A cholinergic feedback circuit to regulate striatal population uncertainty and optimize reinforcement learning
Figures
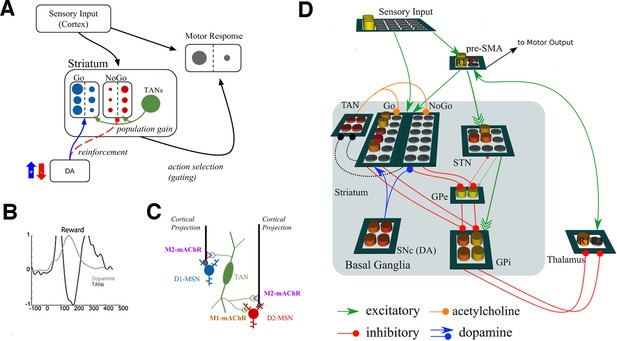
Neural network model.
(A) A simplified diagram of the neural network. Sensory input excites candidate motor actions and corresponding Go (blue circles) and NoGo (red circles) MSN units in the striatum. Distinct columns of Go and NoGo units provide the thalamus with positive and negative evidence for alternative motor responses, learned via DA reinforcement signals. Positive prediction errors result in increased DA during feedback and an increase of Go excitability relative to NoGo units. TANs are endogenously active units that modulate MSN excitability during reinforcement feedback, altering the efficacy of the reinforcement signal. (B) Stereotypical TAN response is temporally concomitant to reward-related phasic DA increase (Adapted from Figure 7 part C, Morris et al., 2004). (C) Schematic representation of TAN–MSN signaling (see below). TAN firing inhibits presynaptic glutamatergic signaling of D1 and D2 MSNs through M2 receptors, but also selectively excites D2 MSNs via M1 receptors. (D) Sensory input provides excitatory signaling to preSMA (representing two candidate motor actions) and corresponding Go and NoGo MSN units in the striatum. Each of (here, two) motor responses is coded by distinct columns of Go and NoGo units, representing positive and negative evidence for alternative that responses, learned via reinforcement conveyed by DA signals in the SNc. The basal ganglia contribute to action selection by disinhibiting thalamocortical activity for representing thatthe response having the largest Go–NoGo activation differential. Go units inhibit globus pallidus internal segment (GPi) units, which otherwise tonically inhibit the thalamus. NoGo units have the opposite effect by inhibiting the external segment (GPe), which in turn inhibits GPi. TANs are represented as a separate layer of endogenously active units that modulate MSN excitability during the dynamic burst–pause pattern windowing the dopaminergic reward prediction error signals. This pause duration can be fixed, or sensitive to the population uncertainty of MSNs (see below). Dotted black lines correspond to proposed feedback mechanism from MSNs to TANs. The STN modulates the threshold by which actions are disinhibited by the BG, included here for consistency with prior work. DA, dopamine; MSN, medium spiny neuron; preSMA, pre-suplementary motor cortex; SNc, substantia nigra pars compacta; STN, subthalamic nucleus.
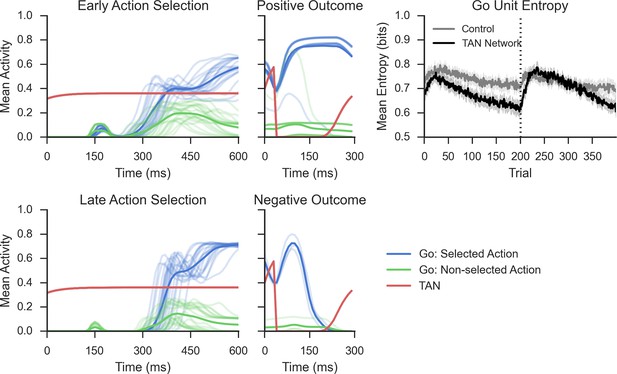
Network activity in a probabilistic reversal learning task.
Top left: Mean within-trial normalized firing rate across population of Go units (simulated striatonigral MSNs) and TANs during action selection during the first epoch of training ('early'). Individual traces represent the mean population activity in a single trial and are collapsed across response for Go units. In the examples shown, Go unit activity ultimately facilitates the selected response (due to disinhibition of thalamus; not shown, see Frank, 2006). Bottom left: Mean within-trial firing rate during action selection in the last epoch of training ('late') prior to reversal. As a consequence of training, an increased differential in Go activity between the selected and non-selected response results in low action-selection uncertainty. Center: Mean firing rate for Go and TAN units during reinforcement for both correct (top) and incorrect (bottom) trials. TAN pauses occur for both types of feedback, providing a window during which the dopaminergic signals differentially modulate Go unit activity and plasticity (long-term potentiation vs long-term depression). Top right: Entropy in population of Go units across training trials. Population-level entropy declined over time prior to reversal (trial 200; dotted line) as the stochastic population of simulated neurons learned the correct action. Following reversal, entropy rose briefly as the network starts to activate the alternative Go response while the learned one still remained active for some time, after which entropy declines once more. This dynamic modulation of entropy is more pronounced in a network with simulated TANs than a control network without TANs. MSNs, medium spiny neurons; TANs, tonically active neurons.
