The evolution of drug resistance in clinical isolates of Candida albicans
Decision letter
-
Emmanouil T DermitzakisReviewing Editor; University of Geneva Medical School, Switzerland
eLife posts the editorial decision letter and author response on a selection of the published articles (subject to the approval of the authors). An edited version of the letter sent to the authors after peer review is shown, indicating the substantive concerns or comments; minor concerns are not usually shown. Reviewers have the opportunity to discuss the decision before the letter is sent (see review process). Similarly, the author response typically shows only responses to the major concerns raised by the reviewers.
Thank you for sending your work entitled “The mutational landscape of gradual acquisition of drug resistance in clinical isolates of Candida albicans” for consideration at eLife. Your article has been evaluated by a Senior editor and 3 reviewers, one of whom is a member of our Board of Reviewing Editors. The manuscript was considered appropriate for eLife but some serious concerns need to be addressed successfully before a final decision can be reached.
The following individuals responsible for the peer review of your submission want to reveal their identity: Manolis Dermitzakis (Reviewing editor), Jacques Fellay (peer reviewer), and Daniel Wilson (peer reviewer).
The Reviewing editor and the other reviewers discussed their comments before we reached this decision, and the Reviewing editor has assembled the following comments to help you prepare a revised submission.
The manuscript by Funt and colleagues provide an in-depth analysis of whole genome sequence of C. albicans progressively in HIV patients to infer selective events and describe patterns of mutations, and those that survive and likely have an impact on resistance. Overall, the study is large and the data collected is very important and relevant with a degree of phenotypic assessment paired with the sequence data. The experimental and sequence analysis methodologies seem to be state of the art.
1) One major concern arises from consideration of all sections of the manuscript. There is very little statistical modeling to assess how likely these combinations of certain mutational patterns are due to real selective events due to specific phenotypic consequences or just by chance. Most of the manuscript is presented in this style, and it is hard to know which signals are truly significant. We provide two of the many examples in the manuscript to illustrate this point:
In the Results section, the authors state: “We consider… two to three isolates”. While this is a reasonable argument, without proper statistics it is not possible to evaluate it. Perhaps some degree of linkage disequilibrium would be useful to consider.
In the Results section, the authors also mention: “The recurring appearance of LOH events that coincide with changes in MIC suggests that they have been positively selected…” This is a “coincidence”, as the authors say, but is it significant?
2) The last part of the Results describes some validation experiments where the specific mutations are brought to an experimental setting with deletion mutants. This is an experiment that was necessary but surprisingly it was not given the proper weight (it was only a small fraction of the total text), plus it was not as conclusive as one would like regarding the MIC phenotype.
3) Considering the central role in the paper of the various classes of mutations called from the sequencing reads, I think that one technical issue in particular deserves further exploration and/or discussion. The SNP calling accuracy was found to be 87.3% on average (using a Sequenome iPLEX genotyping assay; Results, “Sequenome iPLEX genotyping assay”, and Table 2). There was however an important inter-sample variability, with very poor results observed in half of the tested series (<65% calling accuracy). This is worrying. Could this be due to intra-patient diversity, with different Candida subpopulations being sampled for the two assays? Is there any other potential explanation? If not, these inconsistencies should be listed as an important limitation of the study.
4) The paper is presented as a study of the within-host mutations that occur and are selected for in the Candida albicans genome between longitudinally sampled genomes before and after the introduction of antibiotic treatment. However, the data suggest that the variants that distinguish C. albicans genomes at different time points did not arise during the sampling window but were largely, maybe entirely, pre-existing (standing variation). This view is based on: (i) the very considerable genetic diversity (thousands of SNP differences) between isolates of the same strain within the same host, sampled less than a few months apart, in one case on the same day; (ii) the implausible mutation rate that would be needed to explain this variation as newly arising by mutation; and, (iii) the lack of analysis demonstrating that the data shows any signal of “measurable evolution”.
https://doi.org/10.7554/eLife.00662.022Author response
We thank the reviewers’ for their thoughtful comments, which we addressed in full in the revised manuscript. We first highlight the key aspects of the revision and then address each point in the response below. We realize that this re-submission has been delayed: as we explain below this is due to our decision to re-sequence all strains to substantially greater depth to ensure the reproducibility and validity of our results. This was critical as this is a resource intended for the wider community.
