An in silico FSHD muscle fiber for modeling DUX4 dynamics and predicting the impact of therapy
Figures
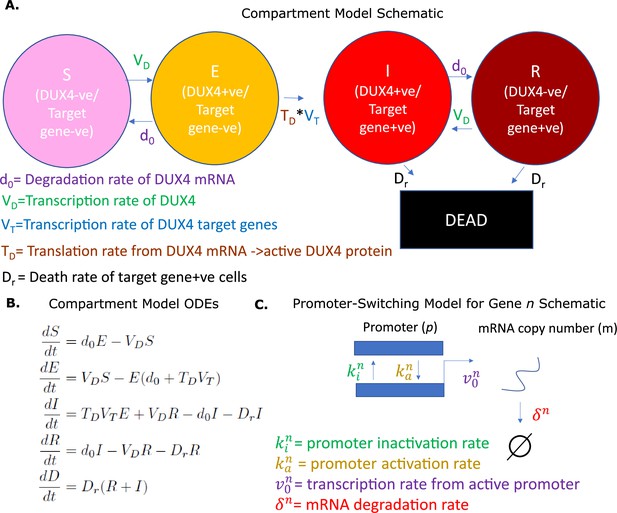
Overview of models.
(A) Schematic of the compartment model describing the transition between five Facioscapulohumeral muscular dystrophy (FSHD) states according to five underlying parameters. (B) Ordinary differential equations describing the compartment model. (C) Schematic of the promoter-switching model of gene expression.
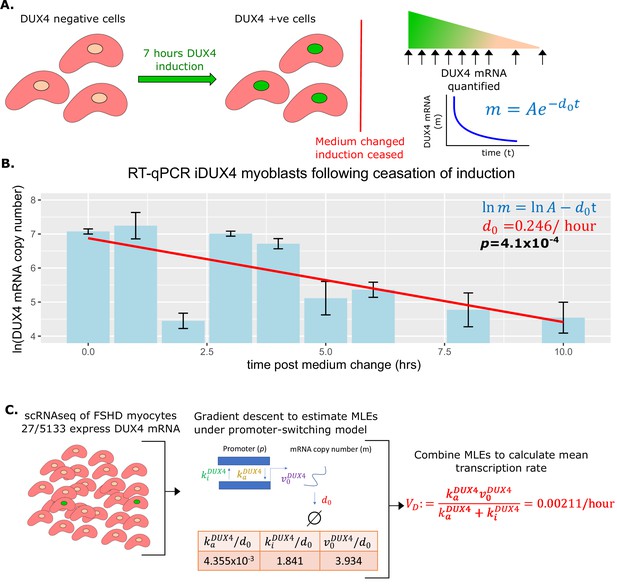
Estimation of DUX4 mRNA degradation rate and average transcription rate .
(A) Schematic of the experiment performed to estimate the DUX4 mRNA degradation rate . iDUX4 myoblasts were induced to express DUX4 with 250 ng/ml of doxycycline for 7 hr before doxycycline was washed away. DUX4 mRNA was quantified at multiple time points post-wash using RT-qPCR. (B) Bar chart displays the RT-qPCR of ln(DUX4 copy number) at post-wash times 0, 1, 2, 3, 4, 5, 6, 8, and 10 hr. Bar represents the average of triplicates and the standard error of the mean is displayed. A line of best fit of ln(DUX4 copy number) against time is displayed alongside the corresponding linear regression p-value (bold) and the slope of the line corresponds to (red). (C) Schematic of the estimation of average DUX4 transcription rate from scRNAseq data of 5133 Facioscapulohumeral muscular dystrophy (FSHD) myocytes. The maximum likelihood estimates (MLEs) for the underlying parameters of DUX4 transcription under the promoter-switching model are estimated via gradient descent and combined to estimate the average DUX4 transcription rate (red text).
