Efficient estimation for large-scale linkage disequilibrium patterns of the human genome
Figures
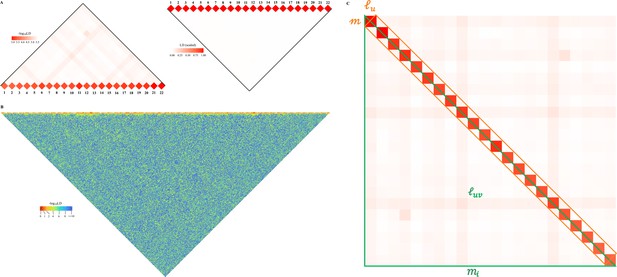
Schematic illustration for large-scale linkage disequilibrium (LD) analysis as exampled for CONVERGE cohort.
(A) The 22 human autosomes have consequently 22 and 231 , without (left) and with (right) scaling transformation; Scaling transformation is given in Equation 8. (B) If zoom into chromosome 2 of 420,946 single-nucleotide polymorphisms (SNPs), a chromosome of relative neutrality is expected to have self-similarity structure that harbors many approximately strong along the diagonal, and relatively weak off-diagonally. Here chromosome 2 of CONVERGE has been split into 1000 blocks and yielded 1000 LD grids, and 499,500 LD grids. (C) An illustration of the construction process for the LD-decay regression model.
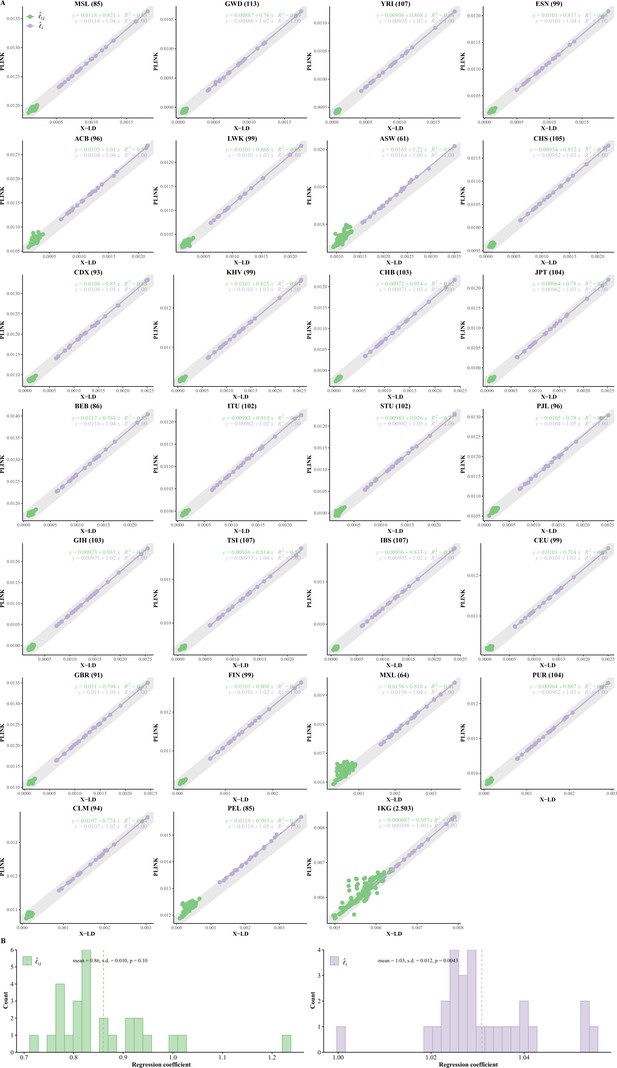
Reconciliation for linkage disequilibrium (LD) estimators in the 26 cohorts of 1KG.
(A) Consistency examination for the 26 1KG cohorts for their and estimated by X-LD and PLINK (--r2). In each figure, the 22 fitting line is in purple, whereas the 231 fitting line is in green. The gray solid line, , in which the sample size of each cohort, represents the expected fit between PLINK and X-LD estimates, and the two estimated regression models at the top-right corner of each plot show this consistency. The sample size of each cohort is in parentheses. (B) Distribution of of and fitting lines is based on X-LD and PLINK algorithms in the 26 cohorts; represents variation explained by the fitted model. 26 1KG cohorts: MSL (Mende in Sierra Leone), GWD (Gambian in Western Division, The Gambia), YRI (Yoruba in Ibadan, Nigeria), ESN (Esan in Nigeria), ACB (African Caribbean in Barbados), LWK (Luhya in Webuye, Kenya), ASW (African Ancestry in Southwest US), CHS (Han Chinese South), CDX (Chinese Dai in Xishuangbanna, China), KHV (Kinh in Ho Chi Minh City, Vietnam), CHB (Han Chinese in Beijing, China), JPT (Japanese in Tokyo, Japan), BEB (Bengali in Bangladesh), ITU (Indian Telugu in the UK), STU (Sri Lankan Tamil in the UK), PJL (Punjabi in Lahore, Pakistan), GIH (Gujarati Indian in Houston, TX), TSI (Toscani in Italia), IBS (Iberian populations in Spain), CEU (Utah residents [CEPH] with Northern and Western European ancestry), GBR (British in England and Scotland), FIN (Finnish in Finland); MXL (Mexican Ancestry in Los Angeles, CA), PUR (Puerto Rican in Puerto Rico), CLM (Colombian in Medellin, Colombia), and PEL (Peruvian in Lima, Peru).