1) Extensive deeper sequencing of each strain shows high concordance in genotype calls: the reviewers pointed out that there was a lower than desired agreement between our Sequenome iPLEX assay genotyping and the corresponding base calls from our sequencing data (87% in the original study on average per patient, 77% averaged over all samples with some strains near 100% and others much lower; new Figure 1–supplement 1A in red). The reviewer raised the possibility of a sample switching. We first ruled out the possibility of a sample switch by repeating our genotyping from newly isolated DNA, but many of the discrepancies remained. We then examined the discordant calls and found that those were typically related with lower sequence coverage. Although our initial analysis used best practices at the time of original data collection, in some regions of lower sequencing coverage our GATK pipeline defaulted to the genotype of the provided reference genome, resulting in erroneous SNP calls when comparing between strains. Because our strains are also a resource used widely by the community, we decided to re-sequence all the strains to high coverage (53-283X, an average of 103X); this was not feasible when our data was initially collected, but major increases in sequencing yields made it readily possible now. The new sequencing data resolved the discrepancies, such that concordance between Sequenom iPlex and Illumina data reached 94% across all patients (see also the response to the issue 3 below; Figure 1–figure supplement 1A in blue). All the key results and conclusions that we originally reported were all reproduced in this deeper analysis.
2) The new data has added many new interesting genes to our list of those most likely to have adaptive polymorphisms. Our repeated sequencing revealed 4,756 persistent SNPs across all isolates and 240 recurrent genes. While previous clustering analysis of persistent and recurrent SNPs failed to produce significant functional enrichments, clustering of the recurrence matrix of our new data reveals significant functional enrichment in four clusters (revised Figure 5). Additionally, of the 240 recurrent genes identified, 23 were available as deletion library mutants (up from five previously), and we evaluated them for phenotypic differences (revised Figure 8). These new data significantly extend our previous manuscript, and have allowed us to make additional conclusions regarding drug resistance and adaptation to the host environment.
3) We have clarified the two possible evolutionary scenarios—de novo mutation and selection or selection on largely pre-existing variation. We now clearly note that, although the data favors selection of pre-existing variation as the predominant mode, we cannot rule out that some of the observed variation was due to de novo events that occurred in the sampling window.
4) We have provided statistical modeling where possible, including stochastic simulations to model the occurrence of (1) persistent non synonymous SNP-containing ORFs associated with changes in MIC, and (2) the expected degree of recurrence amongst these ORFs.
5) We have heeded the reviewers’ suggestion to expand our description and interpretation of the validation experiments with the available deletion mutants for recurrent loci; as the number of recurrent loci grew, we now tested 23 deletion mutants, three of which were significantly more fit than the parental strain (SN250) as determined by growth in standard tissue culture media (RPMI).
6) We assessed the rate and time scale of evolution by applying Bayesian Evolutionary Analysis by Sampling Trees (BEAST) in the one patient series with a sufficient density of longitudinal samples and have included that analysis.
7) We addressed all other comments, including clarification of our use of the word “clonal” as detailed in the point-by-point response below.
Response to specific comments:
The manuscript by Funt and colleagues provide an in-depth analysis of whole genome sequence of C. albicans progressively in HIV patients to infer selective events and describe patterns of mutations, and those that survive and likely have an impact on resistance. Overall, the study is large and the data collected is very important and relevant with a degree of phenotypic assessment paired with the sequence data. The experimental and sequence analysis methodologies seem to be state of the art.
We thank the reviewers for these comments. As we note below, realizing that the data set could serve as a gold standard (and indeed is being used by several labs already), we decided to leverage the advances in sequencing yields, to generate much deeper sequencing and better analysis. Below we address each of the concerns raised.
1) One major concern arises from consideration of all sections of the manuscript. There is very little statistical modeling to assess how likely these combinations of certain mutational patterns are due to real selective events due to specific phenotypic consequences or just by chance. Most of the manuscript is presented in this style, and it is hard to know which signals are truly significant. We provide two of the many examples in the manuscript to illustrate this point:
In the Results section, the authors state: “We consider… two to three isolates”. While this is a reasonable argument, without proper statistics it is not possible to evaluate it. Perhaps some degree of linkage disequilibrium would be useful to consider.