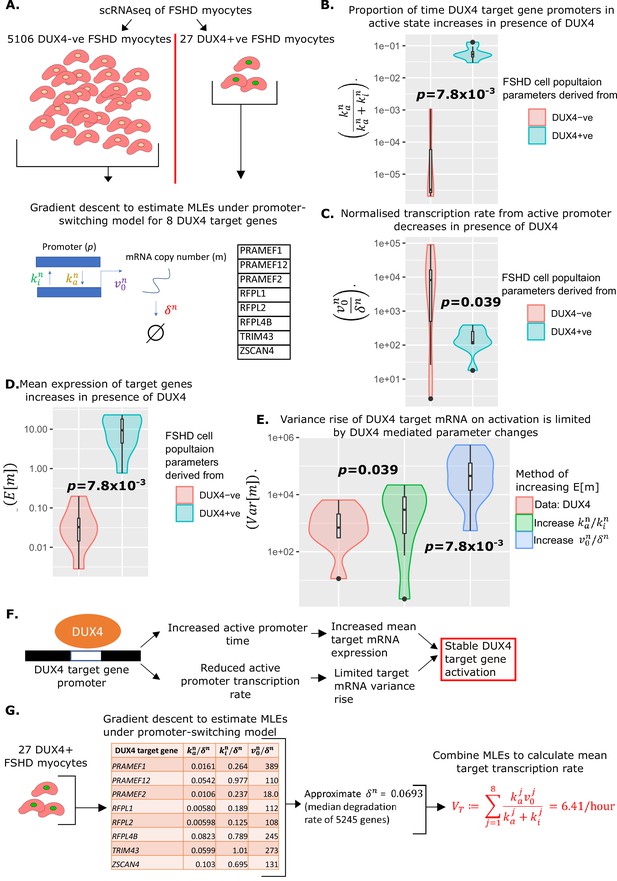
Estimation of promoter-switching model parameters for DUX4 target genes and estimation of .
(A) Schematic of estimation of promoter-switching model parameters for eight DUX4 target genes, across 5106 DUX4 -ve Facioscapulohumeral muscular dystrophy (FSHD) single myocytes and 27 DUX4 +ve FSHD single myocytes from van den Heuvel et al., 2019. Violin plots display (B) the proportion of time in active promoter state (C) normalized active promoter transcription rate and (D) the mean mRNA copy number, for the eight DUX4 target genes in the 5106 DUX4 -ve and 27 DUX4 +ve FSHD myocytes separately, p-values correspond to two-tailed paired Wilcoxon tests. (E) Violin plot displays the variance of mRNA copy number for the eight DUX4 target genes calculated from the 27 DUX4 +ve FSHD myocytes (red) and calculated assuming DUX4 up-regulates targets only via the increase in (blue) the normalized transcription rate or (green) the ratio of active to inactive promoter transition rates . Paired two-tailed Wilcoxon p-values are displayed comparing adjacent distributions. (F) Schematic displaying how the change in parameters underlying the promoter-switching models for the eight DUX4 target genes in the presence of DUX4 leads to stable target gene activation. (G) Schematic of the estimation of the average DUX4 target gene transcription rate , from scRNAseq data of 27 DUX4 +ve FSHD myocytes.
-
Figure 3—source data 1
Source data for Figure 3 provides the parameters of the promoter switching model for each of the 8 DUX4 targets, derived from 5106 DUX4 -ve FSHD single cells and 27 DUX4 +vs FSHD single cells seperately.
- https://cdn.elifesciences.org/articles/88345/elife-88345-fig3-data1-v2.csv
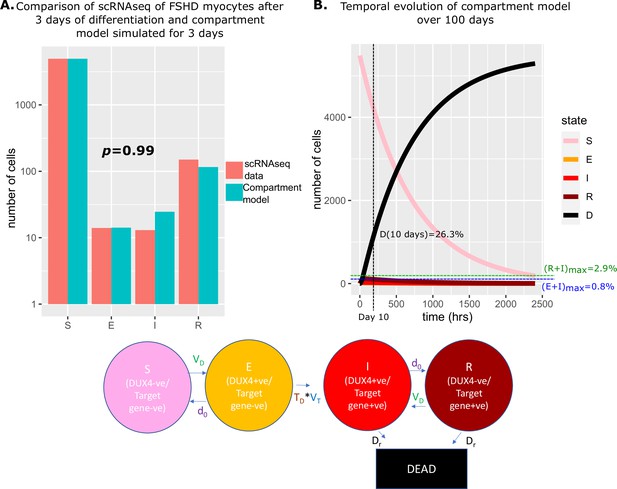
Simulation of the compartment model and comparison with scRNAseq data of unfused Facioscapulohumeral muscular dystrophy (FSHD) myocytes.
(A) Bar chart displays the number of cells in each live cell compartment (blue) in our model following simulation over 3 days using experimentally estimated parameters and (red) in 5133 single FSHD myocytes. A Chi-squared goodness of fit p-value tests the alternate hypothesis that the two distributions are different. (B) Line plot displaying how the number of cells in each of the five compartments of the model changes over 100 days from a starting state of 5133 (1+0.07) cells. The percentage of cells dead after 10 days is displayed alongside the maximum percentage of live cells which are DUX4 +ve (E+I)max and DUX4 target gene +ve (R+I)max.
