Reliance on self-reports and estimated food composition data in nutrition research introduces significant bias that can only be addressed with biomarkers
Figures
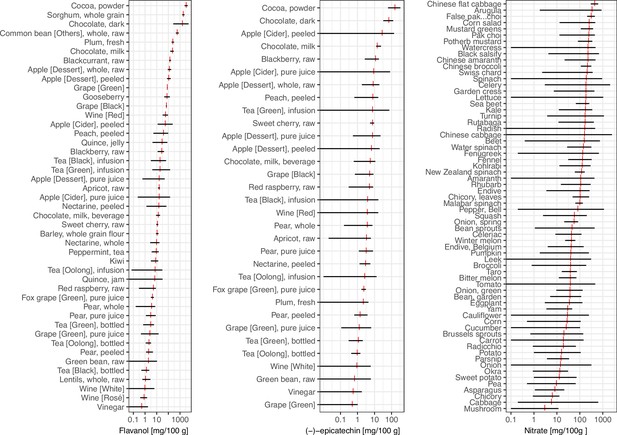
Variability in flavan-3-ol, (–)-epicatechin (Rothwell et al., 2013), and nitrate (Blekkenhorst et al., 2017) content of foods commonly eaten.
Data show the range of food content (black) and mean (red).
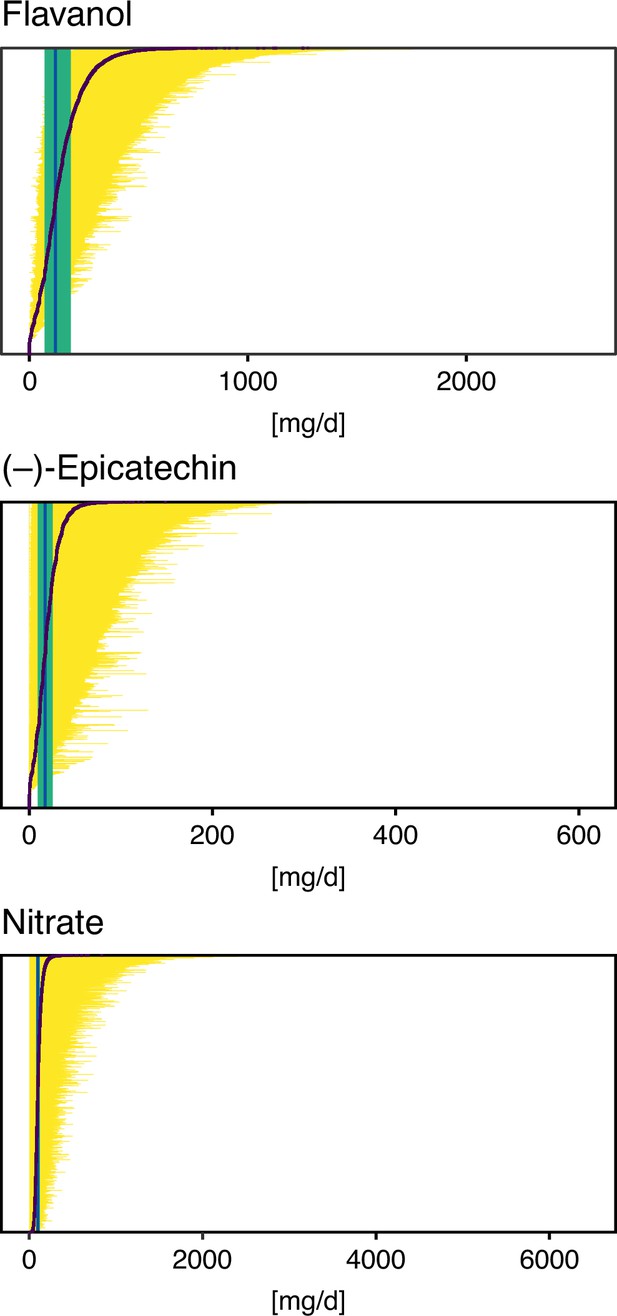
Possible intake ranges of flavan-3-ols, (–)-epicatechin, and nitrate in each individual study participant displayed from low to high possible bioactive intake level.
