Multiple abiotic stimuli are integrated in the regulation of rice gene expression under field conditions
Figures
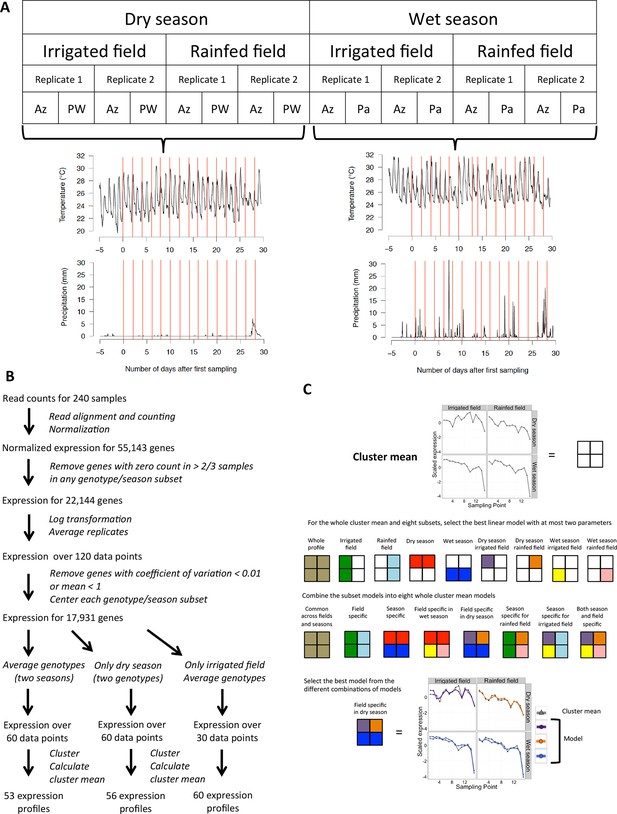
Modeling environmental and developmental effects on rice gene expression in the field.
(A) Experimental design: fifteen sampling timepoints for each of the 16 season/field/replicate/genotype series amounts to 240 samples, representative of 30 different sets of climatic conditions, represented here by the 30 red lines on the graphs for temperature and precipitation (accumulated mm per 5 min) along the sampling period of each growing season. (B) Processing raw RNA sequencing data into main transcriptomic variation for different subsets of our data. (C) Modeling potential differences between fields and seasons for climatic/developmental response within a cluster mean. An example for cluster 15 of the two-season analysis, where the model selected is common to both fields in the wet season but field specific in the dry season. Az, Azucena landrace; Pa, Palawan landrace; PW, Pandan Wangi landrace.
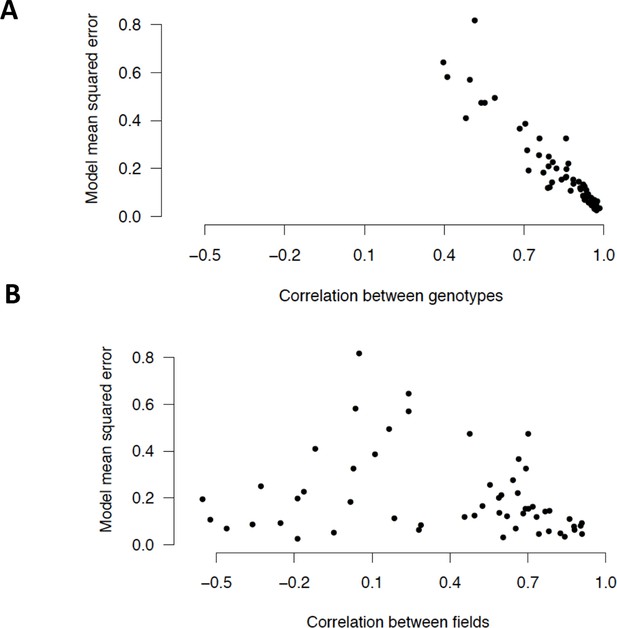
In the dry season analysis, expression patterns of the two genotypes are highly correlated for most clusters while for the few other clusters, differences between the genotypes are poorly explained by the models (high model MSEs for clusters with low correlation).
