Data-driven identification of potential Zika virus vectors
Abstract
Zika is an emerging virus whose rapid spread is of great public health concern. Knowledge about transmission remains incomplete, especially concerning potential transmission in geographic areas in which it has not yet been introduced. To identify unknown vectors of Zika, we developed a data-driven model linking vector species and the Zika virus via vector-virus trait combinations that confer a propensity toward associations in an ecological network connecting flaviviruses and their mosquito vectors. Our model predicts that thirty-five species may be able to transmit the virus, seven of which are found in the continental United States, including Culex quinquefasciatus and Cx. pipiens. We suggest that empirical studies prioritize these species to confirm predictions of vector competence, enabling the correct identification of populations at risk for transmission within the United States.
https://doi.org/10.7554/eLife.22053.001eLife digest
Mosquitoes carry several diseases that pose an emerging threat to society. Outbreaks of these diseases are often sudden and can spread to previously unaffected areas. For example, the Zika virus was discovered in 1947, but only received international attention when it spread to the Americas in 2014, where it caused over 100,000 cases in Brazil alone. While we now recognize the threat Zika can pose for public health, our knowledge about the ecology of the disease remains poor. Nine species of mosquitoes are known to be able to carry the Zika virus, but it cannot be ruled out that other mosquitoes may also be able to spread the disease.
There are hundreds of species of mosquitoes, and testing all of them is difficult and costly. So far, only a small number of species have been tested to see if they transmit Zika. However, computational tools called decision trees could help by predicting which mosquitoes can transmit a virus based on common traits, such as a mosquito's geographic range, or the symptoms of a virus.
Evans et al. used decision trees to create a model that predicts which species of mosquitoes are potential carriers of Zika virus and should therefore be prioritized for testing. The model took into account all known viruses that belong to the same family as Zika virus and the mosquitoes that carry them. Evans et al. predict that 35 species may be able to carry the Zika virus, seven of which are found in the United States. Two of these mosquito species are known to transmit West Nile Virus and are therefore prime examples of species that should be prioritized for testing. Together, the ranges of the seven American species encompass the whole United States, suggesting Zika virus could affect a much larger area than previously anticipated.
The next step following on from this work will be to carry out experiments to test if the 35 mosquitoes identified by the model are actually able to transmit the Zika virus.
https://doi.org/10.7554/eLife.22053.002Introduction
In 2014, Zika virus was introduced into Brazil and Haiti, from where it rapidly spread throughout the Americas. By January 2017, over 100,000 cases had been confirmed in 24 different states in Brazil (http://ais.paho.org/phip/viz/ed_zika_cases.asp), with large numbers of reports from many other counties in South and Central America (Faria et al., 2016). Originally isolated in Uganda in 1947, the virus remained poorly understood until it began to spread within the South Pacific, including an outbreak affecting 75% of the residents on the island of Yap in 2007 (49 confirmed cases) and over 32,000 cases in the rest of Oceania in 2013–2014, the largest outbreak prior to the Americas (2016-present) (Cao-Lormeau et al., 2016; Duffy et al., 2009). Guillian-Barré syndrome, a neurological pathology associated with Zika virus infection, was first recognized at this time (Cao-Lormeau et al., 2016). Similarly, an increase in newborn microcephaly was found to be correlated with the increase in Zika cases in Brazil in 2015 and 2016 (Schuler-Faccini et al., 2016). For this reason, in February 2016, the World Health Organization declared the American Zika virus epidemic to be a Public Health Emergency of International Concern.
Despite its public health importance, the ecology of Zika virus transmission has been poorly understood until recently. It has been presumed that Aedes aegypti and Ae. albopictus are the primary vectors due to epidemiologic association with Zika virus (Messina et al., 2016), viral isolation from and transmission experiments with field populations (especially in Ae. aegypti [Haddow et al., 2012; Boorman and Porterfield, 1956; Haddow et al., 1964]), and association with related arboviruses (e.g. dengue fever virus, yellow fever virus). Predictions of the potential geographic range of Zika virus in the United States,and associated estimates for the size of the vulnerable population, are therefore primarily based on the distributions of Ae. aegypti and Ae. albopictus, which jointly extend across the Southwest, Gulf coast, and mid-Atlantic regions of the United States (Centers for Disease Control and Prevention, 2016). We reasoned, however, that if other, presently unidentified Zika-competent mosquitoes exist in the Americas, then these projections may be too restricted and therefore optimistically biased. Additionally, recent experimental studies show that the ability of Ae. aegypti and Ae. albopictus to transmit the virus varies significantly across mosquito populations and geographic regions (Chouin-Carneiro et al., 2016), with some populations exhibiting low dissemination rates even though the initial viral titer after inoculation may be high (Diagne et al., 2015). This suggests that in some locations other species may be involved in transmission. The outbreak on Yap, for example, was driven by a different species, Ae. hensilli (Ledermann et al., 2014). Closely related viruses of the Flaviviridae family are vectored by over nine mosquito species, on average (see Supplementary Data). Thus, because Zika virus may be associated with multiple mosquito species, we considered it necessary to develop a more comprehensive list of potential Zika vectors.
The gold standard for identifying competent disease vectors requires isolating virus from field-collected mosquitoes, followed by experimental inoculation and laboratory investigation of viral dissemination throughout the body and to the salivary glands (Barnett, 1960; Hardy et al., 1983), and, when possible, successful transmission back to the vertebrate host (e.g. Komar et al., 2003). Unfortunately, these methods are costly, often underestimate the risk of transmission (Bustamante and Lord, 2010), and the amount of time required for analyses can delay decision making during an outbreak (Day, 2001). To address the problem of identifying potential vector candidates in an actionable time frame, we therefore pursued a data-driven approach to identifying candidate vectors aided by machine learning algorithms for identifying patterns in high dimensional data. If the propensity of mosquito species to associate with Zika virus is statistically associated with common mosquito traits, it is possible to rank mosquito species by the degree of risk represented by their traits – a comparative approach similar to the analysis of risk factors in epidemiology. For instance, a model could be constructed to estimate the statistical discrepancy between the traits of known vectors (i.e., Ae. aegypti, Ae. albopictus, and Ae. hensilli) and the traits of all possible vectors. Unfortunately, this simplistic approach would inevitably fail due to the small amount of available data (i.e., sample size of 3). Thus, we developed an indirect approach that leverages the information contained in the associations among many virus-mosquito pairs to inform us about specific associations. Specifically, our method identifies covariates associated with the propensity for mosquito species to vector any flavivirus. From this, we constructed a model of the mosquito-flavivirus network and then extracted from this model the life history profile and species list of mosquitoes predicted to associate with Zika virus, which we recommend be experimentally tested for Zika virus competence.
Results
In total, we identified 132 vector-virus pairs, consisting of 77 mosquito species and 37 flaviviruses. The majority of these species were Aedes (32) or Culex (24) species. Our supplementary dataset consisted of an additional 103 mosquito species suspected to transmit flaviviruses, but for which evidence of a full transmission cycle does not exist. This resulted in 180 potential mosquito-Zika pairs on which to predict with our trained model. As expected, closely related viruses, such as the four strains of dengue, shared many of the same vectors and were clustered in our network diagram (Figure 1). The distribution of vectors to viruses was uneven, with a few viruses vectored by many mosquito species, and rarer viruses vectored by only one or two species. The virus with the most known competent vectors was West Nile virus (31 mosquito vectors), followed by yellow fever virus (24 mosquito vectors). In general, encephalitic viruses such as West Nile virus were found to be more commonly vectored by Culex mosquitoes and hemorrhagic viruses were found to be more commonly vectored by Aedes mosquitoes (see Gould and Solomon (2008) for further distinctions within Flaviviridae) (Figure 1).
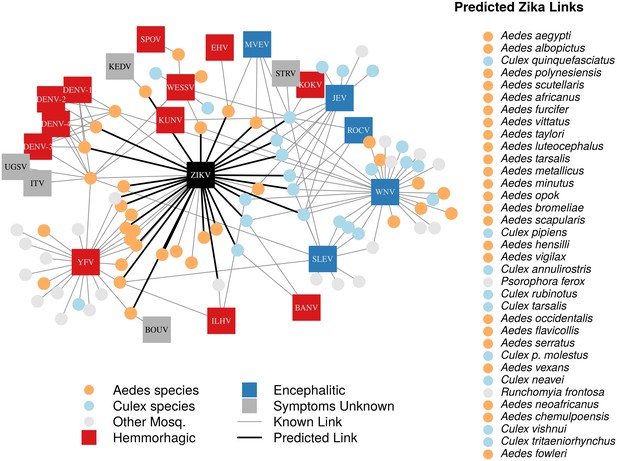
A network diagram of mosquito vectors (circles) and their flavivirus pairs (rectangles).
The Culex mosquitoes (light blue) and primarily encephalitic viruses (blue) are more clustered than the Aedes (orange) and hemmorhagic viruses (red). Notably, West Nile Virus is vectored by both Aedes and Culex species. Predicted vectors of Zika are shown by bolded links in black. The inset shows predicted vectors of Zika and species names, ordered by the model’s propensity scores. Included flaviviruses are Banzi virus (BANV), Bouboui virus (BOUV), dengue virus strains 1, 2, 3 and 4 (DENV-1,2,3,4), Edge Hill virus (EHV), Ilheus virus (ILHV), Israel turkey meningoencephalomyelitis virus (ITV), Japanese encephalitis virus (JEV), Kedougou virus (KEDV), Kokobera virus (KOKV), Kunjin virus (KUNV), Murray Valley encephalitis virus (MVEV), Rocio virus (ROCV), St. Louis encephalitis virus (SLEV), Spondwendi virus (SPOV), Stratford virus (STRV), Uganda S virus (UGSV), Wesselsbron virus (WESSV), West Nile Virus (WNV), yellow fever virus (YFV), and Zika virus (ZIKV).
Our ensemble of BRT models trained on common vector and virus traits predicted mosquito vector-virus pairs in the test dataset with high accuracy (AUC = 0.92 ± 0.02; sensitivity = 0.858 ± 0.04; specificity = 0.872 ± 0.04). Due to non-monotonicity and existence of interactions among predictor variables within our model, one cannot make general statements about the directionality of effect. Thus, we focus on the relative importance of different variables to model performance. The most important variable for accurately predicting the presence of vector-virus pair was the subgenus of the mosquito species, followed by continental range (e.g. continents on which species are present). The number of viruses vectored by a mosquito species and number of mosquito vectors of a virus were the third and fifth most important variables, respectively. Unsurprisingly, this suggests that, when controlling for other variables, mosquitoes and viruses with more known vector-virus pairs (i.e., more viruses vectored and more hosts infected, respectively), are more likely to be part of a predicted pair by the model. Mosquito ecological traits such as larval habitat and salinity tolerance were generally less important than a species’ phylogeny or geographic range (Figure 2).
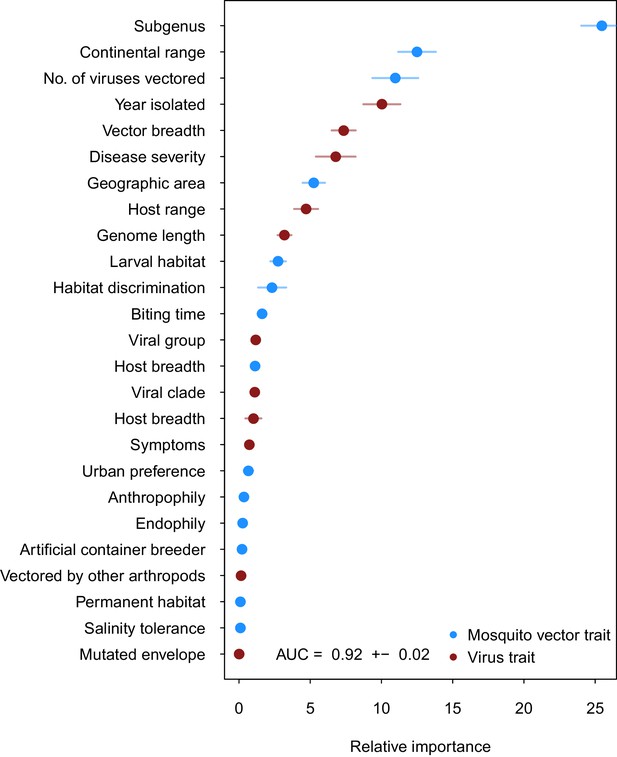
Variable importance by permutation, averaged over 25 models.
