ImmCellTyper facilitates systematic mass cytometry data analysis for deep immune profiling
Figures
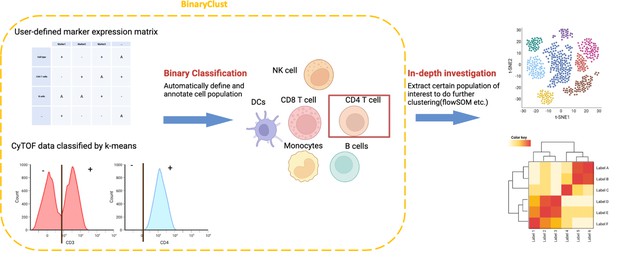
Schematic diagram of BinaryClust framework.
Semi-supervised classification is first performed on selected markers in the user-defined marker expression matrix to classify and annotate major cell types. Population-of-interest can be further extracted and explored using unsupervised clustering methods followed by differential analysis. Created with BioRender.com.
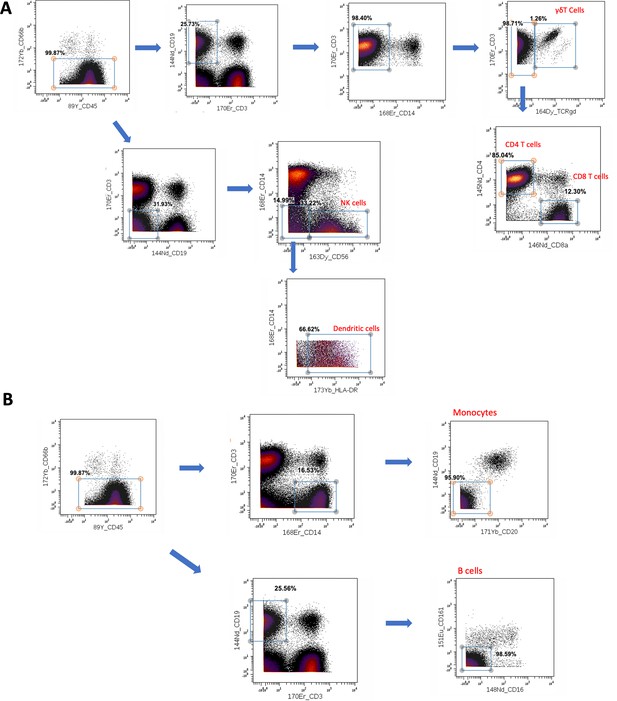
Manual hierarchical gating strategy for main cell linages from human peripheral blood mononuclear cell (PBMC) samples (myeloproliferative neoplasm [MPN] dataset, n = 9).
All FCS files were cleaned up to remove doublets, normalisation beads and debris (please refer to the methods for standard clean-up procedure), and pre-gated for CD45+ leukocytes. (A) Serial bi-axial scatter plots representing the gating diagram for T cell subsets (CD4 T cells, CD8 T cells, and gamma delta T cells), NK cells and dendritic cells based on the indicated phenotypic markers. (B) Serial bi-axial scatter plot indicating the gating strategy to isolate monocytes and B cells from leukocytes. All manual gating was done using Cytobank platform (https://premium.cytobank.org/cytobank/).

Clean-up procedure of cytometry by time-of-flight (CyTOF) data using Cytobank.
