How hibernation in frogs drives brain and reproductive evolution in opposite directions
Abstract
Environmental seasonality can promote the evolution of larger brains through cognitive and behavioral flexibility but can also hamper it when temporary food shortage is buffered by stored energy. Multiple hypotheses linking brain evolution with resource acquisition and allocation have been proposed for warm-blooded organisms, but it remains unclear how these extend to cold-blooded taxa whose metabolism is tightly linked to ambient temperature. Here, we integrated these hypotheses across frogs and toads in the context of varying brumation (hibernation) durations and their environmental correlates. We showed that protracted brumation covaried negatively with brain size but positively with reproductive investment, likely in response to brumation-dependent changes in the socio-ecological context and associated selection on different tissues. Our results provide novel insights into resource allocation strategies and possible constraints in trait diversification, which may have important implications for the adaptability of species under sustained environmental change.
eLife assessment
In this important paper, the authors report a link between brumation (or "hibernation") and tissue size in frogs, summarizing convincing evidence that extended brumation is associated with smaller brain size and increased investment in reproduction-related tissues. The research is of broad interest to ecologists, evolutionary biologists, and those interested in global change biology, as the dataset involves significant field work and advanced statistical analyses for insights into how expensive tissues in these ectothermic animals respond to environmental seasonality.
https://doi.org/10.7554/eLife.88236.3.sa0Introduction
Seasonal food scarcity challenges animal energy budgets. A positive or less negative energy balance across seasons can be achieved by a more constant net energy intake than predicted solely by food abundance (Sol, 2009), or by investing less in costly organs (Heldstab et al., 2018). A link between both strategies is the brain: A relatively large brain can improve cognitive ability and behavioral flexibility (Benson-Amram et al., 2016; Lefebvre et al., 2004; Reader and Laland, 2002; Sol et al., 2005), enabling animals to effectively locate diverse and dispersed food sources to buffer environmental fluctuations in seasonal habitats (cognitive buffer hypothesis) (Allman et al., 1993; Sol, 2009; van Woerden et al., 2012). However, brain tissue has high metabolic costs (Aiello and Wheeler, 1995; Lukas and Campbell, 2000; Mink et al., 1981) that may not be temporarily reducible (Mink et al., 1981), constraining brain size evolution under periodic food scarcity (expensive brain hypothesis) (Isler and van Schaik, 2009).
Food scarcity can also affect physiological responses. For example, a longer digestive tract may permit more efficient resource uptake during a short active period and be favored by selection in species with prolonged hibernation (Sibly, 1981). The evolution of the digestive tract could thus parallel that of the brain. In contrast, there could be an evolutionary trade-off between the two organs (‘expensive tissue hypothesis’; Aiello and Wheeler, 1995; also see Isler and van Schaik, 2006 for a more general ‘energy trade-off hypothesis’). Furthermore, physiological buffering often involves a seasonal reduction in metabolic rate or activity (e.g., hibernation), with energy drawn from stored fat reserves (Heldstab et al., 2016). Buffering lean periods by fat stores and reduced activity contrasts with cognitive abilities to cope with food scarcity, suggesting differential investment in adipose and brain tissue between species in highly seasonal environments (‘fat−brain trade-off hypothesis’; Heldstab et al., 2016; Navarrete et al., 2011). Finally, allocating fat stores to sustained brain function throughout prolonged hibernation might compete with investments in other tissues (Isler, 2011; Isler and van Schaik, 2009).
Species that breed soon after hibernation, such as some mammals (Place et al., 2002; Psenner, 1957) and many amphibians (Fei and Ye, 2001; Wells, 1977), face further unique challenges, as their reproductive tissue must regrow before emergence when stored resources are most limited (Isler, 2011; Isler and van Schaik, 2009). Testes, however, may be subject to intense selection by sperm competition resulting from female multiple mating (Lüpold et al., 2020), a widespread phenomenon where males cannot monopolize their mates (Lüpold et al., 2014). Indeed, across anurans (frogs and toads) that often breed in small water bodies, males invest relatively more in their testes and less in their forelimbs (used in pre-mating competition) as population density increases (Buzatto et al., 2015; Lüpold et al., 2017). If a shorter active period leads to more synchronized breeding and thus increases the risk of sperm competition or sperm depletion (Vahed and Parker, 2012), selection for relatively larger testes would be stronger where fat stores need to last longer, affecting resource demands and allocation during hibernation. Including reproductive investments and breeding patterns in studies of allocation trade-offs in response to hibernation and environmental seasonality would thus seem critical but remains to be done, particularly in the context of brain evolution.
The opposing selection pressures on brain or gonad size (i.e., cognitive or fitness benefits versus metabolic costs), varying degrees of seasonality and diverse strategies for buffering periodic food scarcity (e.g., cognitive versus physiological) between species render environmental fluctuations an ideal context for studying brain size evolution. The different hypotheses for the coevolution of brain size with other organs were developed separately for different mammalian or avian taxa, the two vertebrate classes with the largest brains relative to body size (Jerison, 1973). These hypotheses have yet to be directly tested against one another in a single taxon and ideally in the immediate context of seasonal activity, considering the extent, rather than the mere presence/absence, of hibernation. This last point is important because ‘hibernation’ can range between brief inactive bouts and long dormancy, with different energetic and life-history constraints. Furthermore, understanding the generality of the patterns reported in mammals or birds needs to be validated in other taxa, ideally with smaller brains and different energy demands. Such a generalization would help contextualize brain evolution in the two largest brained taxa in relation to selection on encephalization and metabolic constraints.
A particularly suitable system is presented in ectothermic (cold-blooded) species whose metabolism and activity are tightly linked to ambient temperature (Wells, 2007), possibly leading to stricter physical boundaries to behavioral flexibility than in the endothermic (warm-blooded) mammals or birds. Compared to the divergent groups of mammals, for which most hypotheses on brain evolution have been proposed, anurans are also more homogeneous in body size and shape, diet, and locomotion (Kardong, 2019), while still showing strong environmental effects on brain, reproductive, and other investments (Liao et al., 2022; Lüpold et al., 2017; Wells, 2007). Being less influenced by Bauplan and lifestyle, evolutionary trade-offs with brain size could thus be easier to isolate in anurans than in mammals.
Here, we studied across 116 anuran species how varying ‘hibernation’ periods and their environmental correlates affect male investments in brain, testes, body fat, limb muscles, and the main visceral organs (see Figure 1). Unlike mammals, anurans do not actively depress their metabolism but rather stop activity when ambient temperatures drop below the activity range (referred to here as ‘brumation’ for distinction; Pinder et al., 1992; Wells, 2007). However, in both taxa, hibernators use fixed energy stores across sequential investments, albeit at a lower metabolic rate (Staples, 2016), while non-hibernators can partly replenish resources but need more food even when it is scarce (Heldstab et al., 2016). These contrasting strategies may be associated with a varying cost–benefit balance between organs, including the brain.
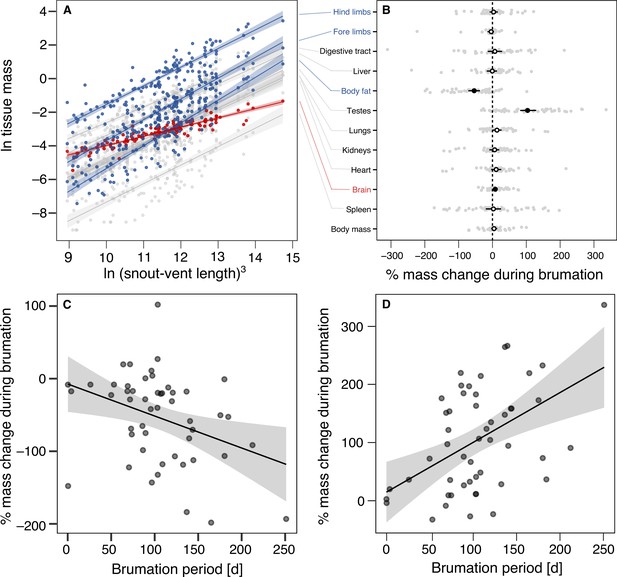
Allometric and seasonal variation in the species-specific tissue masses.
(A) Allometric slopes between the mass of each tissue and cubed snout-vent length (SVL3) so that proportionate scaling follows a slope of 1 on a log–log scale. Each point represents a species-specific mean value in breeding condition (N = 16). Relationships deviating from proportionate scaling (based on bootstrapped 95% confidence intervals) are highlighted in blue (steeper than unity) or red (shallower than unity). (B) Mean percent change with 95% confidence interval for body mass and each individual tissue of 50 anuran species with data from both shortly before and after brumation (=breeding), based on absolute tissue masses between stages and log-transformed to maintain symmetry and additivity (Törnqvist et al., 1985): log(post-brumation/pre-brumation) × 100. The transparent gray dots depict species-specific values. (C) Relationship between brumation period and percent mass change in the amount of body fat. (D) Relationship between brumation period and percent mass change in testis mass. Each point indicates a species.
Although anurans halt general activity during brumation, this does not mean complete brain inactivity. Several species move in their burrows or underwater hibernacula in response to changes in soil temperature or oxygen concentration (Holenweg and Reyer, 2000; Stinner et al., 1994; van Gelder et al., 1986), or when disturbed (Niu et al., 2022; Tattersall and Ultsch, 2008). Moreover, brumating frogs can renew more brain cells than active frogs, possibly to prevent brain damage (Cerri et al., 2009), and this could increase with brain size. Larger brains could also be less tolerant to low oxygen conditions (Pinder et al., 1992; Tattersall and Ultsch, 2008; Wells, 2007) if the findings from other ectotherms extend to anurans (Sukhum et al., 2016). Overall, maintaining a relatively larger brain while brumating may entail higher costs that could constrain brain evolution compared with species with short or no seasonal inactivity.
Instead of the typical indirect proxies or scores (Heldstab et al., 2018; Heldstab et al., 2016; Luo et al., 2017; Navarrete et al., 2011), we quantified seasonal changes in tissue size and estimated brumation duration as the time that temperatures were continuously below the species-specific activity threshold. These estimates aligned with periods of inactivity recorded during field surveys, and were robust across multiple sensitivity analyses (e.g., considering temperature buffering by underground burrows). We then tested the different hypotheses on brain evolution and further integrated variation in breeding context and reproductive evolution in this resource allocation framework. By examining ectothermic species with diverse energy demands and brain sizes, our findings provide a crucial context for understanding brain evolution and resource allocation.
Results
Determinants of brumation duration
For a mean (± standard deviation) of 3.41 ± 0.95 males in each of 116 anuran species, we combined experimentally determined thermal activity thresholds with multi-year temperature fluctuations at their collection sites to estimate the species-specific brumation periods (details on methodology and various sensitivity analyses for validation in Materials and methods). These periods averaged between 0.6 ± 0.5 and 250.5 ± 16.7 days across the 5 years examined, with high repeatability within species (R = 0.95 [95% confidence interval, CI: 0.93, 0.96]; Appendix 1—figure 1; Appendix 1—table 1). These brumation periods largely fell into three groups with ≤9 (N = 22 species), 18–27 (N = 3), and ≥47 days of expected brumation (N = 91), respectively. As the ground microclimate may buffer some of the fluctuations in air temperature and frogs can endure short cold spells without dormancy, we conservatively considered those 25 species with ≤27 days below their experimental temperature threshold unlikely to show any sustained brumation.
We employed phylogenetic generalized least-squares (PGLS) models (Freckleton et al., 2002; Ho and Ané, 2014) to dissect the factors driving brumation duration. Our analyses indicated that the brumation period increased with the latitude and elevation of study sites, along with the extent of annual temperature fluctuations (r ≥ 0.42, t114 ≥ 5.00, p <0 .001; Appendix 1—table 2). In contrast, brumation duration was inversely correlated with the annual mean temperature and precipitation, and dry season length (r < −0.29, t114 < −3.24, p < 0.002), but not significantly covarying with longitude or annual fluctuations in precipitation (r <0 .09, t114 < 0.95, p > 0.34; Appendix 1—table 2). Except for the duration of the dry season, these results persisted for those 91 species with a high probability of sustained brumation (see above; Appendix 1—table 3). Among these species, those from cooler and more seasonal climates exhibited lower entry and emergence temperatures from their inactive state (Appendix 1—table 4), suggesting heightened cold tolerance to maximize their active period.
Impact of brumation on tissue investments
To investigate the repercussions of brumation on individual tissue investments, we used the same males from our 116 species. In separate sets of PGLS models, neither snout-vent length (SVL) nor body mass covaried with brumation duration or any other environmental variable (|r| ≤ 0.15, |t114| ≤ 4.03, p ≥ 0.11; Appendix 1—table 5), except for a weak, non-statistically significant trend toward reduced body mass at higher elevations (r = −0.18, t114 = −1.91, p = 0.06, phylogenetic scaling parameter λ = 0.94 [95% CI: 0.85, 1.00]). The different tissues increased with body size, albeit with different allometric slopes. Body fat and limb muscles showed steeper scaling (all β ≥ 0 [1.03, 1.18]), brain size exhibited a shallower slope (β = 0.49 [0.44, 0.54]; Appendix 1—table 6; Figure 1A), and the remaining tissues did not deviate from proportionate scaling (i.e., 95% CI including 1.00; Appendix 1—table 6; Figure 1A). Hence, the evolution of brain size appears to be more constrained than that of other organs when selection favors larger body size.
Given these distinct allocation patterns between brain and other tissues, we next tested whether extended brumation constrains brain size evolution, akin to suggestions for mammals based on presence/absence of hibernation (Heldstab et al., 2018). In PGLS models, absolute brain size was independent of brumation duration (r = −0.10, t115 = −1.08, p = 0.28, λ = 0.89 [0.73, 0.97]), but accounting for SVL as a body size proxy, species with prolonged brumation had relatively smaller brains, quantified during breeding (partial r, rp = −0.31, t113 = −3.50, p < 0.001, λ = 0.35 [0.00, 0.61]; Figure 2A, Appendix 1—table 7) or pre-hibernation (N = 50 species means based on 2.64 ± 0.94 males each: rp = −0.51, t47 = −4.03, p < 0.001, λ = 0.00 [0.00, 0.56]; Appendix 1—table 7). This trend was distinct from body size changes in response to brumation, as SVL remained independent of brumation duration (Appendix 1—table 5). On average, brumating species tended to possess relatively smaller brains compared to those less likely to experience prolonged brumation (Appendix 1—table 8), aligning with mammalian studies utilizing hibernation presence/absence (Heldstab et al., 2018). This trend extended to those 91 species categorized as brumating for some period (Appendix 1—table 9), reinforcing the link between brumation and brain evolution beyond coarse binary classification. This pattern further persisted when recalculating brumation periods using conservative thresholds of 2 or 4°C below their experimentally derived thresholds, simulating shelter buffering (details and validation in Materials and methods; Appendix 1—table 10).
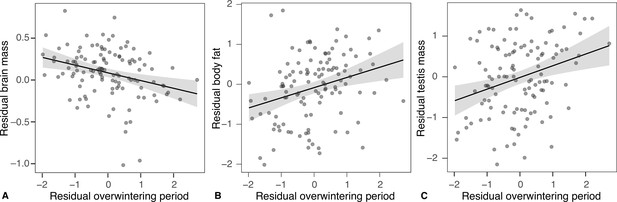
Effects of brumation duration on the relative tissue sizes.
Relationships between brumation duration and the relative mass of the brain (A), body fat (B), and testes (C) across males of 116 anuran species in breeding (post-brumation) condition. All axes are controlled for the snout-vent length and phylogeny.
Species displaying extended brumation periods further exhibited relatively more body fat (rp ≥ 0.25, t113 ≥ 2.72, p ≤ 0.008; Figure 2B) and, particularly during breeding, had relatively larger testes (rp = 0.36, t113 = 4 .06, p < 0.001, λ = 0.77 [0.40, 0.90]; Figure 2C), along with relatively smaller hindleg muscles (rp = −0.22, t113 = −2.37, p = 0.02, λ = 0.22 [0.00, 0.51]; Appendix 1—table 7). These patterns generally held true when using the presence/absence of brumation (Appendix 1—table 8), buffered temperature fluctuations (Appendix 1—table 10), or excluding the 25 species unlikely to brumate (except for the non-significant effect on body fat; Appendix 1—table 9). Other tissue sizes remained independent of brumation (Appendix 1—Tables 7–10) and showed not significant changes between sampling periods (Figure 2).
Comparing pre- and post-brumation males, averaged across all species, we found a roughly 50% reduction in fat tissue and a 100% increase in testis size, indicating resource depletion and testicular regrowth during brumation, respectively (Figure 1B). Only brain size deviated from zero among the remaining tissues, but this change was minimal compared to the body fat and testes, and within the range of many unchanged tissues. Thus, the biological significance of this putative increase in brain size during brumation remains uncertain, possibly reflecting general differences between sampled individuals. We will thus refrain from further interpretation. Among the two tissues with considerable change, the extent of fat depletion increased significantly with the brumation period (r = −0.36, t48 = −2.68, p = 0.01, λ = 0.00 [0.00, 0.55]), as did that of testis regrowth (r = 0.39, t48 = 2.89, p = 0.006, λ = 0.82 [0.16, 0.98]; Figure 1C).