Because some categorical variables were treated as binary by our model (i.e. continental range), the relative importance of each binary variable was summed to result in the overall importance of the categorical variable. Mosquito and virus traits are shown in blue and maroon, respectively. Error bars represent the standard error from 25 models.
When applied to the 180 potential mosquito-Zika pairs, the model predicted thirty-five vectors to be ranked above the threshold (set at the value of the lowest-ranked known vector), for a total of nine known vectors and twenty-six novel, predicted mosquito vectors of Zika (Table 1). Of these vectors, there were twenty-four Aedes species, nine Culex species, one Psorophora species, and one Runchomyia species. The GBM model’s top two ranked vectors for Zika are the most highly-suspected vectors of Zika virus, Ae. aegypti and Ae. albopictus.
Model validation
Our supplementary and primary models generally concur and their ranking of potential Zika virus vectors are highly correlated (ρ = 0.508 and ρ = 0.693 on raw and thresholded predictions, respectively). As one might expect, the supplementary model assigned fewer scores of low propensity (Appendix 1—figure 2), suggesting that incorporating this additional uncertainty in the training dataset eroded the model's ability to distinguish negative links. The supplementary model’s performance on the testing data (AUC = 0.84 ± 0.02), however, indicates that the additional uncertainty did not impede model performance.
When trained on ‘leave-one-out’ datasets, all three models were able to predict the testing data with high accuracy (AUC = 0.91, AUC = 0.91, AUC = 0.92 for West Nile, dengue, and yellow fever viruses, respectively). Performance varied when models were validated against predictions of ‘known outcomes’. A model trained without West Nile virus predicted highly linked vectors reasonably well (AUC = 0.69), however it assigned low scores to rarer ‘known’ vectors, such as Culiseta inornata, which was only associated with West Nile virus. Similarly, the model trained on the dengue-omitted dataset predicted training data and vectors of dengue itself with high accuracy (AUC = 0.92). While the model trained without yellow fever performed well on the testing data, it performed poorly when predicting vectors of yellow fever virus (AUC = 0.47). Unlike West Nile and dengue viruses, the majority of the known vectors of yellow fever are only associated with yellow fever (i.e. a single vector-virus link), and so were excluded completely from the training data when all yellow fever links were omitted. Additionally, several of the vector species are of the Haemagogus genus, which was completely absent from the training data. Given the importance of phylogeny of the vector species in predicting vector-virus links, it follows that a dataset with a novel subgenus would be difficult for the model to predict on, resulting in low model performance. The low performance of this model illustrates that incorporating common traits and additional vector-virus links improves model prediction. When traits were not available in the training dataset, model performance was much lower, suggesting that there exists a statistical association between a vectors’ traits and its ability to transmit a virus.
Discussion
Zika virus is unprecedented among emerging arboviruses in its combination of severe public health hazard, rapid spread, and poor scientific understanding. Particularly crucial to public health preparedness is knowledge about the geographic extent of potentially at risk populations and local environmental conditions for transmission, which are determined by the presence of competent vectors. Until now, identifying additional competent vector species has been a low priority because Zika virus has historically been geographically restricted to a narrow region of equatorial Africa and Asia (Petersen et al., 2016), and the mild symptoms of infection made its range expansion since the 1950’s relatively unremarkable. However, with its relatively recent and rapid expansion into the Americas and its association with severe neurological disorders, the prediction of potential disease vectors in non-endemic areas has become a matter of critical public health importance. We identify these potential vector species by developing a data-driven model that identifies candidate vector species of Zika virus by leveraging data on traits of mosquito vectors and their flaviviruses. We suggest that empirical work should prioritize these species in their evaluation of vector competence of mosquitoes for Zika virus.
Our model predicts that fewer than one third of the potential mosquito vectors of Zika virus have been identified, with over twenty-five additional mosquito species worldwide that may have the capacity to contribute to transmission. The continuing focus in the published literature on two species known to transmit Zika virus (Ae. aegypti and Ae. albopictus) ignores the potential role of other vectors, potentially misrepresenting the spatial extent of risk. In particular, four species predicted by our model to be competent vectors – Ae. vexans, Culex quinquefasciatus, Cx. pipiens, and Cx. tarsalis – are found throughout the continental United States. Further, the three Culex species are primary vectors of West Nile virus (Farajollahi et al., 2011). Cx. quinquefasciatus and Cx. pipiens were ranked 3rd and 17th by our model, respectively, and together these species were the highest-ranking species endemic to the United States after the known vectors (Ae. aegypti and Ae. albopictus). Cx. quinquefasciatus has previously been implicated as an important vector of encephalitic flaviviruses, specifically West Nile virus and St. Louis encephalitis (Turell et al., 2005; Hayes et al., 2005), and a hybridization of the species with Cx. pipiens readily bites humans (Fonseca et al., 2004). The empirical data available on the vector competence of Cx. pipiens and Cx. quinquefasciatus is currently mixed, with some studies finding evidence for virus transmission and others not (Guo et al., 2016; Aliota et al., 2016; Fernandes et al., 2016; Huang et al., 2016). These results suggest, in combination with evidence for significant genotype x genotype effects on the vector competence of Ae. aegypti and Ae. albopictus to transmit Zika (Chouin-Carneiro et al., 2016), that the vector competence of Cx. pipiens and Cx. quinquefasciatus for Zika virus could be highly dependent upon the genetic background of the mosquito-virus pairing, as well as local environmental conditions. Thus, considering their anthropophilic natures and wide geographic ranges, Cx. quinquefasciatus and Cx. pipiens could potentially play a larger role in the transmission of Zika in the continental United States. Further experimental research into the competence of populations of Cx. pipiens to transmit Zika virus across a wider geographic range is therefore highly recommended, and should be prioritized.
The vectors predicted by our model have a combined geographic range much larger than that of the currently suspected vectors of Zika (Figure 3), suggesting that, were these species to be confirmed as vectors, a larger population may be at risk of Zika infection than depicted by maps focusing solely on Ae. aegypti and Ae. albopictus. The range of Cx. pipiens includes the Pacific Northwest and the upper mid-West, areas that are not within the known range of Ae. aegypti or Ae. albopictus (Darsie and Ward, 2005). Furthermore, Ae. vexans, another predicted vector of Zika virus, is found throughout the continental US and the range of Cx. tarsalis extends along the entire West coast (Darsie and Ward, 2005). On a finer scale, these species use a more diverse set of habitats, with Ae. aegypti and Cx. quinquefasciatus mainly breeding in artificial containers, and Ae. vexans and Ae. albopictus being relatively indiscriminate in their breeding sites, including breeding in natural sites such as tree holes and swamps. Therefore, in addition to the wider geographic region supporting potential vectors, these findings suggest that both rural and urban areas could serve as habitat for potential vectors of Zika. We recommend experimental tests of these species for competency to transmit Zika virus, because a confirmation of these vectors would necessitate expanding public health efforts to these areas not currently considered at risk.
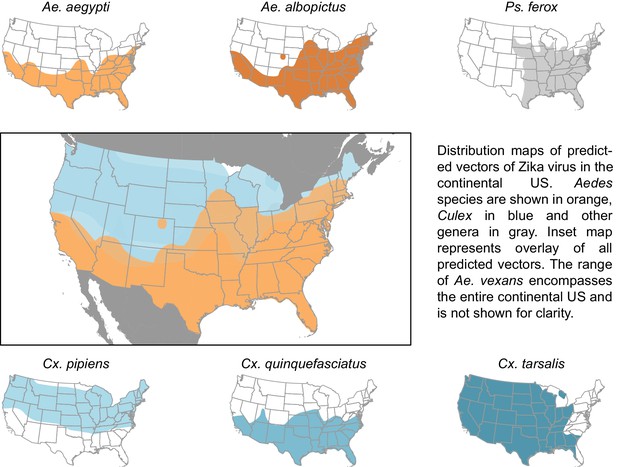
Distribution maps of predicted vectors of Zika virus in the continental US.
Maps of Aedes species are based on Centers for disease control and prevention (2016). All other species’ distributions are georectified maps from Darsie and Ward (2005).
While transmission requires a competent vector, vector competence does not necessarily equal transmission risk or inform vectorial capacity. There are many biological factors that, in conjunction with positive vector competence, determine a vector’s role in disease transmission. For example, although Ae. aegypti mosquitoes are efficient vectors of West Nile virus, they prefer to feed on humans, which are dead-head hosts for the disease, and therefore have low potential to serve as a vector (Turell et al., 2005). Psorophora ferox, although predicted by our model as a potential vector of Zika virus, would likely play a limited role in transmission because it rarely feeds on humans (Molaei et al., 2008). Additionally, vector competence is dynamic, and may be mediated by environmental factors that influence viral development and mosquito immunity (Muturi and Alto, 2011). Therefore, our list of potential vectors of Zika represents a comprehensive starting point, which should be furthered narrowed by empirical work and consideration of biological details that impact transmission dynamics. Given the severe neurological side-effects of Zika virus infection, beginning with the most conservative method of vector prediction ensures that risk is not underestimated, and allows public health agencies to interpret the possibility of Zika transmission given local conditions.
Our model serves as a starting point to streamlining empirical efforts to identify areas and populations at risk for Zika transmission. While our model enables data-driven predictions about the geographic area at potential risk of Zika transmission, subsequent empirical work investigating Zika vector competence and transmission efficiency is required for model validation, and to inform future analyses of transmission dynamics. For example, in spite of its low transmission efficiency in certain geographic regions (Chouin-Carneiro et al., 2016), Ae. aegypti is anthropophilic (Powell and Tabachnick, 2013), and may therefore pose a greater risk of human-to-human Zika virus transmission than mosquitoes that bite a wider variety of animals. On the other hand, mosquito species that prefer certain hosts in rural environments are known to alter their feeding behaviors to bite alternative hosts (e.g., humans and rodents) in urban settings, due to changes in host community composition (Chaves et al., 2010). Environmental factors such as precipitation and temperature directly influence mosquito populations, and determine the density of vectors in a given area (Thomson et al., 2006), an important factor in transmission risk. Additionally, socio-economic factors such as housing type and lifestyle can decrease a populations’ contact with mosquito vectors, and lower the risk of transmission to humans (Moreno-Madriñán and Turell, 2017). Effective risk modeling and forecasting the range expansion of Zika virus in the United States will depend on validating the vector status of these species, as well as resolving behavioral and biological details that impact transmission dynamics.
Although we developed this model with Zika virus in mind, our findings have implications for other emerging flaviviruses and contribute to the recently developed methodology applying machine learning methods to the prediction of unknown agents of infectious diseases. This technique has been used to predict rodent reservoirs of disease (Han et al., 2015) and bat carriers of filoviruses (Han et al., 2016) by training models with host-specific data. Our model, however, incorporates additional data by constructing a vector-virus network that is used to inform predictions of vector-virus associations. The combination of common virus traits with vector-specific traits enabled us to predict potential mosquito vectors of specific flaviviruses, and to train the model on additional information distributed throughout the flavivirus-mosquito network.
Uncertainty in our model arises through uncertainty inherent in our datasets. Vector status is not static (e.g. mutation in the chikungunya virus to increase transmission by Ae. albopictus [Weaver and Forrester, 2015]) and can vary across vector populations (Bennett et al., 2002). When incorporating uncertainty in vector status through our supplementary model, our predictions generally agreed with that of our original model. However, the increased uncertainty did reduce the models’ ability to distinguish negative links, resulting in higher uncertainty in propensity scores (as measured by standard deviation) and a larger number of predicted vectors. Additionally, the model performs poorly when predicting on vector-virus links with trait levels not included in the training data set, as was the case when omitting yellow fever virus. Another source of uncertainty is regarding vector and virus traits. In addition to intraspecific variation in biological traits, many vectors are understudied, and common traits such as biting activity are unknown to the level of species. Additional study into the behavior and biology of less common vector species would increase the accuracy of prediction techniques such as this, and allow for a better of understanding of species’ potential role as vectors.
Interestingly, our constructed flavivirus-mosquito network generally concurs with the proposed dichotomy of Aedes species vectoring hemorrhagic or febrile arboviruses and Culex species vectoring neurological or encephalitic viruses (Grard et al., 2010) (Figure 1). However, there are several exceptions to this trend, notably West Nile virus, which is vectored by several Aedes species. Additionally, our model predicts several Culex species to be possible vectors of Zika virus. While this may initially seem contrary to the common phylogenetic pairing of vectors and viruses noted above, Zika’s symptoms, like West Nile virus, are both febrile and neurological. Thus, its symptoms do not follow the conventional hemorrhagic/encephalitic division. The ability of Zika virus to be vectored by a diversity of mosquito vectors could have important public health consequences, as it may expand both the geographic range and seasonal transmission risk of Zika virus, and warrants further empirical investigation.
