophage subsets with overlapping transcriptomic signatures (right). SPP1+MAM+, n=3840; SPP1+MAM-, n=20,265; TAMs, n=16,130; LAMs, n=61; DAM, n=5645; SAMs, n=415 cells. (H) UMAP dimensionality reduction of SPP1+ macrophages across all tissues, coloured by the disease status (left panel). Proportion of SPP1+MAM+ or SPP1+MAM- amongst SPP1+ macrophages from disease or healthy samples. The over-representation of SPP1+MAM+ cells in disease samples was evaluated by hypergeometric test and indicated. (I) Boxplot showing the percentage of SPP1+MAM+ across all macrophages, stratified by tissue and disease/healthy status. Lung: (control) n=29, (idiopathic pulmonary fibrosis [IPF]) n=40, (SSC) n=6; heart: (control) n=3, (ischemic cardiomyopathy [ICM]) n=4, (dilated cardiomyopathy [DCM]) n=7; skin: (control) n=13, (keloid) n=3, (SSC) n=39; endometrium: (control) n=11, (endometriosis) n=27; kidney: (control) n=7, (chronic kidney disease [CKD]) n=8, (acute kidney injury [AKI]) n=10. Unless otherwise indicated, a two-sided Wilcoxon rank-sum test was used to evaluate the significance of differences between groups.
-
Figure 2—source data 1
Marker genes for subclusters within SPP1+ macrophages.
- https://cdn.elifesciences.org/articles/85530/elife-85530-fig2-data1-v2.xlsx
-
Figure 2—source data 2
Signature genes of SPP1+MAM+ macrophages.
- https://cdn.elifesciences.org/articles/85530/elife-85530-fig2-data2-v2.xlsx
-
Figure 2—source data 3
GSEA analysis of pathways upregulated/downregulated in SPP1+MAM+ macrophages (relative to SPP1+MAM- macrophages).
- https://cdn.elifesciences.org/articles/85530/elife-85530-fig2-data3-v2.xlsx
-
Figure 2—source data 4
Signature genes of "ECM-remodeling processes" upregulated in SPP1+MAM+ macrophages.
- https://cdn.elifesciences.org/articles/85530/elife-85530-fig2-data4-v2.xlsx
-
Figure 2—source data 5
Signature genes of "metabolic processes" upregulated in SPP1+MAM+ macrophages.
- https://cdn.elifesciences.org/articles/85530/elife-85530-fig2-data5-v2.xlsx
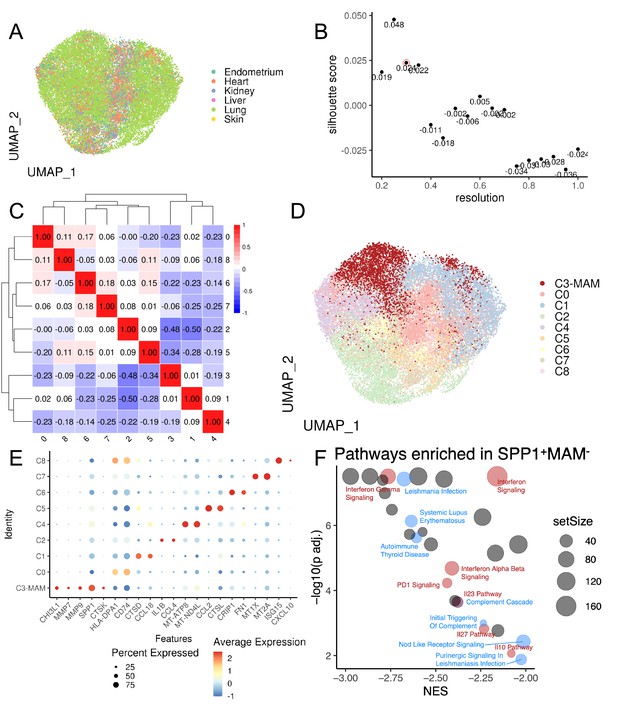
Data-driven clustering of SPP1+ macrophages identifies a matrisome-associated macrophage polarisation state.