To examine whether the increase in relative testis size with prolonged brumation might be mediated by a shorter, more synchronized mating season (Wells, 2007), we tested for links between brumation duration and different breeding parameters. Prolonged brumation notably shortened the breeding season (r = −0.57, t41 = −4.47, p < 0.001, λ = 0.00 [0.00, 0.38]; Appendix 1—figure 2A), exerting a stronger effect than climatic variables (Appendix 1—table 11), particularly when considered together (Appendix 1—table 12). Hence, the effect of these climatic variables may be mediated by brumation. Furthermore, a shorter breeding season increased the probability of dense breeding aggregations (phylogenetic logistic regression: N = 42, z = −3.03, p = 0.002, α = 0.02; Appendix 1—figure 2B). That brumation might mediate climatic impacts on breeding aggregations through the duration of the breeding season was also supported by a phylogenetic confirmatory path analysis (Gonzalez-Voyer and Hardenberg, 2014; von Hardenberg and Gonzalez-Voyer, 2013; Appendix 1—figure 3; Appendix 1—table 13). Finally, when combining these data with published data on the density of breeding populations (Lüpold et al., 2017; N = 8 species overlapping), a trend emerged toward higher mean population densities in species with shorter breeding seasons (r = −0.69, t6 = −2.37, p = 0.06, λ = 0.00 [0.00, 1.00]), albeit with a small sample size (Appendix 1—figure 2C).
To explore possible causal links between breeding parameters and relative testis size, we employed directional tests of trait evolution (Pagel, 1994; Revell, 2012), assessing whether changes in two binary traits are unilaterally or mutually dependent, or independent (Pagel, 1994). To this end, we converted our continuous to binary variables (see Materials and methods). Relating small/large testes to short/long breeding seasons, the best-supported scenario based on the Akaike information criterion (AIC) was independent evolution. Yet, the model with changes in relative testis size dependent on those in the breeding season found similar support (ΔAIC = 0.83, wAIC = 0.33 compared to independent model with wAIC = 0.50; Appendix 1—figure 4A), differing from the remaining two models with clearly higher AIC scores (ΔAIC ≥ 3.40, wAIC ≤ 0.09). In another directional test, between relative testis size and aggregation formation, the independent model was the best upported (wAIC = 0.66), followed by the scenario of increased relative testis size in response to aggregation formation (Appendix 1—figure 4), albeit above a ΔAIC cut-off of 2 (ΔAIC = 2.38, wAIC = 0.20), with the remaining models being clearly less supported (ΔAIC ≥ 3.64, wAIC ≤ 0.11). Hence, it is at least possible that breeding conditions mediate the positive relationship between brumation duration and relative testis size in our relatively small sample of species, while a response of breeding conditions to variation in relative testis size is clearly rejected by our analyses.
Covariation between tissues
As all tissues rely on the same finite resources, their responses to brumation are likely interconnected. Pairwise partial correlations, controlling for SVL and phylogeny, revealed positive covariation or non-significant associations among all tissue masses (Appendix 1—figure 5). As such, our data do not support the expensive tissue (Aiello and Wheeler, 1995) or the more general energy trade-off hypotheses (Isler and van Schaik, 2006), which predict brain size trade-offs with the digestive tract or other costly organs, respectively. However, since brain size differed from fat, hindlimb muscles and testes in allometric relationships and responses to brumation, pairwise correlations may not capture more complex allocation. To examine the relative investments in these four most informative tissues simultaneously, we represented total body mass as proportions of these tissues and the remaining mass, generating a five-variable compositional dataset (van den Boogaart and Tolosana-Delgado, 2013). The combined mass of all four focal tissues scaled proportionately with body size (allometric β = 1.02 [0.96, 1.07], λ = 0.01 [0.00, 0.11]), confirming size-independent proportion of the total resources allocated to the four focal tissues combined. However, the resource distribution among these tissues varied considerably between species. Pairwise correlations between the four focal tissues, transformed to centered log ratios (van den Boogaart and Tolosana-Delgado, 2008) and controlling for phylogeny, showed brain mass to covary negatively with fat and testis mass, while testis mass covaried negatively with hindlimb muscle mass but not with body fat (Figure 3A–D; Appendix 1—table 14).
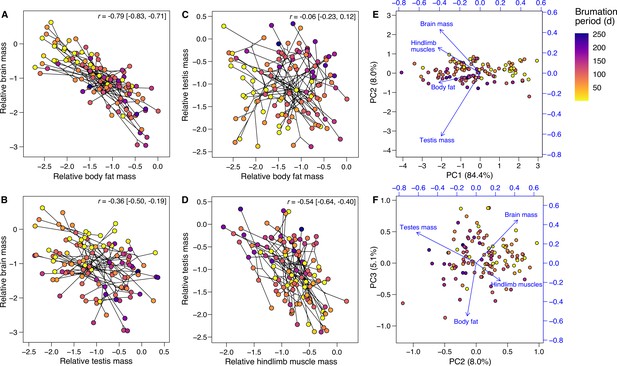
Effects of brumation duration on the relative tissue sizes.
Panels (A–D) depict the phylogenetic correlations (shown as phylomorphospace plots; Revell, 2012) between the relative masses of (A) brain and body fat, (B) brain and testes, (C) testes and body fat, and (D) testes and hindlimb muscles, respectively, across the 116 species (results in Appendix 1—table 14). The relative tissue masses represent the centered log ratios of the compositional data, and the lines connect the nodes of the underlying phylogeny, indicating that phenotypic correlations are not simply the result of phylogenetic clustering. The correlation coefficients and 95% confidence intervals are indicated. The loadings from a phylogenetic principal component analysis (Revell, 2012) on the same variables are also mapped as vectors onto biplots between (E) the first and second or (F) the second and third principal components. In all panels, the point colors reflect the species-specific brumation periods (see legend in panel A). Generally, where brumation was relatively shorter or absent, species also tended to have relatively larger brains, less body fat and smaller testes, respectively, consistent with the univariate analyses (Figure 2).
To further examine the effect of brumation duration on all five variables simultaneously, we conducted a phylogenetic multivariate regression analysis (Clavel et al., 2015) on the same compositional data, but now transformed to isometric log ratios as recommended for multivariate models (van den Boogaart and Tolosana-Delgado, 2013). Brumation duration significantly affected anuran body composition (Pillai’s trace = 0.33, effect size ξ2 = 0.30, p = 0.001). The back-transformed coefficients of body fat (0.23) and testis mass (0.26) exceeded the expected coefficient of 0.20 if brumation had no effect, while brain mass, hindlimb muscles, and the rest of the body had lower coefficients (0.16, 0.17, and 0.18, respectively; also see Figure 3).
Finally, a phylogenetically informed principal component analysis (Revell, 2012) confirmed the negative associations of brain size with body fat and testis mass, and that of testis mass with hindlimb muscles (Figure 3E, F, Appendix 1—table 15). Here, the first three principal components (PC1 to PC3) explained 84.7%, 8.0%, and 5.1% of total variance, respectively. Although PC2 and PC3 explained a relatively small proportion of the total variance, they separated the different tissues. PC2 loaded mainly by brain size (0.30) and testis mass (−0.44), and PC3 by brain size (0.26) and body fat (−0.31). Biplots indicated negative associations between the vectors of the same traits as above within the multivariate trait space (Figure 3F). Furthermore, brumation duration covaried negatively with PC2 (r = −0.53, t114 = −6.59, p < 0.0001, λ = 0.74 [0.49, 0.92]), consistent with reduced brain size and increased in testis mass toward longer brumation, but it was not associated with PC3 (r = −0.12, t114 = −1.30, p = 0.20, λ = 0.41 [0.00, 0.70]).
Direct and indirect effects revealed by path analysis
To untangle the evolutionary links between tissue sizes, and to test competing brain evolution hypotheses in the context of brumation, we conducted a phylogenetic confirmatory path analysis (Gonzalez-Voyer and Hardenberg, 2014; von Hardenberg and Gonzalez-Voyer, 2013) with 28 predetermined path models (Appendix 1—figure 6; Appendix 1—table 16). The averaged model (Figure 4) confirmed the negative effect of prolonged brumation on relative brain size (β = −0.15 [−0.22, −0.07]), along with direct (β = 0.16 [0.07, 0.26]) and indirect positive effects on relative testis size (Appendix 1—figure 7). These effects on testis size were mediated by the relative amount of adipose tissue, which increased with brumation duration (β = 0.13 [0.06, 0.20]) and digestive tract size (β = 0.51 [0.37, 0.65]), and in turn positively influenced relative testis size (β = 0.39 [0.18, 0.59]).
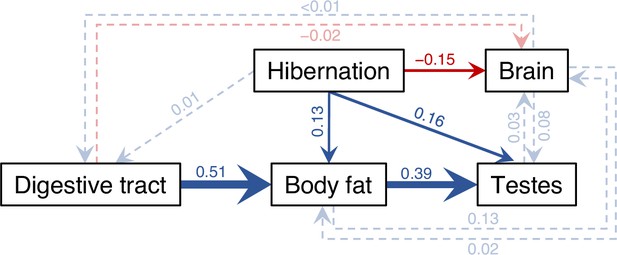
Results of the averaged phylogenetic path model.
Visual representation of the average phylogenetic path model across 116 anuran species. Arrows reflect the direction of the path, with their widths being proportional to the standardized regression coefficients and colors indicating the sign (blue = positive, red = negative). Paths with 95% confidence intervals (CIs) excluding 0 (i.e., arrows highly probable) are drawn as solid arrows, all others as dashed, semi-transparent arrows. For simplicity and to avoid overparameterization, other organs were omitted in path models as they showed little covariation with brumation duration or brain size. All phenotypic traits were log-transformed, and all variables were controlled for body size via additional paths from log SVL. Although snout-vent length (SVL) had a strong effect on all variables (all β > 0.37), its thick blue arrows to each box are omitted in this figure only for visual clarity, but all path coefficients are presented with their 95% CI in Appendix 1—figure 7, with further details in Appendix 1—figure 6 and Appendix 1—table 6.
Discussion
Our study on anurans, with validated brumation periods and direct measures of expensive tissues, provides novel insights into brain and reproductive evolution in ‘cold-blooded’ organisms exposed to environmental seasonality. We found that species with longer brumation exhibited relatively smaller brains and allocated greater fat reserves primarily in reproduction, possibly due to the shorter breeding season with its socio-ecological implications.
Environmental and resource considerations in brain evolution
We demonstrated that species in cooler and more seasonal climates were more cold tolerant, thereby likely optimizing their active period. Yet, low temperatures impair foraging and digestion in ectotherms (e.g., Fontaine et al., 2018; Riddle, 1909), such that high seasonality may lead to longer brumation and smaller brains. These results confirm that, unlike birds (Sol, 2009) and some mammals (van Woerden et al., 2010), challenging and unpredictable environmental conditions select for physiological rather than cognitive buffering in anurans (Luo et al., 2017). Supporting a large brain may not be sustainable without continued resource intake, or larger brains could be less tolerant to hypoxic conditions during brumation (Sukhum et al., 2016). However, selection for relatively larger brains may also simply be stronger in species with longer active (and short brumation) periods owing to extended cognitive benefits such as predator evasion (Kotrschal et al., 2015; Liao et al., 2022) or exploitation of better and more diverse food sources (Jiang et al., 2023; Lefebvre et al., 1997).
In pairwise comparisons, the relative sizes of the tissues examined here, including the brain, were generally positively correlated. These results reject both the expensive tissue and energy trade-off hypotheses (Aiello and Wheeler, 1995; Isler and van Schaik, 2006), which predict trade-offs of brain size with the size of the digestive tract or other costly organs, respectively. This lack of support in anurans aligns with a previous report in mammals (Navarrete et al., 2011) despite their smaller brains and vastly different ecology and physiology, including a lower metabolic rate and largely lacking physiological thermoregulation. When focusing jointly on the four tissues (brain, body fat, testes, and hindlimb muscles) that covaried with brumation duration, however, relative brain size covaried negatively with the relative mass of both fat tissue and testes, supporting the fat−brain trade-off (Navarrete et al., 2011) or expensive sexual tissue hypotheses (Pitnick et al., 2006), respectively.
Brumation and fat tissue
Species with longer brumation periods exhibited relatively more total body fat and a higher degree of its depletion, supporting the hypothesis that anurans buffer lean periods by metabolizing stored fat (Huang et al., 2020; Luo et al., 2017). Although adipose tissue may not itself be metabolically expensive, transporting it adds costs to locomotion, particularly when jumping away from predators (Moreno-Rueda et al., 2020) or climbing trees compared to moving horizontally on land or in water (Alexander, 2003; Hanna et al., 2008). Consistent with this notion, arboreal species tended to be leaner compared to (semi)aquatic or terrestrial species (Appendix 1—table 17), controlling for brumation duration and relative brain size, both of which we had shown to covary with body fat (Figures 2 and 3).
Brumation and testis evolution
Species with prolonged brumation also had relatively smaller hindleg muscles and larger testes. The negative relationship between hindleg muscle mass and brumation duration may be linked to more movement during a longer active period, including predator evasion (Liao et al., 2022; Marchisin and Anderson, 1978). Larger testes may result from a shorter breeding season, leading to denser and more synchronous mating activity (Wells, 2007), as suggested by our path analysis. Breeding aggregations increase male–male competition over fertilization and thus enhanced investments in sperm production (Liao et al., 2018; Lüpold et al., 2020). Our results thus reveal how brumation patterns, influenced by environmental variation and physical constraints, affect the socio-ecological context of breeding, the mode and degree of sexual selection, and ultimately the evolution of mating systems, broadening Emlen and Oring, 1977 general predictions.
In addition to the average size of the testes, their seasonal change also varied with the brumation period. Seasonally breeding anurans regress and regrow their testes between mating seasons (Ogielska and Bartmańska, 2009). Non-brumating species can use energy uptake to compensate for testicular recrudescence, while those with a short breeding season after a prolonged inactive period depend on the stored fat to regrow their testes before or immediately after emergence from their hibernaculum. Hence, resources are diverted away from the brain and other organs, especially in species such as Brachytarsophrys spp., in which the fully developed testes combined weigh 12–14 times more than the brain Source data 1.
Brain–testis trade-off
A phylogenetic path analysis confirmed the negative effect of brumation duration on relative brain size and revealed its direct and indirect positive effects on relative testis size, mediated by the amount of adipose tissue, which responded to variation in the inactive period (energetic demand) and the size of the digestive tract (energy uptake). That body fat did not contribute to brain size evolution in this more comprehensive analysis compared to pairwise correlations suggests that the fat−brain trade-off may not be direct. Rather, longer brumation, and thus a short active period, may enhance selection on fat storage for testicular investments in addition to starvation avoidance, while reducing selection for larger brains due to a shifted balance between cognitive benefits and energetic costs (Figure 3).
A brain–testis trade-off has been reported for bats (Pitnick et al., 2006), but not replicated later in the same (Dechmann and Safi, 2009) or other mammalian taxa (Lemaître et al., 2009). In anurans, the apparent trade-off may result indirectly from opposing selection on brain and testis sizes via environmental seasonality and relative durations of the active and inactive periods. The testes may evolve in response to increased sperm competition and depletion during the shorter and more synchronized breeding season. The brain, while also responding to sexual selection (Mai et al., 2020), is central to various activities, including feeding (Lefebvre et al., 1997) or predator avoidance (Kotrschal et al., 2015; Liao et al., 2022) that are themselves subject to climatic conditions and may independently influence brain evolution. Additionally, whereas testes can regress to save energy when inactive (Ogielska and Bartmańska, 2009), brain metabolism may be less reducible (Mink et al., 1981), resulting in different cost–benefit balances between these organs in relation to seasonality.
Conclusions
In conclusion, our analyses show how brumation in anurans, influenced by high environmental seasonality, may affect resource allocation between costly tissues, directly or through its environmental correlates. The non-independent selective processes promoting diversification in different traits emphasize the need to study the evolutionary trajectory of a given trait such as brain size in the immediate context of both simultaneous investments to other tissues and the species-specific ecology. Our findings also have important implications in the context of sustained environmental change, exacerbated by climate change with its effects on temperature and precipitation patterns around the globe (IPCC, 2022). Such climatic shifts may disrupt the timing of brumation and breeding in many anurans, change their exposure to pathogens or predators through modified activity patterns, affect their ability to find food or suitable breeding sites, or change their population dynamics through resource availability, intra- and interspecific competition, dispersal capabilities, and gene flow (Alves-Ferreira et al., 2022; Blaustein et al., 2010; Blaustein et al., 2001; Carey and Alexander, 2003). In species with temperature-dependent sexual differentiation, thermal shifts may further change operational sex ratios in breeding populations and thus likely mating dynamics and sexual selection (Eggert, 2004; Lüpold et al., 2017; Ruiz-García et al., 2021). All these factors impose intense environmental pressure on resource acquisition and allocation patterns, and it remains to be seen to what extent variation in the adaptability, and thus resilience, between species exposed to environmental change is attributable to such competing needs between investments and species-specific constraints.