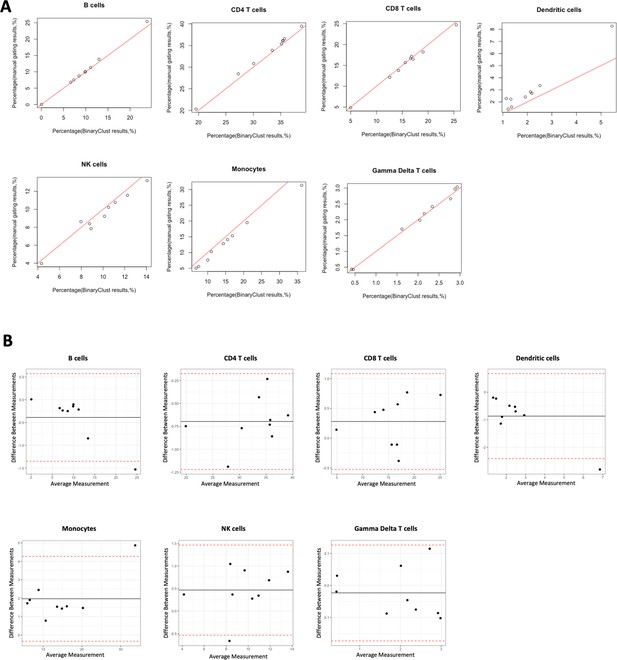
Agreement evaluation comparing manual gating and BinaryClust in myeloproliferative neoplasm (MPN) cohort (n = 9).
Manual gating of B cells, CD4 T cells, CD8 T cells, dendritic cells, NK cells, monocytes, and gamma delta T cells were performed by two independent experts using Cytobank, and mean values of the population percentages were calculated to compare with BinaryClust results. Each dot represents one patient sample. (A) Scatter plot showing the correlation between the two methods, with the red line indicating perfect agreement (correlation coefficient = 1). (B) Bland–Altman plots of the two measurement methods among all the cell populations, with the black line suggesting the mean observed difference and red dotted lines indicating limits of agreement (1.96× standard deviations).
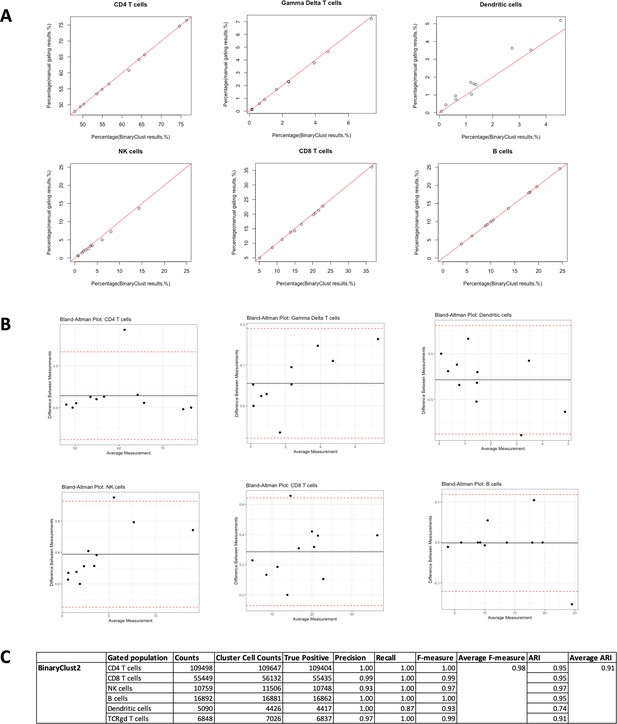
Agreement evaluation between ImmCellTyper and manual gating in influenza dataset (n = 11).
Manual gating was performed using Cytobank and exported manually. (A) Correlation plots between ImmCellTyper results and manual gating results concerning percentages in CD4 T cells, gamma delta T cells, dendritic cells, NK cells, CD8 T cells, and B cells, with red line indicating perfect agreement (correlation coefficient = 1). (B) Bland–Altman plots of the two measurements in the indicated populations, with black line suggesting mean difference between measurements and dotted red line indicating limits of agreement (1.96× standard deviations). (C) Calculation of precision, recall, F-measure for ImmCellTyper method in comparison to manual gating in the indicated cell populations.
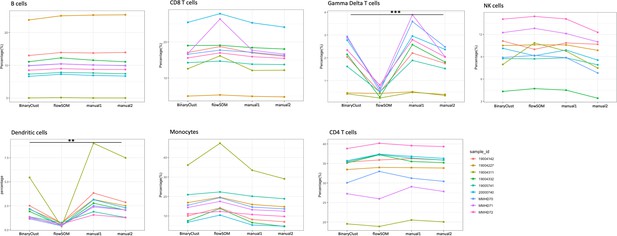
Comparison of manual gating (manual1 and manual2), BinaryClust, and flowSOM clustering results in myeloproliferative neoplasm (MPN) cohort (n = 9).