In the Results section, the authors also mention: “The recurring appearance of LOH events that coincide with changes in MIC suggests that they have been positively selected…” This is a “coincidence”, as the authors say, but is it significant?
We thank the reviewers for this comment. We appreciate the suggestion for linkage or LD analysis, however it is important to emphasize that linkage/LD, by their very nature, are a function of mating and meiosis; and while mating can be engineered in the laboratory, meiosis has never been observed in C. albicans. Instead, C. albicans is thought to proliferate primarily by asexual reproduction and thought to only rarely undergo a cryptic, parasexual lifestyle in which two diploid individuals fuse into a tetraploid, and followed by chromosome loss in subsequent mitotic divisions to a converge on diploidy. This feature of C. albicans biology is a major limitation to genetic dissection of C. albicans population genetics either in the lab or in natural settings, and is important to bear in mind when considering our work.
It is for this reason that we think that those mutations that are simultaneously concomitant with increases in drug resistance and that are also recurrent are likely a footprint of selection (regardless of whether all were selected from existing diversity within the population, all arose de novo, or perhaps, a combination thereof). Without a substantially increased number of samples and non-treated human-passaged C. albicans longitudinal isolates, it is infeasible to adequately model this system for particular point mutations and genes affected. Furthermore, our samples were archived over decades, by clinicians who have typically archived only a single clone (sampled from what is likely a complex heterogeneous population). Our assessment of the larger gene sizes for those genes with recurrence in the original manuscript was an open acknowledgement of the possible contribution of random mutations to our results.
In the revised manuscript, we have now more clearly described the inherent challenges of this system and the limitations of our analysis, and we have attempted to clarify its statistical basis wherever possible. First, we now highlight these challenges early in the manuscript, clearly acknowledging the inherent limitations at deriving direct statistical significance driven by a model of the underlying phenomena. Second, whenever possible, we articulate the statistical basis for our analyses. For example, consider the LOH presented in Figures 3 and 4. There are 30 LOH events, if we limit LOH events to chromosomes arms (of which they occur on 15 out of 16 possible chromosome arms). Of these 30 LOHs, 23 meet our standard for persistence. The right arm of chromosome 3 has a persistent LOH 7 times. If we assume a naïve binary distribution, then the probability of this occurring is less than = 4.76 × 10-4. The same calculation applies to the left of arm 5 (Chr5L), but with 5 LOH events for a probability of 0.0128. Unlike chromosome 3, Chr5L is known to be a functionally significant LOH (Coste et al., 2006; Selmecki et. al, 2006 and 2008). We have added this analysis to the text.
Furthermore, we now use stochastic simulations to generate null distributions of: (1) the expected number of persistent non-synonymous SNP-containing ORFs associated with changes in MIC; and (2) the degree of recurrence amongst these ORFs. These models (described in the revised Methods) suggest that the number of observed cases of each of (1) and (2) is significantly larger than expected by chance (p<0.0001). The distributions are shown in Author response image 1 below, and the results are reported in the revised text.
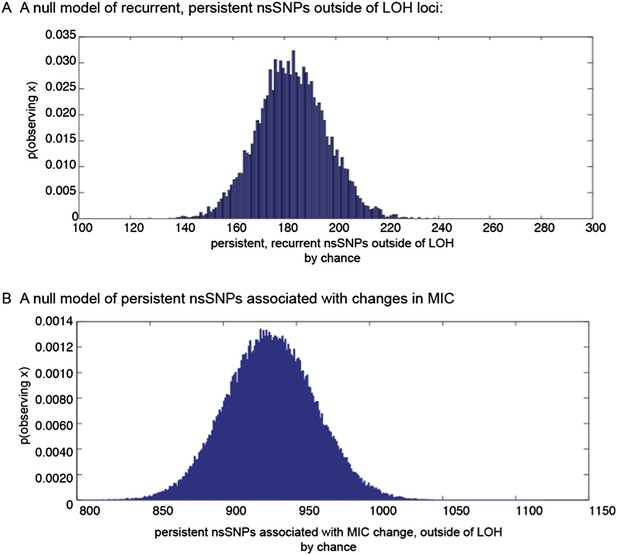
Null distributions of (a) the expected number of persistent, recurrent non synonymous SNPs outside of LOH regions, and (b) persistent non-synonymous SNPs associated with MIC changes outside of LOH regions.