Reconciliation for linkage disequilibrium (LD) estimators in AFR, EAS, and EUR.
In each figure, the 22 fit line is in purple, whereas the 231 fit line is in green. The gray solid line, , in which the sample size, represents the expected fit between PLINK and X-LD, and the two estimated regression models at the top-right corner show this consistency.

The computational efficiency of X-LD algorithm.
Considering the high computational cost of PLINK, only the first chromosome was chosen. In the process of evaluating computational efficiency, we kept adding single-nucleotide polymorphisms (SNPs) until the inclusion of the entire chromosome. The bar chart and line chart show the actual calculation time and theoretical calculation complexity, respectively.
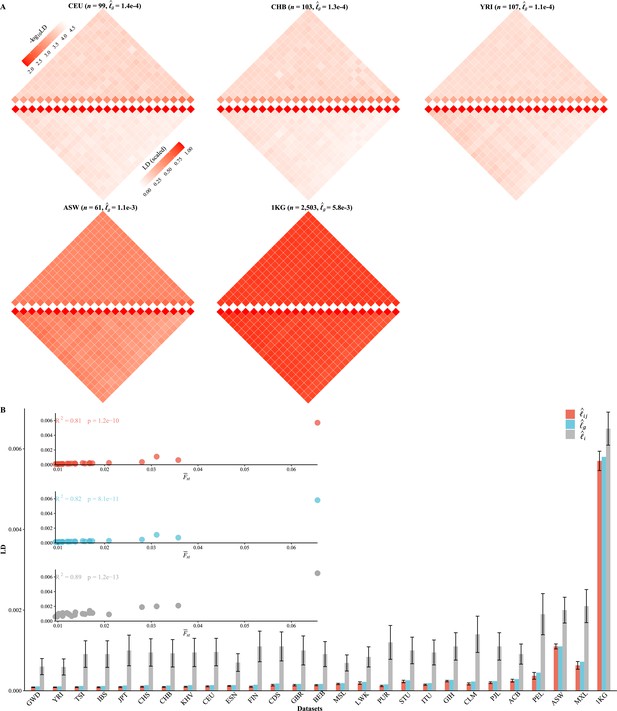
Various linkage disequilibrium (LD) components for the 26 1KG cohorts.
(A) Chromosomal scale LD components for five representative cohorts (CEU, CHB, YRI, ASW, and 1KG). The upper parts of each figure represent (along the diagonal) and (off-diagonal), and the lower part as in Equation 8. For visualization purposes, the quantity of LD before scaling is transformed to a -log10 scale, with smaller values (red hues) representing larger LD, and a value of 0 representing that all single-nucleotide polymorphisms (SNPs) are in LD. (B) The relationship between the degree of population structure (approximated by ) and , , and in the 26 1KG cohorts.
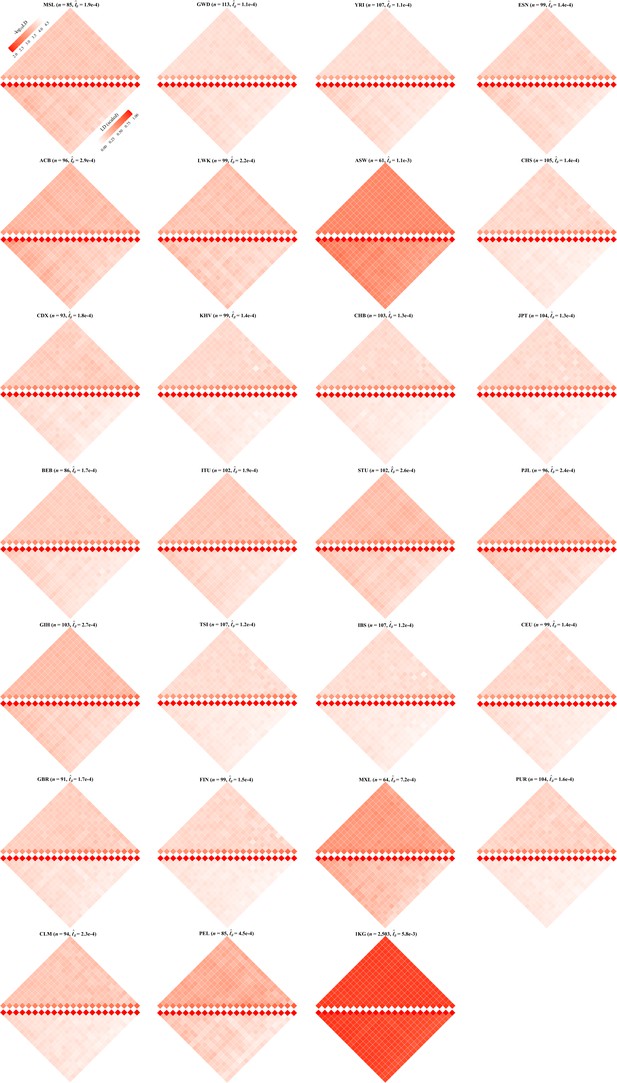
Chromosomal scale linkage disequilibrium (LD) components for 26 cohorts in 1KG.