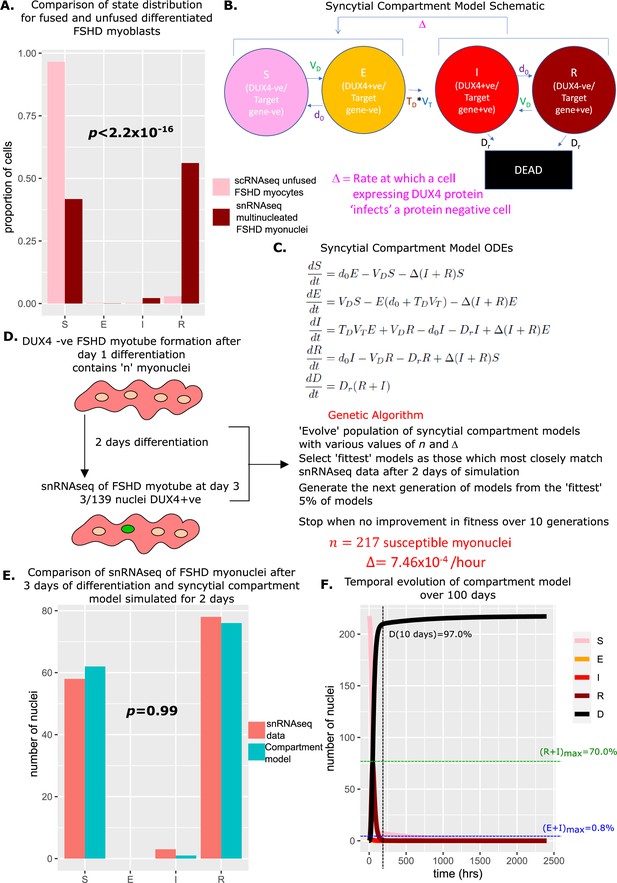
Derivation and simulation of the syncytial compartment model and comparison with snRNAseq data of fused Facioscapulohumeral muscular dystrophy (FSHD) myonuclei.
(A) Bar chart displays the proportion of cells in each live compartment of our model in (pink) 5133 unfused FSHD single myocytes from van den Heuvel et al., 2019 and (dark red) 139 fused FSHD single myonuclei of Jiang et al., 2020. A Chi-squared goodness of fit p-value tests the alternate hypothesis that the two distributions are different. (B) Schematic of the syncytial compartment model, allowing DUX4 protein permissive states I and R, to ‘infect’ non-permissive states S and E. (C) Ordinary differential equations describing the syncytial compartment model. (D) Schematic of the genetic algorithm employed to estimate the DUX4 diffusion rate Δ and the starting population size for the syncytial compartment model from 139 fused FSHD single myonuclei. (E) Bar chart displays the number of cells in each live cell compartment (blue) in our syncytial compartment model following simulation over 2 days using experimentally estimated parameters and (red) in 139 fused single FSHD myonuclei. A Chi-squared goodness of fit p-value tests the alternate hypothesis that the two distributions are different. (F) Line plot displaying how the number of cells in each of the five compartments of the syncytial compartment model changes over 100 days from a starting state of cells. The percentage of cells dead after 10 days is displayed alongside the maximum percentage of live cells which are DUX4 +ve (E+I)max and DUX4 target gene +ve (R+I)max.
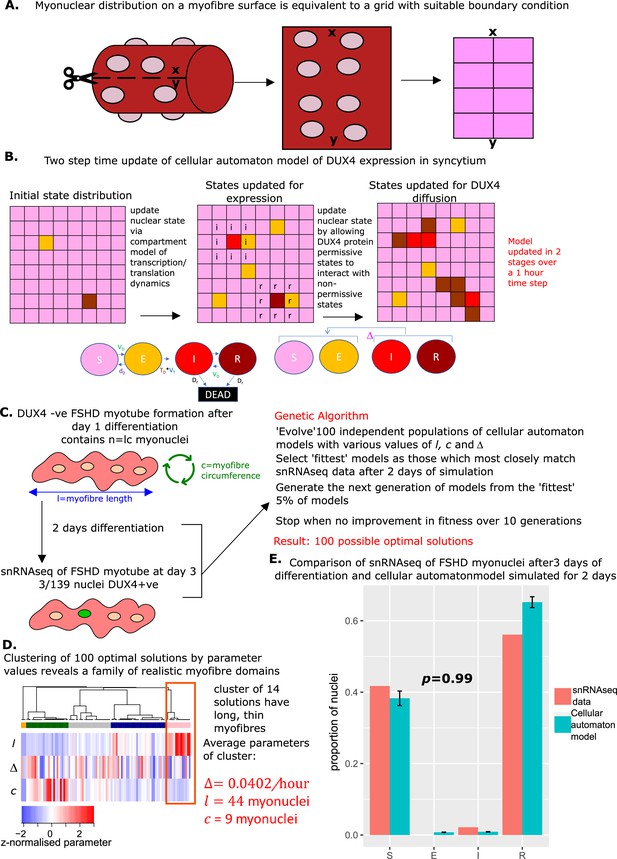
Derivation and simulation of the cellular automaton model and comparison with snRNAseq data of fused Facioscapulohumeral muscular dystrophy (FSHD) myonuclei.