(A) Uniform Manifold Approximation and Projection (UMAP) projection of SPP1+ macrophages, coloured by their tissue of origin. (B) Scatterplot of Silhouette score (see Materials and methods) of single-cell unsupervised Louvain clustering results at different resolutions, which dictates the granularity of clustering. The optimal clustering is determined from a resolution ≥ 0.3 which maximises the Silhouette score, corresponding to a resolution of 0.3 with a score of 0.024 (circled in red). (C) Heatmap showing Spearman correlation between the pseudo-bulk profiles of clusters derived by using the optimal resolution. (D) UMAP projection of SPP1+ macrophages, coloured by the cluster assignment. (E) Dotplot showing the gene expression of top 5 marker genes in SPP1+MAM+, denoted as C3-MAM here, and top 2 marker genes for remaining clusters based on log2-fold-change. (F) Scatterplot summarising the results of gene set enrichment analysis (GSEA) of differential expression between SPP1+MAM+ and SPP1+MAM- macrophages. For each significant pathway (FDR <0.05), x-axis indicates the normalised enrichment score (NES) and y-axis the significance of the enrichment; the size of each dot is proportional to the number of genes in the gene set. Inflammation-related pathways are coloured in blue, interferon/interleukin-related pathways in red, and remaining terms in grey.

Markers of SPP1+MAM+ as predicted by COMET.
Scatterplot ranks the number of occurrences of a gene in a four-marker panel of SPP1+MAM+ macrophages, as predicted by COMET (see Materials and methods). The Elbow method (dashed lines) was employed to select the top markers for SPP1+MAM+ macrophages, where a line connecting the first and the last point on the scatter plot was drawn, and the orthogonal distance of each point to the line was determined. This corresponds to seven markers, of which six genes are present in the SPP1+MAM+ signature (coloured in red, non-signature genes are coloured grey instead). The expression of these six genes in merged SPP1+ macrophages are then plotted for visualisation in Figure 2B.
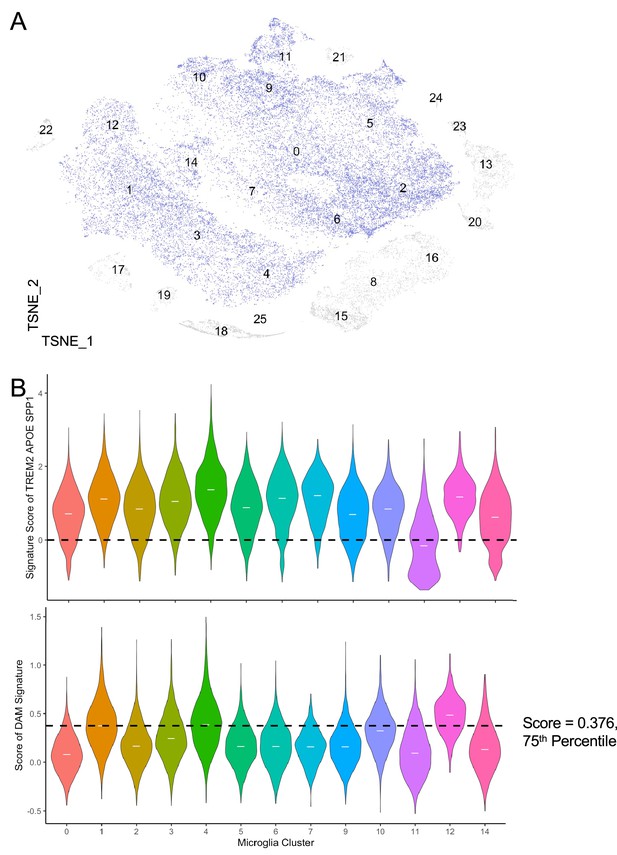
Derivation of disease-associated microglia (DAM) transcriptomic signature from the temporal lobe of epilepsy patients.