Materials and methods
Sample collection and preparation
Request a detailed protocolBetween 2010 and 2020 and as part of concurrent studies, we collected a total of 396 sexually mature males from 116 anuran species (3.41 ± 0.95 males each) in post-brumation breeding condition and an additional 132 adult males from 50 of these species (2.64 ± 0.94 males each) shortly before entering their hibernacula (Source data 1 and Source data 2). For each species, we sampled all males at a single location in southern and western China with known longitude, latitude, and elevation (Source data 3). Upon transfer to the laboratory, we sacrificed the individuals by single-pithing, measured their SVL to the nearest 0.01 mm with calipers and then preserved them in 4% phosphate-buffered formalin for tissue fixation.
After 2 months of preservation, we weighed each complete specimen to the nearest 0.1 mg using an electronic balance to obtain body mass before dissecting them following a strict protocol. We separately extracted the brain, heart, liver, lungs, kidneys, spleen, digestive tract, testes, limb muscles, and fat stores, cleaned these tissues and immediately weighed them to the nearest 0.1 mg with an electronic balance. We additionally measured the length of the digestive tract to the nearest 0.01 mm using calipers. We excluded emaciated individuals or those exhibiting visible organ pathologies from our analyses.
Environmental seasonality
Request a detailed protocolFor each collection site, we retrieved from the 30-year climate history of https://www.meteoblue.com the monthly mean temperature (in °C) and total precipitation (in mm) (Source data 3) and used these values to calculate location-specific annual means and coefficients of variation. We also determined the duration of the dry season, P2T, as the number of months, for which the total precipitation was less than twice the mean temperature (Walter, 1971).
Brumation period
Request a detailed protocolOne way that anurans can physiologically respond to seasonality is by adjusting their thermal sensitivity and thus brumation period (Wells, 2007), which in turn could directly or indirectly affect the evolution of brain size (Heldstab et al., 2018). Hence, we estimated the brumation period for all 116 species. To this end, we visited the field sites for 30 of our species daily around the expected start and end times of brumation (based on prior experience). For each species, we recorded the dates and temperatures (using a Kobold HND-T105 high-precision thermometer to the nearest 0.1) when the last frogs of a given species were seen at the end of their active period (with no further activity detected for at least 7 days) and when the first individuals were detected in the spring. For the same 30 species (and using the same individuals as for morphological measurements), we then experimentally simulated brumation using a Q18 temperature-controlled refrigerator in Shenzhen Pioneer (SAST). We gradually lowered and raised the temperature at a rate of 0.5°C/hr and recorded the temperature at which test subjects entered and left brumation. When entering brumation, anurans drop their heart rate, become sluggish, draw their nictitating membranes across the eyes for protection, spread their legs for stability, and change their physiology to avoid freezing, start breathing through their skin, or switch to anaerobic metabolism (Fei and Ye, 2001; Pinder et al., 1992; Tattersall and Ultsch, 2008; Wells, 2007). To minimize disturbance in our experiment, we used the motion-less four-point stance with the nictitating membranes drawn across the eyes as our proxy of brumation. Our experimental threshold temperatures were tightly associated with the corresponding field measurements both for the start (r = 0.97, t28 = 22.26, p < 0.0001, λ = 0.04 [0.00, 0.47]) and end of the inactive state (r = 0.98, t28 = 28.05, p < 0.0001, λ = 0.04 [0.00, 0.43]). Hence, we assessed the corresponding temperatures for all remaining species in the laboratory and estimated the brumation period based on the daily mean temperatures at the corresponding collection sites as retrieved from Chinese Meteorological Stations (http://www.lishi.tianqi.com) between 2012 and 2016.
We defined the brumation period as the number of consecutive days in each year that remained below this threshold. For simplicity, we determined the active rather than brumation period, starting with the first day that the mean daily temperature rose above the activity threshold and remained there for at least five consecutive days, and ending with the last day before the temperature dropped below the activity threshold and remained there until the end of the calendar year. The brumation period then represented the difference between the activity period and the total number of days in each calendar year. Across these 5 years, the measured temperature thresholds yielded highly repeatable species-specific estimates of the number of days below the activity range (R = 0.95 [95% CI: 0.93–0.96]), as determined by the rpt function in the rptR package (Stoffel et al., 2017) across all 116 species (Appendix 1—figure 1; Appendix 1—table 1). Furthermore, across the 30 species that were examined both in the lab and the field (see above), these predicted brumation periods were also correlated with the observed brumation periods in the field (r = 0.96, t28 = 1 8.03, p < 0.0001, λ = 0.05 [0.00, 0.48]; Appendix 1—figure 8A), which themselves were highly repeatable between years within species (R = 0.98 [0.96–0.99]; Appendix 1—table 1).
Based on this data validation, we used for each species the mean brumation period predicted from our experimentally simulated temperature thresholds. However, to test for potential buffering effects of burrowing in the soil relative to the air temperatures reported by the meteorological stations, we also repeated these estimates by using more conservative thermal thresholds. Here, we restricted the putative brumation days to those with a reported air temperature of either 2 or 4°C below the experimentally derived inactivity thresholds, simulating prolonged activity by seeking shelter in burrows. The 2°C threshold was based on a pilot study comparing direct measurements of air and burrow temperatures for four different burrows in each of five of our study species (burrow depths: 32.0 ± 3.2 to 121.0 ± 17.8 cm; Appendix 1—figure 9). Across these species, the burrow-to-air temperature difference reached 1.03 ± 0.35 to 2.45 ± 0.60°C in measurements around the peak of the brumation period (i.e., early January; Figure S9). However, since these temporal snapshots were based on sites at relatively low elevation (≤320 m a.s.l.) due to accessibility of burrows during winter, we also used a second, more conservative buffer (4°C below activity range) for comparison. These temperature buffers shortened the predicted brumation periods to a varying degree between species (Appendix 1—figure 8); yet the predicted periods covaried strongly between the different temperature thresholds (all r > 0.90, t114 > 21.96, p < 0.0001, all λ <0 .01).
Phylogeny reconstruction
Request a detailed protocolTo reconstruct the phylogeny, we obtained the sequences of three nuclear and six mitochondrial genes from GenBank (for accession numbers and sequence coverage see Source data 4). The three nuclear genes included the recombination-activating gene 1 (RAG1), rhodopsin (RHOD), and tyrosinase (TYR). The six mitochondrial genes were cytochrome b (CYTB), cytochrome oxidase subunit I (COI), NADH dehydrogenase subunits 2 and 4 (ND2 and ND4), and the large and small subunits of the mitochondrial ribosome genes (12S/16S; omitting the adjacent tRNAs as they were difficult to align and represented only a small amount of data). We aligned the sequences by multi-sequence alignment (MUSCLE) in MEGA v.10.2.2 (Tamura et al., 2013) before comparing possible nucleotide substitution models. The best substitution model, as determined by the function modelTest() in the R (R Development Core Team, 2022) package phangorn (Schliep, 2011) based on the corrected Akaike information criterion, AICc, was GTR + Γ + I for all genes except RHOD, for which HKY + Γ had stronger support.
Using BEAUTi and BEAST v.1.10.4 (Suchard et al., 2018), we then constructed the phylogeny with unlinked substitution models, a relaxed uncorrelated log-normal clock, a Yule speciation process, and the best-supported nucleotide substitution models. We omitted time calibration due to a lack of fossil dates. We ran the Markov Chain Monte Carlo simulation for 55 million generations while sampling every 5000th tree with a 10% burn-in. Most effective sample size values by far exceeded 375 (i.e., all well above the recommended threshold of 200) for all but two tree statistics in the program Tracer v.1.7.2 (Rambaut et al., 2018), thus indicating satisfying convergence of the Bayesian chain and adequate model mixing. Finally, we generated a maximum clade credibility tree with mean node heights and a 10% burn-in using TreeAnnotator v.1.10.4 (Suchard et al., 2018), presented in Appendix 1—figure 10.
Breeding conditions
Request a detailed protocolTo test if a prolonged brumation period reduces the time available for reproduction, thereby changing the level of competition over mates and fertilizations (Lüpold et al., 2017), we extracted the start and end dates of the breeding season from our field notes of concurrent studies on species-specific life histories. These data were available for 43 of our species (Source data 3). We used dates when the first and last clutches were observed in focal ponds as a proxy of mating activity, given that males release their sperm during oviposition in these external fertilizers. For each species, dates from at least 2 years were combined and averaged to obtain the mean duration of the breeding season.
We further recorded whether dense mating aggregations are typically observed in these species. We have previously shown that larger mating clusters, with multiple males clasping the same females, have a significant effect on the evolution of testis size due to the resulting competition among sperm for fertilization (Lüpold et al., 2017). Here, we had no detailed data on the sizes of aggregations and so were only able to code the typical presence or absence of aggregations as a binary variable (Source data 3).
Finally, we used our direct estimates of species-specific population densities from our previous study (Lüpold et al., 2017) to test whether a shorter breeding season results in denser breeding populations. Although population density is a more direct measure than the occurrence of aggregations, such data were available for only eight of our species, each based on multiple populations per species (Lüpold et al., 2017). All these data were not necessarily derived from the same years or populations of our main dataset, but given the within-species repeatability in breeding populations (Lüpold et al., 2017) and in the duration of the breeding season (R = 0.88 [0.79–0.93]; Appendix 1—table 1), these differences should be relatively small compared to the interspecific variation and mostly introduce random noise.
Data analyses
General methods
Request a detailed protocolWe conducted all statistical analyses in R v.4.2.0 (R Development Core Team, 2022), using log-transformed data for all phenotypic traits, and for the coefficient of variance (CV) in temperature among the ecological variables. To account for non-independence of data due to common ancestry (Freckleton et al., 2002; Pagel, 1999), we conducted PGLS or phylogenetic logistic regressions (e.g., for occurrence of breeding aggregations), using the R package phylolm (Ho and Ané, 2014) and our reconstructed phylogeny. To account for variation around the species means, we bootstrapped for each model (at 100 fitted replicates) the standardized regression coefficients along with the phylogenetic scaling parameter λ and calculated their corresponding 95% CIs. The λ values indicate phylogenetic independence near zero and strong phylogenetic dependence near one (Freckleton et al., 2002).
Unless stated otherwise, all PGLS models focusing on the relative mass of tissues as the response included SVL as a covariate in addition to the focal predictor variable(s). We chose SVL instead of body mass because it is the commonly used measure of body size in anurans and independent of seasonal fluctuations in tissues such as body fat, testes, or limb muscles. One exception, however, was the analysis of phylogenetically informed allometric relationships, for which we cubed SVL such that a slope of 1 equaled unity (isometry). For these allometric relationships we calculated ordinary (generalized) least-squares rather than reduced major-axis regressions, because their greater sensitivity to changes in the steepness, but lower sensitivity to changes in scatter, capture allometric slopes more adequately (Kilmer and Rodríguez, 2017).
Pairwise correlations between tissues
Request a detailed protocolTo examine the covariation between different tissues across species, we first calculated pairwise partial correlations controlling for SVL and phylogeny. To this end, we calculated the phylogenetic trait variance–covariance matrix between the pairs of focal variable and SVL using the function phyl.vcv() in phytools (Revell, 2012) with λ = 1 (i.e., Brownian motion), which we then scaled into a correlation matrix using cov2cor() in the stats package (R Development Core Team, 2022). Using the resulting correlation coefficients rxy, rxz, and ryz, respectively, we then calculated the partial correlation coefficient rxy.z between the x and y variables of interest while accounting for SVL (z) following Crawley, 2007 equation: , with the associated t-statistics and 95% CIs converted using standard conversion () and the package effectsize (Ben-Shachar et al., 2020), respectively.
Multivariate allocation patterns
Request a detailed protocolSince pairwise correlations do not necessarily capture more complex, multivariate allocation patterns, we used two additional approaches to explore how tissue sizes varied relative to others: A compositional analysis and a principal component analysis. In both analyses, we focused on those four tissues that covaried with brumation duration or deviated from proportionate scaling with body size: brain, body fat, testes, and hindlimb muscles.
Compositional analyses
Request a detailed protocolIn the first approach, we used the function acomp() in the R package compositions (van den Boogaart and Tolosana-Delgado, 2008) to partition total body mass of each species into a five-variable Aitchison composition in a logistic geometry (van den Boogaart and Tolosana-Delgado, 2013), consisting of the proportional representation of the four focal tissues and the remaining body mass combined. Since the focal tissues constituted a size-independent fraction of the total body, the closed composition of this combined mass should be unbiased relative to body size but can instead reveal differential contributions of the four tissues to their total in a multivariate context (Aitchison, 1982; Muldowney et al., 2001; van den Boogaart and Tolosana-Delgado, 2013). For phylogenetic correlations between these variables following the description above, we used centered log ratios obtained by the function clr() in the same package, which maintains the original variable structure. However, owing to the reliance on a full rank of the covariance in multivariate analyses, we used the ilr() function to project the D-part composition isometrically to a D − 1 dimensional simplex (Aitchison, 1982), essentially representing the log ratios between the D parts. This multivariate object we subjected to a phylogenetic multivariate regression against brumation duration using the functions mvgls() and manova.gls() in the package mvMORPH (Clavel et al., 2015). For interpretation in the context of the original variable space, we back-transformed the coefficients using the ilrInv() function in compositions.
Phylogenetic principal component analysis
Request a detailed protocolIn addition to this compositional data analysis, we also peerformed a phylogenetically informed principal component analysis on the same focal tissues as log-transformed species means, using the phyl.pca() function of the package phytools (Revell, 2012). Here, we primarily focused on the directions of the loading vectors relative to the principal components and one another to glean information on the correlations between the original variables in the principal component space.
Phylogenetic confirmatory path analyses
Request a detailed protocolWe performed two phylogenetic confirmatory path analyses (Gonzalez-Voyer and Hardenberg, 2014; von Hardenberg and Gonzalez-Voyer, 2013) based on pre-specified candidate structural equation models. In the first analysis, we explored the direct and indirect effects of climatic variables on the duration of the breeding season or formation of breeding aggregations. Using eight candidate structural equation models, we essentially tested if breeding aggregations were the result of variation in latitude as a proxy of seasonality, either directly or mediated by the duration of brumation and/or of the breeding season. To avoid overparameterization given that this analysis was based on only 43 species, we did not include testis mass (and so necessarily also SVL to control for body size) as additional variables in the same path models. Rather, to test for links between relative testis size and breeding parameters, we conducted separate directional tests of trait evolution (see below).
A second path analysis aimed to disentangle the different interrelationships between traits that could ultimately mediate the effect of brumation duration on brain and reproductive evolution. Here, we used 28 pre-specified structural equation models to test for direct and indirect links between the brumation period and the four main tissues. As brumation is determined primarily by the environment, we focused on models that explained variation in tissue investments rather than, say, brain size affecting brumation patterns. The effects of brumation on the four tissues were direct or indirect, for example mediated by the digestive tract (resource acquisition) and/or body fat (resource storage), thus providing context for the cognitive buffer, expensive brain, expensive tissue, energy trade-off, and fat−brain trade-off hypotheses (Allman et al., 1993; Heldstab et al., 2016; Isler and van Schaik, 2006; Isler and van Schaik, 2009; Navarrete et al., 2011). Furthermore, we allowed brain size to affect testis size and vice versa (i.e., expensive sexual tissue hypothesis; Pitnick et al., 2006), and included models in which these organs explained variation in the digestive tract or fat tissue instead of being affected by them (e.g., selection on brain or testis size might mediate selection for greater resource availability rather than resources influencing brain or testis evolution). Across the 28 candidate models, we tested different combinations of these predictions, with traits being explained by single or multiple predictors, or having individual or shared effects on other traits. Using the R package phylopath (van der Bijl, 2018), we examined the conditional independencies of each model, ranked all candidate models based on their C-statistic information criterion (CICc), and then averaged the coefficients of the models with ΔCICc ≤2 from the top model (von Hardenberg and Gonzalez-Voyer, 2013).
Directional tests of trait evolution
Request a detailed protocolSince the first path analysis did not involve testis size to avoid overparametization, we separately tested for correlated evolution using directional tests of trait evolution (Pagel, 1994; Revell, 2012). One limitation of these models is that they rely on evolutionary transitions between binary states in each trait. Hence, we considered positive residuals of a log–log regression between testis mass and SVL as ‘relatively large testes’ and negative residuals as ‘relatively small testes’. For the duration of the breeding season, we similarly split the distribution based on the mean duration, whereas aggregation formation was already coded as present or absent. Based on (the weight of) the AIC, we then tested if changes in relative testis size and breeding parameters, respectively, were unilaterally dependent, mutually dependent, or independent (Pagel, 1994), using the fitPagel() function in the phytools package (Revell, 2012) with ‘fitDiscrete’ as the optimization method and allowing all rates to differ (i.e., ‘ARD’ model).