Interaction plots showing the individual measurement (percentage) of each study participant with indicated colours by different methods across main cell lineages (B cells, CD8 T cells, gamma delta T cells, NK cells, dendritic cells, monocytes, and CD4 T cells); analysis of variance (ANOVA) was used for statistical testing, and significance was marked by asterisk. *p < 0.05, **p < 0.01, ***p < 0.001, ****p < 0.0001.
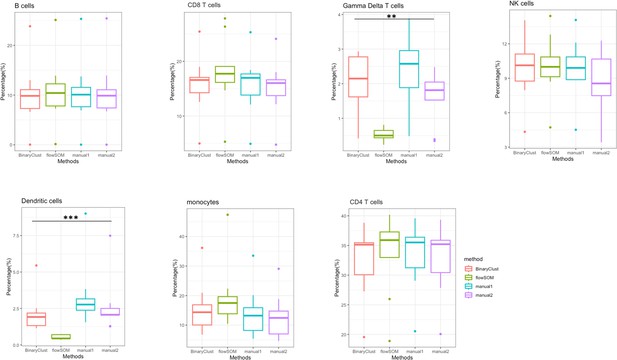
Boxplots of the indicated cell percentages generated by different methods.
Statistical significance was marked by asterisk. *p < 0.05, **p < 0.01, ***p < 0.001.
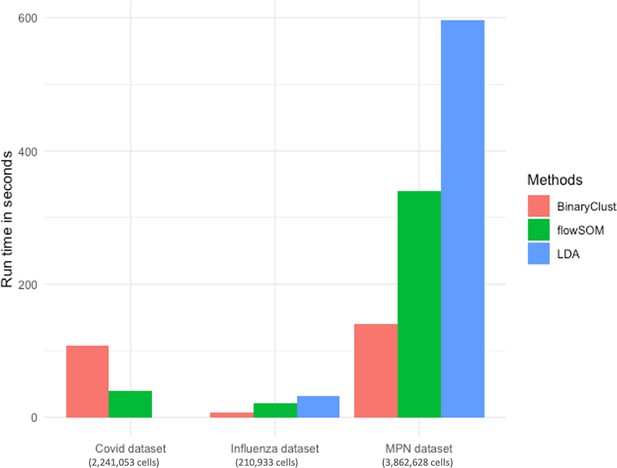
Comparison of BinaryClust, flowSOM, and linear discriminant analysis (LDA) on speed.
Bar chart showing runtime (in seconds) of the three methods in three different datasets.
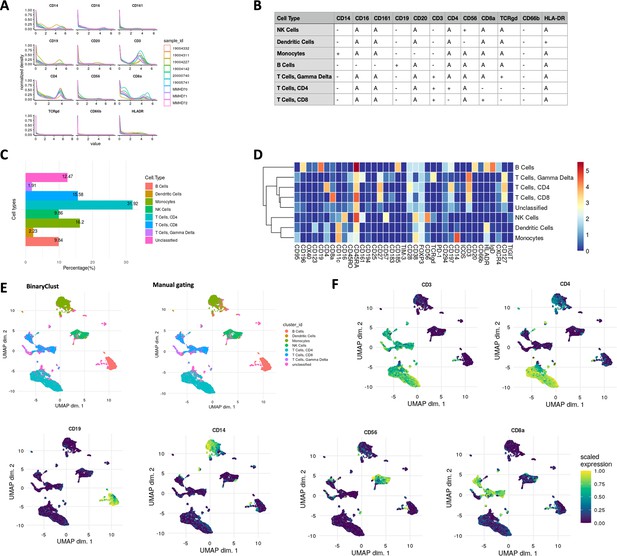
Cell type characterisation and visualisation using ImmCellTyper pipeline in myeloproliferative neoplasm (MPN) dataset (n = 9).