Considering our predictions of potential vector species and their combined ranges, species on the candidate vector list need to be validated to inform the response to Zika virus. Vector control efforts that target Aedes species exclusively may ultimately be unsuccessful in controlling transmission of Zika because they do not control other, unknown vectors. For example, the release of genetically modified Ae. aegypti to control vector density through sterile insect technique is species-specific and would not control alternative vectors (Alphey et al., 2010). Additionally, species’ habitat preferences differ, and control efforts based singularly on reducing Aedes larval habitat will not be as successful at controlling Cx. quinquefasciatus populations (Rey et al., 2006). Predicted vectors of Zika virus must be empirically tested and, if confirmed, vector control efforts would need to respond by widening their focus to control the abundance of all predicted vectors of Zika virus. Similarly, if control efforts are to include all areas at potential risk of disease transmission, public health efforts would need to expand to address regions such as the northern Midwest that fall within the range of the additional vector species predicted by our model. An understanding of the capacity of mosquito species to vector Zika virus is necessary to prepare for the potential establishment of Zika virus in the United States, and we recommend that experimental work start with this list of candidate vector species.
Materials and methods
Data collection and feature construction
Request a detailed protocolOur dataset comprised a matrix of vector-virus pairs relating all known flaviviruses and their mosquito vectors. To construct this matrix, we first compiled a list of mosquito-borne flaviviruses to include in our study (Van Regenmortel et al., 2000; Kuno et al., 1998; Cook and Holmes, 2006). Viruses that only infect mosquitoes and are not known to infect humans were not included. Using this list, we constructed a mosquito-virus pair matrix based on the Global Infectious Diseases and Epidemiology Network database (GIDEON, 2016), the International Catalog of Arboviruses Including Certain Other Viruses of Vertebrates (ArboCat) (Karabatsos, 1985), The Encyclopedia of Medical and Veterinary Entomology (Russell et al., 2013)and Mackenzie et al. (2012).
We defined a known vector-virus pair as one for which the full transmission cycle (i.e, infection of mosquito via an infected host (mammal or avian) or bloodmeal that is able to be transmitted via saliva) has been observed. Basing vector competence on isolation or intrathoracic injection bypasses several important barriers to transmission (Hardy et al., 1983), and may not be true evidence of a mosquito’s ability to transmit an arbovirus. We found our definition to be more conservative than that which is commonly used in disease databases (e.g. Global Infectious Diseases and Epidemiology Network database), which often assumes isolation from wild-caught mosquitoes to be evidence of a mosquito’s role as a vector. Therefore, a supplementary analysis investigates the robustness of our findings with regards to uncertainty in vector status by comparing the analysis reported in the main text to a second analysis in which any kind of evidence for association, including merely isolating the virus in wild-caught mosquitoes, is taken as a basis for connection in the virus-vector network (see Appendix 1 for analysis and results).
Fifteen mosquito traits (Appendix 2—table 1) and twelve virus traits (Appendix 2—table 2) were collected from the literature. For the mosquito species, the geographic range was defined as the number of countries in which the species has been collected, based on Walter Reed Biosystematics Unit, (2016). While there are uncertainties in species’ ranges due to false absences, this represents the most comprehensive, standardized dataset available that includes both rare and common mosquito species. A species’ continental extent was recorded as a binary value of its presence by continent. A species’ host range was defined as the number of taxonomic classes the species is known to feed on, with the Mammalia class further split into non-human primates and other mammals, because of the important role primates play in zoonotic spillovers of vector-borne disease (e.g. dengue, chikungunya, yellow fever, and Zika viruses) (Weaver, 2005; Diallo et al., 2005; Weaver et al., 2016). The total number of unique flaviviruses observed per mosquito species was calculated from our mosquito-flavivirus matrix. All other traits were based on consensus in the literature (see Appendix III for sources by species). For three traits – urban preference, endophily (a proclivity to bite indoors), and salinity tolerance – if evidence of that trait for a mosquito was not found in the literature, it was assumed to be negative.
Predicted vectors of Zika virus, as reported by our model. Mosquito species endemic to the continental United States are bolded. A species is defined as a known vector of Zika virus if a full transmission cycle (see main text) has been observed.
Species | GBM prediction SD | Known vector? |
---|---|---|
Aedes aegypti | 0.81 ± 0.12 | Yes |
Ae. albopictus | 0.54 ± 0.14 | Yes |
Culex quinquefasciatus | 0.38 ± 0.14 | No |
Ae. polynesiensis | 0.36 ± 0.13 | No |
Ae. scutellaris | 0.33 ± 0.13 | No |
Ae. africanus | 0.32 ± 0.11 | No |
Ae. furcifer | 0.31 ± 0.16 | Yes |
Ae. vittatus | 0.30 ± 0.20 | Yes |
Ae. taylori | 0.30 ± 0.16 | Yes |
Ae. luteocephalus | 0.25 ± 0.12 | Yes |
Ae. tarsalis | 0.18 ± 0.11 | Yes |
Ae. metallicus | 0.16 ± 0.08 | No |
Ae. minutus | 0.16 ±0.09 | No |
Ae. opok | 0.14 ± 0.06 | No |
Ae. bromeliae | 0.11 ± 0.06 | No |
Ae. scapularis | 0.10 ± 0.04 | No |
Cx. pipiens | 0.10 ± 0.04 | No |
Ae. hensilli | 0.10 ± 0.06 | Yes |
Ae. vigilax | 0.10 ± 0.05 | No |
Cx. annulirostrix | 0.08 ± 0.03 | No |
Psorophora ferox | 0.08 ± 0.05 | No |
Cx. rubinotus | 0.08 ± 0.07 | No |
Cx. tarsalis | 0.08 ± 0.03 | No |
Ae. occidentalis | 0.08 ± 0.05 | No |
Ae. flavicolis | 0.07 ± 0.04 | No |
Ae. serratus | 0.07 ± 0.04 | No |
Cx. p. molestus | 0.07 ± 0.04 | No |
Ae. vexans | 0.06 ± 0.04 | No |
Cx. neavei | 0.06 ± 0.02 | No |
Runchomyia frontosa | 0.06 ± 0.04 | No |
Ae. neoafricanus | 0.06 ± 0.03 | No |
Ae. chemulpoensis | 0.06 ± 0.03 | No |
Cx. vishnui | 0.05 ± 0.01 | No |
Cx. tritaeniorhynchus | 0.05 ± 0.01 | No |
Ae. fowleri | 0.04 ± 0.03 | Yes |
We collected data on the following virus traits: host range (Mahy, 2009; Mackenzie et al., 2012; Chambers and Monath, 2003; Cook and Zumla, 2009b), disease severity (Mackenzie et al., 2012), human illness (Chambers and Monath, 2003; Cook and Zumla, 2009), the presence of a mutated envelope protein, which controls viral entry into cells (Grard et al., 2010), year of isolation (Karabatsos, 1985), and host range (Karabatsos, 1985). Disease severity was based on Mackenzie et al. (2012), ranging from no known symptoms (e.g. Kunjin virus) to severe symptoms and significant human mortality (e.g. yellow fever virus). For each virus, vector range was calculated as the number of mosquito species for which the full transmission cycle has been observed. Genome length was calculated as the mean of all complete genome sequences listed for each flavivirus in the Virus Pathogen Database and Analysis Resource (http://www.viprbrc.org/). For more recently discovered flaviviruses not yet cataloged in the above databases (i.e., New Mapoon Virus, Iquape virus), viral traits were gathered from the primary literature (sources listed in Appendix 3).
Predictive model
Request a detailed protocolFollowing Han et al. (2015), boosted regression trees (BRT) (Friedman, 2001) were used to fit a logistic-like predictive model relating the status of all possible virus-vector pairs (0: not associated, 1: associated) to a predictor matrix comprising the traits of the mosquito and virus traits in each pair. Boosted regression trees circumvent many issues associated with traditional regression analysis (Elith et al., 2008), allowing for complex variable interactions, collinearity, non-linear relationships between covariates and response variables, and missing data. Additionally, this technique performs well in comparison with other logistic regression approaches (Friedman, 2001). Trained boosted regression tree models are dependent on the split between training and testing data, such that each model might predict slightly different propensity values. To address this, we trained an ensemble of 25 internally cross-validated BRT models on independent partitions of training and testing data. The resulting model demonstrated low variance in relative variable importance and overall model accuracy, suggesting models all converged to a similar result.
Prior to the analysis of each model, we randomly split the data into training (70%) and test (30%) sets while preserving the proportion of positive labels (known associations) in each of the training and test sets. Models were trained using the gbm package in (Ridgeway, 2015), with the maximum number of trees set to 25,000, a learning rate of 0.001, and an interaction depth of 5. To correct for optimistic bias (Smith et al., 2014), we performed 10-fold cross validation and chose a bag fraction of 50% of the training data for each iteration of the model. We estimated the performance of each individual model with three metrics: Area Under the Receiver Operator Curve, specificity, and sensitivity. For specificity and sensitivity, which require a preset threshold, we thresholded predictions on the testing data based on the value which maximized the sum of the sensitivity and specificity, a threshold robust to the ratio of presence to background points in presence-only datasets (Liu et al., 2016). Variable importance was quantified by permutation (Breiman, 2001) to assess the relative contribution of virus and vector traits to the propensity for a virus and vector to form a pair. Because we transformed many categorical variables into binary variables (e.g., continental range as binary presence or absence by continent), the sum of the relative importance for each binary feature was summed to obtain a single value for the entire variable.
Each of our twenty-five trained models was then used to predict novel mosquito vectors of Zika by applying the trained model to a data set consisting of the virus traits of Zika paired with the traits of all mosquitoes for which flaviviruses have been isolated from wild caught individuals, and, depending on the species, may or may not have been tested in full transmission cycle experiments (a total of 180 mosquito species). This expanded dataset allowed us to predict over a large number of mosquito species, while reasonably limiting our dataset to those species suspected of transmitting flaviviruses. The output of this model was a propensity score ranging from 0 to 1. In our case, the final propensity score for each vector was the mean propensity score assigned by the twenty-five models. To label unobserved edges, we thresholded propensity scores at the value of lowest ranked known vector (Liu et al., 2013).
Model validation
Request a detailed protocolIn addition to conventional performance metrics, we conducted additional analyses to further validate both this method of prediction, and our model specifically. To account for uncertainty in the vector-virus links in our initial matrix, we repeated our analysis for a vector-virus matrix with a less conservative definition of a positive link (field isolation and above), referred to as our supplementary model. Vector competence is a dynamic trait, and there exists significant intraspecific variation in the ability of a vector to transmit a virus for certain species of mosquitoes (Diallo et al., 2005; Gubler et al., 1979). Our supplementary model is based on a less conservative definition of vector competence and includes species implicated as vectors, but not yet verified through laboratory competence studies, and therefore accounts for additional uncertainty such as intraspecific variation.
While this approach is well-tested in epidemiological applications (Parascandola, 2004), it has only recently been applied to predict ecological associations, and, as such, has limitations unique to this application. To further evaluate this prediction method, we performed a modified ‘leave-one-out’ analysis, whereby we trained a model to a dataset from which a well-studied virus had been omitted, and then predicted vectors for this virus and compared them against a list of known vectors. We repeated this analysis for West Nile, dengue, and yellow fever viruses, following the same method of training as for our original model. While this analysis differs from our original method, it provides a more stringent evaluation of this method of prediction because the model is trained on an incomplete dataset and predicts on unfamiliar data, a more difficult task than that posed to our original model.
Appendix 1
Comparison model trained on virus isolation data
The primary model is trained on vector-virus pairs for which the full transmission cycle has been observed. However, many sources, such as the Global Infectious Diseases and Epidemiology Network database (GIDEON), interpret isolation of a virus in wild-caught mosquitoes as evidence of a mosquito’s role as a vector. In order to investigate the robustness of our findings, we conducted a supplementary analysis in which any evidence for association, including isolation of the virus, is used as the basis for a link in the vector-virus network.