(A) Model MSE vs. correlation (Pearson coefficient) between the genotypes for the 56 dry season cluster means. (B) Model MSE vs. correlation between the fields for the 56 dry season cluster means. MSE, mean squared error
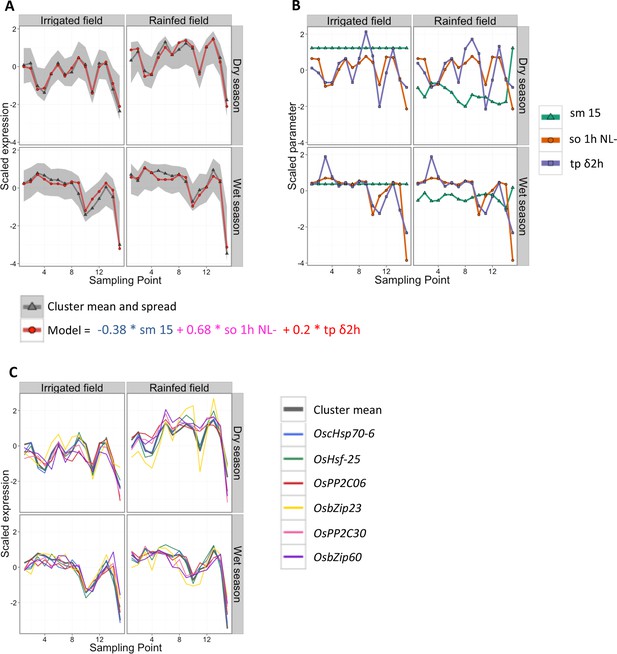
Cluster 9 of the two-season analysis: its environmental/developmental model and some of the genes it contains that have a potential function in environmental response.
(A) Gene expression for the cluster mean (grey) and spread (calculated as 10% and 90% quantile of all genes in the cluster for each data point; grey area), and cluster model (red). (B) Scaled climatic parameters in the model equation for each season. sm 15: soil moisture at 15 cm; so 1h NL-: one hour average of solar radiation transformed to increase the effect of low values; tp δ2h: change in temperature from 2 hr ago. (C) Gene expression pattern (scaled) of six genes in cluster 9 with a potential function in environmental response.
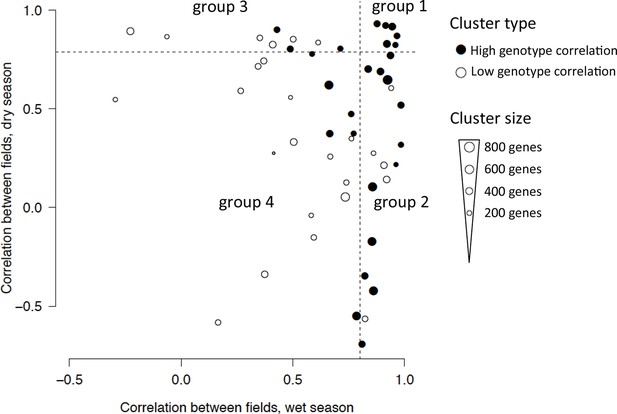
Classification of the 53 clusters from the two-season analysis based on field correlation in the dry and wet seasons.
Each dot represents a cluster with the size of the dot proportional to the number of genes in the cluster. The 27-gene clusters with igh genotype correlation (r > 0.9, Pearson coefficient) are represented with a filled circle and divided into four groups depending on whether their correlation between fields in each season is below or above 0.8 (dashed lines), referred to as high or low correlation. Group 1 clusters have a high correlation between fields in both seasons; group 2 clusters have a high correlation in the wet season but not in the dry season; group 3 clusters have a high field correlation in the dry season but not in the wet season; group 4 clusters have a low field correlation in both seasons.