(A) t-SNE of immune cells isolated from the temporal lobe epilepsy patients (Kumar et al., 2022b; in the manuscript; n=4 patients). Microglial cells as defined in the original paper are coloured in blue; n=26,034 cells. (B) Violin plot displays the expression of TREM2, APOE, and SPP1 (top) and the DAM signature within the microglia (bottom). Median of the expression/score in each microglia cluster is indicated with a white line on the violin. Dashed line marks the DAM signature score at the 75th percentile by z-score (bottom). Clusters 1, 4, and 12 are classified as ‘DAM clusters’.

Derivation of scar-associated macrophages (SAMs) from human liver data.
(A) Uniform Manifold Approximation and Projection (UMAP) of human liver macrophages coloured by a SAM signature score defined using the genes SPP1, TREM2, and CD9. (B) Applying a z-score cutoff of 1.96 to the SAM signature score, SAMs (n=415 cells) were identified and coloured black here (left panel). UMAP of human liver macrophages with SPP1+MAM+ macrophages coloured in red (right panel). (C) UMAP of SPP1+ macrophages with SPP1+MAM+ macrophages coloured in red. (D) UMAP of SPP1+ macrophages, where each cell is coloured by expression of marker genes of SAMs (CD9, TREM2, and SPP1).
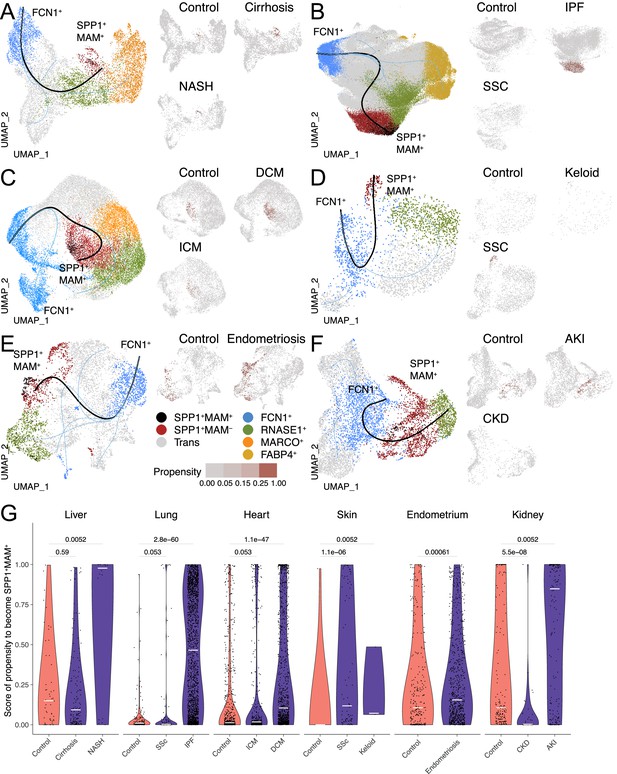
The differentiation trajectory of SPP1+MAM+ macrophages across human tissues.
(A–F) Slingshot differentiation trajectory analyses of macrophages in the liver (A), lung (B), heart (C), skin (D), endometrium (E), and kidney (F). The predicted trajectories are drawn on Uniform Manifold Approximation and Projection (UMAP) projection of macrophages, where the trajectory from FCN1+ monocytes to SPP1+MAM+ macrophages is indicated by a black line, and the one from FCN1+ monocytes to other clusters is indicated by blue lines. Macrophages are further stratified by disease and controls in each tissue and plotted separately on different UMAP, with each SPP1+MAM- macrophage coloured by its propensity to differentiate towards the SPP1+MAM+ state. To improve the visualisation of the propensity score of each cell across all tissues, we binned the score into four bins with different shades of blue colour. (G) Violin plots displaying the SPP1+MAM- macrophage propensity score towards the SPP1+MAM+ state in each tissue, separated by disease conditions. Number of cells is as follows, liver: (control) n=53, (cirrhosis) n=140, (nonalcoholic steatohepatitis [NASH]) n=18; lung: (control) n=167, (SSC) n=78, (idiopathic pulmonary fibrosis [IPF]) n=2,006; heart: (control) n=662, (ischemic cardiomyopathy [ICM]) n=421, (dilated cardiomyopathy [DCM]) n=1001; skin: (control) n=16, (keloid) n=3, (systemic sclerosis [SSC]) n=78; endometrium: (control) n=296, (endometriosis) n=735; kidney: (control) n=213, (chronic kidney disease [CKD]) n=40, (acute kidney injury [AKI]) n=110. Colour of violin delineates disease (purple) and control (orange). Unless otherwise indicated, a two-sided Wilcoxon rank-sum test was used to evaluate the statistical significance (p-value) of differences between two groups.