Data collection
As in the primary model, the mosquito-virus pair matrix was constructed based on the Global Infectious Diseases and Epidemiology Network database (GIDEON, 2016), the International Catalog of Arboviruses Including Certain Other Viruses of Vertebrates (ArboCat) (Karabatsos, 1985), The Encyclopedia of Medical and Veterinary Entomology (Russell et al., 2013) and (Mackenzie et al., 2012). This resulted in a dataset containing 180 mosquito species and 37 viruses, for a total of 334 vector-virus pairs. The vector and virus trait datasets were identical to those used in the primary model (see Appendix 2 for lists of traits).
Predictive model
We used boosted regression trees (Friedman, 2001) to fit a logistic-like predictive model relating the status of all possible virus-vector pairs (0: not associated, 1:associated) to a predictor matrix comprising the traits of the mosquito and virus traits in each pair. We fit a total of 25 models, applying different training and testing datasets to each, to reduce the dependence dependent on the split between training and testing data. Prior to the analysis of each model, we randomly split the data into training (70%) and test (30%) sets while preserving the proportion of positive labels in each of the training and test sets. Models were trained using the gbm package in (Ridgeway, 2015), with the maximum number of trees set to 25,000 and a learning rate of 0.001. To correct for optimistic bias (Smith et al., 2014), we performed 10-fold cross validation and bagged 50% of the training data for each iteration of the model. These methods are identical to those used to train the primary model. We quantified variable importance by permutation (Breiman, 2001) to assess the relative contribution of virus and vector traits to the propensity for a virus and vector to form a pair.Each of our twenty-five trained models was then used to predict novel mosquito vectors of Zika over the whole virus-vector pair dataset, resulting in twenty-five propensity values assigned to each mosquito species, of which we took the mean. Our prediction dataset, therefore, consisted of the common virus traits of Zika paired with the common traits of all mosquitoes in our flavivirus dataset, for a total of 180 species. The output of this model was a propensity score ranging from 0 to 1. In our case, the final propensity score for each vector was the mean propensity score assigned by the twenty-five models. To label unobserved edges, we thresholded propensity at the value of lowest ranked known vector (Liu et al., 2013).
Results
Boosted regression models trained on the weakest evidence of association accurately predicted mosquito vector-virus associations in the test dataset (AUC= 0.84 ±0.02). When thresholded at the value of the lowest ranked known vector, the model predicted 66 potential vectors of ZIKV, including 42 unknown vectors LABEL:table:predictions. The majority of predicted vectors were Aedes species (39 species), with Culex as the second most predicted genus (15 species). It included all but three of the vectors predicted by the main model (Ae. occidentalis, Ru. frontosa, Cx. rubinotus).
Model comparisons
Our supplementary and primary models, trained on virus isolation and above and full transmission cycle, respectively, generally concur. The models are fairly correlated (Spearman’s coefficient, ρ=0.508 when considering the propensities of all 180 species 1. However, when only comparing the correlation of propensities between those vectors above the threshold of lowest ranked known vector, the models become much more correlated (ρ=0.693). This suggests that our model has a higher sensitivity than specificity, and is better able to predict those vectors that are competent for ZIKV than those that are not. The predictive accuracy of our supplementary model was slightly lower than our primary model. However, this may be an indirect effect of a lower positive-negative label ratio in the dataset used in the primary model, which can artificially inflate AUC values (Lobo et al., 2008).
The models differ in their ability to differentiate between vectors and non-vectors. The distribution of propensities for our main model is more skewed towards lower propensity values than is the supplementary model 2. This is logical, as the dataset used to train the main model contains a higher proportion of zeros (e.g. vector-virus pairs with no known association) than the supplementary model. The difference in distributions is accounted for by a similar discrepancy in threshold propensity values based on the lowest ranked known vector. The main model, which has a higher frequency of near-zero propensities, uses a lower threshold value than the supplementary model, however both thresholds qualitatively lie above the majority of the distributions.
Conclusion
In summary, our supplementary model predicts which mosquito species may test positive for ZIKV through isolation in wild-caught individuals. As isolation can be understood as evidence of a vector’s role in transmission of a disease, our supplementary model may also be interpreted as a ranking of potential vectors of ZIKV, similar to our main model. In fact, both models are well correlated in their ranking of species, although the main model, which trains on fewer vector-virus links, predicts fewer vectors than the supplementary model. Those species predicted by both models, such as Cx. quinquefasciatus and Ae. vexans, should be prioritized for further research on their competency to transmit ZIKV. Furthermore, as suggested by the main model, the current geographic range at risk for ZIKV transmission in the United States should be expanded to include the range of these species ranked highly by both our main and supplementary models.
Vector predictions by the supplementary model.
Vector | GBM Prediction | SD |
---|---|---|
Aedes aegypti | 0.84 | 0.06 |
Aedes albopictus | 0.81 | 0.07 |
Aedes vittatus | 0.76 | 0.10 |
Aedes africanus | 0.70 | 0.11 |
Aedes taylori | 0.65 | 0.14 |
Aedes furcifer | 0.65 | 0.14 |
Aedes luteocephalus | 0.59 | 0.12 |
Aedes metallicus | 0.59 | 0.13 |
Aedes opok | 0.58 | 0.13 |
Culex quinquefasciatus | 0.56 | 0.13 |
Aedes tarsalis | 0.56 | 0.12 |
Aedes scutellaris | 0.56 | 0.11 |
Aedes minutus | 0.55 | 0.12 |
Aedes polynesiensis | 0.53 | 0.11 |
Mansonia uniformis | 0.52 | 0.12 |
Aedes fowleri | 0.48 | 0.14 |
Aedes vexans | 0.46 | 0.11 |
Aedes dalzieli | 0.45 | 0.13 |
Culex annulirostris | 0.45 | 0.08 |
Mansonia africana | 0.42 | 0.12 |
Psorophora ferox | 0.39 | 0.14 |
Culex tarsalis | 0.38 | 0.09 |
Culex tritaeniorhynchus | 0.37 | 0.08 |
Culex pipiens | 0.37 | 0.13 |
Culex neavei | 0.34 | 0.06 |
Aedes vigilax | 0.34 | 0.07 |
Aedes flavicollis | 0.33 | 0.14 |
Aedes scapularis | 0.31 | 0.07 |
Aedes taeniarostris | 0.31 | 0.13 |
Aedes jamoti | 0.31 | 0.13 |
Aedes circumluteolus | 0.30 | 0.13 |
Eretmapodites inornatus | 0.30 | 0.15 |
Aedes cumminsii | 0.29 | 0.11 |
Culex vishnui | 0.28 | 0.05 |
Aedes lineatopennis | 0.28 | 0.11 |
Aedes neoafricanus | 0.27 | 0.11 |
Aedes bromeliae | 0.26 | 0.10 |
Culex guiarti | 0.26 | 0.06 |
Culex perfuscus | 0.26 | 0.06 |
Aedes stokesi | 0.26 | 0.12 |
Culex telesilla | 0.25 | 0.06 |
Anopheles gambiae | 0.24 | 0.11 |
Sabethes chloropterus | 0.24 | 0.11 |
Aedes hensilli | 0.24 | 0.09 |
Aedes serratus | 0.23 | 0.06 |
Aedes chemulpoensis | 0.23 | 0.08 |
Aedes normanensis | 0.23 | 0.06 |
Culex bitaeniorhynchus | 0.22 | 0.09 |
Culex pseudovishnui | 0.22 | 0.05 |
Aedes argenteopunctatus | 0.21 | 0.06 |
Wyeomyia vanduzeei | 0.21 | 0.15 |
Culex p. molestus | 0.21 | 0.06 |
Culex salinarius | 0.20 | 0.04 |
Aedes grahami | 0.19 | 0.15 |
Anopheles coustani | 0.19 | 0.08 |
Aedes longipalpis | 0.18 | 0.18 |
Uranotaenia sapphirina | 0.17 | 0.08 |
Aedes domesticus | 0.17 | 0.06 |
Aedes abnormalis | 0.17 | 0.06 |
Aedes natronius | 0.17 | 0.06 |
Eretmapodites chrysogaster | 0.17 | 0.08 |
Aedes mcintoshi | 0.17 | 0.06 |
Aedes ochraceus | 0.16 | 0.06 |
Culex fatigans | 0.16 | 0.07 |
Anopheles amictus | 0.16 | 0.06 |
Eretmapodites quinquevittatus | 0.16 | 0.08 |
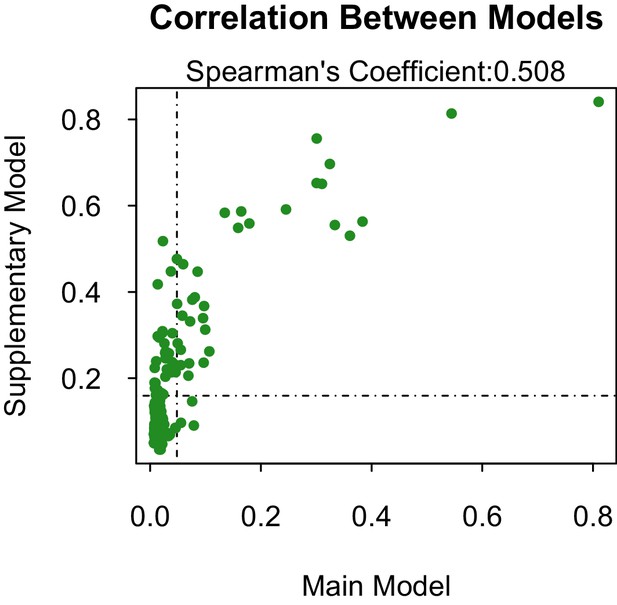
Propensity values of the main and supplementary models.
Dashed lines represent corresponding threshold values for each model based on lowest ranked known vector propensities.
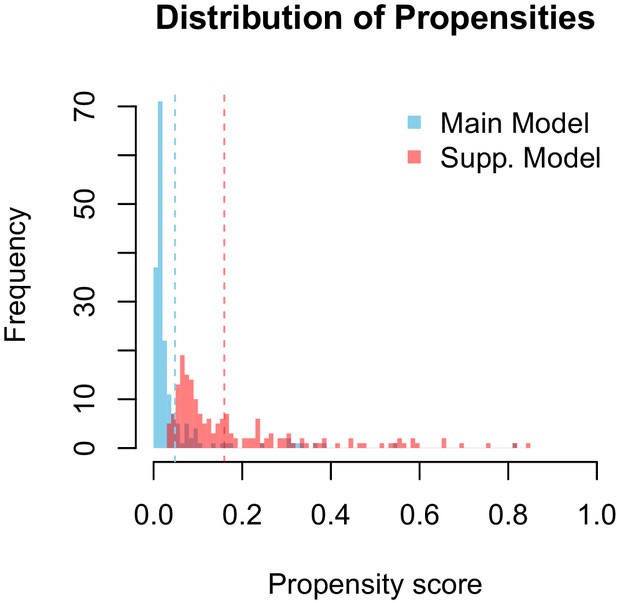
Distribution of propensity values for the main and supplementary models.
Dashed lines represent corresponding threshold values for each model based on lowest ranked known vector propensities.
Appendix 2
Tables of vector and virus traits
Table of mosquito traits used in model.
Trait | Type | Subcategories |
---|---|---|
Anthropophily | binary | NA |
Subgenus | factor | NA |
Host breadth | numeric | NA |
Host range | binary (x4) | Primate, Non-Primate Mammal, Bird, Cold-Blooded Vertebrate |
Geographic area | numeric | NA |
Continental range | binary (x8) | Africa, Middle East, Australia, Pacific, Asia, Europe, North America, South America |
Biting time | binary (x4) | Dawn, Day, Dusk, Night |
Artificia container breeder | binary | NA |
Oviposition site | binary (x8) | Treehole, Natural Container, Permanent Fresh Water, Rockhole, Marsh, Swamp, Temporary Ground Pools, Rice Paddy |
Habitat discrimination | numeric | NA |
Salinity tolerance | binary | NA |
Habitat permanence | binary | NA |
Urban preference | binary | NA |
Endophily | binary | NA |
No. of flaviviruses vectored | numeric | NA |
Table of virus traits used in model.