(A) Schematic demonstrating how with suitable boundary conditions a grid can be topologically equivalent to a cylinder, and thus encapsulate dynamics on the surface of a myofiber. (B) Schematic of the cellular automaton model of DUX4 expression in a syncytium with grid squares representing single myonuclei updating in 1 hr time-steps via a two-stage process. (C) Schematic of the genetic algorithm employed to estimate the DUX4 diffusion rate Δ, myotube length , and myotube circumference for the cellular automaton model from 139 fused FSHD single myonuclei. (D) Heatmap displays the clustering solution of the 100 optimal parameter regimes and Δ produced by fitting 100 genetic algorithms to 139 fused FSHD myonuclei. The highlighted cluster represents a parameter regime in which myotubes are long and thin, the average parameter values of this cluster are displayed in red. (E) Bar chart displays the average proportion of cells in each live cell compartment (blue) in our cellular automaton model following simulation over 2 days using experimentally estimated parameters, alongside standard error of the mean, and (red) in 139 fused single FSHD myonuclei. A Chi-squared goodness of fit p-value tests the alternate hypothesis that the two distributions are different.
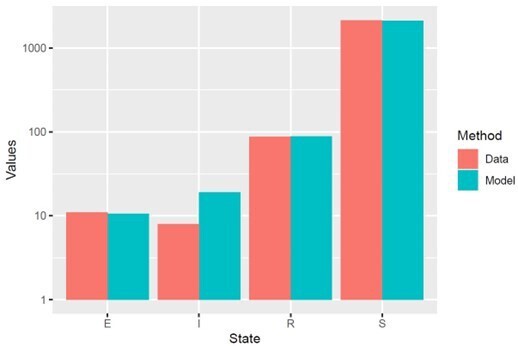
Model simulation with VD derived for patient FSHD1.
1, using appropriate starting cell numbers for this patient: Model vs Data Chi Squared p=0.99.
Tables
Reagent type (species) or resource | Designation | Source or reference | Identifiers | Additional information |
---|---|---|---|---|
Cell line (human) | LHCN-M2-iDUX4 | 10.1038/s41598-018-35150-8 | Immortalized doxycycline-inducible DUX4 expressing human myoblast cell line | |
Sequence-based reagent | DUX4 Fwd | 10.1038/s41598-018-35150-8 | qPCR pimer | ACCTCTCCTAGAAACGGAGGC |
Sequence-based reagent | DUX4 Rev | 10.1038/s41598-018-35150-8 | qPCR primer | CAGCAGAGCCCGGTATTCTTC |
Commercial assay or kit | Quantitect reverse transcription kit | Qiagen, 205311 | RT-kit | |
Commercial assay or kit | Takyon SYBR Green qPCR Mastermix | UF-NSMT-B101 | qPCR Mastermix |
Additional files
-
Supplementary file 1
Demographics of Facioscapulohumeral muscular dystrophy (FSHD) patients assayed in scRNAseq data.
For each FSHD patient described in the scRNAseq dataset of van den Heuvel et al., 2019, we list sex, diagnosis, genotype, number of DUX4 +ve/-ve cells, and % of DUX4 +ve cells. For patients FSHD1.1 and FSHD2.1 where sufficient data was available, patient-specific estimates of are also given.
- https://cdn.elifesciences.org/articles/88345/elife-88345-supp1-v2.xlsx
-
Supplementary file 2
Promoter switching model parameter estimates for DUX4 target genes in patients FSHD1.1 and FSHD2.1.
For each of the eight DUX4 target genes considered the three parameters underlying the promoter switching model (, and ) derived from DUX4 +ve and DUX4-ve cells separately for patients FSHD1.1 and FSHD2.1 separately are presented.
- https://cdn.elifesciences.org/articles/88345/elife-88345-supp2-v2.xlsx
-
MDAR checklist
- https://cdn.elifesciences.org/articles/88345/elife-88345-mdarchecklist1-v2.docx