Appendix 1
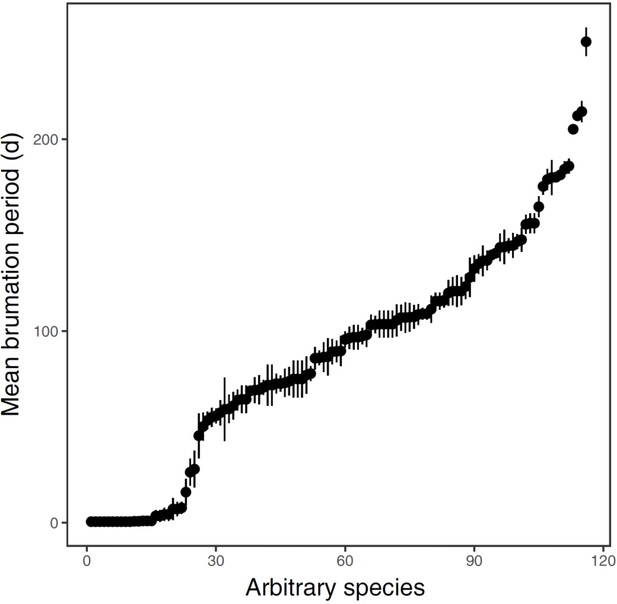
Distribution of brumation durations (in days) across the 116 anuran species.
Each dot represents the species-specific mean ± 1 SE of the 5 years (2012−2016) that specimens were collected. For each year and species, all days with a collection site-specific ambient temperature below the experimentally quantified, species-specific temperature thresholds were summed to estimate the brumation period.
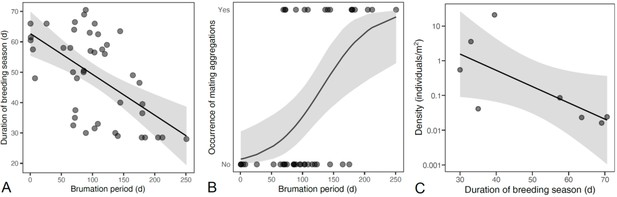
Effects of brumation on breeding conditions.
Prolonged brumation shortened the breeding season (A), thereby increasing the probability of dense breeding aggregations (B). This effect was also supported by a trend toward higher mean population densities in species with a shorter breeding season (C).
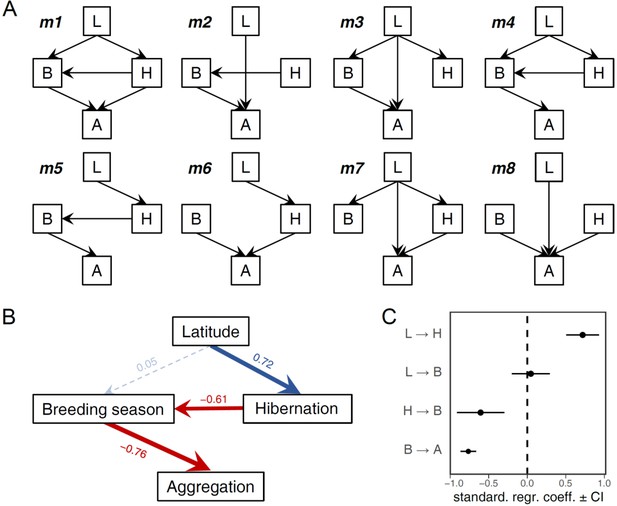
Confirmatory path analysis on the links between environmental variation, the brumation duration and breeding activity and the formation of breeding aggregations.
Directed acyclic graphs representing 8 candidate models (A) formed the predictions for the path analysis, resulting in the average path model (B). The path coefficients (standardised regression coefficients) and their 95% confidence intervals are depicted in (C). The variable letters correspond to the first letters of the full variable names in panel B. The model comparisons are listed in Appendix 1—table 13. The results were qualitatively identical when substituting latitude with elevation, mean temperature, or the coefficient of variation in temperature (except that the effect of mean temperature on brumation duration was negative as predicted). Note that here we used ‘hibernation’ instead of ‘brumation’ to avoid conflicts in codes.
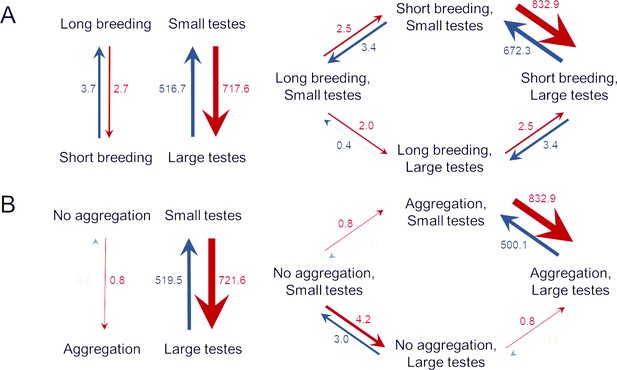
Directional test of trait evolution between relative testis size and breeding conditions.
Transition rates between binary states for relative testis size and (A) the duration of the breeding season or (B) aggregation formation. Independent models are shown on the left, models with changes in relative testis size dependent on the breeding parameters are depicted on the right. For both pairs of variables, the independent model had the highest support (A: wAIC = 0.50, B: wAIC = 0.66), but the dependent models shown here were either not significantly less supported (A: ΔAIC = 0.83, wAIC = 0.33) or relatively weakly so (B: ΔAIC = 2.38, wAIC = 0.20). The remaining two models (changes in breeding parameters depending on those in relative testis size or both variables evolving interdependently) found much less support in both analyses (A: ΔAIC ≥ 3.40, wAIC ≤ 0.09, B: ΔAIC ≥ 3.64, wAIC ≤ 0.11).
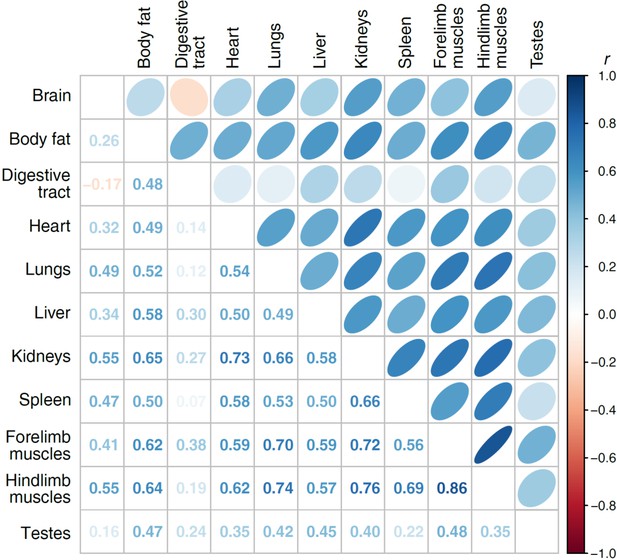
Pairwise partial correlations between the different tissues.
All correlations are controlled for snout-vent length and phylogeny, expressed both by ellipses (above diagonal) and the correlation coefficients (below the diagonal). All correlations with |rp| ≥ 0.19 had P < 0.05 before and after accounting for multiple testing based on the False Discovery Rate.
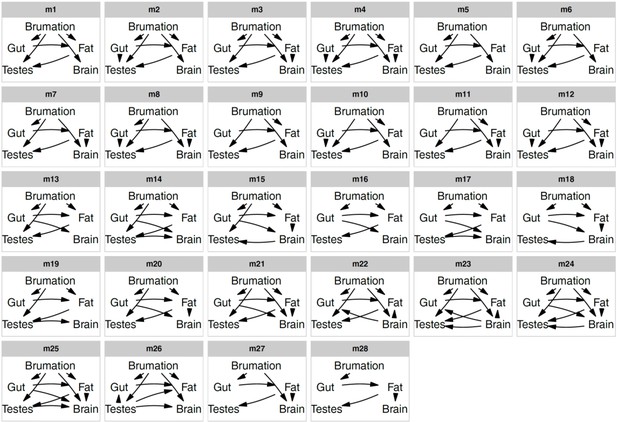
Candidate path models.
Directed acyclic graphs representing 28 candidate models that were compared to disentangle the relationships between five traits through a phylogenetic confirmatory path analysis and multi-model inference. For spatial reasons, the digestive tract is termed gut, and we omitted the paths from snout-vent length to all five variables in each panel to control tissue masses for body size or to improve d-separation (in the case of brumation).
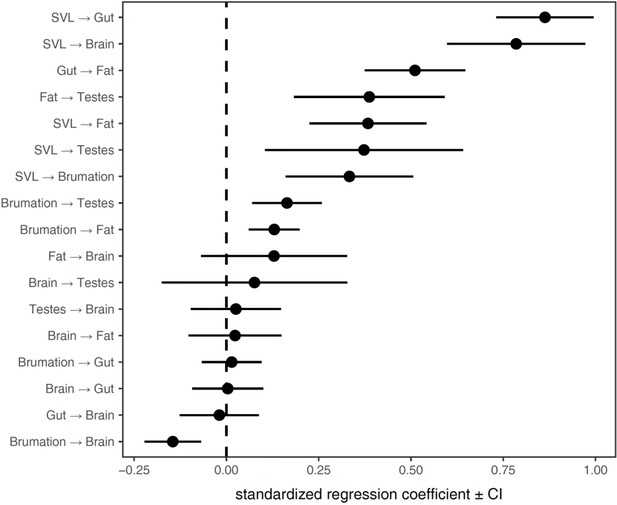
Coefficients of the averaged phylogenetic path model.
The path coefficients (standardised regression coefficients) are depicted with their 95% confidence intervals, corresponding to the path analysis in Figure 4 (directed path models in Appendix 1—figure 6). The paths from SVL to other traits are included only to control for body size (in the case of tissues) or to improve d-separation (SVL → Brumation). For visual clarity, these are omitted in the directed acyclic graphs (Appendix 1—figure 6) and the final averaged model (Figure 4).
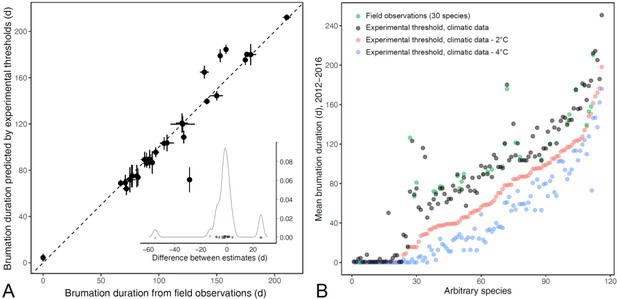
Validation of the different estimates of brumation duration.
(A) Comparisons of field observations with predicted brumation periods based on experimental thermal thresholds and site-specific climate data. The dashed line represents unity. The inserted density plot indicates that twenty-five of the 30 estimated values were within ≤8 days of the field observations across the spectrum, with the remaining five estimates deviating by approx. 13, 26 (3×) or, for one outlier, 55 days. (B) Variation in species-specific brumation periods based on different approaches. The green dots are the same field-based data as in panel A. The black dots represent values that combine the experimental temperature thresholds with the site-specific climatic data (predicted brumation below the threshold). The red and blue dots are based on the same experimental thresholds, but this time following the air temperature profiles of 2 and 4°C below these thresholds, respectively, to account for the potential buffering effect of the soil for underground hibernacula. Since the green dots were most closely associated with the black ones (i.e., without the additional buffer), we focused primarily on those values, but conducted additional analyses using the shorter, buffered brumation periods with no qualitative change. Finally, the horizontal dashed line splits the species into those with brumation likely present (above) or absent (below) to generate binary brumation variables for more direct comparison with previous studies in other taxa. This cut-off of ≤40 versus >40 days was motivated by the relatively large gap in the sequence of brumation periods in both the black and blue dots and that anurans are likely to survive relatively short cold spells without special adaptations. This cut-off generated divergent datasets between the direct experimental thresholds (black: N = 91 species with and 25 without prolonged brumation) and buffered thresholds (blue: N = 47 species with and 69 without prolonged brumation).
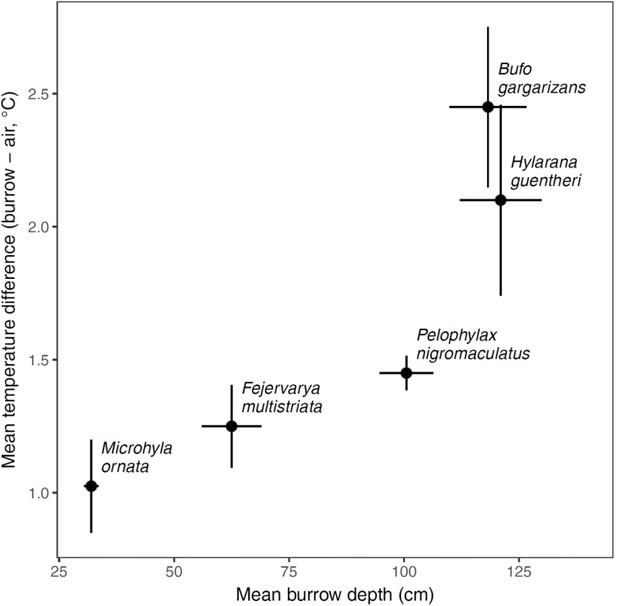
Comparison of air and burrow temperatures.
The difference between burrow and outside air temperature increased with burrow depth across the five species examined in a pilot study. The points reflect the means with standard errors around them based on four burrows per species.
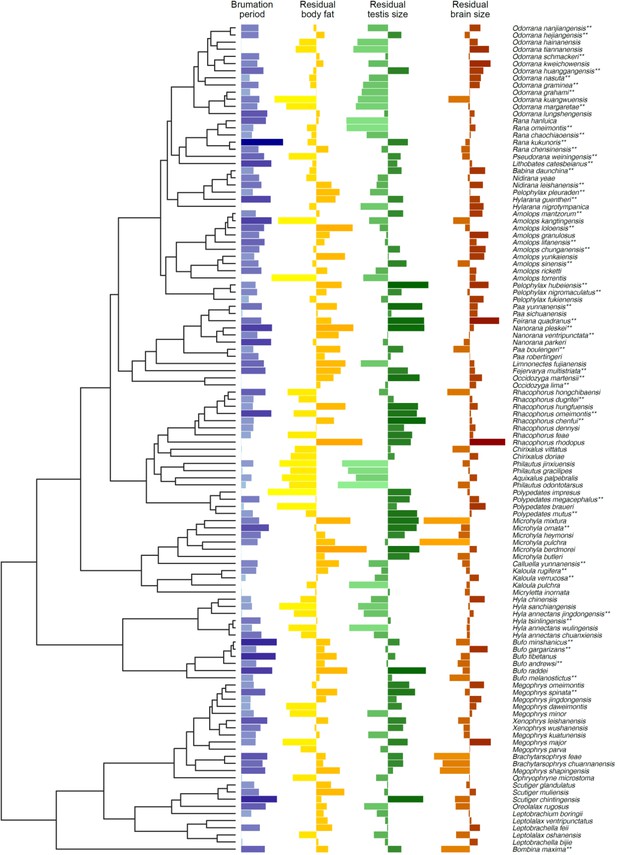
Phylogeny of the species used in the study.
Species with data on pre-brumation condition are labelled with double-asterisks (**). Residual trait sizes are derived from log-log linear regressions against snout-vent length.
Within-species repeatability in male morphology, climatic variables, and brumation duration.
CV = coefficient of variation, P2T = duration of the dry season (in months), with a month defined as dry when its total precipitation is less than two times the mean temperature. All analyses are based on the males (in breeding condition) or specific sampling locations for N = 116 species, except field observations of brumation duration (N = 30 species).
Variable | N | R [95% CI] | P |
---|---|---|---|
Male tissue masses | |||
SVL | 396 | 0.950 [0.933, 0.962] | <0.001 |
Brain | 396 | 0.948 [0.930, 0.961] | <0.001 |
Body fat | 396 | 0.958 [0.942, 0.969] | <0.001 |
Digestive tract | 396 | 0.949 [0.931, 0.962] | <0.001 |
Heart | 396 | 0.918 [0.890, 0.939] | <0.001 |
Lungs | 396 | 0.920 [0.890, 0.939] | <0.001 |
Liver | 396 | 0.915 [0.885, 0.936] | <0.001 |
Kidneys | 396 | 0.921 [0.893, 0.941] | <0.001 |
Spleen | 396 | 0.937 [0.914, 0.952] | <0.001 |
Forelimb muscles | 396 | 0.947 [0.929, 0.960] | <0.001 |
Hindlimb muscles | 396 | 0.961 [0.946, 0.971] | <0.001 |
Testes | 396 | 0.947 [0.929, 0.961] | <0.001 |
Climate variables | |||
Mean temperature | 580 | 0.982 [0.976, 0.986] | <0.001 |
Mean precipitation | 580 | 0.757 [0.693, 0.802] | <0.001 |
CV temperature | 580 | 0.978 [0.971, 0.982] | <0.001 |
CV precipitation | 580 | 0.401 [0.327, 0.486] | <0.001 |
P2T | 580 | 0.688 [0.614, 0.751] | <0.001 |
Brumation | |||
Brumation duration (estimated from lab data) | 580 | 0.947 [0.928, 0.959] | <0.001 |
Brumation duration (field observations) | 66 | 0.982 [0.965, 0.991] | <0.001 |
Breeding season | |||
Breeding season duration | 86 | 0.881 [0.785, 0.936] | <0.001 |
Species-specific environmental effects on the brumation duration.