Trait | Type | Subcategories |
---|---|---|
Group | factor | Japanese Encephalitis, Ntaya, Yellow Fever, Aroa, Dengue, Kokobera, Spondweni |
Continental range | binary(x8) | Africa, Middle East, Australia, Pacific, Asia, Europe, North America, South America |
Clade | factor | VI, VII, IX, X, XI, XII, XIV, |
Year isolated | numeric | NA |
Mutated envelope | binary | NA |
Host breadth | numeric | NA |
Host Range | binary(x6) | Human, Non-Human Primate, Rodent, Other Mammal, Bird, Marsupial |
Mosquito vector breadth | numeric | NA |
Vectored by other arthropods | binary | NA |
Disease symptoms | binary (x2) | Encephalitis, Fever |
Disease severity | numeric | NA |
Genome length | numeric | NA |
Appendix 3
Primary sources used for vector and virus traits
Primary sources for mosquito traits.
Primary sources for virus traits.
Data availability
-
Data and Code to reproduce Evans et al 2017: "Data-driven identification of potential Zika virus vectors"Publicly accessible at figshare under a CC-BY licence ((https://figshare.com/).
References
-
Virus transmission studies with trinidadian mosquitoes II. further observationsThe American Journal of Tropical Medicine and Hygiene 8:41–45.
-
Larval habitat, ecology, seasonal abundance and vectorial role in malaria transmission of anopheles arabiensis in Jazan region of saudi arabiaJournal of the Egyptian Society of Parasitology 41:615–634.
-
Biting activity of mosquito species (Diptera: culicidae) in the Turkey-Armenia border area, ararat valley, turkeyJournal of Medical Entomology 47:22–27.https://doi.org/10.1093/jmedent/47.1.22
-
Feeding patterns of haemagogus janthinomys (Diptera: culicidae) in different regions of brazilJournal of Medical Entomology 42:981–985.https://doi.org/10.1093/jmedent/42.6.981
-
Culex pipiens and aedes triseriatus mosquito susceptibility to zika virusEmerging Infectious Diseases 22:1857–1859.https://doi.org/10.3201/eid2210.161082
-
Sterile-insect methods for control of mosquito-borne diseases: an analysisVector-Borne and Zoonotic Diseases 10:295–311.https://doi.org/10.1089/vbz.2009.0014
-
Nocturnal biting rhythms of six mosquito species (Diptera: culicidae) in Kandy, Sri LankaJournal of the National Science Council of Sri Lanka 22:279–290.
-
Ecology of the mosquito larvae in urban environments of Cairo Governorate, EgyptJournal of the Egyptian Society of Parasitology 42:191–202.https://doi.org/10.12816/0006307
-
Nocturnal activity of mosquitoes (Diptera: culicidae) in a west nile virus focus in ConnecticutJournal of Medical Entomology 44:1102–1108.https://doi.org/10.1093/jmedent/44.6.1102
-
Epidemiology of west nile virus in Connecticut: a five-year analysis of mosquito data 1999-2003Vector-Borne and Zoonotic Diseases 4:360–378.https://doi.org/10.1089/vbz.2004.4.360
-
Mosquito studies (DIPTERA, Culicidae) XXXII. a revision of the genus haemagogusContributions of the American Entomological Institute 10:1–176.
-
[The attack activity of Uranotaenia Unguiculata Edwards, 1913 mosquitoes on man]Meditsinskaia Parazitologiia I Parazitarnye Bolezni 3:39–40.
-
Proceedings of the 11th International Congress of Entomolgoy341–345, The incrimination of arthropods as vectors of disease, Proceedings of the 11th International Congress of Entomolgoy, 1962.
-
Mosquito populations diptera Culicidae of wetlands of an urban areaZeitschrift Fuer Angewandte Zoologie 70:73–90.
-
The ecology and seasonal fluctuations of mosquito larvae in a lake in Dhaka City bangladeshBangladesh Journal of Zoology 14:41–48.
-
The mosquitoes of Burnaby lake British-Columbia CanadaJournal of the Entomological Society of British Columbia 75:.
-
Variation in vector competence for dengue 2 virus among 24 collections of aedes aegypti from Mexico and the united statesThe American Journal of Tropical Medicine and Hygiene 67:85–92.
-
Handbook of ZoonosesSecond Edition: Bacterial, Rickettsial, Chlamydial, and Mycotic Zoonoses, Handbook of Zoonoses, CRC Press.
-
Host feeding patterns of culex vishnui sub group of mosquitoes in dibrugarh district of assamThe Journal of Communicable Diseases 26:133–138.
-
BookMosquitoes of Okinawa and Islands in the Central PacificUS Dept of the Navy, Bureau of Medicine and Surgery.
-
A simple technique for infection of mosquitoes with viruses; transmission of zika virusTransactions of the Royal Society of Tropical Medicine and Hygiene 50:238–242.https://doi.org/10.1016/0035-9203(56)90029-3
-
Habitat preference of host-seeking Coquillettidia perturbans (Walker) in relation to birds and eastern equine encephalomyelitis virus in new jerseyJournal of Vector Ecology : Journal of the Society for Vector Ecology 26:103–109.
-
Inventaire actualisé des moustiques (Diptera : culicidae) de l’île de La Réunion, océan IndienBulletin De La Société De Pathologie Exotique 106:113–125.https://doi.org/10.1007/s13149-013-0288-7
-
Distribution of resting female aedes vexans (Meigen) in wooded and nonwooded areas of metropolitan Minneapolis-St. Paul, MinnesotaJournal of the American Mosquito Control Association 15:128–132.
-
BookEmerging Zoonoses: Eco-Epidemiology, Involved Mechanisms and Public Health ImplicationsFrontiers Media SA.
-
BookMosquitoes of the Southeastern United States (1st edition)Tuscaloosa: University of Alabama Press.
-
Sources of error in the estimation of mosquito infection rates used to assess risk of arbovirus transmissionAmerican Journal of Tropical Medicine and Hygiene 82:1172–1184.https://doi.org/10.4269/ajtmh.2010.09-0323
-
Habitat characteristics of Coquillettidia perturbans in central floridaJournal of the American Mosquito Control Association 3:176–180.
-
Mosquitoes in bromeliads at ground level of the brazilian Atlantic forest: the relationship between mosquito fauna, water volume, and plant typeAnnals of the Entomological Society of America 108:449–458.https://doi.org/10.1093/aesa/sav040
-
Yellow fever virus in haemagogus leucocelaenus and aedes serratus mosquitoes, southern brazil, 2008Emerging Infectious Diseases 16:1918–1924.https://doi.org/10.3201/eid1612.100608
-
Seasonal abundance, biting cycle, parity and vector potential of the mosquito haemagogus equinus in TrinidadMedical and Veterinary Entomology 7:141–146.https://doi.org/10.1111/j.1365-2915.1993.tb00667.x
-
Distribution of haemagogus mosquitoes on small islands off Trinidad, W. IMosquito Systematics 17:147–153.
-
Seasonality, biting cycle and parity of the yellow fever vector mosquito haemagogus janthinomys in TrinidadMedical and Veterinary Entomology 6:143–148.https://doi.org/10.1111/j.1365-2915.1992.tb00592.x
-
Diel biting activity of culex (Melanoconion) caudelli in Trinidad, west indiesMedical and Veterinary Entomology 3:231–237.https://doi.org/10.1111/j.1365-2915.1989.tb00221.x
-
Downscaling modeling of the aggressiveness of mosquitoes vectors of diseasesEcological Modelling 204:540–546.https://doi.org/10.1016/j.ecolmodel.2007.01.024
-
A study of the host selection patterns of the mosquitoes of the Kisumu area of KenyaTransactions of the Royal Society of Tropical Medicine and Hygiene 69:415–425.https://doi.org/10.1016/0035-9203(75)90200-X
-
Observations on aedes Melanimon and A. dorsalis in NevadaAnnals of the Entomological Society of America 53:706–708.https://doi.org/10.1093/aesa/53.6.706
-
Population structure and dispersal of the saltmarsh mosquito aedes vigilax in Queensland, AustraliaMedical and Veterinary Entomology 13:423–430.https://doi.org/10.1046/j.1365-2915.1999.00195.x
-
Blood feeding patterns of mosquitoes: random or structured?Frontiers in Zoology 7:3–11.https://doi.org/10.1186/1742-9994-7-3
-
Exposure of sheep to mosquito bites: possible consequences for the transmission risk of rift valley fever in SenegalMedical and Veterinary Entomology 18:247–255.https://doi.org/10.1111/j.0269-283X.2004.00511.x
-
Differential susceptibilities of aedes aegypti and aedes albopictus from the americas to zika virusPLOS Neglected Tropical Diseases 10:e0004543.https://doi.org/10.1371/journal.pntd.0004543
-
Anopheles gambiae in Brazil, 1930-40American Journal of Public Health and the Nations Health 34:75–76.https://doi.org/10.2105/AJPH.34.1.75
-
Iguape: a newly recognized Flavivirus from São Paulo state, BrazilIntervirology 36:144–152.
-
The anopheline fauna of papua new guineaJournal of the American Mosquito Control Association 22:213–221.https://doi.org/10.2987/8756-971X(2006)22[213:TAFOPN]2.0.CO;2
-
A note on the biting behaviour of the mosquito, aedes ochraceus, in a village in KenyaEast African Medical Journal 39:511–514.
-
The blood-feeding habits of aedes sollicitans (Walker) in relation to eastern equine encephalitis virus in coastal areas of new jersey. 2. results of experiments with caged mosquitoes and the effects of temperature and physiological age on host selectionJournal of Vector Ecology 21:1–5.
-
The blood-feeding habits of Aedes sollicitans (Walker) in relation to eastern equine encephalitis virus in coastal areas of New Jersey. 3. Habitat preference, vertical distribution, and diel periodicity of host-seeking adultsJournal of Vector Ecology 21:6–13.
-
Transmission of eastern equine encephalomyelitis virus in central AlabamaThe American Journal of Tropical Medicine and Hygiene 68:495–500.
-
BookIdentification and Geographical Distribution of the Mosquitos of North America, North of MexicoUniversity Press of Florida.
-
Attraction of culex portesi senevet & abonnenc and culex taeniopus dyar & knab (Diptera: culicidae) to 20 animal species exposed in a Trinidad forest. I. baits ranked by numbers of mosquitoes caught and engorgedBulletin of Entomological Research 68:707–719.https://doi.org/10.1017/S0007485300009664
-
The identification of the blood-meal in west african mosquitoes by means of the precipitin test. a preliminary report*American Journal of Epidemiology 14:130–141.https://doi.org/10.1093/oxfordjournals.aje.a117751
-
Predicting St. louis encephalitis virus epidemics: lessons from recent, and not so recent, outbreaksAnnual Review of Entomology 46:111–138.https://doi.org/10.1146/annurev.ento.46.1.111
-
Research on the mosquitoes of Angola XXI - Description of Eretmapodites angolensis sp. nov. and Eretmapodites dundo sp. nov. of the oedipodeios groupGarcia De Orta. Série De Zoologia 17:31–35.
-
Alguns aspectos da ecologia dos mosquitos (Diptera: culicidae) de Uma area de planicie (Granjas Calabria), em Jacarepagua, rio de janeiroII. Frequencia Mensal E No Ciclo Lunar. Memoirs Instituto Oswaldo Cruz-Fiocruz 80:123–133.
-
Biting cycle of Culicidae in french guianaCahiers O.R.S.T.O.M. (Office De La Recherche Scientifique Et Technique Outre-Mer) Serie Entomologie Medicale Et Parasitologie 16:73–84.
-
Mosquitoes feeding on brushtail possums (Trichosurus vulpecula) and humans in a native forest fragment in the Auckland region of New ZealandThe New Zealand Medical Journal 120:U2830.
-
[Anopheles of Senegal. an annotated and illustrated list]Bulletin De La Société De Pathologie Exotique 87:267–277.
-
Landscape ecology of sylvatic chikungunya virus and mosquito vectors in southeastern senegalPLoS Neglected Tropical Diseases 6:e1649.https://doi.org/10.1371/journal.pntd.0001649
-
Potential role of sylvatic and domestic african mosquito species in dengue emergenceThe American Journal of Tropical Medicine and Hygiene 73:445–449.
-
An arbovirus disease of present interest: yellow fever, its natural history facing an haemorragic fever, rift valley feverBulletin De La Société De Pathologie Exotique 92:343–348.
-
Studies of arthropod-borne virus infections in QueenslandAustralian Journal of Experimental Biology and Medical Science 42:149–164.https://doi.org/10.1038/icb.1964.16
-
Forest mosquitoes of the ivory coast republic. V. observations on the breeding places of mosquitoes of the genus Eretmapodites in the banco forest, AbidjanBulletin De La Société De Pathologie Exotique 54:1253–1265.