(A) Intensity distribution of selected phenotypic markers used for BinaryClust classification, coloured by sample_id. (B) Pre-defined expression classification matrix for the MPN dataset, ‘+’ indicates positive, ‘-’ indicates negative, and ‘A’ suggests ‘any’. (C) Proportion of the main cell lineages of all cells in the concatenated FCS files after classification. (D) Median marker expression heatmap of BinaryClust classification results. (E) UMAP plot of random downsample of 2000 cells per patient coloured by main cell types based on BinaryClust classification (left) and manual gating results (right). (F) UMAP plots coloured by normalised expression of indicated markers (CD3, CD4, CD8a, CD20, CD19, CD14, and CD56) across 2000 cells per sample.
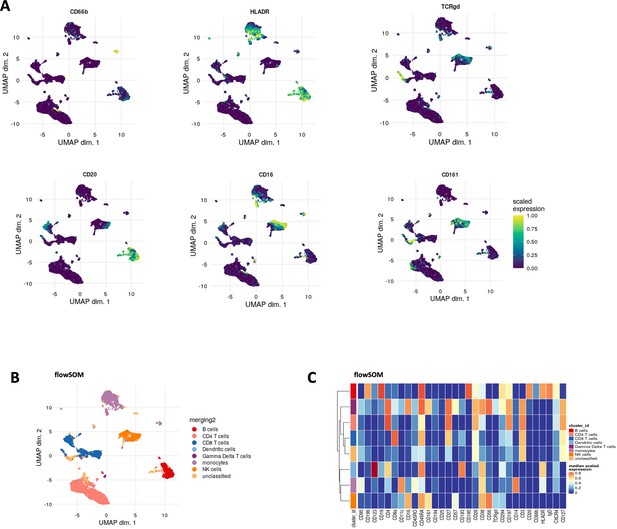
Comparison of different clustering methods in MPN cohort.
(A) UMAP plots of normalised expression of indicated markers (CD66b, HLADR, TCRgd, CD20, CD16, and CD161) across 2000 cells per sample in myeloproliferative neoplasm (MPN) dataset. (B) FlowSOM clustering was performed on the same dataset to compare with BinarClust, k = 20 was chosen followed by manual annotation of each cluster. UMAP plot was projected with merged flowSOM clusters with biological annotation (downsample 2000 cells per sample). (C) The corresponding median marker expression heatmap after flowSOM clustering and annotation.
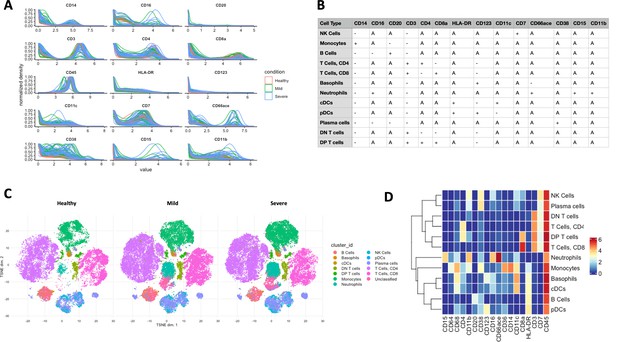
Applying ImmCellTyper pipeline on COVID-19 patient dataset (n = 82) published by Chevrier et al., 2021.
(A) Marker intensity distribution of selected phenotypic markers used for BinaryClust classification, coloured by disease severity (n = 22 healthy individuals, 28 mild COVID-19 patients, and 38 severe COVID-19 patients). (B) Pre-defined marker expression classification matrix used for BinaryClust. (C) t-Distributed stochastic neighbour embedding (t-SNE) plots, with 1000 cells per sample, were coloured by the main cell types generated by BinaryClust and faceted by different study groups. (D) The corresponding median marker expression heatmap of BinaryClust results for the COVID-19 dataset.