The upper and lower parts of each figure represent the LD before and after scaling according to Equation 8. and are represented by the diagonal and the off-diagonal elements, respectively. For visualization purposes, LD before scaling is transformed to a -log10-scale, with smaller values (red hues) representing larger LD, and a value of 0 representing that all single-nucleotide polymorphisms (SNPs) are in LD.
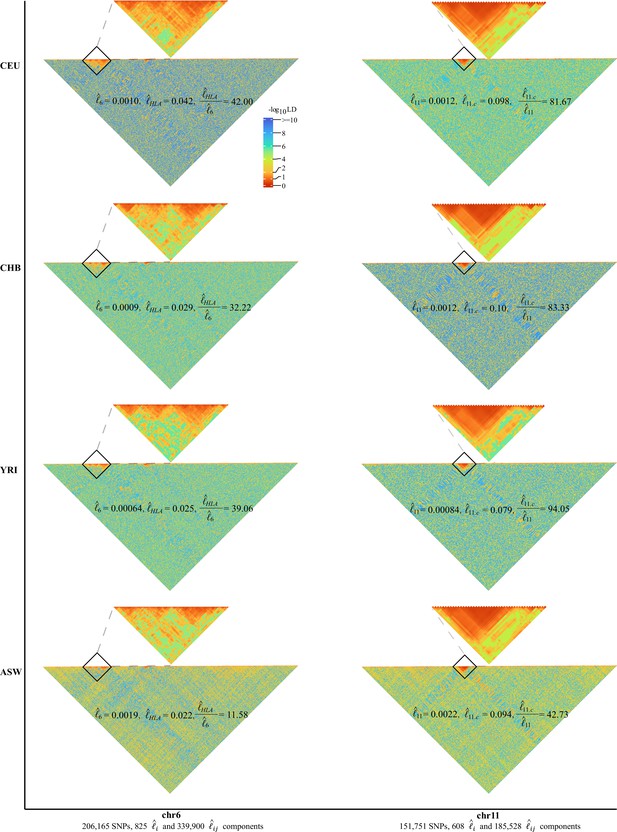
High-resolution illustration for linkage disequilibrium (LD) grids for CEU, CHB, YRI, and ASW ().
For each cohort, we partition chromosomes 6 and 11 into high-resolution LD grids (each LD grid contains 250 ×250 single-nucleotide polymorphism [SNP] pairs). The bottom half of each figure shows the LD grids for the entire chromosome. Further zooming into HLA on chromosome 6 and the centromere region on chromosome 11, and their detailed LD in the relevant regions are also provided in the upper half of each figure. For visualization purposes, LD is transformed to a -log10-scale, with smaller values (red hues) representing larger LD, and a value of 0 representing that all SNPs are in LD.

High-resolution illustration for linkage disequilibrium (LD) grids for CEU, CHB, YRI, and ASW ().
For each cohort, we partitioned each chromosome into consecutive LD grids (each LD grid containing 500 single-nucleotide polymorphisms [SNPs]). For visualization purposes, LD is transformed to a -log10-scale, with smaller values (red hues) representing larger LD, and a value of 0 representing that all SNPs are in LD.

Influence of HLA region on chromosome 6 and centromere region on chromosome 11 on chromosomal linkage disequilibrium (LD) in CEU, CHB, YRI, and ASW.
When another region was removed, to avoid chance, the same number of consecutive single-nucleotide polymorphisms (SNPs) as HLA region or centromere region was randomly removed from the genomic region, and this operation was repeated 100 times.
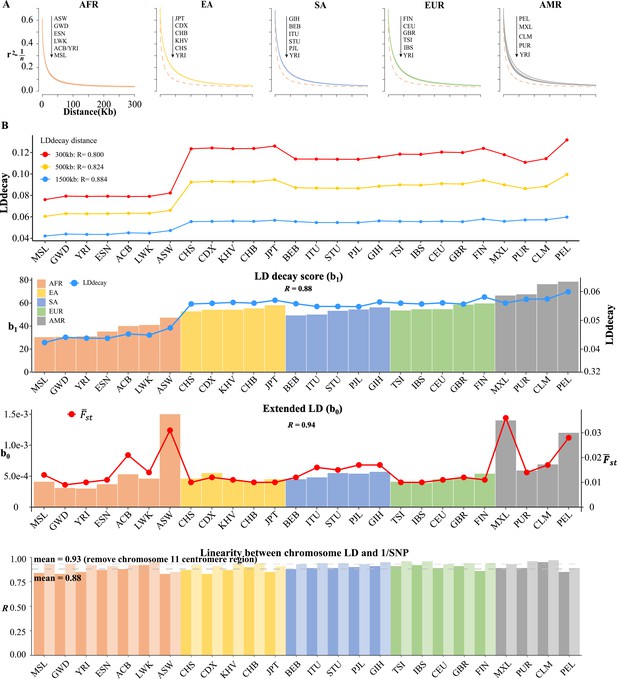
Linkage disequilibrium (LD) decay analysis for 26 1KG cohorts.