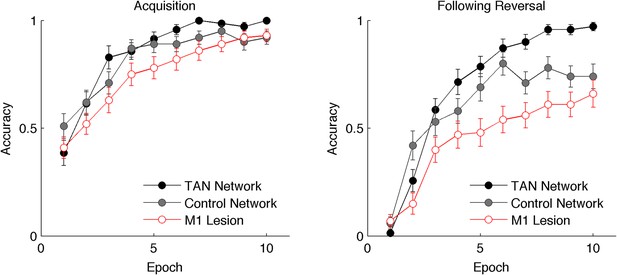
Performance of the neural network on reversal learning task.
Left: Accuracy during acquisition of probabilistic contingencies. During initial acquisition, accuracy for networks simulated with TANs (black) is similar to accuracy in control networks that do not contain TANs (grey). Simulated M1 blockade (red) does not meaningfully impair performance during acquisition. Right: Accuracy following reversal. Networks with TANs (black) reach higher asymptotic performance than control networks (grey). Networks with simulated M1 blockade (red) show pronounced performance impairments. TANs, tonically active neurons.
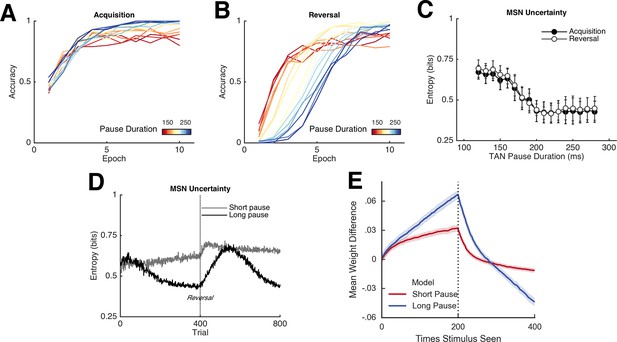
Network behavior as a function of TAN pause duration.
(A, B): Accuracy of the neural network simulations over a range of fixed pause durations (120–280 ms) during initial acquisition (A) and following reversal (B). Networks with short TAN pauses (red) learned more quickly following reversal than networks with a long TAN pauses (blue) but did not achieve the same level of asymptotic accuracy during either acquisition or following reversal. (C) Final MSN entropy after training for both acquisition and reversal, as a function of TAN pause duration. Longer pauses elicited lower entropic MSN representations, facilitating asymptotic performance, whereas the higher entropy in short pause networks facilitated faster reversal. (D) Action selection uncertainty across MSN population across all trials for both stimuli. Entropy in networks with a long TAN pause (black) declines over time and reaches asymptotic level both prior to and following reversal. (E) Mean difference in corticostriatal Go weights (synaptic efficacy) coding for the more rewarded versus less rewarded response (defined during acquisition) are shown across training trials for a single stimulus, in an 85:15% reward environment. Greater accumulation in weight differences result in more deterministic choice but difficulty in unlearning. Data shown for networks trained on with a long (270 ms) or short (150 ms) fixed duration TAN pause. Reversal at trial 200 denoted with dotted line. MSN, medium spiny neurons; TANs, tonically active neurons.
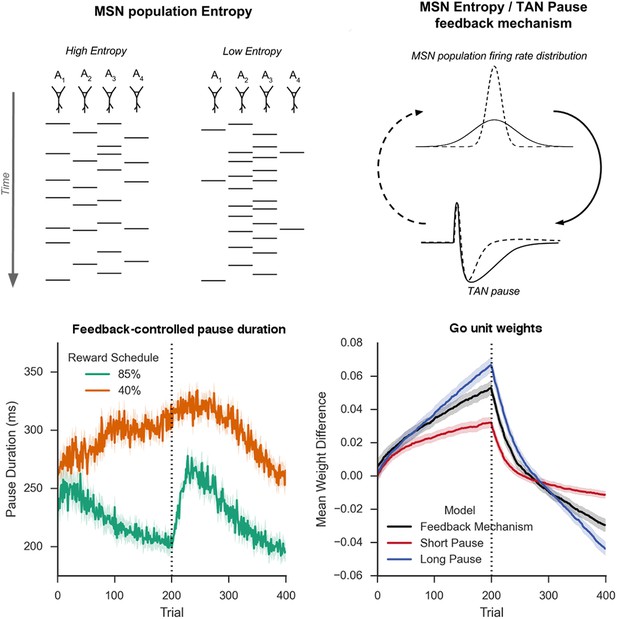
Schematic Representation of MSN entropy and self-tuning mechanism.