CV = coefficient of variation, P2T = duration of the dry season (in months), with a month defined as dry when its total precipitation is less than two times the mean temperature. All analyses are based on df = 114, and confidence intervals are bootstrapped (N = 100 simulations). λ = phylogenetic scaling parameter.
Predictor | β [95% CI] | r [95% CI] | t | P | λ [95% CI] |
---|---|---|---|---|---|
Latitude | 0.72 [ 0.61, 0.82] | 0.72 [ 0.63, 0.78] | 11.03 | <0.001 | 0.00 [0.00, 0.10] |
Longitude | 0.09 [-0.06, 0.28] | 0.09 [-0.09, 0.27] | 1.00 | 0.321 | 0.47 [0.00, 0.69] |
Elevation | 0.43 [ 0.24, 0.62] | 0.43 [ 0.28, 0.56] | 5.15 | <0.001 | 0.00 [0.00, 0.10] |
Mean temperature | –0.56 [-0.69,–0.38] | –0.56 [-0.66,–0.42] | –7.14 | <0.001 | 0.00 [0.00, 0.11] |
Mean precipitation | –0.30 [-0.45,–0.13] | –0.30 [-0.45,–0.13] | –3.42 | <0.001 | 0.00 [0.00, 0.10] |
CV temperature | 0.65 [ 0.51, 0.78] | 0.65 [ 0.54, 0.73] | 9.08 | <0.001 | 0.00 [0.00, 0.11] |
CV precipitation | 0.01 [-0.18, 0.21] | 0.01 [-0.17, 0.19] | 0.06 | 0.948 | 0.42 [0.00, 0.65] |
P2T | –0.37 [-0.52,–0.19] | –0.38 [-0.51,–0.21] | –4.38 | <0.001 | 0.42 [0.00, 0.66] |
Species-specific environmental effects on the brumation duration, excluding those 25 species that are unlikely to hibernate (≤27 days).
CV = coefficient of variation, P2T = duration of the dry season (in months), with a month defined as dry when its total precipitation is less than two times the mean temperature. All analyses are based on df = 89, and confidence intervals are bootstrapped (N = 100 simulations). λ = phylogenetic scaling parameter.
Predictor | β [95% CI] | r [95% CI] | t | P | λ [95% CI] |
---|---|---|---|---|---|
Latitude | 0.46 [ 0.28, 0.65] | 0.46 [ 0.29, 0.60] | 4.94 | <0.001 | 0.00 [0.00, 0.11] |
Longitude | –0.15 [-0.35, 0.08] | –0.15 [-0.33, 0.06] | –1.38 | 0.170 | 0.00 [0.00, 0.07] |
Elevation | 0.42 [ 0.21, 0.61] | 0.42 [ 0.24, 0.56] | 4.38 | <0.001 | 0.00 [0.00, 0.11] |
Mean temperature | –0.36 [-0.58,–0.15] | –0.36 [-0.52,–0.17] | –3.69 | <0.001 | 0.00 [0.00, 0.10] |
Mean precipitation | –0.35 [-0.51,–0.12] | –0.35 [-0.51,–0.16] | –3.54 | <0.001 | 0.00 [0.00, 0.08] |
CV temperature | 1.17 [ 0.47, 1.80] | 0.72 [ 0.28, 0.87] | 3.42 | 0.006 | 0.79 [0.00, 1.00] |
CV precipitation | 0.03 [-0.16, 0.25] | 0.03 [-0.18, 0.23] | 0.27 | 0.785 | 0.00 [0.00, 0.06] |
P2T | –0.21 [-0.38,–0.01] | –0.21 [-0.39, 0.00] | –2.02 | 0.046 | 0.00 [0.00, 0.09] |
Species-specific environmental effects on the temperature at which males entered or left their inactive state.
All analyses are limited to those 91 species that are likely to overwinter (≥47 days below experimental temperature threshold), with df = 89, and confidence intervals are bootstrapped (N = 100 simulations). CV = coefficient of variation, P2T = duration of the dry season (in months), with a month defined as dry when its total precipitation is less than two times the mean temperature, λ = phylogenetic scaling parameter.
Predictor | β [95% CI] | r [95% CI] | t | P | λ |
---|---|---|---|---|---|
Entering inactive state: | |||||
Latitude | –0.10 [-0.26, 0.09] | –0.12 [-0.31, 0.09] | –1.16 | 0.247 | 0.32 [0.00, 0.56] |
Longitude | 0.24 [ 0.09, 0.41] | 0.31 [ 0.11, 0.47] | 3.02 | 0.003 | 0.00 [0.00, 0.07] |
Elevation | –1.42 [-1.94,–0.99] | –0.51 [-0.63,–0.34] | –5.56 | <0.001 | 0.07 [0.00, 0.23] |
Mean temperature | 0.24 [ 0.13, 0.35] | 0.46 [ 0.28, 0.59] | 4.84 | <0.001 | 0.00 [0.00, 0.10] |
Mean precipitation | 0.01 [-0.01, 0.03] | 0.07 [-0.13, 0.27] | 0.71 | 0.480 | 0.39 [0.00, 0.61] |
CV temperature | –1.78 [-2.69,–0.88] | –0.39 [-0.54,–0.20] | –4.03 | <0.001 | 0.00 [0.00, 0.07] |
CV precipitation | –3.62 [-6.87, 0.10] | –0.21 [-0.39, 0.00] | –1.99 | 0.049 | 0.00 [0.00, 0.06] |
P2T | 0.35 [ 0.01, 0.63] | 0.22 [ 0.02, 0.40] | 2.18 | 0.032 | 0.43 [0.00, 0.66] |
Leaving inactive state: | |||||
Latitude | –0.12 [-0.30, 0.08] | –0.14 [-0.33, 0.07] | –1.34 | 0.184 | 0.47 [0.00, 0.68] |
Longitude | 0.28 [ 0.12, 0.46] | 0.35 [ 0.15, 0.50] | 3.48 | <0.001 | 0.37 [0.00, 0.59] |
Elevation | –1.63 [-2.13,–1.18] | –0.55 [-0.66,–0.39] | –6.23 | <0.001 | 0.23 [0.00, 0.46] |
Mean temperature | 0.21 [ 0.09, 0.32] | 0.39 [ 0.20, 0.54] | 3.97 | <0.001 | 0.35 [0.00, 0.59] |
Mean precipitation | 0.01 [ 0.00, 0.03] | 0.14 [-0.07, 0.33] | 1.32 | 0.191 | 0.47 [0.00, 0.68] |
CV temperature | –1.33 [-2.30,–0.39] | –0.30 [-0.46,–0.10] | –2.93 | 0.004 | 0.44 [0.00, 0.66] |
CV precipitation | –4.43 [-8.14,–0.72] | –0.25 [-0.42,–0.05] | –2.43 | 0.017 | 0.42 [0.00, 0.64] |
P2T | 0.22 [-0.13, 0.53] | 0.14 [-0.07, 0.33] | 1.33 | 0.188 | 0.53 [0.00, 0.73] |
Associations between species-specific environmental effects and male body size.
Associations of either snout-vent length or body mass as two measures of body size with brumation duration and different environmental variables. All analyses based on df = 114, and confidence intervals are bootstrapped (N = 100 simulations). CV = coefficient of variation, P2T = duration of the dry season (in months), with a month defined as dry when its total precipitation is less than two times the mean temperature, and λ = phylogenetic scaling parameter.
Predictor | β [95% CI] | r [95% CI] | t | P | λ [95% CI] |
---|---|---|---|---|---|
Snout-vent length: | |||||
Brumation duration | 0.02 [-0.04, 0.07] | 0.06 [-0.13, 0.23] | 0.59 | 0.557 | 0.93 [0.82, 0.99] |
Latitude | 0.04 [-0.03, 0.09] | 0.13 [-0.06, 0.30] | 1.36 | 0.177 | 0.93 [0.82, 0.99] |
Elevation | –0.01 [-0.07, 0.03] | –0.05 [-0.23, 0.13] | –0.53 | 0.597 | 0.94 [0.85, 1.00] |
Mean temperature | –0.01 [-0.05, 0.05] | –0.04 [-0.21, 0.15] | –0.38 | 0.702 | 0.93 [0.82, 0.99] |
CV temperature | 0.01 [-0.05, 0.06] | 0.04 [-0.14, 0.22] | 0.42 | 0.676 | 0.93 [0.82, 0.99] |
P2T | 0.01 [-0.02, 0.06] | 0.05 [-0.13, 0.23] | 0.55 | 0.583 | 0.94 [0.83, 0.99] |
Body mass: | |||||
Brumation duration | –0.03 [-0.23, 0.14] | –0.03 [-0.21, 0.15] | –0.36 | 0.722 | 0.92 [0.80, 0.98] |
Latitude | 0.10 [-0.12, 0.27] | 0.09 [-0.09, 0.27] | 1.00 | 0.321 | 0.91 [0.77, 0.97] |
Elevation | –0.16 [-0.32,–0.02] | –0.18 [-0.34, 0.01] | –1.91 | 0.058 | 0.94 [0.85, 1.00] |
Mean temperature | 0.03 [-0.11, 0.23] | 0.03 [-0.15, 0.21] | 0.37 | 0.710 | 0.92 [0.79, 0.99] |
CV temperature | –0.03 [-0.22, 0.12] | –0.03 [-0.21, 0.15] | –0.36 | 0.721 | 0.92 [0.79, 0.99] |
P2T | 0.14 [0.02, 0.29] | 0.16 [-0.02, 0.33] | 1.72 | 0.087 | 0.93 [0.81, 0.98] |
Associations between tissue size and body size.
Log-log association of tissue mass with snout-vent length. To facilitate the interpretation of the allometric slopes (β), SVL is cubed (SVL3) so that β = 1 equals isometry. All analyses are based on df = 114, and confidence intervals are bootstrapped (N = 100 simulations). λ = phylogenetic scaling parameter.
Response | β [95% CI] | r [95% CI] | t | P | λ [95% CI] |
---|---|---|---|---|---|
Brain | 0.49 [0.44, 0.54] | 0.88 [0.84, 0.91] | 19.66 | <0.001 | 0.73 [0.35, 0.87] |
Body fat | 1.36 [1.28, 1.48] | 0.94 [0.92, 0.95] | 29.50 | <0.001 | 0.39 [0.00, 0.64] |
Digestive tract | 0.95 [0.88, 1.01] | 0.91 [0.88, 0.93] | 23.60 | <0.001 | 0.00 [0.00, 0.10] |
Heart | 1.07 [0.98, 1.17] | 0.89 [0.86, 0.92] | 21.27 | <0.001 | 0.18 [0.00, 0.45] |
Lungs | 1.06 [0.96, 1.19] | 0.87 [0.83, 0.90] | 18.81 | <0.001 | 0.23 [0.00, 0.50] |
Liver | 1.03 [0.96, 1.10] | 0.93 [0.91, 0.95] | 27.12 | <0.001 | 0.00 [0.00, 0.10] |
Kidneys | 1.03 [0.97, 1.09] | 0.95 [0.93, 0.96] | 30.90 | <0.001 | 0.00 [0.00, 0.10] |
Spleen | 1.10 [0.98, 1.26] | 0.84 [0.79, 0.88] | 16.56 | <0.001 | 0.38 [0.00, 0.64] |
Forelimb muscles | 1.23 [1.16, 1.30] | 0.94 [0.93, 0.96] | 30.62 | <0.001 | 0.00 [0.00, 0.10] |
Hindlimb muscles | 1.10 [1.03, 1.18] | 0.95 [0.93, 0.96] | 31.01 | <0.001 | 0.49 [0.00, 0.71] |
Testes | 1.06 [0.94, 1.22] | 0.82 [0.76, 0.86] | 15.27 | <0.001 | 0.56 [0.00, 0.77] |
Results of phylogenetically controlled relationships (λ = phylogenetic scaling parameter) between the brumation duration and the relative size of different male tissues in post-brumation breeding (N = 116 species) or pre-brumation condition (N = 50 species), measured as tissue mass.
All associations are controlled for snout-vent length (SVL), and confidence intervals are bootstrapped (N = 100 simulations).
Response | Predictors | β [95% CI] | Partial r [95% CI] | t | P | λ [95% CI] |
---|---|---|---|---|---|---|
Males in breeding condition (df = 113) | ||||||
Brain | Brumation | –0.09 [-0.15,–0.04] | –0.31 [-0.46,–0.14] | –3.50 | <0.001 | 0.35 [0.00, 0.61] |
SVL | 0.65 [ 0.59, 0.71] | 0.91 [ 0.87, 0.93] | 22.76 | <0.001 | ||
Body fat | Brumation | 0.20 [0.04, 0.34] | 0.25 [0.07, 0.40] | 2.72 | 0.008 | 0.42 [0.00, 0.66] |
SVL | 1.55 [1.37, 1.73] | 0.87 [0.83, 0.90] | 18.72 | <0.001 | ||
Digestive tract | Brumation | 0.03 [-0.11, 0.14] | 0.04 [-0.14, 0.22] | 0.45 | 0.656 | 0.00 [0.00, 0.11] |
SVL | 1.12 [ 1.01, 1.25] | 0.86 [ 0.81, 0.89] | 17.66 | <0.001 | ||
Heart | Brumation | –0.07 [-0.22, 0.06] | –0.09 [-0.26, 0.09] | –0.96 | 0.340 | 0.23 [0.00, 0.51] |
SVL | 1.35 [ 1.20, 1.50] | 0.87 [ 0.82, 0.90] | 18.49 | <0.001 | ||
Lungs | Brumation | –0.05 [-0.23, 0.09] | –0.06 [-0.24, 0.12] | –0.69 | 0.490 | 0.21 [0.00, 0.50] |
SVL | 1.31 [ 1.15, 1.49] | 0.83 [ 0.77, 0.87] | 15.86 | <0.001 | ||
Liver | Brumation | 0.05 [-0.09, 0.16] | 0.07 [-0.11, 0.25] | 0.77 | 0.445 | 0.00 [0.00, 0.11] |
SVL | 1.23 [ 1.11, 1.36] | 0.87 [ 0.83, 0.90] | 19.12 | <0.001 | ||
Kidneys | Brumation | –0.02 [-0.14, 0.08] | –0.03 [-0.21, 0.15] | –0.37 | 0.715 | 0.00 [0.00, 0.11] |
SVL | 1.25 [ 1.15, 1.36] | 0.90 [ 0.87, 0.92] | 22.15 | <0.001 | ||
Spleen | Brumation | –0.12 [-0.31, 0.04] | –0.12 [-0.30, 0.06] | –1.32 | 0.189 | 0.23 [0.00, 0.52] |
SVL | 1.34 [ 1.15, 1.55] | 0.80 [ 0.74, 0.85] | 14.27 | <0.001 | ||
Foreleg muscles | Brumation | –0.06 [-0.20, 0.05] | –0.09 [-0.27, 0.09] | –0.98 | 0.329 | 0.00 [0.00, 0.11] |
SVL | 1.52 [ 1.40, 1.66] | 0.91 [ 0.88, 0.93] | 23.29 | <0.001 | ||
Hindleg muscles | Brumation | –0.13 [-0.25,–0.03] | –0.22 [-0.38,–0.04] | –2.37 | 0.019 | 0.22 [0.00, 0.51] |
SVL | 1.35 [ 1.23, 1.48] | 0.91 [ 0.88, 0.93] | 22.98 | <0.001 | ||
Testes | Brumation | 0.30 [0.15, 0.44] | 0.36 [0.19, 0.50] | 4.06 | <0.001 | 0.77 [0.40, 0.90] |
SVL | 1.21 [1.01, 1.41] | 0.78 [0.71, 0.83] | 13.30 | <0.001 | ||
Males in pre-brumation condition (df = 47) | ||||||
Brain | Brumation | –0.15 [-0.21,–0.09] | –0.51 [-0.67,–0.27] | –4.03 | <0.001 | 0.00 [0.00, 0.56] |
SVL | 0.60 [ 0.55, 0.66] | 0.92 [ 0.88, 0.95] | 16.30 | <0.001 | ||
Body fat | Brumation | 0.33 [0.21, 0.46] | 0.53 [0.29, 0.68] | 4.26 | <0.001 | 0.55 [0.00, 0.88] |
SVL | 1.21 [1.04, 1.40] | 0.89 [0.82, 0.92] | 13.21 | <0.001 | ||
Digestive tract | Brumation | 0.08 [-0.04, 0.20] | 0.15 [-0.14, 0.40] | 1.01 | 0.320 | 0.64 [0.00, 0.92] |
SVL | 1.16 [ 0.99, 1.33] | 0.88 [ 0.81, 0.92] | 12.85 | <0.001 | ||
Heart | Brumation | –0.02 [-0.15, 0.11] | –0.03 [-0.30, 0.25] | –0.22 | 0.825 | 0.24 [0.00, 0.74] |
SVL | 0.89 [ 0.74, 1.07] | 0.83 [ 0.73, 0.88] | 10.11 | <0.001 | ||
Lungs | Brumation | 0.02 [-0.14, 0.18] | 0.03 [-0.24, 0.31] | 0.24 | 0.812 | 0.55 [0.00, 0.89] |
SVL | 1.03 [ 0.81, 1.26] | 0.80 [ 0.68, 0.86] | 9.02 | <0.001 | ||
Liver | Brumation | 0.11 [-0.02, 0.23] | 0.19 [-0.09, 0.44] | 1.34 | 0.186 | 0.00 [0.00, 0.56] |
SVL | 1.16 [ 1.05, 1.30] | 0.90 [ 0.85, 0.93] | 14.56 | <0.001 | ||
Kidneys | Brumation | –0.06 [-0.17, 0.04] | –0.14 [-0.39, 0.15] | –0.97 | 0.338 | 0.00 [0.00, 0.56] |
SVL | 0.99 [ 0.90, 1.11] | 0.91 [ 0.85, 0.94] | 14.85 | <0.001 | ||
Spleen | Brumation | 0.11 [-0.10, 0.32] | 0.12 [-0.16, 0.38] | 0.83 | 0.410 | 0.00 [0.00, 0.56] |
SVL | 1.11 [ 0.93, 1.35] | 0.77 [ 0.64, 0.85] | 8.35 | <0.001 | ||
Foreleg muscles | Brumation | –0.07 [-0.18, 0.05] | –0.14 [-0.39, 0.15] | –0.94 | 0.354 | 0.00 [0.00, 0.56] |
SVL | 1.32 [ 1.22, 1.45] | 0.93 [ 0.90, 0.96] | 18.00 | <0.001 | ||
Hindleg muscles | Brumation | –0.09 [-0.18, 0.01] | –0.21 [-0.45, 0.07] | –1.49 | 0.142 | 0.57 [0.00, 0.89] |
SVL | 1.13 [ 1.01, 1.27] | 0.93 [ 0.88, 0.95] | 16.96 | <0.001 | ||
Testes | Brumation | 0.02 [-0.14, 0.22] | 0.02 [-0.26, 0.29] | 0.15 | 0.880 | 0.82 [0.15, 0.98] |
SVL | 1.00 [ 0.72, 1.24] | 0.73 [ 0.58, 0.82] | 7.34 | <0.001 |
Results of phylogenetically controlled relationships (λ = phylogenetic scaling parameter) of brumation on the relative mass of male tissues (N = 116 species).