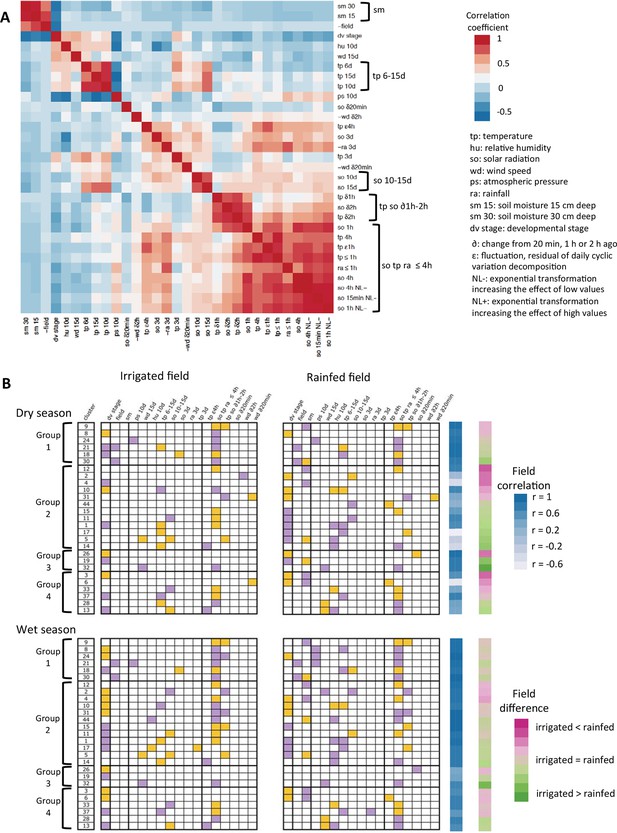
Summary of the ED models selected for the 27-gene clusters with high genotype correlation in the two-season analysis.
(A) Justification for the grouping of model parameters: heat-map of the correlation between the ED parameters selected at least once in the models. For some parameters that have strong negative correlations with other parameters, we used negative values to better see groups of correlated parameters independently of the sign of the correlation. (B) The selection of a parameter into a model is represented by a colored box, orange for a positive term and purple for a negative term. Field correlation is the correlation between irrigated and rainfed field profiles for each season. Field difference is the average expression level in the rainfed field minus the average expression level in the irrigated field. ED, environmental/developmental
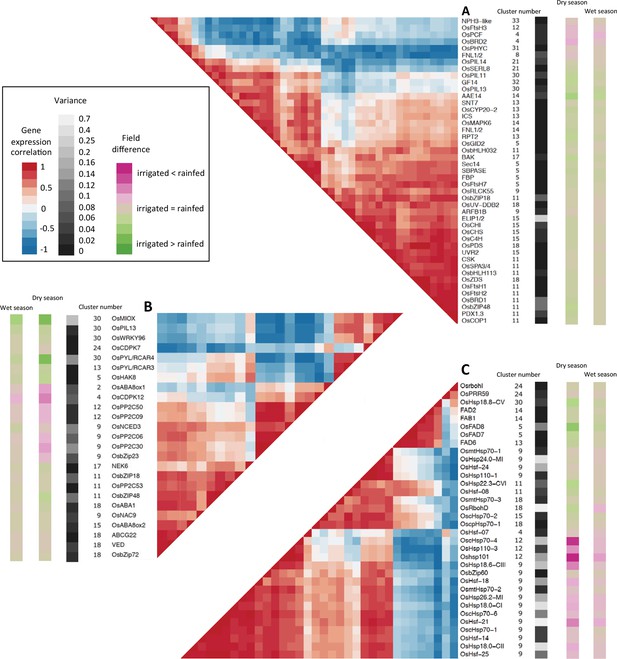
Co-expression and variance of genes involved in environmental response.
High variance is represented with lighter shades of gray. The genes represented have a genotype correlation above 0.8. Gene names starting with “Os” are rice gene names, others are the names of A. thaliana orthologous or homologous genes or gene families. Field difference is average expression level in the rainfed field minus average expression level in the irrigated field. Variance is the mean of the expression variance of each gene across the four season/field environment subsets. (A) Genes potentially involved in response to light. (B) Genes potentially involved in response to drought. (C) Genes potentially involved in response to temperature.