Top left: Action potentials (horizontal lines) for a population of neurons with both high and low population entropy are shown across time. In the example, both entropy conditions produce the same number of action potentials in the displayed time window. In the population with high entropy, action potentials are distributed equally across the neurons thus reflecting more competition across MSNs representing different behavioral actions, and hence uncertainty about which action to select. In the population with low entropy, spike rates are concentrated in fewer neurons. Top right: Homeostatic feedback mechanism. The population entropy across spiny neurons can influence the duration of TAN pauses, which reciprocally influences the excitability of spiny neurons and hence entropy. This feedback control system optimizes the TAN pause duration and allows the population to be dynamically sensitive to unexpected outcomes. A high-entropy MSN population firing rate distribution (top, black line) leads to a longer TAN pause (bottom, black line), increasing weight divergence and creating downward pressure on MSN entropy. Low MSN entropy (top, dotted line) decreases TAN pause duration (bottom, dotted line) creating upward pressure on MSN entropy. Bottom left: Feedback-mechanism self-regulates TAN pause duration (ms) as a function of entropy across learning. Pauses become shorter for more deterministic environments as a function of learning, preventing divergence in synaptic weights and over-learning, but then increase at change points. Reversal at trial 200 denoted with dotted line. Bottom right: Feedback mechanism adaptively regulates synaptic weights to facilitate learning. Mean difference in corticostriatal Go weights (synaptic efficacy) coding for the more rewarded versus less rewarded response (defined during acquisition) are shown across training trials, in an 85:15% reward environment. Greater accumulation in weight differences result in more deterministic choice but difficulty in unlearning. Data shown for networks trained on with a long (270 ms) or short (150 ms) fixed duration TAN pause, as well as a network employing an entropy-driven feedback mechanism. Reversal at trial 200 denoted with dotted line. MSNs, medium spiny neurons; TANs, tonically active neurons.
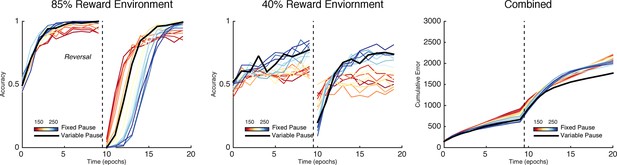
Learning in the neural network across multiple reward schedules.
Left: Networks with fixed short TAN pauses across training (red) exhibited higher performance in an environment with 85:15% reward contingencies for the optimal and suboptimal actions, compared with networks with long TAN pauses (blue), due to increased learning speed following reversal. Center: Networks with long TAN pauses (blue) were able to acquire a task with 40:10% contingency, unlike networks with a short TAN pause (red). Networks with variable pause durations as a function of MSN entropy are shown in black, with good performance in both environments. Right: Across both reward schedules, networks in which TAN pause duration was allowed to vary with MSN entropy (black) made fewer errors than networks of any fixed duration. Reversal is denoted with dotted line in all panels. MSNs, medium spiny neurons; TANs, tonically active neurons.
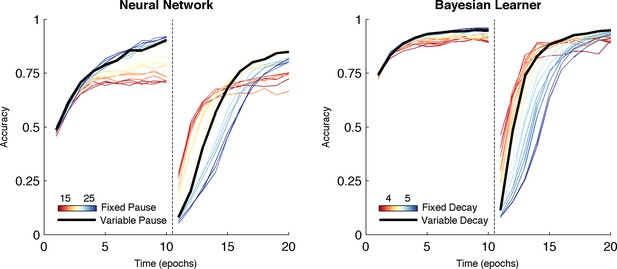
Comparison of behavior between neural network and Bayesian learner collapsed across multiple reward schedules.
The performance of the Bayesian learner (i) is qualitatively similar to the performance of the neural network model (left). A slow decay rate in the Bayesian learner (right, blue) has the same effect as a long TAN pause (left, blue) and results in higher asymptotic accuracy at a cost of slower learning following reversal. A fast decay rate (right, red) has the same effect as a short TAN pause (left, red) and results in fasters learning following reversal with lower asymptotic accuracy. Varying the decay rate and pause duration with entropy in the Bayesian learner and neural network, respectively, mitigates the trade-off. Reversal is denoted with dotted line in both panels.