2) The last part of the Results describes some validation experiments where the specific mutations are brought to an experimental setting with deletion mutants. This is an experiment that was necessary but surprisingly it was not given the proper weight (it was only a small fraction of the total text), plus it was not as conclusive as one would like regarding the MIC phenotype.
We thank the reviewers for the suggestion to further emphasize these results . The diploid genome and lack of a complete sexual cycle make genetic manipulation of C. albicans challenging. Therefore, we relied on an existing resource of available homozygous deletion mutants from a deletion strain collection of 674 loci to gain insight into the contribution of these loci to drug resistance and fitness. In the original manuscript, we tested all those loci recurrently mutated by our original analysis for which a deletion mutant was available.
Following our deeper sequencing, we could reliably detect a larger number of recurrently mutated loci, and hence could test 23 deletion strains (revised Figure 8). In this expanded panel of tests, consistent with a role in host adaptation, 8 out of 23 mutants affected in vitro fitness in a culture medium thought to approximate in vivo conditions. Strikingly, three mutants show an increased level of fitness relative to the WT parental isolate, SN250. We have clarified the text to convey this point. The results reported in the original submission were from fitness experiments conducted using the drug-resistant reference strain (also used in the fitness experiments illustrated in revised Figure 7B). In principle, any reference strain can be used in relative fitness experiments. However, in this revision we used the same reference strain to quantify the fitness of the clinical isolates and the deletion mutants, such that that results in Figure 7A and Figure 8 can be readily comparable by the reader.
3) Considering the central role in the paper of the various classes of mutations called from the sequencing reads, I think that one technical issue in particular deserves further exploration and/or discussion. The SNP calling accuracy was found to be 87.3% on average (using a Sequenome iPLEX genotyping assay; Results, “Sequenome iPLEX genotyping assay”, and Table 2). There was however an important inter-sample variability, with very poor results observed in half of the tested series (<65% calling accuracy). This is worrying. Could this be due to intra-patient diversity, with different Candida subpopulations being sampled for the two assays? Is there any other potential explanation? If not, these inconsistencies should be listed as an important limitation of the study.
The same genomic DNA from a single archived clone was used for both the original sequencing and the Sequenom assay. We were originally concerned that a switching in samples was the cause of the discrepancy, but repeated genotyping and re-examination of the Sequenome iPLEX versus the sequencing data suggested that when sequence coverage was relatively low, our GATK pipeline defaulted to the genotype of the provided reference genome resulting in erroneous SNPs calls across isolates in the same time course. Given that the extensive sequence data set is a major strength of our study, and with the substantial increase in sequencing yields since the time when we originally sequenced these isolates, we chose to re-sequence all the strains to high coverage (53-283X, average depth of coverage: 103X).
The new sequencing data resolved the discordance between the Sequenom and sequencing genotype calls (Table 2) such that our average concordance rose to 94% (85%-98%, Figure 1–figure supplement 1A). To further understand the nature of our discordant sites, and importantly, determine if improved filtering of the SNP calls could improve concordance, we examined the discordant sites for any potential base bias (Figure 1–figure supplement 1). From this analysis, it is apparent that the majority of discordant sites are those that are identified as homozygous by Sequenom iPlex (Figure 1–figure supplement 1B, teal bars), but as heterozygous by Illumina sequencing (Figure 1–figure supplement 1B, orange bars). We compared several quality metrics for these sites in our Illumina data for discordant vs. concordant sites (Figure 1–figure supplement 1C-G: depth of coverage (DP), mapping quality (MQ), PHRED scaled quality scores (QUAL), the QD score (confidence of a variant call), and the ratio of alternative allele to reference allele (AB)). We found that while the distributions of some quality scores for discordant sites are somewhat lower, the degree of overlap is so extensive that any improvement in specificity will come at a substantial sensitivity cost. Given that the discordance rate is already low, and that we focus on SNPs that both persist within a series and recur across series (and are thus called many independent times), we opted not to use any stricter thresholds for SNP calling. We present this comparison in the revised text, in the Results section.