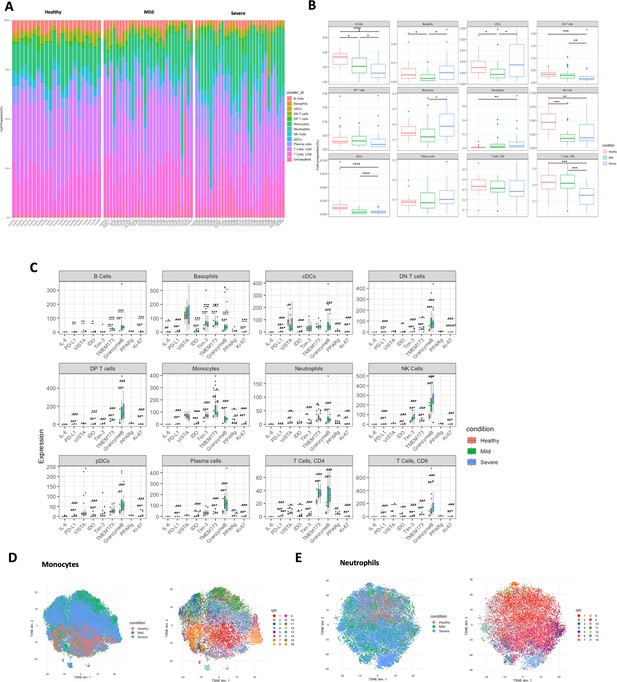
Quantification and statistical analysis comparing the study conditions in COVID-19 dataset (n = 82).
(A) Stacked histogram of main cell type composition per individual generated by BinaryClust, and grouped by study conditions (healthy, mild, and severe). (B) Boxplots representing cell abundance frequencies among the study conditions, faceted by different main cell types. (C) State marker expression intensities with comparison of the study groups across the main cell types. (D) Clusters of monocytes and neutrophils were extracted from the whole cells for downstream interrogation. t-Distributed stochastic neighbour embedding (t-SNE) plots with random downsample of 1000 monocyte cells and (E) neutrophils per sample were coloured by study conditions and Phenograph clustering results (k = 60), respectively. Statistical significance was marked by asterisk. *p < 0.05, **p < 0.01, ***p < 0.001, ****p < 0.0001.
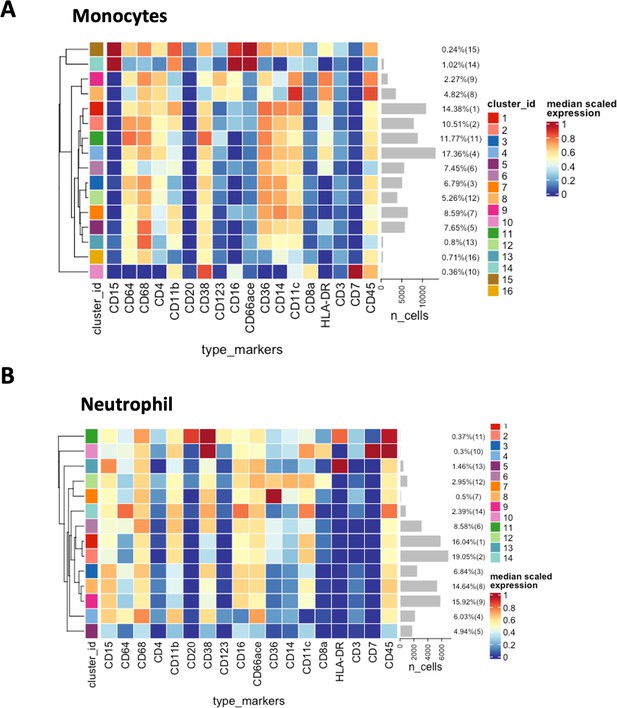
Expression heatmaps of monocytes and neotrophils in COVID-19 dataset.
Marker expression heatmap of (A) monocytes and (B) neutrophils generated by Phenograph clustering.
Tables
Precision, recall, F-measure, and ARI of indicated clustering methods.
