Archaic introgression contributed to shape the adaptive modulation of angiogenesis and cardiovascular traits in human high-altitude populations from the Himalayas
Figures
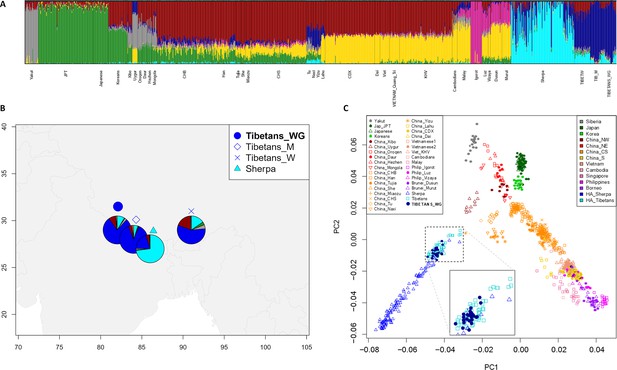
Population structure analyses performed on the extended dataset including Tibetan, Sherpa, and lowland East-Asian individuals.
(A) Admixture analysis showed the best predictive accuracy when seven (K = 7) population clusters were tested. Populations included in the dataset are labelled according to population names and acronyms reported in Supplementary file 1a. (B) Map showing geographic location and admixture proportions at K = 7 of the high-altitude groups included in the extended dataset. The label Tibetans_WG indicates whole-genome sequence data for individuals of Tibetan ancestry analysed in the present study. Additional information about the considered samples (e.g., number of individuals per group, reference study, and used abbreviations) are reported in Supplementary file 1a. (C) Principal components analysis (PCA) plot considering PC1 vs PC2 and summarizing genomic divergence between high-altitude Tibetan/Sherpa people and the cline of variation observable for lowland East-Asian populations. The enlarged square displays clustering between Tibetan samples sequenced for the whole genome (i.e., blue dots) and Tibetan samples characterized by genome-wide data (i.e., light-blue squares).
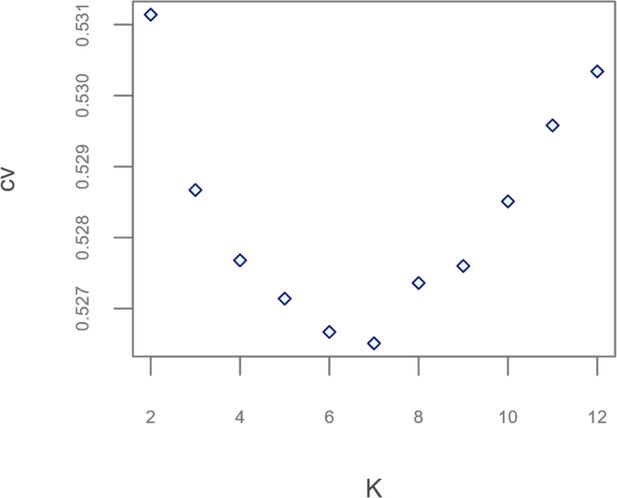
Scatterplot showing the number of possible population clusters (K) tested by the different ADMIXTURE runs performed and the cross-validation (CV) errors associated to them.

Admixture analyses performed on the extended dataset for K = 2 to K = 12.
Numbers of K increase from the top to the bottom of the plot and the considered populations are named according to the labels reported in Supplementary file 1a.