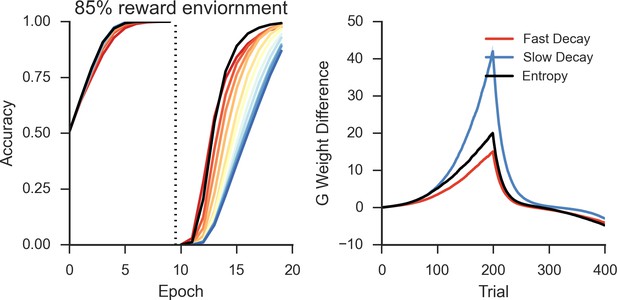
Peformance of OpAL in an 85% reward environment.
Left: Fast decay in synaptic weights (red lines) result in lower asymptotic accuracy but speeded learning following reversal while slow weight decay (blue lines) result in the opposite pattern. A model that varies the decay rate with policy uncertainty (black line) mitigates this trade-off. Right: Slow decay rates result in a large divergence between G weights for the two possible actions prior to reversal (trial 200) that correlates with high asymptotic accuracy and slower learning speed following reversal, as this divergence must be unlearned. An entropy modulated decay rate shows a high initial rate of divergence sufficient to improve accuracy but slows as the model learns the task, facilitating reversal.
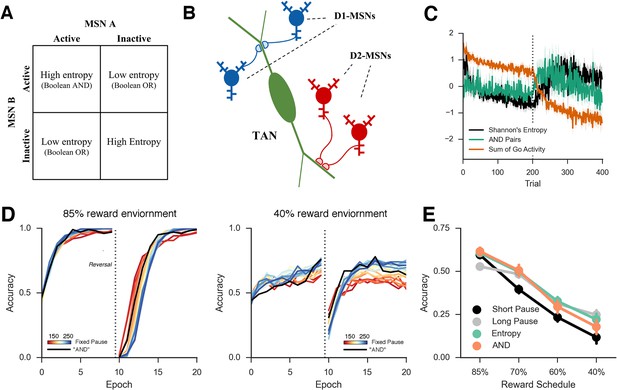
Approximations of MSN population uncertainty.
(A) Shannon’s entropy for two MSNs can be expressed with Boolean logic. Low entropy occurs when only one MSN is active (bottom left or top right box) and is the exclusive-OR function. High entropy is the logical opposite. (B) Spatially organized synapses could allow the detection of activity in two MSNs associated with separate motor responses, indicating high entropy. Activity in pairs of D1-MSNs signal high uncertainty given evidence for multiple responses, activity in pairs of D2-MSNs signal high uncertainty given evidence against multiple responses. (C) Detection of 'AND' pairs in Go population approximates Shannon’s entropy across time, whereas simple summation of all Go unit activity does not. Dotted line denotes reversal at mid-point in training. (D) Neural network with AND detection performs well in both an 85% reward (left) and 40% reward (right) environments as compared to networks with fixed TAN behavior. (E) Varying pause duration with Shannon’s entropy (green line) or the detection of AND conjunctions (orange line) results in similar behavior. MSNs, medium spiny neurons; TANs, tonically active neurons.
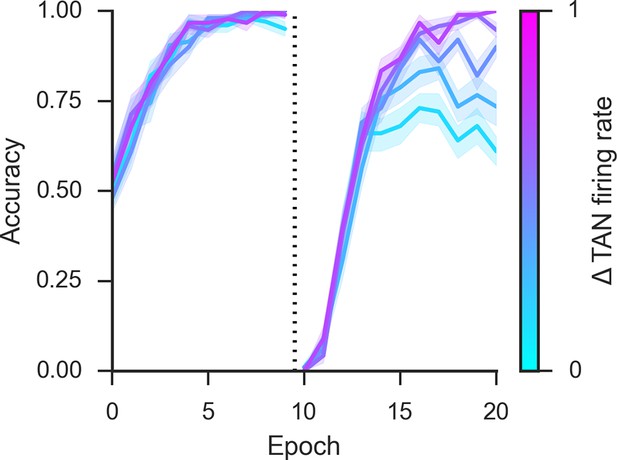
Post-pause TAN burst.
An increase in phasic TAN activity following the feedback-related pause modulates asymptotic performance following reversal. Simulations shown with a fixed TAN pause of intermediate duration (190 ms) in an 85% reward environment, post-pause TAN firing rates are presented in normalized units of change relative over a baseline firing rate corresponding to the tonic firing rate. TAN, tonically active neuron.