Gated population | Counts | Cluster cell counts | True positive | Precision | Recall | F-measure | Average F-measure | ARI | Average ARI | |
---|---|---|---|---|---|---|---|---|---|---|
BinaryClust2 | CD4 T cells | 1,270,041 | 1,232,832 | 1,226,712 | 1.00 | 0.97 | 0.98 | 0.93 | 0.95 | 0.91 |
CD8 T cells | 601,995 | 601,639 | 582,402 | 0.97 | 0.97 | 0.97 | 0.95 | |||
NK cells | 389,261 | 380,999 | 376,561 | 0.99 | 0.97 | 0.98 | 0.97 | |||
Monocytes | 576,624 | 625,735 | 568,924 | 0.91 | 0.99 | 0.95 | 0.92 | |||
B cells | 397,279 | 380,170 | 374,501 | 0.99 | 0.94 | 0.96 | 0.95 | |||
Dendritic cells | 135,894 | 86,012 | 84,130 | 0.98 | 0.62 | 0.76 | 0.74 | |||
TCRgd T cells | 87,194 | 73,599 | 73,562 | 1.00 | 0.84 | 0.91 | 0.91 | |||
flowSOM | CD4 T cells | 1,270,041 | 1,268,929 | 1,226,712 | 0.97 | 0.97 | 0.97 | 0.70 | 0.90 | 0.66 |
(k = 20) | CD8 T cells | 601,995 | 692,374 | 571,344 | 0.83 | 0.95 | 0.88 | 0.81 | ||
NK cells | 389,261 | 393,646 | 365,403 | 0.93 | 0.94 | 0.93 | 0.91 | |||
Monocytes | 576,624 | 792,447 | 568,284 | 0.72 | 0.99 | 0.83 | 0.73 | |||
B cells | 397,279 | 405,886 | 390,077 | 0.96 | 0.98 | 0.97 | 0.96 | |||
Dendritic cells | 135,894 | 20,832 | 18,254 | 0.88 | 0.13 | 0.23 | 0.22 | |||
TCRgd T cells | 87,194 | 19,720 | 4967 | 0.25 | 0.06 | 0.09 | 0.08 | |||
LDA | CD4 T cells | 634,733 | 665,481 | 632,700 | 0.95 | 1.00 | 0.97 | 0.93 | 0.93 | 0.90 |
CD8 T cells | 301,138 | 327,469 | 299,309 | 0.91 | 0.99 | 0.95 | 0.92 | |||
NK cells | 194,470 | 202,110 | 187,259 | 0.93 | 0.96 | 0.94 | 0.92 | |||
Monocytes | 288,338 | 301,387 | 282,836 | 0.94 | 0.98 | 0.96 | 0.94 | |||
B cells | 198,582 | 211,090 | 195,908 | 0.93 | 0.99 | 0.96 | 0.94 | |||
Dendritic cells | 68,152 | 90,456 | 61,785 | 0.68 | 0.91 | 0.78 | 0.75 | |||
TCRgd T cells | 43,405 | 43,064 | 39,976 | 0.93 | 0.92 | 0.92 | 0.92 |
-
ARI, Adjusted Rand Index; LDA, linear discriminant analysis.
Reagent type (species) or resource | Designation | Source or reference | Identifiers | Additional information |
---|---|---|---|---|
Antibody | Maxpar Direct Immune Profiling Assay | Standard Biotools | Cat# 201325 | |
Antibody | anti-CD95 (RRID:AB_314546, mouse, monoclonal) | Biolegend | Cat# 305607 | 1:200, 1.5 μl |
Antibody | anti-TIGIT (mouse, monoclonal) | Standard Biotools | Cat# 201406 (Maxpar Direct T cell expansion panel 2) | 1:300, 1 μl |
Antibody | anti-PD1 (mouse, monoclonal) | Standard Biotools | Cat# 201406 (Maxpar Direct T cell expansion panel 2) | 1:200, 1.5 μl |
Antibody | anti-ICOS (hamster, monoclonal) | Standard Biotools | Cat# 201406 (Maxpar Direct T cell expansion panel 2) | 1:200, 1.5 μl |
Antibody | anti-TIM3 (mouse, monoclonal) | Standard Biotools | Cat# 201406 (Maxpar Direct T cell expansion panel 2) | 1:300, 1 μl |
Antibody | anti-OX40 (mouse monoclonal) | Standard Biotools | Cat# 201406 (Maxpar Direct T cell expansion panel 2) | 1:300, 1 μl |
Antibody | anti-CXCR4 (mouse, monoclonal) | Standard Biotools | Cat# 201406 (Maxpar Direct T cell expansion panel 2) | 1:300, 1 μl |
Genetic reagent; Recombinant DNA reagent | Pierce 16% Formaldehyde (methanol-free) | Thermo Fisher | Cat# 28906 | |
Genetic reagent; Recombinant DNA reagent | Trypan blue solution (0.4%) | Gibco | Cat# 15250-061 | |
Genetic reagent; Recombinant DNA reagent | Human TruStain FcX | Biolegend | Cat# 422302 | |
Genetic reagent; Recombinant DNA reagent | EQ Four Element Calibration beads | Standard Biotools | Cat# 201078 |
CyTOF antibody panel for MPN cohort.