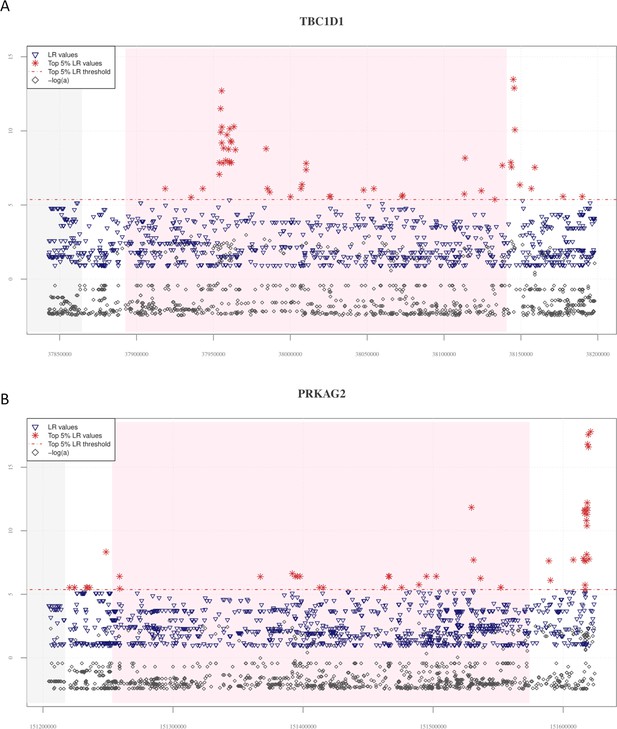
Distribution of VolcanoFinder statistics suggestive of putative adaptive introgrossed loci across the TBC1D1 and PRKAG2 genomic regions.
On the x-axis are reported genomic positions of each single-nucleotide variant (SNV), while on the y-axis are displayed the related statistics obtained. Pink background indicates the chromosomal interval occupied by the considered genes, while the grey background identifies those genes (i.e., PGM2 in the TBC1D1 downstream genomic region and the RHEB gene in the upstream PRKAG2 region) possibly involved in regulatory transcription mechanisms. The dashed red line identifies the threshold set to filter for significant likelihood ratio (LR) values (i.e., top 5% of LR values). For both these genomic regions, the distribution of LR and −logα are concordant with those observed at the EPAS1 positive control for adaptive introgression (AI). (A) A total of 50 significant LR values (red stars) and −logα (grey diamonds) values resulted collectively elevated in both the TBC1D1 gene and its downstream genomic regions. A remarkable concentration of significant LR values characterizing 19 SNVs was especially observable in the first portion of the gene. (B) The entire PRKAG2 genomic region was found to comprise 46 SNVs showing significant LR values, with the greatest peaks being located in the downstream region associated to such gene. Peaks detected for the LR statistic are accompanied by peaks of −logα values.

Distribution of VolcanoFinder statistics across the EPAS1 and EGLN1 positive and negative controls for adaptive introgression.
In the plots, the chart base reports genomic positions of variants, pink background indicates the starting and the ending positions of the genes, while the grey background identifies those genes (i.e., the LINC02583 gene in the EPAS1 downstream genomic region, the SPRTN gene in the upstream EGLN1 region) possibly involved in regulatory transcription mechanisms. The dashed red line identifies the significant threshold set to filter likelihood ratio (LR) values (i.e., top 5% LR values). (A) The 19 single-nucleotide variants (SNVs) showing significant LR values (i.e., red stars) resulted closely distributed in the ending portion of the EPAS1 gene and in both up- and downstream genomic regions flanking such locus. The −logα values (i.e., grey diamonds) appeared consistently distributed in the entire EPAS1 region. A similar a pattern is observed also for the TBC1D1, PRKAG2, and RASGRF2 new candidate AI genes, as reported in Figure 2A, B and in Figure 2—figure supplement 3. (B) The EGLN1 genomic region is characterized by only three LR significant values among which only one strongly deviates from the significant LR threshold. Several SNVs distributed in the EGLN1 starting portion, as well as in its flaking regions, showed elevated −logα values supporting the action of natural selection on them in the considered Tibetan population.