(A) Conventional LD decay analysis in PLINK for 26 cohorts. To eliminate the influence of sample size, the inverse of sample size has been subtracted from the original LD values. The YRI cohort, represented by the orange dotted line, is chosen as the reference cohort in each plot. The top-down arrow shows the order of LDdecay values according to Table 5. (B) Model-based LD decay analysis for the 26 1KG cohorts. We regressed each autosomal against its corresponding inversion of the single-nucleotide polymorphism (SNP) number for each cohort. Regression coefficient quantifies the averaged LD decay of the genome and intercept provides a direct estimate of the possible existence of long-distance LD. The values in the first three plots indicate the correlation between and LD decay score in three different physical distance and the correlation between (left-side vertical axis) and LD decay score (right-side vertical axis) and the correlation between (left-side vertical axis) and (right-side vertical axis), respectively. The last plot assessed the impact of centromere region of chromosome 11 on the linear relationship between chromosomal LD and the inverse of the SNP number. The dark and light gray dashed lines represent the mean of the with and without the presence of centromere region of chromosome 11.
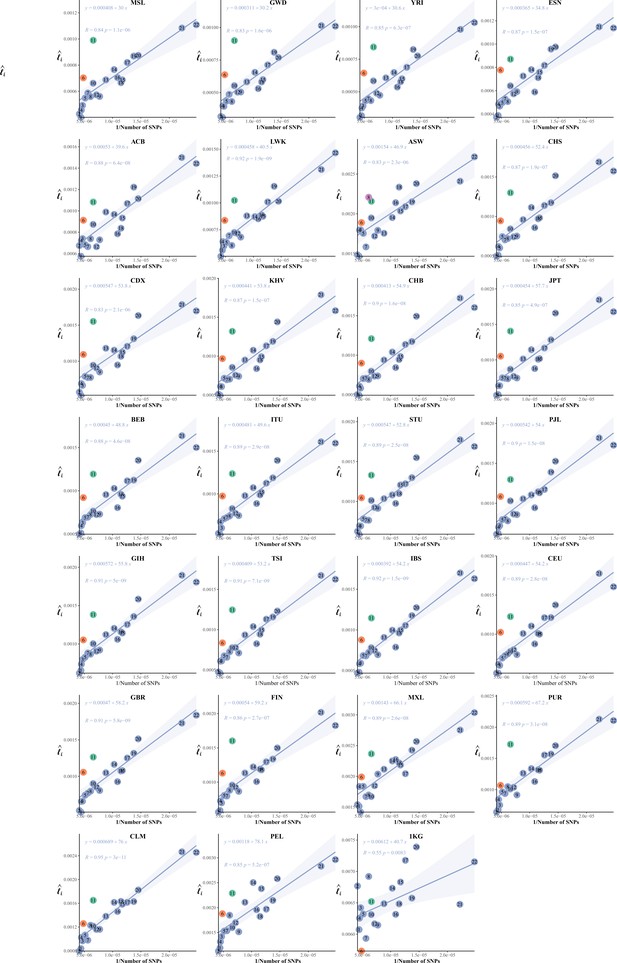
The correlation between the inverse of the single-nucleotide polymorphism (SNP) number and chromosomal linkage disequilibrium (LD) in 26 cohorts of 1KG.
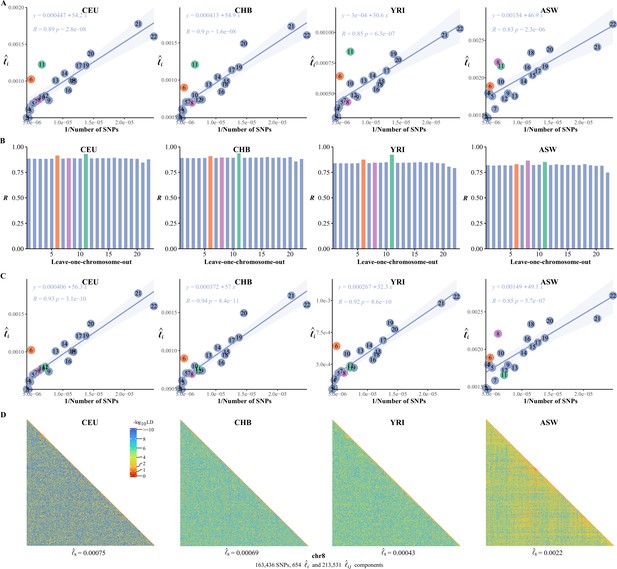
The correlation between the inversion of the single-nucleotide polymorphism (SNP) number and .