Monocle analysis recapitulates the conserved differentiation trajectory from FCN1+ monocytes to SPP1+ macrophages across multiple tissues.
Monocle differentiation trajectory analyses in the liver, lung, heart, skin, endometrium, and kidney (see Table 1 for information regarding the origin of human tissues and the number of sequenced cells). The predicted trajectories by Monocle are based on Uniform Manifold Approximation and Projection (UMAP) projection of monocyte/macrophages in each organ. The differentiation trajectories from FCN1+ monocytes towards SPP1+MAM+ macrophages are shown with a thick black line, and other trajectories are in purple.
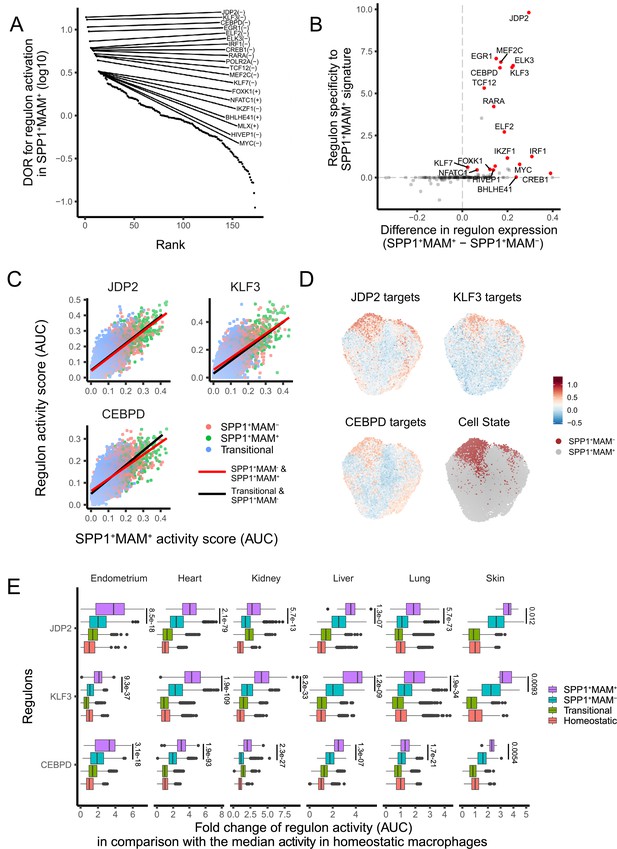
A core set of regulon activity associate with SPP1+MAM+ differentiation.