-
Zika virus outbreak on Yap Island, federated states of MicronesiaNew England Journal of Medicine 360:2536–2543.https://doi.org/10.1056/NEJMoa0805715
-
Culex restuans (Diptera: culicidae) relative abundance and vector competence for west nile virusJournal of Medical Entomology 42:838–843.https://doi.org/10.1093/jmedent/42.5.838
-
A working guide to boosted regression treesJournal of Animal Ecology 77:802–813.https://doi.org/10.1111/j.1365-2656.2008.01390.x
-
Spatiotemporal distribution of diurnal yellow fever vectors (Diptera: culicidae) at two sylvan interfaces in Kenya, east africaVector-Borne and Zoonotic Diseases 7:129–142.https://doi.org/10.1089/vbz.2006.0561
-
Notes on Freetown mosquitos, with descriptions of new and Little-Known speciesAnnals of Tropical Medicine & Parasitology 20:97–108.https://doi.org/10.1080/00034983.1926.11684481
-
Breeding habitats of culex tritaeniorhynchus (Diptera: culicidae), A Japanese encephalitis vector, and associated mosquitoes in Mysore, IndiaJournal of the Entomological Research Society 10:1–9.
-
West nile virus transmission in sentinel chickens and potential mosquito vectors, Senegal river Delta, 2008-2009International Journal of Environmental Research and Public Health 10:4718–4727.https://doi.org/10.3390/ijerph10104718
-
"Bird biting" mosquitoes and human disease: a review of the role of Culex pipiens complex mosquitoes in epidemiologyInfection, Genetics and Evolution 11:1577–1585.https://doi.org/10.1016/j.meegid.2011.08.013
-
The tree-hole species of mosquitoes of peiping, ChinaChinese Medical Journal 2:503–525.
-
Culex quinquefasciatus from rio de janeiro is not competent to transmit the local zika virusPLOS Neglected Tropical Diseases 10:e0004993.https://doi.org/10.1371/journal.pntd.0004993
-
Natural enzootic vectors of venezuelan equine encephalitis virus, Magdalena Valley, ColombiaEmerging Infectious Diseases 9:49–54.https://doi.org/10.3201/eid0901.020136
-
An altitude biting study of culex tritaeniorhynchus (Giles) and other associated mosquitoes in japanJournal of Economic Entomology 52:490–492.https://doi.org/10.1093/jee/52.3.490
-
Emerging vectors in the culex pipiens complexScience 303:1535–1538.https://doi.org/10.1126/science.1094247
-
New vectors of rift valley fever in west africaEmerging Infectious Diseases 4:289–293.https://doi.org/10.3201/eid0402.980218
-
Unexpected anthropophily in the potential secondary malaria vectors anopheles coustani s.l. and anopheles squamosus in Macha, ZambiaVector-Borne and Zoonotic Diseases 11:1173–1179.https://doi.org/10.1089/vbz.2010.0082
-
Greedy function approximation: a gradient boosting machineThe Annals of Statistics 29:1189–1232.https://doi.org/10.1214/aos/1013203451
-
Natural History of Culiseta Impatiens (Wlk.), (Diptera, Culicidae), in Alaska. Transactions of American Microscopical Society103–118, Natural History of Culiseta Impatiens (Wlk.), (Diptera, Culicidae), in Alaska. Transactions of American Microscopical Society, 72, 10.2307/3223507.
-
Ecological observations on forest mosquitoes of an endemic yellow fever area in PanamaThe American Journal of Tropical Medicine and Hygiene 31:98–137.
-
Observations on diurnal forest mosquitoes in relation to sylvan yellow fever in PanamaThe American Journal of Tropical Medicine and Hygiene 30:533–574.
-
Bionomics of Sabethes chloropterus humboldt, a vector of sylvan yellow fever in middle americaThe American Journal of Tropical Medicine and Hygiene 7:429–440.
-
The aedes (Aedimorphus) Domesticus group (Diptera, Culicidae)Mosquito Systematics 19:100–110.
-
Isolation of the yellow fever virus from the aedes of the Aedes-Africanus group in the Central-African-Republic the importance of the humid and semi humid savanna in the emergence zone of the amaril virusCahiers O.R.S.T.O.M. (Office De La Recherche Scientifique Et Technique Outre-Mer) Serie Entomologie Medicale Et Parasitologie 14:125–140.
-
Mosquito species composition, phenology and distribution (Diptera: culicidae) on prince Edward islandJournal of the Acadian Entomological Society 3:7–27.
-
The anophelinae of Africa south of the sahara (Ethiopian zoogeographical region)Publications of the South African Institute for Medical Research 54:1–343.
-
Ecologia de Haemagogus e Sabethes (Diptera: Culicidae) em áreas epizoóticas do vírus da febre amarela, Rio Grande do Sul, BrasilEpidemiologia e Serviços de Saúde 19:101–113.
-
Description of the new species anopheles artemievi sp.nDiptera, Culicidae). ResearchGate 2:4–5.
-
Genomics and evolution of Aedes-borne flavivirusesJournal of General Virology 91:87–94.https://doi.org/10.1099/vir.0.014506-0
-
Factors influencing transmission of japanese B encephalitis virus by a colonized strain of culex tritaeniorhynchus giles, from infected pigs and chicks to susceptible pigs and birdsThe American Journal of Tropical Medicine and Hygiene 7:365–373.
-
Variation in susceptibility to oral infection with dengue viruses among geographic strains of aedes aegyptiThe American Journal of Tropical Medicine and Hygiene 28:1045–1052.
-
Culex pipiens quinquefasciatus: a potential vector to transmit zika virusEmerging Microbes & Infections 5:e102.https://doi.org/10.1038/emi.2016.102
-
Genetic characterization of zika virus strains: geographic expansion of the asian lineagePLoS Neglected Tropical Diseases 6:e1477.https://doi.org/10.1371/journal.pntd.0001477
-
Twelve isolations of Zika virus from Aedes (Stegomyia) africanus (Theobald) taken in and above a Uganda forestBulletin of the World Health Organization 31:57–69.
-
The mosquito fauna and climate of native huts at Kisumu, KenyaBulletin of Entomological Research 33:91–142.https://doi.org/10.1017/S0007485300026389
-
The mosquitoes of bwamba county, Uganda. IV.- Studies on the genus Eretmapodites, theobaldBulletin of Entomological Research 37:57–82.https://doi.org/10.1017/S0007485300021994
-
The mosquitoes of bwamba county, UgandaBulletin of Entomological Research 37:57.https://doi.org/10.1017/S0007485300021994
-
Observations on the biting habits of mosquitos in the forest canopy at Zika, Uganda, with special reference to the crepuscular periodsBulletin of Entomological Research 55:589–608.https://doi.org/10.1017/S0007485300049695
-
Culex annulirostris (Diptera: Culicidae) host feeding patterns and Japanese encephalitis virus ecology in northern AustraliaJournal of Medical Entomology 49:371–377.https://doi.org/10.1603/ME11148
-
Dengue virus-mosquito interactionsAnnual Review of Entomology 53:273–291.https://doi.org/10.1146/annurev.ento.53.103106.093326
-
Undiscovered bat hosts of filovirusesPLOS Neglected Tropical Diseases 10:e0004815.https://doi.org/10.1371/journal.pntd.0004815
-
Notes on the biology of Orthopodomyia in IllinoisJournal of the American Mosquito Control Association 11:375–376.
-
Uranotaenia (Pseudoficalbia) mashonaensis, an afrotropical species found in northern israelJournal of the American Mosquito Control Association 23:224–225.https://doi.org/10.2987/8756-971X(2007)23[224:UPMAAS]2.0.CO;2
-
The mosquitoes of the subgenus culex in southwestern Asia and Egypt (Diptera: culicidae)ResearchGate 24:1–240.
-
Intrinsic factors affecting vector competence of mosquitoes for arbovirusesAnnual Review of Entomology 28:229–262.https://doi.org/10.1146/annurev.en.28.010183.001305
-
Epidemiology and transmission dynamics of west nile virus diseaseEmerging Infectious Diseases 11:1167–1173.https://doi.org/10.3201/eid1108.050289a
-
Changes in mosquito populations with expansion of the ross river reservoir, Australia, from stage 1 to stage 2AJournal of the American Mosquito Control Association 11:211–224.
-
Collection records of the project "Mosquitoes of Middle America"Mosquito Systematics 12:179–284.
-
Bioecologie comparee d’Aedes opok Corbet et Van Someren et A. africanus Theobald dans une galerie forestiere du sud de la Republique CentrafricaineCah ORSTOM Ser Ent Med Et Parasitol 14:235–244.
-
Influence de la clarte lunaire sur l’activite trophique d’Aedes taylori (Diptera, Culicidae)Cah ORSTOM Ser Ent Med Et Parasitol 24:59–65.
-
Observations on Eretmapodites silvestris Conchobius Edwards (Culicidae) in the Anglo-Egyptian SudanThe American Journal of Tropical Medicine and Hygiene 31:659–664.
-
Mosquitoes of the ethiopian region I. larval bionomics of mosquitoes and taxonomy of culicine larvaeAdlard & Son, Ltd, British Museum of Natural History.
-
Culex species mosquitoes and zika virusVector-Borne and Zoonotic Diseases 16:673–676.https://doi.org/10.1089/vbz.2016.2058
-
Consistently high estimates for the proportion of human exposure to malaria vector populations occurring indoors in rural africaInternational Journal of Epidemiology 42:235–247.https://doi.org/10.1093/ije/dys214
-
Determination of mosquito (Diptera: culicidae) bloodmeal sources in western Australia: implications for arbovirus transmissionJournal of Medical Entomology 46:1167–1175.https://doi.org/10.1603/033.046.0527
-
The laboratory colonization of culex (Culex) univittatus theobald (Diptera: culicidae) from material collected in the highveld region of south AfricaJournal of the Entomological Society of Southern Africa 30:34–39.
-
Studies on an outbreak of wesselsbron virus in the free state province, South AfricaJournal of the American Mosquito Control Association 14:40–45.
-
Laboratory vector competence experiments with yellow fever virus and five south african mosquito species including aedes aegyptiTransactions of the Royal Society of Tropical Medicine and Hygiene 96:493–498.https://doi.org/10.1016/S0035-9203(02)90417-7
-
Culex (Eumelanomyia) rubinotus theobald as vector of banzi, Germiston and witwatersrand virusesJournal of Medical Entomology 12:647–651.https://doi.org/10.1093/jmedent/12.6.647
-
A bionomic study of adult aedes (Neomelaniconion) circumluteolus in northern Kwazulu, South AfricaJournal of the American Mosquito Control Association 3:131–136.
-
Larval habitats of culicine mosquitoes (Diptera: culcidae) in a sewage effluent disposal area in the south african high veldJournal of Entomology of South Africa 30:243–250.
-
Bionomics of culex epidesmus associated with japanese encephalitis virus in IndiaJournal of the American Mosquito Control Association 19:151–154.
-
International catalog of arboviruses including certain other viruses of vertebratesThe American Journal of Tropical Medicine and Hygiene 27:372–440.
-
[The culicidian fauna and its nuisance in Kinshasha (Zaire)]Bulletin De La Société De Pathologie Exotique 86:68–75.
-
[Anopheles paludis: important vector of malaria in zaire]Bulletin De La Société De Pathologie Exotique 85:388–389.
-
Invasion biology of aedes japonicus japonicus (Diptera: culicidae)Annual Review of Entomology 59:31–49.https://doi.org/10.1146/annurev-ento-011613-162012
-
The importance of subterranean mosquito habitat to arbovirus vector control strategies in North Queensland, AustraliaJournal of Medical Entomology 37:846–853.https://doi.org/10.1603/0022-2585-37.6.846
-
Host-feeding patterns of the mosquito community (Diptera: culicidae) in Aswan Governorate, EgyptJournal of Medical Entomology 24:35–39.https://doi.org/10.1093/jmedent/24.1.35
-
Characterization of rice field mosquito habitats in Sharkia Governorate, EgyptJournal of the Egyptian Society of Parasitology 28:449–459.
-
Studies on the transmission of experimental yellow fever by culex thalassius and mansonia uniformisAnn Trop Med and Parasitol [Liverpool] 26:119–127.https://doi.org/10.1080/00034983.1932.11684709
-
Fauna and larval habitats of mosquitoes (Diptera: culicidae) of west Azerbaijan province, northwestern iranJournal of Arthropod-Borne Diseases 8:163–173.