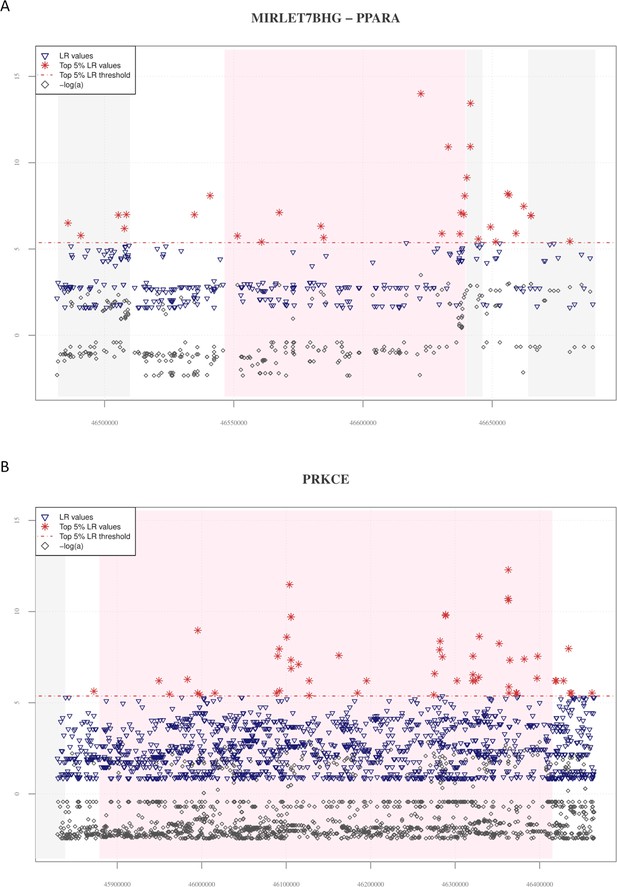
Distribution of VolcanoFinder statistics across MIRLET7BHG, PPARA, and PRKCE genes.
In the plots, the chart base reports genomic positions of variants, pink background indicates the starting and the ending positions of the PPARA and PRKCE genes, while the grey background identifies those loci (i.e., MIRLET7BHG long non-coding, CDPF1 and TTC38 genes located in PPARA up- and downstream regions and the SRBD1 gene located in the PRKCE upstream genomic region, respectively) possibly involved in regulatory transcription mechanisms. The red horizontal dashed line displayed the significant threshold set for filtering LR significant values. (A) A total of 32 single-nucleotide variants (SNVs) showed significant LR values (red stars) covering all the genomic region considered. LR greatest peaks are observed in the ending portion of the PPARA gene and in the CDPF1 gene. Collectively, the genomic regions comprising significant LR scores also showed elevated −logα values (grey diamonds). (B) The 55 significant LR scores and the elevated −logα values cover the entire region of the PRKCE gene (i.e., a gene located in a genomic region nearby to EPAS1).
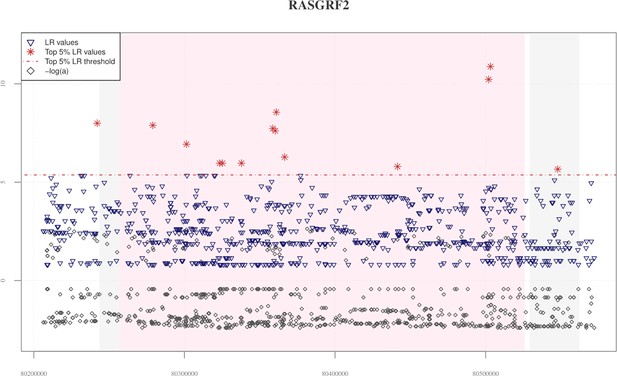
Distribution of VolcanoFinder statistics across the RASGRF2 candidate adaptive introgression (AI) gene.
The chart base reports genomic positions of variants, pink background indicates the starting and the ending positions of the RASGRF2 gene, while the grey background identifies those genes (i.e., RASGRF2-AS1 antisense RNA gene and CKMT2 located in the RASGRF2 up- and downstream regions, respectively) possibly involved in regulatory transcription mechanisms. The red horizontal dashed line displayed the significant threshold set for filtering likelihood ratio (LR) significant values. A total of 14 significant LR values (red stars) were equally distributed across the entire genomic region considered, with the greatest peak recovered in the ending portion of the RASGRF2 gene. Elevated peaks of −logα are observable in both RASGRF2 gene and its flanking genomic regions. Such a pattern resulted in line with that observed for the EPAS1 positive control for AI, as reported in Figure 2—figure supplement 1, and for both TBC1D1 and PRKAG2 genomic regions, as reported in Figure 2A, B.