(A) Rank plot for the 173 regulons active in SPP1+MAM+ macrophages, ordered by the diagnostic odd ratio (DOR) (y-axis), which calculates the odds of the regulon being activated in SPP1+MAM+ cells over the odds of being activated in SPP1+MAM- macrophages (see Materials and methods). A positive DOR is associated with the regulon activation in SPP1+MAM+ macrophages. (B) Scatterplot showing the specificity of regulon genes to the SPP1+MAM+ signature genes (y-axis) against the difference in expression of a regulon between SPP1+MAM+ and SPP1+MAM- macrophages (x-axis). Briefly, the specificity scores the degree of overlap between regulon genes and the SPP1+MAM+ signature genes (see Materials and methods). (C) Scatterplot showing each cell’s activity score of selected regulons (y-axis) against the cell’s SPP1+MAM+ signature activity score (x-axis) across all tissues. Cells are coloured based on their identity: transitional, SPP1+MAM- and SPP1+MAM+ macrophages. For each regulon, separate linear regression models have been fitted for each trajectory: from the transitional to SPP1+MAM- macrophages (black regression line), and from SPP1+MAM- to SPP1+MAM+ macrophages (red regression line). (D) Uniform Manifold Approximation and Projection (UMAP) of SPP1+ macrophages coloured by the expression level of selected regulons, and by SPP1+MAM+ or SPP1+MAM- status. (E) Boxplots summarising the fold-change (FC) of regulon gene expression from SPP1+MAM+, SPP1+MAM-, transitional macrophages compared with regulon expression in homeostatic macrophages. Within each tissue, the ratio (FC) between the regulon activity score (area under the curve score [AUC]) in each cell from SPP1+MAM+, SPP1+MAM-, or transitional macrophage with respect to the median AUC in the homeostatic macrophages was calculated. The differences in FCs in regulon expression between SPP1+MAM+ macrophages and other macrophages (i.e., SPP1+MAM+ vs SPP1+MAM-, SPP1+MAM+ vs transitional macrophages, or SPP1+MAM+ vs homeostatic macrophages) were all statistically significant (adjusted p-value <0.05) after a two-tailed Wilcoxon rank-sum test. Adjusted p-value for comparisons between SPP1+MAM- and SPP1+MAM+ macrophages are indicated.
-
Figure 4—source data 1
Member genes of regulons listed in Figure 4D.
- https://cdn.elifesciences.org/articles/85530/elife-85530-fig4-data1-v2.xlsx
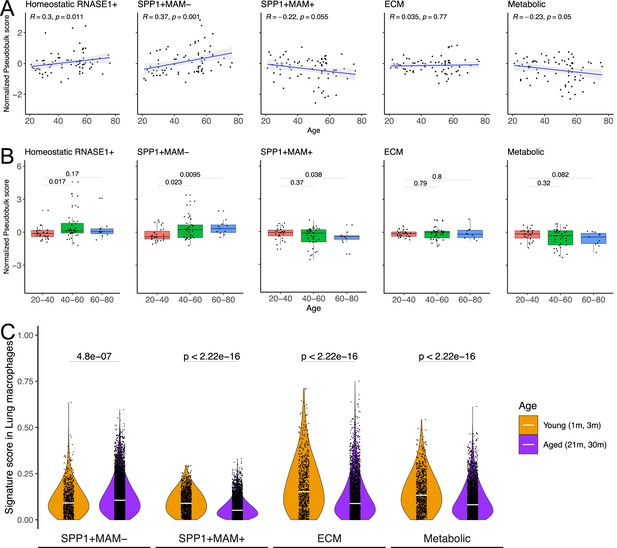
SPP1+MAM- gene signature is associated with ageing in mice and humans.
(A) Scatterplot evaluating the pseudo-bulk expression of the homeostatic RNASE1+, SPP1+MAM-, SPP1+MAM+, SPP1+MAM+ extracellular matrix (ECM) remodelling, and SPP1+MAM+ metabolic processes signatures (y-axis) in healthy human lung macrophages against age (x-axis) Here, for each sample, the pseudo-bulk expression signature was z-scaled after taking the median of the signature score from each cell. For each regression, the grey band around the line represents the 95% confidence interval of the regression line. Number of individuals = 85, number of cells = 86,484. (B) Boxplot summarising the pseudo-bulk expression of the homeostatic RNASE1+, SPP1+MAM-, SPP1+MAM+, SPP1+MAM+ ECM remodelling, and SPP1+MAM+ metabolic processes signatures in healthy human lung macrophages, stratified by age groups. (C) Violin plots summarising the SPP1+MAM-, SPP1+MAM+, SPP1+MAM+ ECM remodelling, and SPP1+MAM+ metabolic processes signatures in healthy mouse lung macrophages taken from Tabula Muris Senis (Almanzar et al., 2020), stratified into aged (21–30 months) or young mice (1–3 months). Aged, n=7878 cells, young, n=1529 cells. Unless otherwise indicated, two-tailed Wilcoxon rank-sum test was used to evaluate the statistical significance (p-value) of differences between the groups.