Range of bioactive intake was calculated using an approach similar to probabilistic modelling by sampling randomly from the distribution of possible food composition (n = 10,000 iterations). Intake based on mean bioactive content, as is common practice, is indicated by a black line. Green line shows the median intake of the entire cohort and the green box the interquartile range.
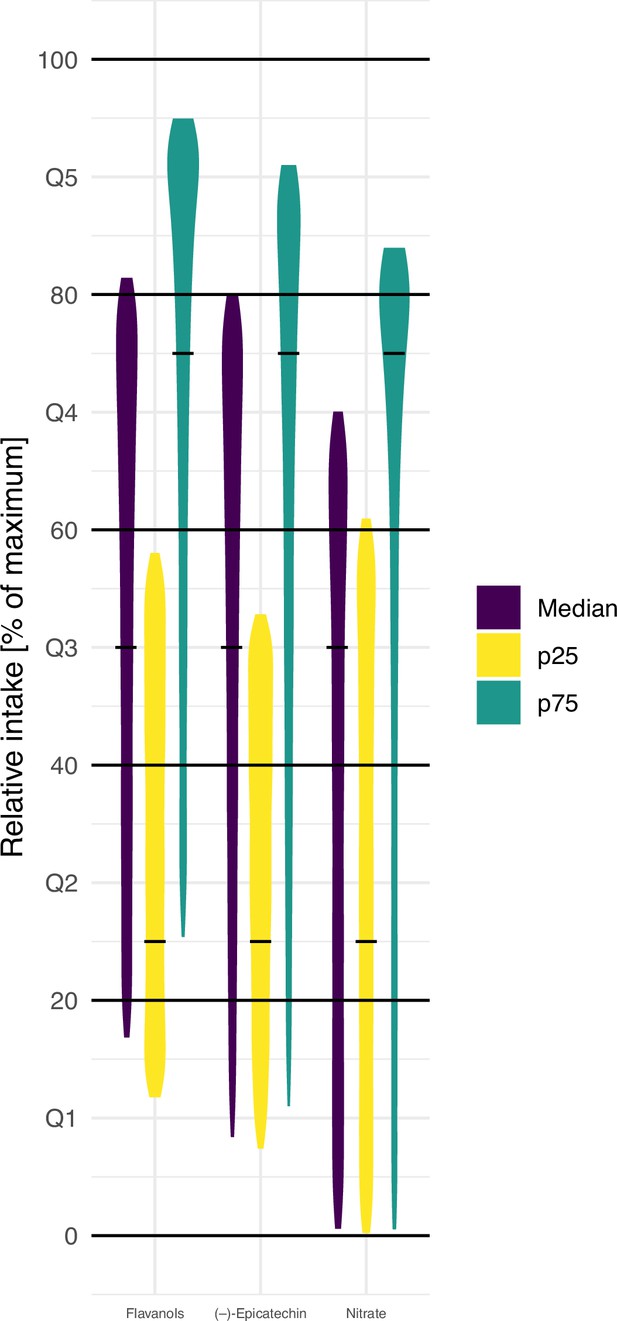
Simulation of the effect of variability in food composition on relative intakes of flavanols, (–)-epicatechin, and nitrate of EPIC Norfolk participants with low (25th centile, p25), medium (median), and high (75th centile, p75) estimated intake of bioactive (based on 24 hr diet recall [24HDR] and mean food content – indicated by the black line).
Data shown are relative intake (100% is the maximum intake) of 10,000 simulations.