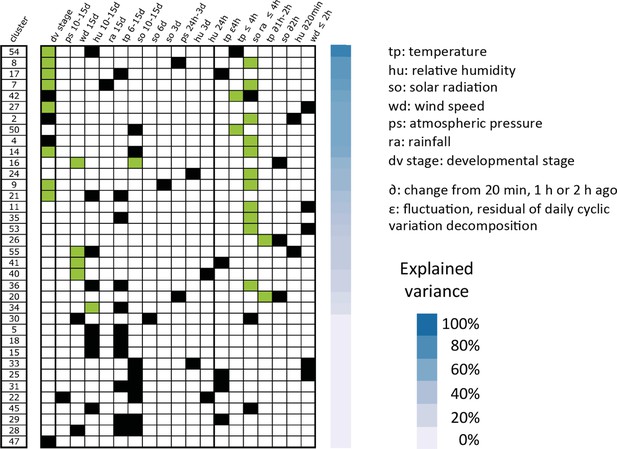
Transferability of two seasons irrigated field models to an independent dataset of rice gene expression under temperate climate.
Models were selected for 60 clusters using our expression data in the irrigated field during the wet and dry seasons. The parameters from these models that could be transferred to explain the mean expression of the cluster mean in the PND are shown with a green box, the ones that were not transferred are indicated with a black box. The variance of the PND cluster mean explained by the partially transferred model is represented with decreasing shades of blue. PND, partial Nagano dataset.
Tables
Climatic parameters for the environmental/developmental models and their abbreviations. Parameters calculated from the weather data are mostly ordinary averages (linear: L) for a large range of time windows before sampling but also include exponentially transformed averages (non-linear: NL) modeling stronger effects at either low (NL-) or high (NL+) values. For the most dynamic climatic parameters, we calculated differences (D) between the measurement at the sampling timepoint and the measurement short amounts of time before sampling. To estimate fluctuations, we calculated averages of the residual term (R) from the seasonal decomposition of daily variation into cyclic and trend components. The abbreviation for each climatic factor and parameter type and time-window is given in parentheses.
Average/change for the last | Temperature (tp) | Relative humidity (hu) | Solar radiation (so) | Wind speed (wd) | Atmospheric pressure (ps) | Rainfall (ra) | |
---|---|---|---|---|---|---|---|
Sampling time | 15 min (15 min) | L | L | L NL− NL+ | L | L | L |
Short-term averages | 1 hr (1 hr) | L | L | L NL− NL+ | L | L | L |
4 hr (4 hr) | L | L | L NL− NL+ | L | L | L | |
24 hr (24 hr) | L | L | L | L | L | L | |
Long-term averages | 3 d (3 d) | L | L | L | L | L | L |
6 d (6 d) | L | L | L | L | L | L | |
10 d (10 d) | L | L | L | L | L | L | |
15 d (15 d) | L | L | L | L | L | L | |
Recent change | 20 min (δ20 min) | D | D | D | D | ||
1 hr (δ1 hr) | D | D | D | D | |||
2 hr (δ2 hr) | D | D | D | D | |||
Fluctuations | 1 hr (ε1 hr) | R | |||||
4 hr (ε4 hr) | R | ||||||
24 hr (ε24 hr) | R |
Additional files
-
Supplementary file 1
Detailed characteristics and models for all the clusters in the two-season analysis; results used for the comparison of the partial Nagano dataset with our analysis.
- https://doi.org/10.7554/eLife.08411.011
-
Supplementary file 2
Weather data for the dry and wet season experiments.
- https://doi.org/10.7554/eLife.08411.012
-
Source code 1
Clustering, model selection for the two-season analysis and analysis of the partial Nagano dataset.
- https://doi.org/10.7554/eLife.08411.013