-
Figure 5—source data 1
Signature genes of SPP1+MAM- macrophages.
- https://cdn.elifesciences.org/articles/85530/elife-85530-fig5-data1-v2.xlsx
-
Figure 5—source data 2
Homeostatic signature regression model.
- https://cdn.elifesciences.org/articles/85530/elife-85530-fig5-data2-v2.xlsx
-
Figure 5—source data 3
SPP1+MAM- signature regression model.
- https://cdn.elifesciences.org/articles/85530/elife-85530-fig5-data3-v2.xlsx
-
Figure 5—source data 4
SPP1+MAM+ signature regression model.
- https://cdn.elifesciences.org/articles/85530/elife-85530-fig5-data4-v2.xlsx
-
Figure 5—source data 5
SPP1+MAM+ ECM signature regression model.
- https://cdn.elifesciences.org/articles/85530/elife-85530-fig5-data5-v2.xlsx
-
Figure 5—source data 6
SPP1+MAM+ Metabolic signature regression model.
- https://cdn.elifesciences.org/articles/85530/elife-85530-fig5-data6-v2.xlsx

Homeostatic macrophage signatures modelled against age and stratified by smoking status.
The homeostatic RNASE1+ signature, pseudo-bulk at the donor level, is further stratified according to the donor’s smoking status and linear regression is performed separately on each of the donor subsets.
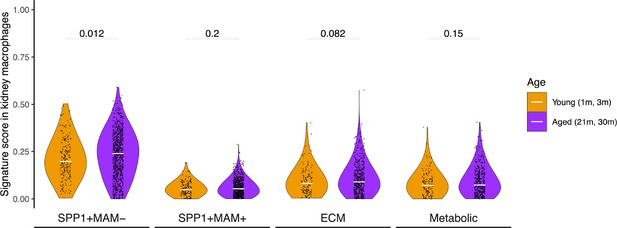
SPP1+MAM- signature is associated with ageing in mice kidneys.
Violin plots summarising the SPP1+MAM-, SPP1+MAM+, SPP1+MAM+ extracellular matrix (ECM) remodelling, and SPP1+MAM+ metabolic processes signatures in healthy mouse kidney macrophages taken from Tabula Muris Senis, stratified into aged (21–30 months) or young mice (1–3 months). Aged, n=2728 cells, young, n=736 cells. Unless otherwise indicated, two-tailed Wilcoxon rank-sum test was used to evaluate the statistical significance (p-value) between the groups.
Tables
Summary of scRNA-seq datasets analysed in this study.
For each study, the sample size (number of single-cell datasets), age/gender, disease status, the number (and percentage) of tissue resident macrophages (Mφ), and the total number of live cells sequenced are indicated. For studies that opted for positive or negative cell sorting methods (e.g., CD45+ cells), the total number of live cells is indicated with the cell isolation method. IPF: idiopathic pulmonary fibrosis, SSC: systemic sclerosis; DCM: dilated cardiomyopathy; ICM: ischemic cardiomyopathy; CKD: chronic kidney disease; AKI: acute kidney injury. 1The number of CD45+ cells has been derived from the dataset downloaded from GEO (otherwise this number has been provided in the original publication and reported here). 2Massive parallel single-cell RNA-sequencing (Mars-seq) was used to profile single-cell transcriptomics instead of 10X Chromium kit.