For justification of assignment of brumating and non-brumating species, see Appendix 1—figure 7. All associations are controlled for snout-vent length (SVL), and confidence intervals are bootstrapped (N = 100 simulations).
Response | Predictors | β [95% CI] | Partial r [95% CI] | t | P | λ [95% CI] |
---|---|---|---|---|---|---|
Binary classification of brumation (N=91 brumating, 25 non-brumating species; total df = 113) | ||||||
Brain | Brumation | –0.12 [-0.25, 0.03] | –0.17 [-0.34, 0.01] | –1.86 | 0.066 | 0.34 [0.00, 0.60] |
SVL | 0.64 [ 0.58, 0.71] | 0.90 [ 0.86, 0.92] | 21.56 | <0.001 | ||
Body fat | Brumation | 0.53 [0.21, 0.93] | 0.26 [0.08, 0.42] | 2.91 | 0.004 | 0.50 [0.00, 0.72] |
SVL | 1.53 [1.34, 1.71] | 0.86 [0.82, 0.89] | 18.26 | <0.001 | ||
Digestive tract | Brumation | 0.01 [-0.30, 0.36] | 0.00 [-0.18, 0.19] | 0.04 | 0.965 | 0.00 [0.00, 0.10] |
SVL | 1.13 [ 1.02, 1.27] | 0.86 [ 0.81, 0.89] | 17.64 | <0.001 | ||
Heart | Brumation | –0.22 [-0.56, 0.16] | –0.12 [-0.30, 0.06] | –1.34 | 0.184 | 0.21 [0.00, 0.49] |
SVL | 1.36 [ 1.21, 1.51] | 0.87 [ 0.82, 0.90] | 18.61 | <0.001 | ||
Lungs | Brumation | –0.07 [-0.47, 0.36] | –0.04 [-0.22, 0.15] | –0.39 | 0.697 | 0.20 [0.00, 0.48] |
SVL | 1.31 [ 1.14, 1.48] | 0.83 [ 0.77, 0.87] | 15.71 | <0.001 | ||
Liver | Brumation | 0.03 [-0.28, 0.39] | 0.02 [-0.16, 0.20] | 0.21 | 0.833 | 0.00 [0.00, 0.10] |
SVL | 1.24 [ 1.13, 1.38] | 0.87 [ 0.83, 0.90] | 19.11 | <0.001 | ||
Kidneys | Brumation | –0.11 [-0.38, 0.20] | –0.08 [-0.25, 0.11] | –0.83 | 0.408 | 0.00 [0.00, 0.10] |
SVL | 1.26 [ 1.16, 1.38] | 0.90 [ 0.87, 0.92] | 22.20 | <0.001 | ||
Spleen | Brumation | –0.39 [-0.83, 0.10] | –0.17 [-0.34, 0.01] | –1.83 | 0.071 | 0.23 [0.00, 0.51] |
SVL | 1.36 [ 1.17, 1.55] | 0.81 [ 0.74, 0.85] | 14.47 | <0.001 | ||
Foreleg muscles | Brumation | –0.07 [-0.39, 0.29] | –0.04 [-0.22, 0.14] | –0.45 | 0.653 | 0.00 [0.00, 0.10] |
SVL | 1.51 [ 1.39, 1.66] | 0.91 [ 0.88, 0.93] | 22.87 | <0.001 | ||
Hindleg muscles | Brumation | –0.15 [-0.44, 0.16] | –0.11 [-0.28, 0.08] | –1.12 | 0.264 | 0.17 [0.00, 0.45] |
SVL | 1.34 [ 1.22, 1.44] | 0.90 [ 0.87, 0.93] | 22.41 | <0.001 | ||
Testes | Brumation | 0.49 [ 0.13, 0.89] | 0.23 [ 0.05, 0.39] | 2.49 | 0.014 | 0.75 [0.36, 0.90] |
SVL | 1.23 [ 1.02, 1.43] | 0.77 [ 0.70, 0.82] | 12.91 | <0.001 | ||
Binary classification of brumation with a 4 °C buffer (N=47 brumating, 69 non-brumating species; total df = 113) | ||||||
Brain | Brumation | –0.21 [-0.31,–0.14] | –0.38 [-0.51,–0.21] | –4.33 | <0.001 | 0.35 [0.00, 0.62] |
SVL | 0.64 [ 0.59, 0.71] | 0.91 [ 0.88, 0.93] | 23.47 | <0.001 | ||
Body fat | Brumation | 0.29 [ 0.00, 0.52] | 0.19 [ 0.01, 0.36] | 2.07 | 0.041 | 0.46 [0.00, 0.72] |
SVL | 1.57 [ 1.39, 1.77] | 0.87 [ 0.83, 0.90] | 18.88 | <0.001 | ||
Digestive tract | Brumation | 0.07 [-0.17, 0.23] | 0.06 [-0.13, 0.23] | 0.60 | 0.553 | 0.00 [0.00, 0.11] |
SVL | 1.12 [ 1.01, 1.24] | 0.87 [ 0.82, 0.90] | 18.43 | <0.001 | ||
Heart | Brumation | –0.25 [-0.52,–0.07] | –0.18 [-0.35, 0.00] | –1.97 | 0.051 | 0.23 [0.00, 0.51] |
SVL | 1.35 [ 1.21, 1.52] | 0.88 [ 0.83, 0.90] | 19.23 | <0.001 | ||
Lungs | Brumation | –0.31 [-0.61,–0.10] | –0.20 [-0.36,–0.01] | –2.13 | 0.035 | 0.21 [0.00, 0.50] |
SVL | 1.32 [ 1.17, 1.51] | 0.84 [ 0.79, 0.88] | 16.68 | <0.001 | ||
Liver | Brumation | –0.09 [-0.34, 0.06] | –0.07 [-0.25, 0.11] | –0.76 | 0.45 | 0.00 [0.00, 0.11] |
SVL | 1.25 [ 1.14, 1.38] | 0.89 [ 0.85, 0.91] | 20.31 | <0.001 | ||
Kidneys | Brumation | –0.06 [-0.27, 0.08] | –0.05 [-0.23, 0.13] | –0.55 | 0.586 | 0.00 [0.00, 0.11] |
SVL | 1.24 [ 1.15, 1.35] | 0.91 [ 0.88, 0.93] | 23.04 | <0.001 | ||
Spleen | Brumation | –0.19 [-0.54, 0.05] | –0.10 [-0.28, 0.08] | –1.12 | 0.265 | 0.23 [0.00, 0.51] |
SVL | 1.33 [ 1.14, 1.54] | 0.80 [ 0.74, 0.85] | 14.42 | <0.001 | ||
Foreleg muscles | Brumation | –0.26 [-0.50,–0.10] | –0.19 [-0.35,–0.01] | –2.04 | 0.043 | 0.00 [0.00, 0.11] |
SVL | 1.52 [ 1.41, 1.65] | 0.92 [ 0.89, 0.94] | 24.62 | <0.001 | ||
Hindleg muscles | Brumation | –0.23 [-0.45,–0.08] | –0.20 [-0.36,–0.02] | –2.16 | 0.033 | 0.24 [0.00, 0.52] |
SVL | 1.33 [ 1.22, 1.47] | 0.91 [ 0.88, 0.93] | 23.04 | <0.001 | ||
Testes | Brumation | 0.38 [ 0.09, 0.60] | 0.24 [ 0.06, 0.40] | 2.63 | 0.01 | 0.74 [0.34, 0.89] |
SVL | 1.25 [ 1.03, 1.44] | 0.78 [ 0.71, 0.83] | 13.31 | <0.001 |
Effects of the brumation duration on relative tissue size in males, excluding those 25 species that are unlikely to overwinter (≤27 days).
Results of phylogenetically controlled relationships (λ = phylogenetic scaling parameter) between the brumation duration and the relative mass of different male tissues in breeding condition (df = 88). All associations are controlled for snout-vent length (SVL), and confidence intervals are bootstrapped (N = 100 simulations).
Response | Predictors | β [95% CI] | Partial r [95% CI] | t | P | λ [95% CI] |
---|---|---|---|---|---|---|
Brain | Brumation | –0.07 [-0.13,–0.02] | –0.27 [-0.44,–0.07] | –2.62 | 0.010 | 0.49 [0.00, 0.72] |
SVL | 0.56 [ 0.50, 0.62] | 0.88 [ 0.84, 0.91] | 17.52 | <0.001 | ||
Body fat | Brumation | 0.07 [-0.08, 0.22] | 0.10 [-0.11, 0.29] | 0.92 | 0.36 | 0.38 [0.00, 0.61] |
SVL | 1.43 [ 1.26, 1.60] | 0.88 [ 0.83, 0.91] | 17.08 | <0.001 | ||
Digestive tract | Brumation | 0.04 [-0.09, 0.20] | 0.06 [-0.15, 0.26] | 0.55 | 0.587 | 0.00 [0.00, 0.11] |
SVL | 0.99 [ 0.84, 1.13] | 0.83 [ 0.77, 0.87] | 14.03 | <0.001 | ||
Heart | Brumation | –0.07 [-0.20, 0.09] | –0.10 [-0.29, 0.11] | –0.93 | 0.355 | 0.13 [0.00, 0.33] |
SVL | 1.28 [ 1.14, 1.44] | 0.88 [ 0.83, 0.91] | 17.14 | <0.001 | ||
Lungs | Brumation | –0.11 [-0.27, 0.06] | –0.15 [-0.34, 0.06] | –1.40 | 0.165 | 0.23 [0.00, 0.46] |
SVL | 1.24 [ 1.08, 1.42] | 0.84 [ 0.78, 0.88] | 14.75 | <0.001 | ||
Liver | Brumation | 0.00 [-0.14, 0.16] | –0.01 [-0.21, 0.20] | –0.06 | 0.949 | 0.05 [0.00, 0.19] |
SVL | 1.13 [ 0.99, 1.29] | 0.85 [ 0.80, 0.89] | 15.38 | <0.001 | ||
Kidneys | Brumation | 0.03 [-0.08, 0.16] | 0.05 [-0.16, 0.25] | 0.48 | 0.635 | 0.00 [0.00, 0.11] |
SVL | 1.15 [ 1.03, 1.27] | 0.90 [ 0.87, 0.93] | 19.88 | <0.001 | ||
Spleen | Brumation | –0.06 [-0.23, 0.13] | –0.07 [-0.26, 0.14] | –0.61 | 0.541 | 0.29 [0.00, 0.52] |
SVL | 1.32 [ 1.12, 1.53] | 0.82 [ 0.75, 0.86] | 13.32 | <0.001 | ||
Foreleg muscles | Brumation | –0.10 [-0.23, 0.06] | –0.16 [-0.35, 0.05] | –1.49 | 0.139 | 0.00 [0.00, 0.11] |
SVL | 1.41 [ 1.26, 1.55] | 0.91 [ 0.87, 0.93] | 20.34 | <0.001 | ||
Hindleg muscles | Brumation | –0.16 [-0.27,–0.04] | –0.30 [-0.47,–0.10] | –2.93 | 0.004 | 0.15 [0.00, 0.35] |
SVL | 1.27 [ 1.16, 1.40] | 0.92 [ 0.89, 0.94] | 21.76 | <0.001 | ||
Testes | Brumation | 0.19 [ 0.02, 0.35] | 0.25 [0.04, 0.42] | 2.38 | 0.019 | 0.55 [0.03, 0.76] |
SVL | 1.21 [ 1.01, 1.39] | 0.80 [0.72, 0.85] | 12.56 | <0.001 |
Effects of brumation on relative tissue size in males, using different temperature thresholds.
Phylogenetically controlled relationships (λ = phylogenetic scaling parameter) between the brumation period and the relative male tissue mass in breeding condition (N = 116 species), with a 2 or 4°C buffer relative to Appendix 1—table 7. All associations are controlled for snout-vent length, confidence intervals are bootstrapped (N = 100 simulations).