Importantly, all previous conclusions were reproduced in this enhanced analysis, and we were able to draw additional conclusions based on these higher quality data. For example, the new set of 240 mutated genes is now enriched for several functions known to influence pathogenicity, including fungal-type cell wall (18 genes, p<0.0012) and cell surface genes (24 genes, p<0.00012), with several members in each of three cell wall gene families important for biofilm formation and virulence (Hoyer et al., 2008): the Hyr/Iff proteins (HYR1 and 3, IFF8 and 6), the ALS adhesins (ALS1-4,7,9), and the PGA-30-like proteins (7 genes) (Figure 5–source data 1). All three families are specifically expanded in the genomes of pathogenic Candida species (Butler et al., 2009). In addition, seven members of the FGR gene family, involved in filamentous growth that is specifically expanded in C. albicans (Butler et al., 2009), are also among the 240 genes (Figure 5–source data 1).
4) The paper is presented as a study of the within-host mutations that occur and are selected for in the Candida albicans genome between longitudinally sampled genomes before and after the introduction of antibiotic treatment. However, the data suggest that the variants that distinguish C. albicans genomes at different time points did not arise during the sampling window but were largely, maybe entirely, pre-existing (standing variation). This view is based on: (i) the very considerable genetic diversity (thousands of SNP differences) between isolates of the same strain within the same host, sampled less than a few months apart, in one case on the same day; (ii) the implausible mutation rate that would be needed to explain this variation as newly arising by mutation; and, (iii) the lack of analysis demonstrating that the data shows any signal of “measurable evolution”.
We completely agree with the reviewers that, given the evidence of considerable genetic diversity existing in the same host, selection may primarily be driving an increase in the frequency of variants that already existed in the population. We have revised the text throughout the manuscript to clarify this point (especially in the Introduction and Results sections). Nevertheless, there is also ample evidence that mutation rates are increased under stressful conditions (e.g., drug treatment) in many systems, including yeasts, akin to what occurs in cancer (Galhardo et al., 2007). Mitotic recombination events that give rise to LOH occur at a much higher rate (3 x 10-5) than single-nucleotide mutation rates and are also increased by stress. Specifically relevant here, LOH rates are elevated in C. albicans treated with fluconazole (Forche et al., 2011). Thus, we cannot rule out the possibility that some genetic changes, including LOH events, occurred de novo during the treatment and sampling window. We discuss this point in the revised manuscript, in the Discussion.
Importantly, we are unable to distinguish pre-existing from de novo events with the data in hand. The rate of collection and number of samples we have for the majority of patients likely does not constitute a dataset that can be analyzed by existing approaches to ‘measurably evolving population’ (MEP, as per Drummond et al., 2003), and we do not want to overstate our evolutionary scenario. However, even if, as the reviewers suggest, “all ‘persistent mutations’ are those pre-existing variants driven to sufficiently high frequency by selection to ensure repeated sampling, whereas transiently observed variants are not”, this scenario is still an evolutionary process, involving heritable variation and selection, and can still inform our functional understanding of host-pathogen interactions.
With these limitations in mind, we were intrigued by the reviewers’ suggestion to assess the rate and time scale of evolution in these strains. While the majority of our patient series represent only a few sequentially isolated clonal samples, Patient 1’s series consists of 16 clonal, sequential isolates sampled over a period of nearly two years, and thus these samples can be analyzed for evidence of measurable evolution. We have used a Bayesian Markov Chain Monte-Carlo approach (Bayesian Evolutionary Analysis by Sampling Trees, BEAST; Drummond et al., 2012) to assess the rate of mutation in these populations and the likely time to most recent common ancestor (TMRCA).