Tissue | Study(ref in article) | Repository | Disease status | Sample size (n) | Sex (%M) | Age (years)± SD | TissueMφ (%) | Total cells (#) |
---|---|---|---|---|---|---|---|---|
Liver | Ramachandran et al., 2019 | GSE136103 | Cirrhosis | 5 | 80.0 | 56.6±5.8 | 8332 (13.9%) | 60,094 CD45+ cells1 |
Normal | 5 | 60.0 | 57.4±7.9 | |||||
Liver | Fred et al., 2022 | Author provided data | NASH | 10 | 50.0 | 47.0±7.5 | 2069 (12.1%) | 17,154 |
Lung | Morse et al., 2019 | GSE128033 | IPF | 3 | 33.3 | 69.3±0.6 | 17,570 (39.3%) | 44,652 |
Normal | 4 | 50.0 | 38.3±20.6 | |||||
Lung | Reyfman et al., 2019 | GSE122960 | IPF | 4 | 75 | 66.5±5.0 | 32,136 (41.7%) | 77,079 |
Normal | 8 | 25 | 42.9±15.5 | |||||
SSC | 2 | 0 | 46.0±9.9 | |||||
Lung | Adams et al., 2020 | GSE136831 | IPF | 32 | 81.3 | 65.4±5.4 | 117,184 (37.4%) | 312,928 |
Normal | 17 | 58.8 | 44.4±18.9 | |||||
Lung | Valenzi et al., 2019 | GSE128169 GSE156310 | IPF | 1 | 100.0 | 68.0 | 17,407 (44.3%) | 39,252 |
SSC | 4 | 75.0 | 56.8±9.5 | |||||
Heart | Koenig et al., 2022 | GSE183852 | DCM | 5 | 60.0 | 50.0±19.2 | 3,922 (7.9%) | 49,665 |
Normal | 2 | 100.0 | 50.5±17.7 | |||||
Heart | Rao et al., 2021 | GSE145154 | DCM | 2 | 100.0 | 61.0±1.4 | 20,539 (30.0%) | 68,516 CD45+ cells |
ICM | 4 | 100.0 | 47.3±10.6 | |||||
Normal | 1 | 100.0 | 53.0 | |||||
Skin2 | Gur et al., 2022 | GSE195452 | Normal | 22 | 22.7 | 44.8±10.4 | 2456 (15.6%) | 15,700 CD45+ cells |
SSC | 55 | 5.4 | 50.1±13.2 | |||||
Skin | Deng et al., 2021 | GSE163973 | Keloid | 3 | 66.7 | 25.6±7.3 | 320 (0.7%) | 45,094 |
Normal | 3 | 66.7 | 31.0±7.0 | |||||
Endo- metrium | Tan et al., 2022 | GSE179640 | Endometriosis | 9 | 0.0 | 35.8±5.8 | 3079 (8.6%) | 35,941 |
Normal | 3 | 0.0 | 33.3±10.3 | |||||
Endo- metrium | Fonseca et al., 2023 | GSE213216 | Endometriosis | 22 | 0.0 | 34.1±7.6 | 2951 (1.8%) | 163,882 |
Normal | 8 | 0.0 | 37.9±10.1 | |||||
Kidney | Kuppe et al., 2021 | Zenodo 4059315 | CKD | 6 | 66.7 | 70.8±12.5 | 3596 (7.5%) | 48,096 CD10- cells |
Normal | 4 | 100.0 | 65.3±11.3 | |||||
Kidney | Lake et al., 2023 | atlas.kpmp.org | AKI | 11 | 72.7 | 50.2±19.1 | 1597 (3.5%) | 46,249 |
CKD | 15 | 66.7 | 61.9±12.7 | |||||
Normal | 14 | 50.0 | 45.9±10.3 | |||||
Kidney | Malone et al., 2020 | GSE145927 | AKI | 3 | 100.0 | 49.3±18.2 | 2772 (4.6%) | 60,080 |
Normal | 2 | 50.0 | 54.0±1.0 |