Distribution of VolcanoFinder statistics across the KRAS candidate adaptive introgression (AI) gene.
The pink and the grey rectangulars represent the portion of the genome covered by the KRAS and ETFRF1 genes, respectively. Although only three significant likelihood ratio (LR) values can be observed for such a genomic region, the KRAS gene was included in our set of new candidate AI genes because it was confirmed by all the subsequent validation analyses performed and according with previous evidenced advanced by Hu et al., 2017 and Browning et al., 2018, which suggest that a portion of variants included in the KRAS gene, as well as in its downstream region, shows signatures of archaic Denisovan introgression in both Tibetan and CHB populations. Elevated −logα values are instead consistently distributed across the KRAS gene and its surrounding genomic regions.
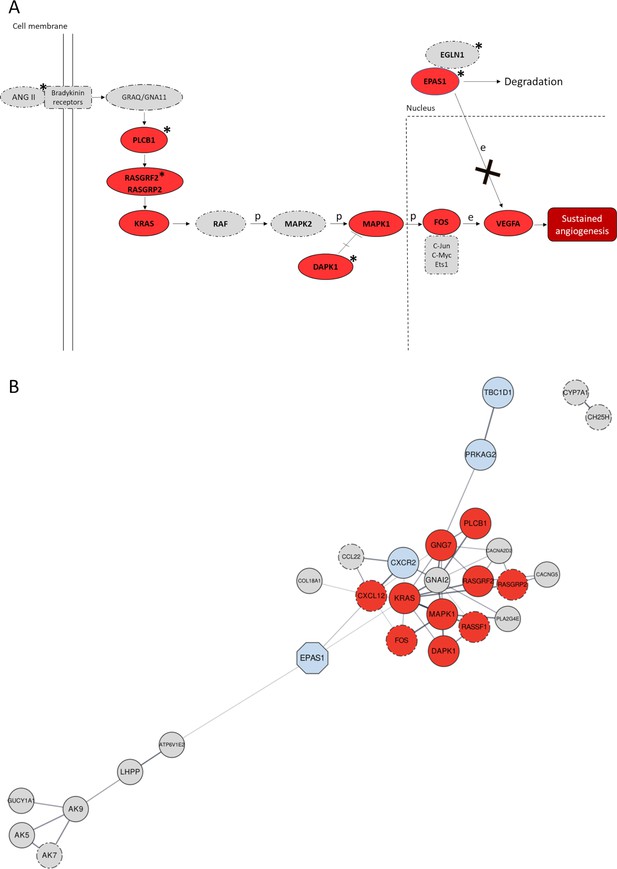
Significant gene networks including Denisovan-like derived alleles according to the Signet analysis.
(A) Schematic representation of the activation of the RAS/MAPK(ERK) axis after interaction of the bradykinin receptors with their ligands (e.g., ANG II) within the framework of the Pathways in Cancer network. Genes supported by both Signet (i.e., belonging to the significant network associated to Pathways in cancer) and VolcanoFinder (i.e., including at least a single-nucleotide variant (SNV) showing likelihood ratio (LR) value within top 5% of the obtained results) analyses as potentially introgressed loci, are highlighted in red and present solid outline. Grey circles with dotted-dashed contour instead indicate genes supported only by Signet, while loci marked with stars are those including genomic windows showing LASSI T statistic within top 5% of the related distribution. After the interaction between ANG II (active enzyme angiotensin II) and bradykinin receptors, activation of the Ras protein encoded by KRAS mediated by RAS-GTPases (e.g., RASGRF2) comports a series of phosphorylation reactions that eventually promotes angiogenesis (Kranenburg et al., 2004). In detail, phosphorylation of the MAPK1 protein and prevention of MAPK1-DAPK-1-dependent apoptosis leads to increased MAPK1 activity (Kanehisa and Goto, 2000; Stevens et al., 2007) that causes improved FOS mRNA expression (Monje et al., 2005). FOS together with other proteins (e.g., Jun) forms the AP-1 transcription factor, which bounds to the VEGF promoter region upregulating its expression in endothelial cells (Catar et al., 2013) and sustaining angiogenesis when the hypoxia inducible factor 1 (HIF-1) signalling cascade is inhibited (Lorenzo et al., 2014). (B) Gene network built by setting co-expression as force function and by displaying the entire set of genes identified by the Signet algorithm as belonging to significant pathways including Denisovan-like derived alleles. Genes whose variation pattern was supported by both VolcanoFinder and Signet analyses (e.g., TBC1D1) as shaped by archaic introgression are displayed with a solid black outline. The EPAS1 positive control locus that has been previously proved to have mediated adaptive introgression in Tibetan populations was represented as light-blue octagonal. Genes included in pathways involved in angiogenesis (e.g., RASGRF2) and/or activated in hypoxic conditions (e.g., PRKAG2) are reported as dark red and light-blue circles, respectively, while the remining fraction of significant genes are represented as light-grey circles. The closeness or the distance between all nodes reflects the tendency to be co-expressed with each other and all the connections inferred are characterized by a confident score ≥0.7.