Antibody | Clone | Metal tag | Manufacturer |
---|---|---|---|
Anti-CD45 | HI30 | 89Y | Standard BioTools |
Live/dead Indicator | N/A | 103Rh | Standard BioTools |
Anti-CD95 | DX2 | APC | Biolegend |
Anti-APC | APC003 | 106Cd | Standard BioTools |
Anti-CD196 | G034E3 | 141Pr | Standard BioTools |
Anti-OX40 | ACT35 | 142Nd | Standard BioTools |
Anti-CD123 | 6H6 | 143Nd | Standard BioTools |
Anti-CD19 | HIB19 | 144Nd | Standard BioTools |
Anti-CD4 | RPA-T4 | 145Nd | Standard BioTools |
Anti-CD8a | RPA-T8 | 146Nd | Standard BioTools |
Anti-CD11c | Bu15 | 147Sm | Standard BioTools |
Anti-CD16 | 3G8 | 148Nd | Standard BioTools |
Anti-CD45RO | UCHL1 | 149Sm | Standard BioTools |
Anti-CD45RA | HI100 | 150Nd | Standard BioTools |
Anti-CD161 | HP-3G10 | 151Eu | Standard BioTools |
Anti-CD194 | L291H4 | 152Sm | Standard BioTools |
Anti-CD25 | BC96 | 153Eu | Standard BioTools |
Anti-CD27 | O323 | 154Sm | Standard BioTools |
Anti-CD57 | HCD57 | 155Gd | Standard BioTools |
Anti-CD183 | G025H7 | 156Gd | Standard BioTools |
Anti-CD185 | J252D4 | 158Gd | Standard BioTools |
Anti-TIM-3 | F38-2E2 | 159Tb | Standard BioTools |
Anti-CD28 | CD28.2 | 160Gd | Standard BioTools |
Anti-CD38 | HB-7 | 161Dy | Standard BioTools |
Anti-CD56 | NCAM16.2 | 163Dy | Standard BioTools |
Anti-TCRgd | B1 | 164Dy | Standard BioTools |
Anti-PD-1 | EH12.2H7 | 165Ho | Standard BioTools |
Anti-CD294 | BM16 | 166Er | Standard BioTools |
Anti-CD197 | G043H7 | 167Er | Standard BioTools |
Anti-CD14 | 63D3 | 168Er | Standard BioTools |
Anti-ICOS | C398.4A | 169Tm | Standard BioTools |
Anti-CD3 | UCHT1 | 170Er | Standard BioTools |
Anti-CD20 | 2H7 | 171Yb | Standard BioTools |
Anti-CD66b | G10F5 | 172Yb | Standard BioTools |
Anti-HLA-DR | LN3 | 173Yb | Standard BioTools |
Anti-IgD | IA6-2 | 174Yb | Standard BioTools |
Anti-CXCR4 | 12G5 | 175Lu | Standard BioTools |
Anti-CD127 | A019D5 | 176Yb | Standard BioTools |
Anti-TIGIT | MBSA43 | 209Bi | Standard BioTools |