(A) The correlation between the inversion of the SNP number and in CEU, CHB, YRI, and ASW. (B) Leave-one-chromosome-out strategy is adopted to evaluate the contribution of a certain chromosome on the correlation between the inverse of the SNP number and . (C) The correlation between the inversion of the SNP number and chromosomal linkage disequilibrium (LD) in CEU, CHB, YRI, and ASW after removing the centromere region of chromosome 11. (D) High-resolution illustration for LD grids for chromosome 8 in CEU, CHB, YRI, and ASW. For each cohort, we partition chromosome 8 into consecutive LD grids (each LD grid contains 250 ×250 SNP pairs). For visualization purposes, LD is transformed to a -log10-scale, with smaller values (red hues) representing larger LD, and a value of 0 representing that all SNPs are in LD.

Influence of expanding of single-nucleotide polymorphism (SNP) numbers on the correlation between the inverse of the SNP number and chromosomal linkage disequilibrium (LD) in ASW.
Randomly selected SNPs that were presented in ASW but were not 2,997,635 consensus SNPs were added to the ASW cohort to demonstrate the stable pattern of chromosome 8.
Tables
Notation definitions.
Notation | Definition |
---|---|
The number of chromosomes. | |
and | Subscripts index chromosome and . |
The number of SNP segments of chromosome , each of which has SNPs. | |
The difference between the observed and expected haplotype frequencies, with . | |
The inbreeding coefficient. | |
Genetic relatedness matrix for chromosome , and two vectors, and , from , where stacks the off-diagonal elements and stacks the diagonal elements. | |
Subscript indexes individual. | |
and | Subscripts index a pair of SNPs. |
The number of SNPs; the number of SNPs on chromosome . | |
The number of samples; , the number of samples in subpopulation . | |
and | Frequency of the lth reference allele and alternative allele in the population. |
The relatedness score between individual and . | |
The genotype for the kth individual at the lth biallelic locus. | |
and | Genotype and standardized genotype matrixes for chromosome . |
Squared Pearson’s correlation coefficient for any pair of SNPs, including an SNP to itself when . | |
Squared Pearson’s correlation metric for LD but estimated from PLINK (--r2) or PopLDdecay. | |
The mean LD of the whole genome-wide SNP pairs. | |
The intra-chromosomal mean LD for the ith chromosome of SNP pairs. | |
The inter-chromosomal mean LD ith and jth chromosomal SNP pairs, a scaled version is . | |
The mean LD for a diagonal grid. | |
The mean LD for off-diagonal grids. |
-
LD, linkage disequilibrium; SNP, single-nucleotide polymorphism.
Computational time for the demonstrated estimation tasks.
Cohort | Task description | Time cost | Computational time complex |
---|---|---|---|
CHB (, ) | Estimation for 22 autosomal , and 231 inter-chromosomal . For results, see Figure 3 and Table 3. | 101,34 s | |
1KG (, ) | Same as above. | 3008.29 s | Same as above |
CONVERGE (, ) | Same as above. For results, see Figure 1A. | 77,508.00 s | Same as above |
Estimation for high-resolution LD interaction given bin size of 250 SNPs | |||
CHB (, ) | Chromosome 2, estimation for 965 , and 465,130 . For results, see Figure 4. | 66.86 s | |
CHB (, ) | Chromosome 22, estimation for 162 , and 13,041 . For results, see Figure 4. | 3.22 s | Same as above |
CONVERGE (, ) | Chromosome 22, estimation for 286 , and 40,755 . | 8,736.29 s | Same as above |
CONVERGE (, ) | Chromosome 2, estimation for 1000 , and 499,500 . For results, see Figure 1B. | 45,125.00 s | Chromosome 2 was split into 1000 blocks, each of which had about 420 SNPs |
-
For the sake of fair comparison, 10 CPUs were used for multi-thread computing.
-
LD, linkage disequilibrium; SNP, single-nucleotide polymorphism.
X-LD estimation for complex LD components (2,997,635 SNPs).