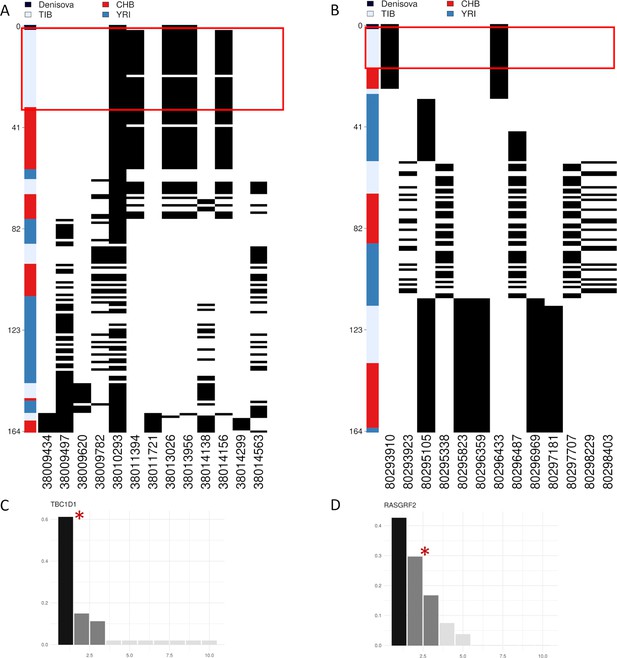
Representation of genetic distances between modern and archaic haplotypes and barplots showing haplotype frequency spectra for TBC1D1 and RASGRF2 candidate adaptive introgression (AI) genes.
Haplotypes are reported in rows, while derived (i.e., black square) and ancestral (i.e., white square) alleles are displayed in columns. Haplotypes are ranked from top to bottom according to their number of pairwise differences with respect to the Denisovan sequence. (A) Heatmap displaying divergence between Tibetan, CHB and YRI TBC1D1 haplotypes with respect to the Denisovan genome. A total of 33 TBC1D1 haplotypes (i.e., 61% of the overall haplotypes inferred for such a region) belonging to individuals with Tibetan ancestry are plotted in the upper part of the heatmap thus presenting the smallest number of pairwise differences with respect to the Denisovan sequence. (B) Heatmap displaying divergence between Tibetan, CHB and YRI RASGRF2 haplotypes with respect to the Denisovan genome. A total of 16 Tibetan haplotypes in the RASGRF2 genomic region present no differences with respect to the Denisovan sequence. As regards barplots, on the x-axis are reported the haplotypes detected in the considered genomic windows, while on the y-axis is indicated the frequency for each haplotype. The black and dark-grey bars indicate the more frequent haplotypes (i.e., the putative adaptive haplotypes inferred by the LASSI method), while red stars mark those haplotypes carrying Denisovan-like derived alleles. (C) TBC1D1 haplotype frequency spectrum. The TBC1D1 gene presents a haplotype pattern qualitatively comparable to that observed at EPAS1 (Figure 4—figure supplement 3A), with a predominant haplotype carrying archaic derived alleles and reaching elevated frequencies in Tibetan populations. In line with this observation, such a pattern was inferred by LASSI as conformed with a non-neutral evolutionary scenario, even if it seems to be characterized by a soft rather than a hard selective sweep due to the occurrence of three sweeping haplotypes. (D) RASGRF2 haplotype frequency spectrum. A soft selective sweep was inferred also for the considered RASGRF2 genomic window, although frequencies reached by the sweeping haplotypes turned out to be more similar with each other. The second most represented haplotype was that carrying the archaic derived alleles and, reached a frequency of 29% in the Tibetan group.