-
West nile virus risk assessment and the bridge vector paradigmEmerging Infectious Diseases 11:425–429.https://doi.org/10.3201/eid1103.040364
-
Two new species of mosquitoes of the genus Ficalbia from netherlands new guineaProceedings of the Entomological Society of Washington 48:186–190.
-
Experimental infection of north american birds with the New York 1999 strain of west nile virusEmerging Infectious Diseases 9:311–322.https://doi.org/10.3201/eid0903.020628
-
Day-Time resting habitats of culicine mosquitoes and their preponderanceJournal of Communicable Diseases 20:280–286.
-
Biting rhythm of the vectors of malayan filariasis, mansonia annulifera, M. uniformis & M. indiana in shertallai (Kerala state), IndiaThe Indian Journal of Medical Research 89:52–55.
-
The virus of IlhéUs encephalitis; isolation, serological specificity and transmission. journal of immunology (Baltimore, md1950) 55:61–67.
-
Culex nigripalpus theobald (Diptera, Culicidae) feeding habit at the parque ecologico do Tiete, Sao Paulo, brazilRevista Brasileira De Entomologia 52:663–668.https://doi.org/10.1590/S0085-56262008000400019
-
Description of the larva, pupa and female of aedes (N.) jemoti and revision of the keys to the subgenus neomelaniconion in Africa south of the SaharaBulletin De La Société De Pathologie Exotique 53:1054–1064.
-
Aedes hensilli as a potential vector of Chikungunya and zika virusesPLoS Neglected Tropical Diseases 8:e3188.https://doi.org/10.1371/journal.pntd.0003188
-
[Seasonal prevalence and behaviour of aedes togoi]The Korean Journal of Parasitology 33:19–26.https://doi.org/10.3347/kjp.1995.33.1.19
-
Vertical transmission of arboviruses in mosquitoes: a historical perspectiveInfection, Genetics and Evolution : Journal of Molecular Epidemiology and Evolutionary Genetics in Infectious Diseases 28:681–690.https://doi.org/10.1016/j.meegid.2014.07.025
-
Observations on the dispersal and survival of a population of aedes lineatopennis (Ludlow) (Diptera: culicidae) in KenyaBulletin of Entomological Research 75:661.https://doi.org/10.1017/S0007485300015923
-
On the selection of thresholds for predicting species occurrence with presence-only dataEcology and Evolution 6:337–348.https://doi.org/10.1002/ece3.1878
-
Selecting thresholds for the prediction of species occurrence with presence-only dataJournal of Biogeography 40:778–789.https://doi.org/10.1111/jbi.12058
-
One year’s observations on the development and habits of A. sinensis and C. fatigans in FoochowActa Ent Sinica 10:86–95.
-
AUC: a misleading measure of the performance of predictive distribution modelsGlobal Ecology and Biogeography 17:145–151.https://doi.org/10.1111/j.1466-8238.2007.00358.x
-
Mosquito species collected from a marsh in western Kenya during the long rainsJournal of the American Mosquito Control Association 7:395–399.
-
Ecology of mosquitoes (Diptera: culicidae) in natural and artificial breeding sites of rural area in the north of Parana state, Brazil. 4. wild species breeding in artificial reservoirsArquivos De Biologia E Tecnologia 39:671–676.
-
Ecology of mosquitoes (Diptera, Culicidae) in natural and artificial rural breeding in places in northern Parana state, brazilVI. Larvae Collections in the Home Surroundings. Revista Brasileira De Zoologia 14:571–578.https://doi.org/10.1590/S0101-81751997000300007
-
The bionomics of three sympatric Eretmapodites (Diptera: culicidae) at the Kenya coastBulletin of Entomological Research 70:309–320.https://doi.org/10.1017/S0007485300007598
-
Blood meal analysis and virus detection in blood-fed mosquitoes collected during the 2006-2007 rift valley fever outbreak in KenyaVector Borne and Zoonotic Diseases 14:656–664.https://doi.org/10.1089/vbz.2013.1564
-
Arbovirus infections in Sarawak: observations on the mosquitoesJournal of Medical Entomology 1:335–347.https://doi.org/10.1093/jmedent/1.4.335
-
Host feeding patterns of culex mosquitoes (Diptera: culicidae) in east baton rouge parish, LouisianaJournal of Medical Entomology 47:238–248.https://doi.org/10.1093/jmedent/47.2.238
-
[Registry and distribution update of the species of the haemagogus (Diptera: culicidae) genus in the Caribbean region of Colombia]Biomedica : Revista Del Instituto Nacional De Salud 33 Suppl 1:185–189.
-
Host preferences of deinocerites pseudes dyar & knabJournal of Medical Entomology 10:206–208.https://doi.org/10.1093/jmedent/10.2.206
-
Further observations on the natural hosts of three species of mansonia blanchard (Diptera, Culicidae) in UgandaAnnals of Tropical Medicine & Parasitology 54:300–304.https://doi.org/10.1080/00034983.1960.11685990
-
An entomological review of invasive mosquitoes in EuropeBulletin of Entomological Research 105:637–663.https://doi.org/10.1017/S0007485315000103
-
Molecular identification of blood-meal sources in Culiseta melanura and Culiseta morsitans from an endemic focus of eastern equine encephalitis virus in New YorkThe American Journal of Tropical Medicine and Hygiene 75:1140–1147.
-
Factors of concern regarding zika and other aedes aegypti-Transmitted viruses in the United StatesJournal of Medical Entomology tjw212.https://doi.org/10.1093/jme/tjw212
-
Experimental transmission of west nile virus by culex nigripalpus from hondurasVector Borne and Zoonotic Diseases 7:279–284.https://doi.org/10.1089/vbz.2006.0557
-
Larval population dynamics in a community of nearctic aedes inhabiting a temporary vernal poolJournal of the American Mosquito Control Association 8:52–57.
-
Mosquitoes of the genus culex in the suez canal governoratesJournal of the Egyptian Society of Parasitology 20:265–268.
-
Observations sur quelques anophèles exophiles au camerounBulletin De La Société De Pathologie Exotique 50:378–381.
-
Larval environmental temperature and insecticide exposure alter aedes aegypti competence for arbovirusesVector-Borne and Zoonotic Diseases 11:1157–1163.https://doi.org/10.1089/vbz.2010.0209
-
Host-feeding patterns of culex tritaeniorhynchus and anopheles sinensis (Diptera: culicidae) in a ricefield agroecosystemMedical Entomology and Zoology 50:267–273.https://doi.org/10.7601/mez.50.267
-
Spatial distribution and habitat characterisation of anopheles larvae along the kenyan coastJournal of Vector Borne Diseases 44:44–51.
-
Seasonal mosquito larval abundance and composition in Kibwezi, lower eastern KenyaJournal of Vector Borne Diseases 46:65–71.
-
On the adult mosquito fauna (Diptera, Culicidae) in an hydroelectric project area in the Parana river basin, BrazilRevista Brasileira De Entomologia 41:213–216.
-
New Sabethes (Diptera: culicidae) species records for Ecuador, from Colonso-Chalupas biological reserve, province of napo (Amazon)Journal of Entomology and Zoology Studies 3:169–172.
-
Vector competence of culex neavei (Diptera: culicidae) for usutu virusThe American Journal of Tropical Medicine and Hygiene 86:993–996.https://doi.org/10.4269/ajtmh.2012.11-0509
-
Some characteristics of Israel turkey virusArchiv Fur Die Gesamte Virusforschung 36:105–114.https://doi.org/10.1007/BF01250300
-
Identification of new flaviviruses in the kokobera virus complexThe Journal of General Virology 86:121–124.https://doi.org/10.1099/vir.0.80381-0
-
Observations on breeding sites of mosquitoes in lake Manyara, a saline lake in east african rift valleyBulletin of Entomological Research 60:473.https://doi.org/10.1017/S0007485300040426
-
The flight activity of mosquitoes in Ibadan NigeriaNigerian Journal of Entomology 3:81–92.
-
Biting rhythm of vector mosquitoes in a rural ecosystem of south IndiaInternational Journal of Mosquito Research 2:106–113.
-
Skepticism, statistical methods, and the cigarette: a historical analysis of a methodological debatePerspectives in Biology and Medicine 47:244–261.https://doi.org/10.1353/pbm.2004.0032
-
Some culicine mosquitoes (Diptera, Culicidae) at Ndumu, republic of south AfricaMedical Proceedings 10:188–192.
-
Japanese encephalitis in Sri Lanka--the study of an epidemic: vector incrimination, porcine infection and human diseaseTransactions of the Royal Society of Tropical Medicine and Hygiene 86:307–313.https://doi.org/10.1016/0035-9203(92)90325-7
-
The larval development and ecology of aedes (Stegomyia) scutellaris (Walker, 1859) in New GuineaThe Journal of Parasitology 33:43–50.https://doi.org/10.2307/3273619
-
Rapid spread of zika virus in the americas--implications for public health preparedness for mass gatherings at the 2016 Brazil olympic gamesInternational Journal of Infectious Diseases 44:11–15.https://doi.org/10.1016/j.ijid.2016.02.001
-
The occurrence of deinocerites pseudes dyar and knab in the united states, with additional notes on the biology of deinocerites species of TexasMosquito News 24:449–458.
-
History of domestication and spread of aedes aegypti--a reviewMemórias Do Instituto Oswaldo Cruz 108 Suppl 1:11–17.https://doi.org/10.1590/0074-0276130395
-
Biology of culex sitiens, a predominant mosquito in Phang Nga, Thailand after a tsunamiJournal of Insect Science 12:1–8.https://doi.org/10.1673/031.012.1101
-
Field evaluation of a large-scale barrier application of bifenthrin on a golf course to control floodwater mosquitoesJournal of the American Mosquito Control Association 28:219–224.https://doi.org/10.2987/12-6255R.1
-
Feeding behavior and spatial distribution of culex mosquitoes (Diptera: culicidae) in wetland areas of the czech republicJournal of Medical Entomology 50:1097–1104.https://doi.org/10.1603/ME13029
-
Distribution of the genera Coquillettidia, orthopodomyia and uranotaenia in EuropeEuropean Mosquito Bulletin 10:25–29.
-
Phylogeny and classification of ochlerotatus and allied taxa (Diptera: culicidae: aedini) based on morphological data from all life stagesZoological Journal of the Linnean Society 153:29–114.https://doi.org/10.1111/j.1096-3642.2008.00382.x
-
Contributions to the mosquito fauna of southeast asiaContributions of the American Entomological Institute 5:1–44.
-
Albuginosus, a new subgenus of aedes Meigem (Diptera: Culicidae) described from the afrotropical regionMosquito Systematics 3:307–326.
-
Observations on the diel activity patterns of some punjab Pakistan mosquitoes diptera CulicidaeBiologia 22:67–78.
-
Host finding, feeding patterns and evidence for a memorized Home-RangeMedical and Veterinary Entomology 8:187–193.https://doi.org/10.1111/j.1365-2915.1994.tb00162.x
-
Spatial-Dispersion patterns of larval aedes cantans (diptera, Culicidae)Bulletin of Entomological Research 85:125–133.https://doi.org/10.1017/s0007485300052081
-
Mosquito blood feeding patterns as a factor in the epidemiology of japanese encephalitis in southern IndiaThe American Journal of Tropical Medicine and Hygiene 46:654–663.
-
Habitat segregation of mosquito arbovirus vectors in south FloridaJournal of Medical Entomology 43:1134–1141.https://doi.org/10.1093/jmedent/43.6.1134
-
Gbm: generalized boosted regression modelsGbm: generalized boosted regression models, R package version 2.1.1.
-
Urban insects and arachnids: a handbook of urban entomologyCambridge University Press.https://doi.org/10.1017/CBO9780511542718
-
Salt marsh as culex salinarius larval habitat in coastal New YorkJournal of the American Mosquito Control Association 24:359–367.https://doi.org/10.2987/5748.1
-
Habitats and distribution of anopheles sinensis and associated Anopheles hyrcanus group in JapanJournal of the American Mosquito Control Association 21:458–463.https://doi.org/10.2987/8756-971X(2006)21[458:HADOAS]2.0.CO;2
-
Larval habitats of culex tarsalis (Diptera: culicidae) in MinnesotaMosquito News 24:39–42.