Cohort () | Ancestry | * | (SE)† | (SD) ‡ | (SD) ‡ | (SD) ‡ | Lower bound of LD § |
---|---|---|---|---|---|---|---|
MSL (85) | AFR | 1.10 (0.013) | 1.9e-4 (1.21e-6) | 6.9e-4 (2.0e-4) | 1.7e-4 (1.7e-5) | 0.26 (0.053) | 0.161971831 |
GWD (113) | AFR | 1.07 (0.009) | 1.1e-4 (5.61e-7) | 6.0e-4 (2.0e-4) | 8.7e-5 (8.1e-6) | 0.16 (0.037) | 0.247218789 |
YRI (107) | AFR | 1.05 (0.010) | 1.1e-4 (4.23e-7) | 5.9e-4 (2.0e-4) | 8.8e-5 (6.9e-6) | 0.16 (0.04) | 0.242001641 |
ESN (99) | AFR | 1.09 (0.011) | 1.4e-4 (7.67e-7) | 7.0e-4 (2.2e-4) | 1.2e-4 (1.2e-5) | 0.19 (0.043) | 0.217391304 |
ACB (96) | AFR | 2.01 (0.021) | 2.9e-4 (3.78e-6) | 9.1e-4 (2.5e-4) | 2.5e-4 (3.6e-5) | 0.29 (0.070) | 0.147727273 |
LWK (99) | AFR | 1.35 (0.014) | 2.2e-4 (2.38e-6) | 8.4e-4 (2.5e-4) | 1.9e-4 (3.2e-5) | 0.24 (0.052) | 0.173913043 |
ASW (61) | AFR | 1.90 (0.031) | 1.1e-3 (2.73e-5) | 2.0e-3 (3.2e-4) | 1.1e-3 (6.2e-5) | 0.57 (0.059) | 0.079681275 |
CHS (105) | EA | 1.08 (0.010) | 1.4e-4 (9.39e-7) | 9.5e-4 (3.4e-4) | 1.0e-4 (1.3e-5) | 0.12 (0.030) | 0.31147541 |
CDX (93) | EA | 1.11 (0.012) | 1.8e-4 (1.38e-6) | 1.1e-3 (3.6e-4) | 1.4e-4 (2.0e-5) | 0.14 (0.040) | 0.272277228 |
KHV (99) | EA | 1.07 (0.011) | 1.4e-4 (7.67e-7) | 9.5e-4 (3.5e-4) | 1.0e-4 (1.2e-5) | 0.12 (0.031) | 0.31147541 |
CHB (103) | EA | 1.07 (0.010) | 1.3e-4 (6.94e-7) | 9.3e-4 (3.4e-4) | 9.5e-5 (1.1e-5) | 0.11 (0.030) | 0.317948718 |
JPT (104) | EA | 1.06 (0.010) | 1.3e-4 (7.22e-7) | 1.0e-3 (3.8e-4) | 9.3e-5 (1.2e-5) | 0.10 (0.028) | 0.338638673 |
BEB (86) | SA | 1.07 (0.012) | 1.7e-4 (8.09e-7) | 9.1e-4 (3.1e-4) | 1.4e-4 (1.5e-5) | 0.17 (0.042) | 0.236363636 |
ITU (102) | SA | 1.61 (0.016) | 1.9e-4 (1.84e-6) | 9.5e-4 (3.1e-4) | 1.5e-4 (1.7e-5) | 0.18 (0.044) | 0.231707317 |
STU (102) | SA | 1.56 (0.015) | 2.6e-4 (3.21e-6) | 1.0e-3 (3.3e-4) | 2.3e-4 (3.1e-5) | 0.23 (0.047) | 0.171526587 |
PJL (96) | SA | 1.67 (0.017) | 2.4e-4 (2.74e-6) | 1.1e-3 (3.4e-4) | 2.0e-4 (2.2e-5) | 0.21 (0.048) | 0.20754717 |
GIH (103) | SA | 1.73 (0.017) | 2.7e-4 (3.41e-6) | 1.1e-3 (3.4e-4) | 2.4e-4 (1.9e-5) | 0.23 (0.049) | 0.179153094 |
TSI (107) | EUR | 1.07 (0.010) | 1.2e-4 (6.10e-7) | 9.1e-4 (3.3e-4) | 9.0e-5 (1.1e-5) | 0.11 (0.029) | 0.325 |
IBS (107) | EUR | 1.07 (0.010) | 1.2e-4 (6.10e-7) | 9.1e-4 (3.3e-4) | 8.8e-5 (1.1e-5) | 0.11 (0.028) | 0.329949239 |
CEU (99) | EUR | 1.07 (0.011) | 1.4e-4 (7.67e-7) | 9.6e-4 (3.4e-4) | 1.1e-4 (1.3e-5) | 0.12 (0.030) | 0.293577982 |
GBR (91) | EUR | 1.11 (0.012) | 1.7e-4 (1.08e-6) | 1.0e-3 (3.6e-4) | 1.4e-4 (1.8e-5) | 0.15 (0.036) | 0.253807107 |
FIN (99) | EUR | 1.09 (0.011) | 1.5e-4 (9.69e-7) | 1.1e-3 (3.8e-4) | 1.0e-4 (1.5e-5) | 0.10 (0.027) | 0.34375 |
MXL (64) | AMR | 2.29 (0.036) | 7.2e-4 (1.49e-5) | 2.1e-3 (4.1e-4) | 6.3e-4 (9.6e-5) | 0.32 (0.072) | 0.136986301 |
PUR (104) | AMR | 1.43 (0.014) | 1.6e-4 (1.30e-6) | 1.2e-3 (4.2e-4) | 1.2e-4 (1.7e-5) | 0.11 (0.026) | 0.322580645 |
CLM (94) | AMR | 1.58 (0.017) | 2.3e-4 (2.49e-6) | 1.4e-3 (4.5e-4) | 1.7e-4 (2.6e-5) | 0.13 (0.035) | 0.281690141 |
PEL (85) | AMR | 2.38 (0.028) | 4.5e-4 (7.33e-6) | 1.9e-3 (5.1e-4) | 3.7e-4 (8.5e-5) | 0.21 (0.062) | 0.196483971 |
1KG (2503) | MIX | 164.20 (0.066) | 5.8e-3 (4.63e-6) | 6.5e-3 (4.1e-4) | 5.7e-3 (2.4e-4) | 0.88 (0.028) | 0.051505547 |
-
LD, linkage disequilibrium; SNPs, single-nucleotide polymorphisms.