Response | Predictors | β [95% CI] | Partial r [95% CI] | t | P | λ [95% CI] |
---|---|---|---|---|---|---|
Brumation with 2 °C temperature buffer (df = 113) | ||||||
Brain | Brumation | –0.08 [-0.14,–0.04] | –0.30 [-0.45,–0.12] | –3.29 | 0.001 | 0.35 [0.00, 0.61] |
SVL | 0.65 [ 0.59, 0.71] | 0.91 [ 0.87, 0.93] | 22.65 | <0.001 | ||
Body fat | Brumation | 0.17 [ 0.02, 0.29] | 0.21 [ 0.03, 0.37] | 2.29 | 0.024 | 0.44 [0.00, 0.69] |
SVL | 1.56 [ 1.38, 1.75] | 0.87 [ 0.83, 0.90] | 18.79 | <0.001 | ||
Digestive tract | Brumation | 0.03 [-0.09, 0.12] | 0.04 [-0.14, 0.22] | 0.43 | 0.667 | 0.00 [0.00, 0.11] |
SVL | 1.12 [ 1.01, 1.25] | 0.86 [ 0.81, 0.89] | 17.91 | <0.001 | ||
Heart | Brumation | –0.10 [-0.24, 0.01] | –0.14 [-0.31, 0.05] | –1.45 | 0.15 | 0.23 [0.00, 0.51] |
SVL | 1.35 [ 1.21, 1.51] | 0.87 [ 0.83, 0.90] | 18.84 | <0.001 | ||
Lungs | Brumation | –0.09 [-0.25, 0.03] | –0.11 [-0.29, 0.07] | –1.22 | 0.226 | 0.23 [0.00, 0.51] |
SVL | 1.32 [ 1.16, 1.50] | 0.83 [ 0.78, 0.87] | 16.13 | <0.001 | ||
Liver | Brumation | 0.01 [-0.11, 0.11] | 0.02 [-0.17, 0.20] | 0.17 | 0.867 | 0.00 [0.00, 0.11] |
SVL | 1.24 [ 1.13, 1.37] | 0.88 [ 0.84, 0.91] | 19.53 | <0.001 | ||
Kidneys | Brumation | –0.01 [-0.11, 0.08] | –0.01 [-0.19, 0.17] | –0.10 | 0.924 | 0.00 [0.00, 0.11] |
SVL | 1.24 [ 1.14, 1.36] | 0.90 [ 0.87, 0.92] | 22.33 | <0.001 | ||
Spleen | Brumation | –0.12 [-0.30, 0.02] | –0.13 [-0.30, 0.05] | –1.39 | 0.167 | 0.24 [0.00, 0.52] |
SVL | 1.34 [ 1.16, 1.55] | 0.80 [ 0.74, 0.85] | 14.38 | <0.001 | ||
Foreleg muscles | Brumation | –0.09 [-0.21, 0.01] | –0.13 [-0.30, 0.05] | –1.43 | 0.156 | 0.00 [0.00, 0.11] |
SVL | 1.53 [ 1.42, 1.66] | 0.91 [ 0.88, 0.93] | 23.80 | <0.001 | ||
Hindleg muscles | Brumation | –0.16 [-0.28,–0.08] | –0.28 [-0.43,–0.10] | –3.07 | 0.003 | 0.25 [0.00, 0.54] |
SVL | 1.35 [ 1.24, 1.48] | 0.91 [ 0.88, 0.93] | 23.46 | <0.001 | ||
Testes | Brumation | 0.25 [ 0.12, 0.39] | 0.31 [ 0.13, 0.46] | 3.46 | <0.001 | 0.75 [0.37, 0.89] |
SVL | 1.23 [ 1.02, 1.42] | 0.78 [ 0.71, 0.83] | 13.31 | <0.001 | ||
Brumation with 4 °C temperature buffer (df = 113) | ||||||
Brain | Brumation | –0.09 [-0.14,–0.05] | –0.33 [-0.47,–0.16] | –3.72 | <0.001 | 0.34 [0.00, 0.61] |
SVL | 0.65 [ 0.59, 0.71] | 0.91 [ 0.88, 0.93] | 23.01 | <0.001 | ||
Body fat | Brumation | 0.14 [ 0.00, 0.26] | 0.17 [-0.01, 0.34] | 1.86 | 0.065 | 0.44 [0.00, 0.69] |
SVL | 1.56 [ 1.38, 1.76] | 0.87 [ 0.82, 0.90] | 18.63 | <0.001 | ||
Digestive tract | Brumation | 0.03 [-0.08, 0.12] | 0.05 [-0.14, 0.22] | 0.48 | 0.63 | 0.00 [0.00, 0.11] |
SVL | 1.12 [ 1.02, 1.25] | 0.86 [ 0.81, 0.89] | 17.98 | <0.001 | ||
Heart | Brumation | –0.07 [-0.19, 0.04] | –0.10 [-0.27, 0.09] | –1.05 | 0.295 | 0.23 [0.00, 0.52] |
SVL | 1.35 [ 1.21, 1.50] | 0.87 [ 0.83, 0.90] | 18.67 | <0.001 | ||
Lungs | Brumation | –0.12 [-0.26, 0.00] | –0.15 [-0.32, 0.03] | –1.66 | 0.099 | 0.24 [0.00, 0.53] |
SVL | 1.33 [ 1.17, 1.51] | 0.84 [ 0.78, 0.87] | 16.25 | <0.001 | ||
Liver | Brumation | 0.01 [-0.11, 0.10] | 0.01 [-0.17, 0.19] | 0.10 | 0.92 | 0.00 [0.00, 0.11] |
SVL | 1.24 [ 1.14, 1.37] | 0.88 [ 0.84, 0.91] | 19.63 | <0.001 | ||
Kidneys | Brumation | 0.00 [-0.10, 0.08] | –0.01 [-0.19, 0.18] | –0.06 | 0.956 | 0.00 [0.00, 0.11] |
SVL | 1.24 [ 1.15, 1.35] | 0.90 [ 0.87, 0.93] | 22.41 | <0.001 | ||
Spleen | Brumation | –0.11 [-0.26, 0.03] | –0.12 [-0.29, 0.07] | –1.25 | 0.213 | 0.23 [0.00, 0.52] |
SVL | 1.34 [ 1.16, 1.54] | 0.80 [ 0.74, 0.85] | 14.34 | <0.001 | ||
Foreleg muscles | Brumation | –0.10 [-0.21,–0.01] | –0.15 [-0.32, 0.04] | –1.56 | 0.121 | 0.00 [0.00, 0.11] |
SVL | 1.53 [ 1.42, 1.66] | 0.91 [ 0.89, 0.93] | 23.95 | <0.001 | ||
Hindleg muscles | Brumation | –0.15 [-0.25,–0.07] | –0.26 [-0.42,–0.09] | –2.91 | 0.004 | 0.27 [0.00, 0.55] |
SVL | 1.35 [ 1.23, 1.48] | 0.91 [ 0.88, 0.93] | 23.24 | <0.001 | ||
Testes | Brumation | 0.22 [ 0.08, 0.34] | 0.28 [ 0.10, 0.43] | 3.10 | 0.002 | 0.76 [0.39, 0.90] |
SVL | 1.22 [ 1.01, 1.42] | 0.78 [ 0.70, 0.83] | 13.05 | <0.001 |
Species-specific environmental effects on the duration of the breeding season.
CV = coefficient of variation, P2T = duration of the dry season (in months), with a month defined as dry when its total precipitation is less than two times the mean temperature. All analyses based on df = 41. The regression slope β is presented with its bootstrapped confidence interval, λ is the phylogenetic scaling parameter.
Predictor | β [95% CI] | r [95% CI] | t | P | λ [95% CI] |
---|---|---|---|---|---|
Brumation | –8.12 [-11.70,–5.01] | –0.57 [-0.72,–0.33] | –4.47 | <0.001 | 0.00 [0.00, 0.38] |
Latitude | –4.86 [-8.95,–1.50] | –0.34 [-0.56,–0.05] | –2.33 | 0.025 | 0.00 [0.00, 0.39] |
Longitude | 3.43 [-0.94, 8.44] | 0.24 [-0.06, 0.49] | 1.59 | 0.119 | 0.00 [0.00, 0.37] |
Elevation | –5.56 [-9.84,–1.61] | –0.39 [-0.60,–0.10] | –2.73 | 0.009 | 0.00 [0.00, 0.38] |
Mean temperature | 5.65 [1.78, 9.93] | 0.40 [0.11, 0.60] | 2.78 | 0.008 | 0.00 [0.00, 0.31] |
Mean precipitation | 3.62 [-0.75, 7.62] | 0.26 [-0.05, 0.50] | 1.69 | 0.098 | 0.00 [0.00, 0.40] |
CV temperature | –6.06 [-10.14,–2.06] | –0.43 [-0.62,–0.15] | –3.03 | 0.004 | 0.00 [0.00, 0.42] |
CV precipitation | –1.44 [-5.70, 2.87] | –0.10 [-0.38, 0.20] | –0.65 | 0.518 | 0.00 [0.00, 0.42] |
P2T | 2.73 [-1.28, 7.59] | 0.19 [-0.11, 0.45] | 1.25 | 0.217 | 0.00 [0.00, 0.40] |
Species-specific environmental effects on the duration of the breeding season when accounting for brumation duration.
CV = coefficient of variation, P2T = duration of the dry season (in months), with a month defined as dry when its total precipitation is less than two times the mean temperature. All analyses based on df = 40. The regression slope β is presented with its bootstrapped confidence interval, λ is the phylogenetic scaling parameter.
Predictors | β [95% CI] | r [95% CI] | t | P | λ [95% CI] |
---|---|---|---|---|---|
Latitude | 2.01 [ –2.72, 6.60] | 0.12 [-0.19, 0.40] | 0.77 | 0.448 | 0.00 [0.00, 0.40] |
Brumation | –9.56 [-14.76,–4.87] | –0.50 [-0.67,–0.23] | –3.64 | <0.001 | |
Longitude | 1.35 [ –2.38, 4.50] | 0.11 [-0.20, 0.39] | 0.71 | 0.482 | 0.00 [0.00, 0.34] |
Brumation | –7.76 [-10.87,–4.37] | –0.54 [-0.70,–0.29] | –4.09 | <0.001 | |
Elevation | –2.12 [ –5.76, 1.96] | –0.16 [-0.43, 0.15] | –1.02 | 0.313 | 0.00 [0.00, 0.57] |
Brumation | –7.09 [-12.59,–3.16] | –0.48 [-0.66,–0.20] | –3.41 | 0.001 | |
Temperature | 2.45 [ –1.38, 5.77] | 0.19 [-0.12, 0.45] | 1.20 | 0.235 | 0.00 [0.00, 0.46] |
Brumation | –7.00 [-11.08,–3.04] | –0.48 [-0.66,–0.21] | –3.44 | 0.001 | |
Precipitation | 0.23 [ –3.31, 3.77] | 0.02 [-0.28, 0.31] | 0.12 | 0.909 | 0.00 [0.00, 0.27] |
Brumation | –8.02 [-11.26,–4.34] | –0.53 [-0.69,–0.27] | –3.95 | <0.001 | |
CV temperature | –2.41 [ –6.91, 1.41] | –0.18 [-0.44, 0.13] | –1.13 | 0.267 | 0.00 [0.00, 0.46] |
Brumation | –6.83 [-10.85,–2.64] | –0.45 [-0.64,–0.17] | –3.19 | 0.003 | |
CV precipitation | 0.64 [ –2.76, 3.95] | 0.05 [-0.25, 0.34] | 0.34 | 0.739 | 0.00 [0.00, 0.49] |
Brumation | –8.28 [-11.95,–4.51] | –0.57 [-0.72,–0.32] | –4.36 | <0.001 | |
P2T | 1.20 [ –2.12, 4.46] | 0.10 [-0.21, 0.38] | 0.64 | 0.524 | 0.00 [0.00, 0.44] |
Brumation | –7.89 [-10.79,–3.66] | –0.56 [-0.71,–0.31] | –4.23 | <0.001 |
Comparison of candidate models in a phylogenetic path analysis linking environmental variation to brumation duration and the breeding patterns (breeding season and aggregations).
All candidate models are ranked according to their C-statistic Information Criterion (CICc). Those with ΔCICc < 2 are highlighted in bold and were used to calculate the average model, which is depicted in Appendix 1—figure 4 along with all hypotheses.
Model | k | q | C | P | CICc | ΔCICc | wi |
---|---|---|---|---|---|---|---|
m5 | 3 | 7 | 3.32 | 0.77 | 20.52 | 0.00 | 1.00 |
m4 | 2 | 8 | 1.72 | 0.79 | 21.95 | 1.43 | 0.49 |
m1 | 1 | 9 | 0.29 | 0.87 | 23.74 | 3.22 | 0.20 |
m3 | 2 | 8 | 15.06 | 0.00 | 35.29 | 14.77 | 0.00 |
m6 | 3 | 7 | 22.03 | 0.00 | 39.23 | 18.71 | 0.00 |
m2 | 3 | 7 | 35.60 | 0.00 | 52.80 | 32.28 | 0.00 |
m8 | 3 | 7 | 60.09 | 0.00 | 77.29 | 56.77 | 0.00 |
m7 | 2 | 8 | 83.01 | 0.00 | 103.25 | 82.73 | 0.00 |
-
k = number of independence claims; q = number of parameters; C = Fisher’s C statistics; CICc = C-statistic Information Criterion; ΔCICc = difference in CICc from the best-fitting model; wi = CICc weight.
Correlation matrix between the compositional data of the four focal traits and the rest of the body.
The upper triangle lists the correlation coefficients with 95% confidence intervals (controlling for phylogeny), the lower triangle the corresponding P-values before (upper) and after (lower) correction for multiple testing based on the false discovery rate. Statistically significant correlations are highlighted in bold.
Brain | Body fat | Testes | Hindlimb muscles | Rest | |
---|---|---|---|---|---|
Brain | –0.79 [-0.83,–0.71] | –0.36 [-0.50,–0.19] | –0.08 [-0.26, 0.10] | 0.30 [0.12, 0.44] | |
Body fat | <0.001 < 0.001 | –0.06 [-0.23, 0.12] | –0.03 [-0.20, 0.15] | –0.17 [-0.34, 0.01] | |
Testes | <0.001 < 0.001 | 0.531 0.590 | –0.54 [-0.64,–0.40] | –0.57 [-0.67,–0.44] | |
Hindlimb muscles | 0.370 0.527 | 0.776 0.776 | <0.001 < 0.001 | 0.07 [-0.11, 0.24] | |
Rest | 0.001 0.002 | 0.060 0.101 | <0.001 < 0.001 | 0.466 0.582 |
Results of the phylogenetic principal component analysis.
Shown are the eigenvalues and percent of the total variance explained by the four principal components, as well as the loadings of the original variables. Loadings >0.25 are highlighted in bold. Phylogenetic scaling parameter λ = 0.75.
PC1 | PC2 | PC3 | PC4 | |
---|---|---|---|---|
Eigenvalues | 3.38 | 0.32 | 0.21 | 0.09 |
% variance | 84.4 | 8.0 | 5.2 | 2.3 |
Loadings | ||||
Brain | –0.91 | 0.31 | 0.26 | –0.12 |
Body fat | –0.93 | –0.07 | –0.31 | –0.16 |
Testes | –0.88 | –0.44 | 0.18 | 0.04 |
Hindlimb muscles | –0.95 | 0.18 | –0.11 | 0.23 |
Phylogenetic path analysis linking brumation to relative trait investments.
All candidate models are ranked according to their CICc. Those with ΔCICc < 2 are highlighted in bold and were used to calculate the average model (see Figure 4 in main text). For candidate models and averaged path coefficients see Appendix 1—figures 6 and 7.
Model | k | q | C | P | CICc | ΔCICc | wi |
---|---|---|---|---|---|---|---|
m11 | 4 | 17 | 8.28 | 0.41 | 48.50 | 0.00 | 0.25 |
m25 | 1 | 20 | 0.99 | 0.61 | 49.80 | 1.31 | 0.13 |
m23 | 1 | 20 | 1.28 | 0.53 | 50.10 | 1.59 | 0.11 |
m24 | 1 | 20 | 1.28 | 0.53 | 50.10 | 1.59 | 0.11 |
m3 | 3 | 18 | 7.44 | 0.28 | 50.50 | 1.96 | 0.09 |
m12 | 3 | 18 | 7.53 | 0.28 | 50.60 | 2.05 | 0.09 |
m9 | 5 | 16 | 13.48 | 0.20 | 51.00 | 2.45 | 0.07 |
m21 | 2 | 19 | 6.52 | 0.16 | 52.40 | 3.91 | 0.03 |
m4 | 2 | 19 | 6.68 | 0.15 | 52.60 | 4.08 | 0.03 |
m1 | 4 | 17 | 12.64 | 0.13 | 52.90 | 4.35 | 0.03 |
m10 | 4 | 17 | 12.72 | 0.12 | 53.00 | 4.44 | 0.03 |
m22 | 2 | 19 | 7.54 | 0.11 | 53.50 | 4.93 | 0.02 |
m2 | 3 | 18 | 11.88 | 0.06 | 54.90 | 6.41 | 0.01 |
m26 | 3 | 18 | 14.45 | 0.03 | 57.50 | 8.98 | 0.00 |
m7 | 4 | 17 | 23.32 | 0.00 | 63.60 | 15.04 | 0.00 |
m19 | 4 | 17 | 23.95 | 0.00 | 64.20 | 15.66 | 0.00 |
m8 | 3 | 18 | 22.57 | 0.00 | 65.60 | 17.09 | 0.00 |
m5 | 5 | 16 | 28.52 | 0.00 | 66.00 | 17.49 | 0.00 |
m14 | 3 | 18 | 23.59 | 0.00 | 66.60 | 18.11 | 0.00 |
m13 | 4 | 17 | 26.53 | 0.00 | 66.80 | 18.25 | 0.00 |
m20 | 3 | 18 | 24.03 | 0.00 | 67.10 | 18.56 | 0.00 |
m6 | 4 | 17 | 27.76 | 0.00 | 68.00 | 19.48 | 0.00 |
m16 | 5 | 16 | 36.26 | 0.00 | 73.80 | 25.23 | 0.00 |
m27 | 5 | 16 | 37.41 | 0.00 | 74.90 | 26.38 | 0.00 |
m15 | 3 | 18 | 34.57 | 0.00 | 77.60 | 29.10 | 0.00 |
m17 | 4 | 17 | 37.68 | 0.00 | 77.90 | 29.39 | 0.00 |
m28 | 6 | 15 | 50.57 | 0.00 | 85.40 | 36.85 | 0.00 |
m18 | 4 | 17 | 59.08 | 0.00 | 99.30 | 50.80 | 0.00 |
-
k = number of independence claims; q = number of parameters; C = Fisher’s C statistics; CICc = C-statistic Information Criterion; ΔCICc = difference in CICc from the best-fitting model; wi = CICc weight.
Effect of the predominant habitat of each species on the relative amount of body fat in the context of the brain−fat trade-off hypothesis.