-
Seasonal abundance of mosquitoes in a native forest of the murray valley of Victoria, 1979?1985Australian Journal of Entomology 25:235–240.https://doi.org/10.1111/j.1440-6055.1986.tb01109.x
-
A review of the status and significance of the species within the culex pipiens group in AustraliaJournal of the American Mosquito Control Association 28:24–27.https://doi.org/10.2987/8756-971X-28.4s.24
-
Emergence trapping of mosquitoes (Diptera: culicidae) in brackish forest habitats in Maroochy Shire, south-east Queensland, Australia, and a management option for verrallina funerea (Theobald) and aedes procax (Skuse)Australian Journal of Entomology 39:212–218.https://doi.org/10.1046/j.1440-6055.2000.00169.x
-
Seasonal abundance & biting behaviour of mansonia annulifera, M. uniformis & M. indiana & their relative role in the transmission of malayan filariasis in shertallai (Kerala state)The Indian Journal of Medical Research 93:253–258.
-
Revision of the spissipes section of culex (Melanoconion) (Diptera:culicidae)Journal of the American Mosquito Control Association 12:517–600.
-
Possible association between zika virus infection and microcephaly - Brazil, 2015MMWR. Morbidity and Mortality Weekly Report 65:59–62.https://doi.org/10.15585/mmwr.mm6503e2
-
Contributions a l’etude de la Biologie de Taeniorhynchus (Mansonoides) africanus et de Taeniorhynchus (Coquillettidia) aurites au Congo BeigeRevue De Zoologie Et De Botanique Africaines 4:311–330.
-
Mosquito (Diptera: culicidae) fauna in an area endemic for west nile virusJournal of Vector Ecology 35:156–162.https://doi.org/10.1111/j.1948-7134.2010.00072.x
-
The biting activity rhythm in aedini mosquitoes of MaduraiComparative Physiological Ecology 17:66–70.
-
Behavioural adaptation of coquillettidia (Coquillettidia) richiardii larvae to underwater life: environmental cues governing plant–insect interactionEntomologia Experimentalis Et Applicata 120:195–200.https://doi.org/10.1111/j.1570-7458.2006.00444.x
-
The ecology of the Tree-Hole breeding mosquitoes in the northern guinea savanna of NigeriaThe Journal of Applied Ecology 2:1–16.https://doi.org/10.2307/2401689
-
The identification of blood-meals from culicine mosquitos from northern NigeriaBulletin of Entomological Research 55:637–643.https://doi.org/10.1017/S0007485300049749
-
Mosquito ecology: field sampling methodsSpringer Science & Business Media.https://doi.org/10.1007/978-94-015-8113-4
-
Larval habitat diversity and ecology of anopheline larvae in EritreaJournal of Medical Entomology 40:921–929.https://doi.org/10.1603/0022-2585-40.6.921
-
Seasonal larval and adult population dynamics and breeding habitat diversity of culex theileri theobald, 1903 (Diptera: culicidae) in the golbasi district, Ankara, turkeyTurkish Journal of Zoology 28:337–344.
-
Culex-adamesi new-species from panama diptera culicidaeMosquito Systematics 12:25–34.
-
Correcting for optimistic prediction in small data setsAmerican Journal of Epidemiology 180:318–324.https://doi.org/10.1093/aje/kwu140
-
Mountain mosquitoes of the gothic, Colorado, areaAmerican Midland Naturalist 76:125–150.https://doi.org/10.2307/2423238
-
The feeding habits of some west african culex (Dipt., Culicidae) mosquitoesBulletin of Entomological Research 62:517–526.https://doi.org/10.1017/S0007485300004041
-
The host-feeding patterns of some culicine mosquitoes (Diptera: culicidae) in the GambiaBulletin of Entomological Research 68:695–706.https://doi.org/10.1017/S0007485300009652
-
The female and early stages of culex (Culex) nakuruensis mattingly, with a description of a new subspecies of culex (Culex) shoae hamon & ovazzaProceedings of the Royal Entomological Society of London. Series B, Taxonomy 36:11–16.https://doi.org/10.1111/j.1365-3113.1967.tb00528.x
-
Notes on activities of Alaskan Culiseta adults (Diptera: culicidae)Mosquito News 24:60–64.
-
Culicidae (Diptera) selection of humans, chickens and rabbits in three different environments in the province of chaco, ArgentinaMemórias Do Instituto Oswaldo Cruz 108:563–571.https://doi.org/10.1590/S0074-02762013000500005
-
Aëdes (Ochlerotatus) caballus theobald, the south african vector of rift valley feverSouth African Medical Journal = Suid-Afrikaanse Tydskrif Vir Geneeskunde 29:1114–1120.
-
Culex gelidus: an emerging mosquito vector with potential to transmit multiple virus infectionsJournal of Vector Borne Diseases 51:251–258.
-
Aedes species in treeholes and fruit husks between dry and wet seasons in southeastern senegalJournal of Vector Ecology 38:237–244.https://doi.org/10.1111/j.1948-7134.2013.12036.x
-
Taxonomic and ecological notes on culex (Melanoconion) Spissipes (Theobald)Journal of Medical Entomology 5:329–331.https://doi.org/10.1093/jmedent/5.3.329
-
Isolation and genetic characterization of a tembusu virus strain isolated from mosquitoes in Shandong, ChinaTransboundary and Emerging Diseases 62:209–216.https://doi.org/10.1111/tbed.12111
-
Entomological surveys of malaria in Khammouane Province, lao PDR, in 1999 and 2000The Southeast Asian Journal of Tropical Medicine and Public Health 33:532–546.
-
Aedes mosquito larvae collected from Ishigaki-jima and Taketomi-jima islands in southern JapanThe Southeast Asian Journal of Tropical Medicine and Public Health 43:1375–1379.
-
An update on the potential of north american mosquitoes (Diptera: culicidae) to transmit west nile virusJournal of Medical Entomology 42:57–62.https://doi.org/10.1093/jmedent/42.1.57
-
Vector competence of north american mosquitoes (Diptera: culicidae) for west nile virusJournal of Medical Entomology 38:130–134.https://doi.org/10.1603/0022-2585-38.2.130
-
Notes on haemagogus anastasionis dyar of curaçaoDocumenta Neerlandica Et Indonesica De Morbis Tropicis; Quarterly Journal of Tropical Medicine and Hygiene 1:142–144.
-
BookVirus Taxonomy : Classification and Nomenclature of Viruses : Seventh Report of the International Committee on Taxonomy of VirusesSan Diego: Academic Press.
-
Avian malaria infections in western european mosquitoesParasitology Research 111:637–645.https://doi.org/10.1007/s00436-012-2880-3
-
Nationwide inventory of mosquito biodiversity (Diptera: culicidae) in Belgium, EuropeBulletin of Entomological Research 103:193–203.https://doi.org/10.1017/S0007485312000521
-
Caiman-biting mosquitoes and the natural vectors of Hepatozoon caimani in brazilJournal of Medical Entomology 47:670–676.https://doi.org/10.1093/jmedent/47.4.670
-
Studies on cyclic passage of yellow fever virus in south american mammals and mosquitoes - Marmosets (callithrix-Aurita) and Cebus monkeys (cebus-Versutus) in combination with Aedes-Aegypti and Haemagogus-EquinusAmerican Journal of Tropical Medicine 25:225–230.
-
Host preference of mosquito vectors of japanese encephalitisZhonghua Minguo Wei Sheng Wu Xue Za Zhi = Chinese Journal of Microbiology 8:274–279.
-
Biting activity and host attractancy of mosquitoes (Diptera: culicidae) in Manzhouli, ChinaJournal of Medical Entomology 49:1283–1288.https://doi.org/10.1603/ME11131
-
L’habitat des Phlebotomes cavernicoles de Thys-ville (Congo Beige)Archives De l’Institute Pasteur d’Algerie 24:153–156.
-
Chikungunya: evolutionary history and recent epidemic spreadAntiviral Research 120:32–39.https://doi.org/10.1016/j.antiviral.2015.04.016
-
BookHost range, amplification and arboviral disease emergenceIn: Peters CJ, Calisher CH, editors. Infectious Diseases From Nature: Mechanisms of Viral Emergence and Persistence. Springer-Verlag/Wien: Springer Vienna. pp. 33–44.https://doi.org/10.1007/3-211-29981-5_4
-
The biology of mansonia mosquitoes in relation to the transmission of filariasis in MalayaBulletin - Institute for Medical Research, Kuala Lumpur 11:1–114.
-
A preliminary investigation of the ecology of arboviruses in the Derby area of the Kimberley region, Western AustraliaAustralian Journal of Experimental Biology and Medical Science 59:357–367.https://doi.org/10.1038/icb.1981.30
-
Mosquitoes of rarotonga, cook islands: a survey of breeding sitesNew Zealand Journal of Zoology 34:57–61.https://doi.org/10.1080/03014220709510064
Article and author information
Author details
Funding
National Science Foundation (DEB-1640780)
- Courtney C Murdock
University of Georgia (Presidential Fellowship)
- Michelle V Evans
National Institutes of Health (U01GM110744)
- John M Drake
The funders had no role in study design, data collection and interpretation, or the decision to submit the work for publication.
Acknowledgements
The authors acknowledge Pasha Feinberg for assistance with data collection.
Copyright
© 2017, Evans et al.
This article is distributed under the terms of the Creative Commons Attribution License, which permits unrestricted use and redistribution provided that the original author and source are credited.
Metrics
-
- 4,502
- views
-
- 917
- downloads
-
- 65
- citations
Views, downloads and citations are aggregated across all versions of this paper published by eLife.
Download links
Downloads (link to download the article as PDF)
Open citations (links to open the citations from this article in various online reference manager services)
Cite this article (links to download the citations from this article in formats compatible with various reference manager tools)
Further reading
-
- Computational and Systems Biology
- Medicine
Excessive consumption of sucrose, in the form of sugar-sweetened beverages, has been implicated in the pathogenesis of metabolic dysfunction‐associated fatty liver disease (MAFLD) and other related metabolic syndromes. The c-Jun N-terminal kinase (JNK) pathway plays a crucial role in response to dietary stressors, and it was demonstrated that the inhibition of the JNK pathway could potentially be used in the treatment of MAFLD. However, the intricate mechanisms underlying these interventions remain incompletely understood given their multifaceted effects across multiple tissues. In this study, we challenged rats with sucrose-sweetened water and investigated the potential effects of JNK inhibition by employing network analysis based on the transcriptome profiling obtained from hepatic and extrahepatic tissues, including visceral white adipose tissue, skeletal muscle, and brain. Our data demonstrate that JNK inhibition by JNK-IN-5A effectively reduces the circulating triglyceride accumulation and inflammation in rats subjected to sucrose consumption. Coexpression analysis and genome-scale metabolic modeling reveal that sucrose overconsumption primarily induces transcriptional dysfunction related to fatty acid and oxidative metabolism in the liver and adipose tissues, which are largely rectified after JNK inhibition at a clinically relevant dose. Skeletal muscle exhibited minimal transcriptional changes to sucrose overconsumption but underwent substantial metabolic adaptation following the JNK inhibition. Overall, our data provides novel insights into the molecular basis by which JNK inhibition exerts its metabolic effect in the metabolically active tissues. Furthermore, our findings underpin the critical role of extrahepatic metabolism in the development of diet-induced steatosis, offering valuable guidance for future studies focused on JNK-targeting for effective treatment of MAFLD.
-
- Computational and Systems Biology
Manifold-learning is particularly useful to resolve the complex cellular state space from single-cell RNA sequences. While current manifold-learning methods provide insights into cell fate by inferring graph-based trajectory at cell level, challenges remain to retrieve interpretable biology underlying the diverse cellular states. Here, we described MGPfactXMBD, a model-based manifold-learning framework and capable to factorize complex development trajectories into independent bifurcation processes of gene sets, and thus enables trajectory inference based on relevant features. MGPfactXMBD offers a more nuanced understanding of the biological processes underlying cellular trajectories with potential determinants. When bench-tested across 239 datasets, MGPfactXMBD showed advantages in major quantity-control metrics, such as branch division accuracy and trajectory topology, outperforming most established methods. In real datasets, MGPfactXMBD recovered the critical pathways and cell types in microglia development with experimentally valid regulons and markers. Furthermore, MGPfactXMBD discovered evolutionary trajectories of tumor-associated CD8+ T cells and yielded new subtypes of CD8+ T cells with gene expression signatures significantly predictive of the responses to immune checkpoint inhibitor in independent cohorts. In summary, MGPfactXMBD offers a manifold-learning framework in scRNA-seq data which enables feature selection for specific biological processes and contributing to advance our understanding of biological determination of cell fate.