To test the hypothesis that the majority of mutations occurred before the sampling period, we sampled trees generated using a set of parameters based on available data. Because there are no estimates of the per-basepair, per-generation mutation rate in C. albicans, we began with the assumption that C. albicans mutates at a rate similar to the per-base pair, per-generation rate of S. cerevisiae (Lynch et al., 2008). We then adjusted this rate to a per-day rate based on the generation time observed for C. albicans in a mouse model (1.56 generations per day, Forche et al, 2009), and determined the TMRCA for the isolates from Patient 1. Based on these assumptions, the TMRCA for these 16 isolates is ∼ 235 years (Author response image 2A). Indeed, using these parameters, many of the nodes separating two isolates are dated to be ∼150 years in the past. While little is known of the genetic diversity present in C. albicans populations at the time of colonization, it seems unlikely that the most recent common ancestor for the clonal population seen in Patient 1 evolved 235 years prior to isolation: if colonization occurs primarily during birth, it would mean that much of the diversity present in this patient actually evolved in the patient’s grandmother, or great grandmother.
To better understand the diversity present in the population, we repeated this analysis, but with a broader estimate of mutation rate (for the prior, the same mean as above, 5.5 x 10-10 mutations/bp/day, but with a larger standard deviation), resulting in a 5 to 55 fold increase in the estimated substitution rate, and a corresponding reduction in the likely TMRCA (Author response image 2B,C). To determine which of these three models best fit the data, we performed a pairwise calculation of Bayes factors (Author response image 2D), and we find that model (C), with an estimated substitution rate of 8.48 x 10-8 best approximates our data. These results are consistent with the reviewer’s comment that considerable variation developed before the onset of treatment and sampling; however, they also suggest that a substantial portion of the observed variation occurred during the treatment period in Patient 1. Importantly, they also suggest that an elevated mutation rate likely explains some of the diversity we observe, consistent with previous observations of in vivo hypermutability during infection and treatment (Oliver et al, Science 2000, Galhardo et al., 2007, Forche et al., 2011).
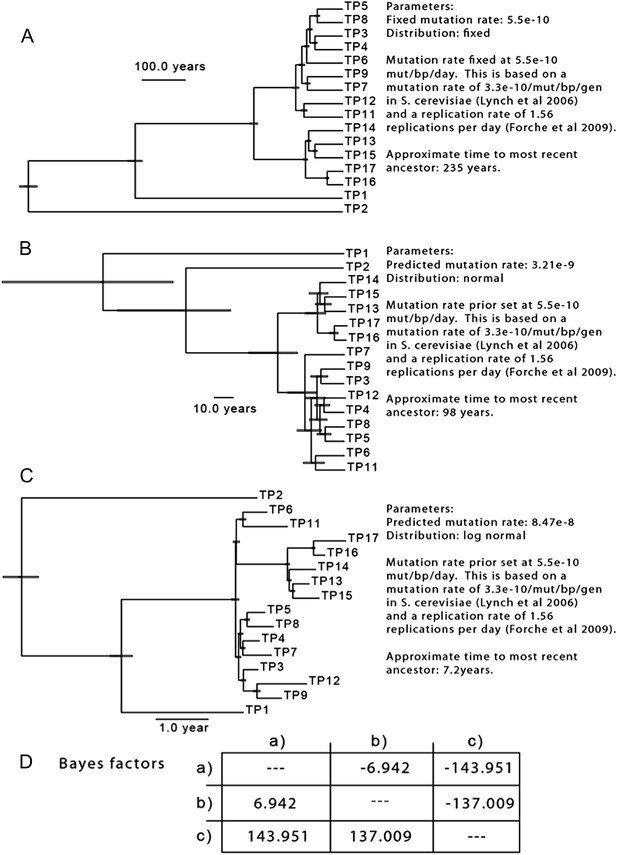
The results of three separate MCMC analyses based on differing priors for mutation rate are shown. This analysis is based only on SNPs from isolates of Patient 1.
Further work is necessary to determine: (1) the extent of population diversity at any given time point, (2) the extent of the bottlenecks during colonization and drug treatment, and (3) the impact of the host immune response and antifungal therapy on the mutation rate Candida albicans. In light of these issues—which are relevant to a variety of pathogens—this analysis is particularly provocative. However, as many of the parameters necessary to make these estimates are poorly informed and our sampling limits the analysis to only one patient series, we do not feel this analysis merits inclusion in the manuscript.
https://doi.org/10.7554/eLife.00662.023