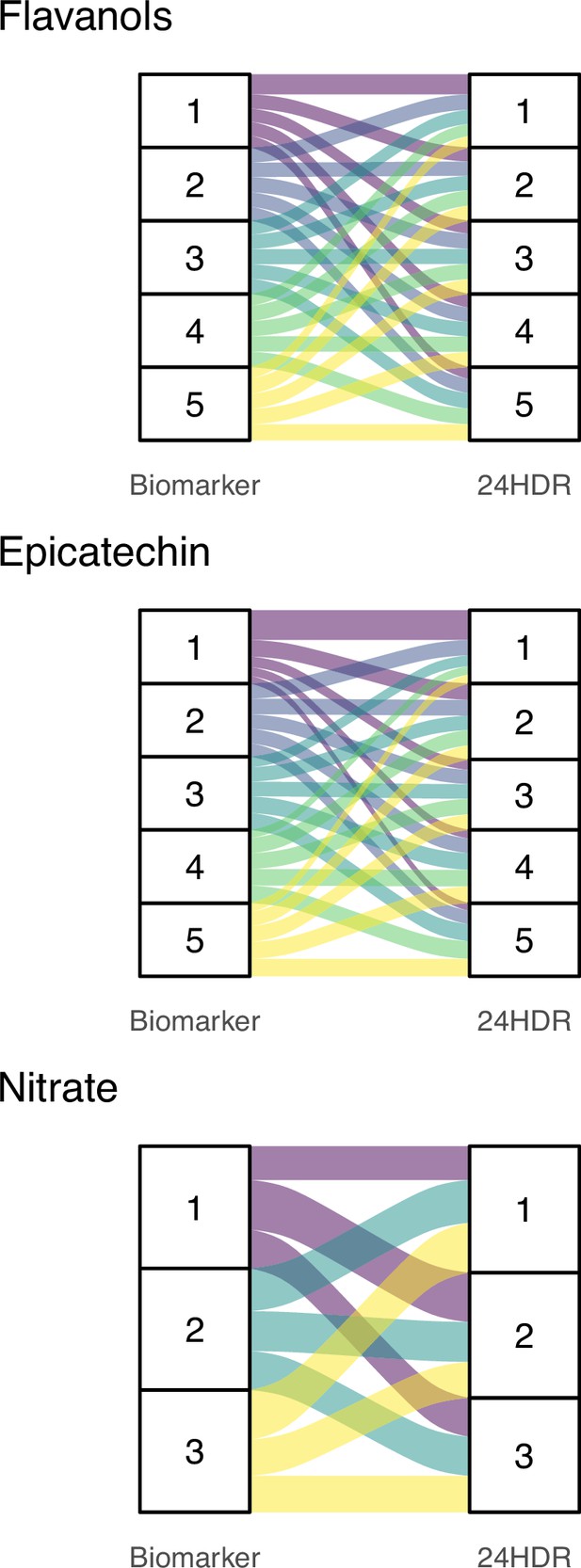
Alluvial plots comparing quantiles of bioactive intake estimated with biomarkers (left) and 24 hr dietary recall with food-composition data (24HDR – DD-FCT method, right).
Bands of the same colour show participants in the same biomarker-estimated quantile of intake and their respective quantile of intake based on the DD-FCT method. 24HDR estimated quantiles of intake were determined using the common approach of using mean content of flavan-3-ols, (–)-epicatechin, and nitrate in each food item as reported in databases.
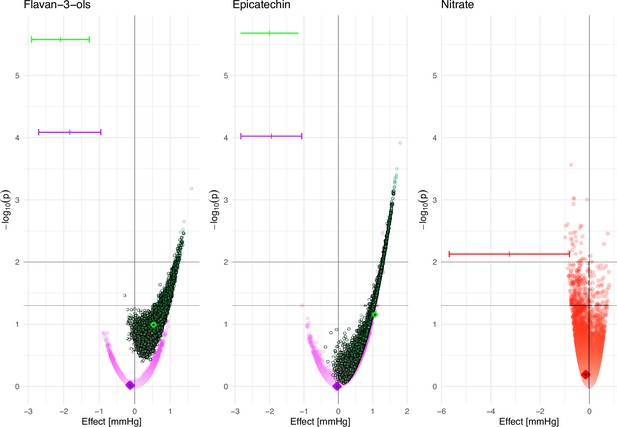
Association between estimated bioactive intake (flavan-3-ols, (–)-epicatechin, and nitrate, based on the 24 hr dietary recall and food composition data [DD-FCT method]) and systolic blood pressure at baseline (estimated difference between low [p10] and high [p90] intake and p-value for Wald-test (as -log10(p)) in men [purple], women [green], and all participants [red]).