-
*
Eigenvalue was estimated. In parentheses is the ratio between the listed largest eigenvalue and the sample size. Since there exists an approximation that , the ratio can be taken as an approximation of population structure.
-
†
Standard error was calculated as , as Equation 7.
-
‡
Estimated empirically from chromosomal ; Estimated empirically from inter-chromosomal .
-
§
It is estimated by , indicating lower bound of true LD.
Estimates for 22 autosomal in CEU, CHB, YRI, and ASW, respectively.
Chromosome | SNP number | |||||
---|---|---|---|---|---|---|
CEU | CHB | YRI | ASW | |||
1 | 225,967 | 5.0e-4 (8.2e-6) | 0.00049 (7.8e-6) | 0.00032 (4.3e-6) | 0.0015 (4e-05) | |
2 | 241,241 | 5.0e-4 (8.1e-6) | 5.0e-4 (7.9e-6) | 3.0e-4 (4.1e-6) | 0.0015 (4e-05) | |
3 | 212,670 | 6.0e-04 (1.0e-5) | 0.00058 (9.5e-6) | 0.00039 (5.7e-6) | 0.0018 (5.1e-5) | |
4 | 222,241 | 0.00062 (1.0e-5) | 0.00061 (1.0e-5) | 0.00038 (5.4e-6) | 0.0018 (5.0e-5) | |
5 | 193,632 | 0.00069 (1.2e-5) | 7.0e-04 (1.2e-5) | 0.00043 (6.5e-6) | 0.0018 (4.9e-5) | |
6 | 206,165 | 0.0010 (1.9e-5) | 9.0e-04 (1.6e-5) | 0.00064 (1.0e-5) | 0.0019 (5.4e-5) | |
7 | 177,414 | 0.00073 (1.3e-5) | 0.00071 (1.2e-5) | 0.00045 (6.8e-6) | 0.0016 (4.3e-5) | |
8 | 163,436 | 0.00075 (1.3e-5) | 0.00069 (1.2e-5) | 0.00043 (6.5e-6) | 0.0022 (6.4e-5) | |
9 | 129,440 | 0.00074 (1.3e-5) | 0.00074 (1.3e-5) | 0.00047 (7.2e-6) | 0.0018 (5.0e-5) | |
10 | 152,251 | 0.00078 (1.4e-5) | 8.0e-04 (1.4e-5) | 0.00058 (9.3e-6) | 0.0019 (5.6e-5) | |
11 | 151,751 | 0.0012 (2.3e-5) | 0.0012 (2.2e-5) | 0.00084 (1.4e-5) | 0.0022 (6.2e-5) | |
12 | 139,684 | 8.0e-4 (1.4e-5) | 0.00073 (1.2e-5) | 0.00049 (7.5e-6) | 0.0017 (4.8e-5) | |
13 | 113,390 | 0.0010 (1.8e-5) | 0.00094 (1.6e-5) | 0.00061 (9.8e-6) | 0.0018 (4.9e-5) | |
14 | 97,335 | 0.0011 (2.0e-5) | 0.0010 (1.8e-5) | 0.00065 (1.1e-5) | 0.0020 (5.6e-5) | |
15 | 85,307 | 0.0010 (1.8e-5) | 0.00098 (1.7e-5) | 6.0e-4 (9.6e-6) | 0.0020 (5.8e-5) | |
16 | 92,007 | 0.00088 (1.6e-5) | 0.00084 (1.5e-5) | 0.00054 (8.4e-6) | 0.0021 (6.2e-5) | |
17 | 79,478 | 0.0012 (2.3e-5) | 0.0011 (2.0e-5) | 0.00069 (1.1e-5) | 0.0021 (6.0e-5) | |
18 | 87,105 | 0.0010 (1.8e-5) | 0.00095 (1.7e-5) | 0.00058 (9.2e-6) | 0.0023 (6.8e-5) | |
19 | 72,794 | 0.0012 (2.3e-05) | 0.0012 (2.1e-5) | 0.00082 (1.4e-5) | 0.0022 (6.2e-5) | |
20 | 68,881 | 0.0014 (2.6e-5) | 0.0015 (2.7e-5) | 0.00078 (1.3e-5) | 0.0024 (7.0e-5) | |
21 | 45,068 | 0.0018 (3.4e-5) | 0.0017 (3.2e-5) | 0.00098 (1.7e-5) | 0.0024 (7.1e-5) | |
22 | 40,378 | 0.0016 (3.1e-5) | 0.0016 (2.9e-5) | 0.0010 (1.8e-5) | 0.0027 (8.1e-5) |
-
Each and its standard error are in parentheses, as estimated in Equation 7.