Output of PGLS models examining the effect of the predominant species-specific habitat (and so mode of locomotion) on the relative amount of body fat, accounting for brain mass and brumation duration as other sources of variation. Three different models were conducted: (1) using raw species means with log body fat as the response variable and controlling for body size by including log snout-vent length as a covariate; (2) using the compositional data (centred log ratio of both brain mass as the response and brain mass); and (3) using PC3 of the principal component analysis, which was primarily loaded by brain mass (+0.26) and body fat (−0.31; Figure 3). Statistically significant effects are highlighted in bold, and the different habitat types are compared against aquatic species as the reference in the intercept.
Predictors | β [95% CI] | r [95% CI] | t | P |
---|---|---|---|---|
Raw species means (df = 109, λ=0.17 [0.00, 0.51]) | ||||
(Intercept) | –12.97 [-17.49,–8.41] | –0.44 [-0.57,–0.28] | –5.12 | <0.001 |
Log Brain mass | 0.50 [0.12, 0.94] | 0.18 [0.00, 0.35] | 1.94 | 0.055 |
Habitat [arboreal] | –0.97 [-1.49,–0.38] | –0.3 [-0.45,–0.12] | –3.31 | 0.001 |
Habitat [semiaquatic] | –0.02 [-0.31, 0.35] | –0.01 [-0.19, 0.18] | –0.09 | 0.925 |
Habitat [terrestrial] | –0.3 [-0.83, 0.35] | –0.11 [-0.28, 0.08] | –1.12 | 0.264 |
Brumation | 0.22 [0.09, 0.37] | 0.27 [0.09, 0.42] | 2.90 | 0.005 |
Log SVL | 2.94 [2.13, 3.76] | 0.52 [0.37, 0.63] | 6.34 | <0.001 |
Compositional data (df = 110, λ=0.01 [0.00, 0.03]) | ||||
(Intercept) | –1.94 [-2.14,–1.69] | –0.86 [-0.89,–0.82] | –17.96 | <0.001 |
Brain mass | –0.61 [-0.74,–0.52] | –0.68 [-0.76,–0.58] | –9.81 | <0.001 |
Habitat [arboreal] | –0.28 [-0.51,–0.06] | –0.22 [-0.38,–0.04] | –2.39 | 0.018 |
Habitat [semiaquatic] | –0.10 [-0.31, 0.06] | –0.10 [-0.28, 0.09] | –1.07 | 0.286 |
Habitat [terrestrial] | 0.01 [-0.19, 0.20] | 0.01 [-0.17, 0.19] | 0.12 | 0.901 |
Brumation | 0.08 [0.01, 0.17] | 0.19 [0.00, 0.35] | 1.98 | 0.050 |
PC3 (df = 111, λ=0.20 [0.00, 0.59]) | ||||
(Intercept) | –0.03 [-0.26, 0.16] | –0.06 [-0.24, 0.12] | –0.27 | 0.785 |
Habitat [arboreal] | 0.31 [0.12, 0.52] | 0.26 [0.08, 0.42] | 2.83 | 0.006 |
Habitat [semiaquatic] | –0.01 [-0.15, 0.13] | –0.01 [-0.19, 0.17] | –0.10 | 0.920 |
Habitat [terrestrial] | 0.10 [-0.06, 0.30] | 0.09 [-0.09, 0.27] | 0.99 | 0.326 |
Brumation | –0.02 [-0.08, 0.02] | –0.08 [-0.26, 0.10] | –0.88 | 0.381 |
Data availability
Source data and associated codes are available at figshare https://doi.org/10.6084/m9.figshare.21078052. In the source data, annual mean temperature and total precipitation are retrieved from https://www.meteoblue.com (Source data 1), and daily mean temperatures are retrieved from http://www.lishi.tianqi.com (Source data 5).
-
figshareData from: How hibernation in frogs drives brain and reproductive evolution in opposite directions.https://doi.org/10.6084/m9.figshare.21078052
References
-
The statistical analysis of compositional dataJournal of the Royal Statistical Society 44:139–160.https://doi.org/10.1111/j.2517-6161.1982.tb01195.x
-
Unraveling global impacts of climate change on amphibians distributions: a life-history and biogeographic-based approachFrontiers in Ecology and Evolution 10:987237.https://doi.org/10.3389/fevo.2022.987237
-
Effectsize: estimation of effect size indices and standardized parametersJournal of Open Source Software 5:2815.https://doi.org/10.21105/joss.02815
-
Amphibian breeding and climate changeConservation Biology 15:1804–1809.https://doi.org/10.1046/j.1523-1739.2001.00307.x
-
Climate change and amphibian declines: is there a link?Diversity and Distributions 9:111–121.https://doi.org/10.1046/j.1472-4642.2003.00011.x
-
mvMORPH: an R package for fitting multivariate evolutionary models to morphometric dataMethods in Ecology and Evolution 6:1311–1319.https://doi.org/10.1111/2041-210X.12420
-
Comparative studies of brain evolution: a critical insight from the ChiropteraBiological Reviews of the Cambridge Philosophical Society 84:161–172.https://doi.org/10.1111/j.1469-185X.2008.00067.x
-
Sex determination: the amphibian modelsReproduction, Nutrition, Development 44:539–549.https://doi.org/10.1051/rnd:2004062
-
BookThe Colour Handbook of the Amphibians of SichuanChengdu: China Forestry Publishing House.
-
Environmental temperature alters the digestive performance and gut microbiota of a terrestrial amphibianThe Journal of Experimental Biology 221:jeb187559.https://doi.org/10.1242/jeb.187559
-
Phylogenetic analysis and comparative data: a test and review of evidenceThe American Naturalist 160:712–726.https://doi.org/10.1086/343873
-
BookAn introduction to Phylogenetic path analysisIn: Garamszegi LZ, editors. Modern Phylogenetic Comparative Methods and Their Application in Evolutionary Biology.. Berlin: Springer-Verlag. pp. 201–229.https://doi.org/10.1007/978-3-662-43550-2_8
-
Being fat and smart: A comparative analysis of the fat-brain trade-off in mammalsJournal of Human Evolution 100:25–34.https://doi.org/10.1016/j.jhevol.2016.09.001
-
Hibernation constrains brain size evolution in mammalsJournal of Evolutionary Biology 31:1582–1588.https://doi.org/10.1111/jeb.13353
-
A linear-time algorithm for Gaussian and non-Gaussian trait evolution modelsSystematic Biology 63:397–408.https://doi.org/10.1093/sysbio/syu005
-
BookClimate Change 2022: Impacts, Adaptation, and VulnerabilityCambridge: Cambridge University Press.https://doi.org/10.1017/9781009325844
-
Costs of encephalization: the energy trade-off hypothesis tested on birdsJournal of Human Evolution 51:228–243.https://doi.org/10.1016/j.jhevol.2006.03.006
-
The expensive brain: a framework for explaining evolutionary changes in brain sizeJournal of Human Evolution 57:392–400.https://doi.org/10.1016/j.jhevol.2009.04.009
-
Anuran brain size predicts food availability-driven population densityScience China. Life Sciences 66:415–417.https://doi.org/10.1007/s11427-022-2177-2
-
Ordinary least squares regression is indicated for studies of allometryJournal of Evolutionary Biology 30:4–12.https://doi.org/10.1111/jeb.12986
-
Brain size affects female but not male survival under predation threatEcology Letters 18:646–652.https://doi.org/10.1111/ele.12441
-
Feeding innovations and forebrain size in birdsAnimal Behaviour 53:549–560.https://doi.org/10.1006/anbe.1996.0330
-
Brains, innovations and evolution in birds and primatesBrain, Behavior and Evolution 63:233–246.https://doi.org/10.1159/000076784
-
Sperm competition and brain size evolution in mammalsJournal of Evolutionary Biology 22:2215–2221.https://doi.org/10.1111/j.1420-9101.2009.01837.x
-
How sperm competition shapes the evolution of testes and sperm: a meta-analysisPhilosophical Transactions of the Royal Society of London. Series B, Biological Sciences 375:20200064.https://doi.org/10.1098/rstb.2020.0064
-
Relative brain size is predicted by the intensity of intrasexual competition in frogsThe American Naturalist 196:169–179.https://doi.org/10.1086/709465
-
Ratio of central nervous system to body metabolism in vertebrates: its constancy and functional basisAmerican Journal of Physiology-Regulatory, Integrative and Comparative Physiology 241:R203–R212.https://doi.org/10.1152/ajpregu.1981.241.3.R203
-
Compositional data analysis in the study of carcass composition of beef cattleLivestock Production Science 67:241–251.https://doi.org/10.1016/S0301-6226(00)00200-1
-
Physiological ecology of winter hibernation by the high-altitude frog Nanorana parkeriPhysiological and Biochemical Zoology 95:201–211.https://doi.org/10.1086/718764
-
BookSpermatogenesis and male reproductive system in Amphibia—AnuraIn: Ogielska M, editors. Reproduction of Amphibians. Science Publishers. pp. 34–99.https://doi.org/10.1201/9781482280135
-
Detecting correlated evolution on phylogenies: a general method for the comparative analysis of discrete charactersProceedings of the Royal Society of London. Series B, Biological Sciences 255:37–45.https://doi.org/10.1098/rspb.1994.0006
-
BookEstivation and HibernationIn: Feder ME, Burggren WW, editors. Environmental Physiology of the Amphibians.. Chicago: University of Chicago Press. pp. 250–274.
-
Mating system and brain size in batsProceedings of the Royal Society of London. Series B, Biological Sciences 273:719–724.https://doi.org/10.1098/rspb.2005.3367
-
Energy expenditure and testosterone in free-living male yellow-pine chipmunksThe Journal of Experimental Zoology 292:460–467.https://doi.org/10.1002/jez.10085
-
Posterior summarization in bayesian phylogenetics using tracer 1.7Systematic Biology 67:901–904.https://doi.org/10.1093/sysbio/syy032
-
SoftwareR: A language and environment for statistical computingR Foundation for Statistical Computing, Vienna, Austria.
-
phytools: an R package for phylogenetic comparative biology (and other things)Methods in Ecology and Evolution 3:217–223.https://doi.org/10.1111/j.2041-210X.2011.00169.x
-
The rate of digestion in cold-blooded vertebrates. —The influence of season and temperatureAmerican Journal of Physiology-Legacy Content 24:447–458.https://doi.org/10.1152/ajplegacy.1909.24.5.447
-
phangorn: phylogenetic analysis in RBioinformatics 27:592–593.https://doi.org/10.1093/bioinformatics/btq706
-
BookStrategies of digestion and DefecationIn: Townsend CR, Calow P, editors. Physiological Ecology: An Evolutionary Approach to Resource Use.. Blackwell Scientific Publications. pp. 109–139.
-
BookThe cognitive-buffer hypothesis for the evolution of large brainsIn: Dukas R, Ratcliff JR, editors. Cognitive Ecology II. Chicago: University of Chicago Press. pp. 111–134.https://doi.org/10.7208/chicago/9780226169378.001.0001
-
Metabolic flexibility: hibernation, torpor, and estivationComprehensive Physiology 6:737–771.https://doi.org/10.1002/cphy.c140064
-
Overwintering behavior of adult bullfrogs, Rana catesbeiana, in northeastern OhioThe Ohio Journal of Science 94:8–13.
-
rptR: repeatability estimation and variance decomposition by generalized linear mixed‐effects modelsMethods in Ecology and Evolution 8:1639–1644.https://doi.org/10.1111/2041-210X.12797
-
The costs of a big brain: extreme encephalization results in higher energetic demand and reduced hypoxia tolerance in weakly electric African fishesProceedings of the Royal Society of London. Series B, Biological Sciences 283:20162157.https://doi.org/10.1098/rspb.2016.2157
-
MEGA6: molecular evolutionary genetics analysis version 6.0Molecular Biology and Evolution 30:2725–2729.https://doi.org/10.1093/molbev/mst197
-
Physiological ecology of aquatic overwintering in ranid frogsBiological Reviews of the Cambridge Philosophical Society 83:119–140.https://doi.org/10.1111/j.1469-185X.2008.00035.x
-
How should relative changes be measured?The American Statistician 39:43–46.https://doi.org/10.1080/00031305.1985.10479385
-
“compositions”: a unified R package to analyze compositional dataComputers and Geosciences 34:320–338.https://doi.org/10.1016/j.cageo.2006.11.017
-
Effects of seasonality on brain size evolution: evidence from strepsirrhine primatesThe American Naturalist 176:758–767.https://doi.org/10.1086/657045
-
The social behaviour of anuran amphibiansAnimal Behaviour 25:666–693.https://doi.org/10.1016/0003-3472(77)90118-X
-
BookThe Ecology and Behavior of Amphibians.Chicago: University of Chicago Press.https://doi.org/10.7208/chicago/9780226893334.001.0001
Article and author information
Author details
Funding
National Natural Science Foundation of China (32370456)
- Wenbo Liao
National Natural Science Foundation of China (31970393)
- Wenbo Liao
National Natural Science Foundation of China (31772451)
- Wenbo Liao
Natural Science Foundation of Sichuan Province (22NSFSC0011)
- Wenbo Liao
Swiss National Science Foundation (PP00P3_170669)
- Stefan Lüpold
Swiss National Science Foundation (PP00P3_202662)
- Stefan Lüpold
The funders had no role in study design, data collection, and interpretation, or the decision to submit the work for publication.
Acknowledgements
We thank K Isler and C van Schaik for helpful comments on an earlier draft of our manuscript and J Santin for insightful discussions. Financial support was provided by the National Natural Sciences Foundation of China (32370456, 31970393 and 31772451 to WBL), the Key Project of Natural Science Foundation of Sichuan Province (22NSFSC0011 to WBL) and by the Swiss National Science Foundation (PP00P3_170669 and PP00P3_202662 to SL).
Version history
- Sent for peer review: April 21, 2023
- Preprint posted: April 24, 2023 (view preprint)
- Preprint posted: July 27, 2023 (view preprint)
- Preprint posted: November 6, 2023 (view preprint)
- Version of Record published: December 12, 2023 (version 1)
Cite all versions
You can cite all versions using the DOI https://doi.org/10.7554/eLife.88236. This DOI represents all versions, and will always resolve to the latest one.
Copyright
© 2023, Liao et al.
This article is distributed under the terms of the Creative Commons Attribution License, which permits unrestricted use and redistribution provided that the original author and source are credited.
Metrics
-
- 766
- views
-
- 61
- downloads
-
- 2
- citations
Views, downloads and citations are aggregated across all versions of this paper published by eLife.
Download links
Downloads (link to download the article as PDF)
Open citations (links to open the citations from this article in various online reference manager services)
Cite this article (links to download the citations from this article in formats compatible with various reference manager tools)
Further reading
-
- Evolutionary Biology
Enantiornithines were the dominant birds of the Mesozoic, but understanding of their diet is still tenuous. We introduce new data on the enantiornithine family Bohaiornithidae, famous for their large size and powerfully built teeth and claws. In tandem with previously published data, we comment on the breadth of enantiornithine ecology and potential patterns in which it evolved. Body mass, jaw mechanical advantage, finite element analysis of the jaw, and traditional morphometrics of the claws and skull are compared between bohaiornithids and living birds. We find bohaiornithids to be more ecologically diverse than any other enantiornithine family: Bohaiornis and Parabohaiornis are similar to living plant-eating birds; Longusunguis resembles raptorial carnivores; Zhouornis is similar to both fruit-eating birds and generalist feeders; and Shenqiornis and Sulcavis plausibly ate fish, plants, or a mix of both. We predict the ancestral enantiornithine bird to have been a generalist which ate a wide variety of foods. However, more quantitative data from across the enantiornithine tree is needed to refine this prediction. By the Early Cretaceous, enantiornithine birds had diversified into a variety of ecological niches like crown birds after the K-Pg extinction, adding to the evidence that traits unique to crown birds cannot completely explain their ecological success.
-
- Evolutionary Biology
The RNA world hypothesis proposes that during the early evolution of life, primordial genomes of the first self-propagating evolutionary units existed in the form of RNA-like polymers. Autonomous, non-enzymatic, and sustained replication of such information carriers presents a problem, because product formation and hybridization between template and copy strands reduces replication speed. Kinetics of growth is then parabolic with the benefit of entailing competitive coexistence, thereby maintaining diversity. Here, we test the information-maintaining ability of parabolic growth in stochastic multispecies population models under the constraints of constant total population size and chemostat conditions. We find that large population sizes and small differences in the replication rates favor the stable coexistence of the vast majority of replicator species (‘genes’), while the error threshold problem is alleviated relative to exponential amplification. In addition, sequence properties (GC content) and the strength of resource competition mediated by the rate of resource inflow determine the number of coexisting variants, suggesting that fluctuations in building block availability favored repeated cycles of exploration and exploitation. Stochastic parabolic growth could thus have played a pivotal role in preserving viable sequences generated by random abiotic synthesis and providing diverse genetic raw material to the early evolution of functional ribozymes.