Haplotype frequency spectra of the top windows detected as adaptively evolved by LASSI in the EPAS1 and EGLN1 genomic regions.
Barplots showing haplotype frequency spectra in the genomic windows associated with the highest T value and linked to (A) EPAS1 and (B) EGLN1 genes. The x-axis reports the haplotypes detected in the windows, while on y-axes are indicated frequencies of each haplotype. For these windows the haplotype frequency spectra clearly reflect the pattern of diversity expected under the hard selective sweeps model in which a single predominant haplotype carrying adaptive variants (i.e., sweeping haplotypes represented with the black bars) reaches elevated frequencies in the population.
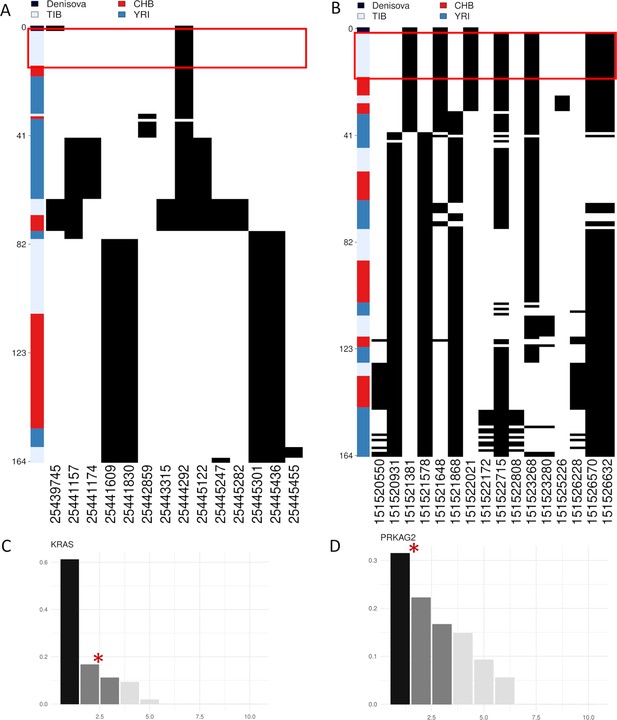
Representation of genetic distances between modern and archaic haplotypes.
Heatmap displaying the divergence between Tibetan, CHB and YRI KRAS and PRKAG2 haplotypes with respect to the Denisovan sequence. Haplotypes are reported in rows, while derived (i.e., black square) and ancestral (i.e., white square) alleles are displayed in columns. Haplotypes are ranked from top to bottom according to their number of pairwise differences with respect to the Denisovan sequence. The red square identifies the cluster of Tibetan haplotypes classiefed by the LASSI method as sweeping haplotypes (i.e., haplotypes with elevated or moderate frequencies and which carry putative adaptive variants). (A) 16% of Tibetan haplotypes inferred for KRAS conformed with a non-neutral evolutionary scenario according to LASSI results and presented the smallest number of pairwise differences with respect to the Denisovan genome, being plotted in the upper part of the heatmap. (B) 33% of Tibetan PRKAG2 haplotypes cluster in the upper part of the heatmap being among the most close haplotypes with respect to the Denisovan sequence and presenting only four pairwise differences with it. Barplots showing haplotype frequency spectrum of KRAS and PRKAG2 windows suggestive of adaptations mediated by soft selective sweeps in Tibetans. In both the plots are reported on the x-axis the haplotypes detected in the considered windows, while on y-axes are indicated the frequencies of each haplotype. The black and dark-grey bars indicate the more frequent haplotypes (i.e., the sweeping haplotypes inferred by LASSI), while the red star marks those haplotypes carrying Denisovan-like derived variants. (C) KRAS presents a pattern qualitatively comparable to that expected for a non-neutral evolution (i.e, positive likelihood T values), with two main haplotypes carrying putative adaptive variants and reaching elevated frequencies in Tibetans. The second sweeping haplotype carries the Denisovan-like derived variant and reaches 16% of frequency. (D) The most frequent sweeping haplotype detected in this PRKAG2 window reches 33% of frequency in Tibetans and carries the Denisovan-like derived variant.