-
SNP, single-nucleotide polymorphism.
LD decay regression analysis for 26 cohorts.
Cohort () | LD-decay regression* | Population parameters† | |||||
---|---|---|---|---|---|---|---|
LD decay score | Ancestry | True LD ‡ | |||||
MSL (85) | 0.00041 | 29.97 | 0.84 | 0.0421 | 0.013 | AFR | 0.62727273 |
GWD (113) | 0.00031 | 30.17 | 0.83 | 0.0439 | 0.009 | AFR | 0.65934066 |
YRI (107) | 0.00030 | 30.64 | 0.85 | 0.0436 | 0.010 | AFR | 0.66292135 |
ESN (99) | 0.00037 | 34.82 | 0.87 | 0.0436 | 0.011 | AFR | 0.65420561 |
ACB (96) | 0.00053 | 39.62 | 0.88 | 0.0451 | 0.021 | AFR | 0.63194444 |
LWK (99) | 0.00046 | 40.52 | 0.92 | 0.0447 | 0.014 | AFR | 0.64615385 |
ASW (61) | 0.0015 | 46.88 | 0.83 | 0.0472 | 0.031 | AFR | 0.57142857 |
CHS (105) | 0.00046 | 52.36 | 0.87 | 0.0555 | 0.010 | EA | 0.67375887 |
CDX (93) | 0.00055 | 53.77 | 0.83 | 0.0557 | 0.012 | EA | 0.66666667 |
KHV (99) | 0.00044 | 53.79 | 0.87 | 0.0560 | 0.011 | EA | 0.68345324 |
CHB (103) | 0.00041 | 54.90 | 0.90 | 0.0558 | 0.010 | EA | 0.69402985 |
JPT (104) | 0.00045 | 57.75 | 0.85 | 0.0568 | 0.010 | EA | 0.68965517 |
BEB (86) | 0.00045 | 48.84 | 0.88 | 0.0556 | 0.012 | SA | 0.66911765 |
ITU (102) | 0.00048 | 49.58 | 0.89 | 0.0546 | 0.016 | SA | 0.66433566 |
STU (102) | 0.00055 | 52.84 | 0.89 | 0.0546 | 0.015 | SA | 0.64516129 |
PJL (96) | 0.00054 | 54.00 | 0.90 | 0.0546 | 0.017 | SA | 0.67073171 |
GIH (103) | 0.00057 | 55.81 | 0.91 | 0.0562 | 0.017 | SA | 0.65868263 |
TSI (107) | 0.00041 | 53.17 | 0.91 | 0.0558 | 0.010 | EUR | 0.68939394 |
IBS (107) | 0.00039 | 54.22 | 0.92 | 0.0555 | 0.010 | EUR | 0.7 |
CEU (99) | 0.00045 | 54.23 | 0.89 | 0.0559 | 0.011 | EUR | 0.68085106 |
GBR (91) | 0.00047 | 58.23 | 0.91 | 0.0555 | 0.012 | EUR | 0.68027211 |
FIN (99) | 0.00054 | 59.24 | 0.86 | 0.0579 | 0.011 | EUR | 0.67073171 |
MXL (64) | 0.0014 | 66.13 | 0.89 | 0.0558 | 0.036 | AMR | 0.6 |
PUR (104) | 0.00059 | 67.20 | 0.89 | 0.0571 | 0.014 | AMR | 0.67039106 |
CLM (94) | 0.00069 | 75.97 | 0.95 | 0.0572 | 0.017 | AMR | 0.66985646 |
PEL (85) | 0.0012 | 78.15 | 0.85 | 0.0598 | 0.028 | AMR | 0.61290323 |
1KG (2503) | 0.0061 | 40.65 | 0.55 | 0.066 | Mixed | 0.51587302 |
-
LD, linkage disequilibrium; SNP, single-nucleotide polymorphism.
-
*
The regression intercept and the coefficients are as represented in Equation 3.
-
†
The column for LD decay score was taken as the mean of the estimated from PopLDdecay in a physical distance of 1500 kb, which was approximated to the area under the curve in Figure 5A for each cohort; was approximated by , in which the largest eigenvalue for the cohort. was the estimated LD statistic from PLINK (--r2).
-
‡
True LD is defined as .
Additional files
-
MDAR checklist
- https://cdn.elifesciences.org/articles/90636/elife-90636-mdarchecklist1-v2.docx
-
Supplementary file 1
Extended data for 1KG LD estimation.
- https://cdn.elifesciences.org/articles/90636/elife-90636-supp1-v2.xlsx