Data are based on 10,000 simulations and adjusted for age, body mass index (BMI), plasma vitamin C, smoking status, physical activity, and self-reported health at baseline; additionally for menopausal status for women and sex for nitrate. Results based on the intake estimated by simulating food content within minimum and maximum food content reported in databases (circle), intake based on mean food content as reported in databases (diamond), and intake based on biomarker data (|). 95% CI is shown for biomarker only.
Tables
Characteristics of dietary bioactives used as model system of dietary compounds to investigate the limitation of using single-point estimates to assess intake and investigate health outcomes in nutrition research.
Dietary compound | Dietary distribution | Factors for variability | Biomarker of intake | Potential health effect |
---|---|---|---|---|
Flavan-3-ols | Tea, apple, and cocoa-derived products | Cultivar, agricultural conditions, storage, and processing | Urinary concentrations of gut microbiome-derived flavan-3-ol metabolites (phenyl-γ-valerolactone metabolites) (Ottaviani et al., 2018a) | Reduce cardiovascular events and deaths (Sesso et al., 2022. Reduce blood pressure Ottaviani et al., 2018b) Improve cognitive performance (Sloan et al., 2021) |
(–)-Epicatechin | Tea, apple, and cocoa-derived products | Cultivar, agricultural conditions, storage, and processing (including epimerisation) | Urinary concentrations of structural-related metabolites derived from phase II conjugation (Ottaviani et al., 2019) | Improve vascular function (Schroeter et al., 2006; Dicks et al., 2022) and reduce blood pressure (Ottaviani et al., 2018b) |
Nitrate | Vegetables, drinking water | Depends on a wide range of environmental factors such as fertilisation, light exposure, and water supply | Urinary nitrate status can be used as a surrogate marker of intake (Green et al., 1981; Pannala et al., 2003; Smallwood et al., 2017) | Dietary nitrate can reduce blood pressure (Larsen et al., 2006) |
Study population and baseline characteristics of 18,684 participants of EPIC Norfolk, for whom all data were available.
Data shown are mean (SD) or absolute number and proportion. Data for urinary nitrate was available for 1027 samples.
Women | Men | |
---|---|---|
n | 10,167 | 8517 |
Age (years) | 59 (9) | 59 (9) |
Body mass index (kg/m2) | 26.1 (4.2) | 26.4 (3) |
Systolic blood pressure (mmHg) | 134 (19) | 138 (17.6) |
Physical activity | ||
Inactive | 2997 (30%) | 2577 (30%) |
Moderately inactive | 3258 (32%) | 2096 (25%) |
Moderately active | 2309 (23%) | 1990 (23%) |
Active | 1603 (16%) | 1854 (22%) |
Smoking status | ||
Current | 1121 (11%) | 998 (12%) |
Former | 3250 (32%) | 4647 (55%) |
Never | 5796 (57%) | 2872 (34%) |
Intake of different bioactive compounds in EPIC Norfolk (median and interquartile range) when determined using different food composition data.
Results are shown for estimates calculated using minimum, mean, and maximum food content and self-reported dietary data based on 24 hr diet recall (24HDR).
Bioactive intake (mg/day) | |||
---|---|---|---|
Minimum food content | Mean food content | Maximum food content | |
Flavan-3-ols | 48 (28–82) | 120 (70–190) | 329 (172–451) |
(–)-Epicatechin | 1.5 (1.0–2.5) | 19 (9–25) | 33 (65–100) |
Nitrate | 5.5 (4.6–57) | 100 (80–124) | 204 (151–305) |