Representation of genetic distances between modern and archaic haplotypes.
Heatmap displaying the divergence between Tibetan, CHB and YRI EPAS1 and EGLN1 haplotypes with respect to the Denisovan sequence. Haplotypes are reported in rows, while derived (i.e., black square) and ancestral (i.e., white square) alleles are displayed in columns. Haplotypes are ranked from top to bottom according to their number of pairwise differences with respect to the Denisovan sequence. The red square identifies the cluster of Tibetan haplotypes classified by LASSI as sweeping haplotypes (i.e., haplotypes with elevated or moderate frequencues which carry putative adaptive variants). (A) The first homogeneus cluster of haplotypes visible in upper part of the heatmap belongs to Tibetan individuals (i.e., light-blue cluster). These haplotypes are among the closest ones to the archaic Denisovan sequence indicated in black, thus confirming archaic introgression at EPAS1. As concerning the haplotypes inferred for the other population in the plot, only one YRI haplotype presents one pairwise difference less than the haplotypes in the first Tibetan cluster. (B) Except for two Tibetan haplotypes, which appeared the closest ones to the archaic reference, the cluster of haplotypes presenting the lowest number of differences with respect to the Denisovan sequence belongs to the Han Chinese population. The most frequent Tibetan haplotype did not present any variant shared with the archaic reference, counting 14 pairwise differences with respect to Denisovan genome and thus not supporting the archaic origin of these EGLN1 variants. Barplots showing the haplotype frequencies spectrum of the (C) EPAS1 and (D) EGLN1 windows suggestive of adaptation mediated by hard selective sweeps in Tibetans. In both the plots are reported on the x-axis the haplotypes detected in the considered windows, while on y-axes are indicated the frequencies of each haplotype. The black and dark-grey bars indicate the more frequent haplotypes (i.e., sweeping haplotypes inferred by LASSI), while the red star marks those sweeping haplotypes carrying Denisovan-like derived variants. For these windows, haplotype frequency spectra clearly reflect patterns expected under the hard selective sweep model in which a single haplotype carrying adaptive variants (i.e., sweeping haplotypes represented with the black bars) reaches elevated frequencies in the population. However, only for EPAS1 such haplotype effetively carries the Denisovan-like derived variant.
Additional files
-
Supplementary file 1
Supplementary tables 1a-1f.
(a) Populations included in the extended dataset. (b) Single-nucleotide variants (SNVs) associated with values falling in top 5% of the distribution of likelihood ratio (LR) statistic calculated by VolcanoFinder. (c) SNVs associated with values falling in top 5% of the distribution of the LR statistic and comprised in the genomic region of the EPAS1 gene (i.e., 2:46474546–2:46663836). (d) SNVs associated with values falling in top 5% of the distribution of the LR statistic and comprised in the genomic region of the EGLN1 gene (i.e., 1:231449502–1:231608033). (e) Adaptive intregressed genes confirmed by VolcanoFinder and previous studies. (f) Gene networks including Denisovan-like derived alleles identified according to the Signet approach.
- https://cdn.elifesciences.org/articles/89815/elife-89815-supp1-v1.xlsx
-
MDAR checklist
- https://cdn.elifesciences.org/articles/89815/elife-89815-mdarchecklist1-v1.pdf