Neural computations underlying inverse reinforcement learning in the human brain
Figures
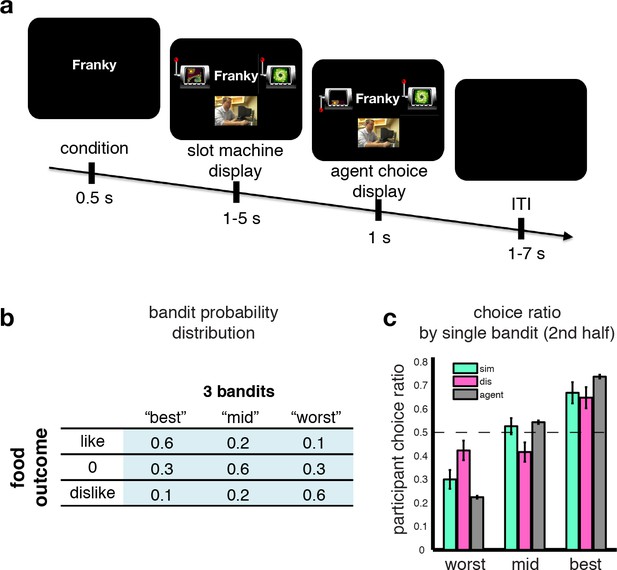
Observational slot machine task with hidden outcomes.
(A) Trial timeline. The first screen signals whether the agent or the participant has to make a choice. Subsequently two slot machines are presented, along with on agent trials a (pseudo) video feed of the agent making a selection, and the choice of the slot machine is revealed after a jittered interval. (B) The probabilistic food distribution behind the slot machines: each of the three slot machines is labeled by a unique fractal and yields the same three food items, but at differing stationary probabilities, as indicated in the table. (C) Agent and participant choice ratio. Choice ratio is defined as choice frequency of a slot machine given its total number of presentations. Agent performance (grey bars) are collapsed across conditions, and depicted in agent-referential space. Participant performance (cyan for similar, pink for dissimilar) is depicted in self-referential space. Regardless of whether they are observing a similar or dissimilar agent, participants are equally good at choosing their best slot machine, nearly matching the agent’s performance.
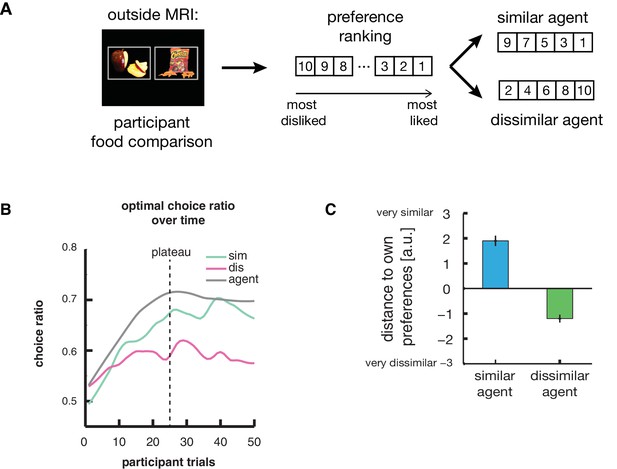
Additional task information.
(A) Pre-MRI phase and agent preferences construction: Participants first made pairwise food comparisons before entering the MRI sessions. From their actual food rating, we then constructed one similar and one dissimilar agent (B) Learning curve of agent (across both conditions, grey) and participant (separately for similar and dissimilar). Dotted line depicts plateau of agent behavior (C) Debrief: All participants classified agents correctly as similar, resp. dissimilar.
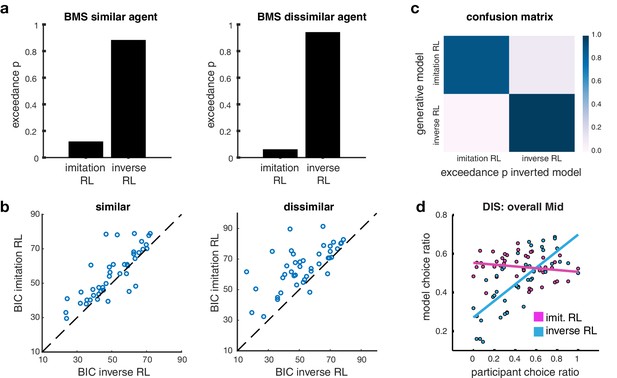
Model comparison.
(A) Bar plots illustrating the results of the Bayesian Model Selection (BMS) for the two main model frameworks. The inverse RL algorithm performs best, across both conditions (similar and dissimilar). On the left, the plot depicts the BMS between the two models in the similar condition; on the right the plot shows the BMS in the dissimilar condition (BMS analysis of auxiliary models are shown in Figure 2—figure supplement 1A). (B) Scatter plots depicting direct model comparisons for all participants. The lower the Bayesian Information Criterion (BIC), the better the model performs, hence if a participant’s point lies over the diagonal, the inverse RL explains the behavior better. The figure on the left illustrates the similar condition; the plot on the right depicts the dissimilar condition. (C) Confusion matrix of the two models to evaluate the performance of the BMS, in the dissimilar condition. Each square depicts the frequency with which each behavioral model wins based on data generated under each model and inverted by itself and all other models. The matrix illustrates that the two models are not 'confused’, hence they capture different specific strategies. Confusion matrices of the similar condition and for auxiliary models are shown in Figure 2—figure supplement 1C) (D) Scatter plots depict the participant choice ratio of the mid slot machine plotted against the predictions of the inverse and imitation RL models.
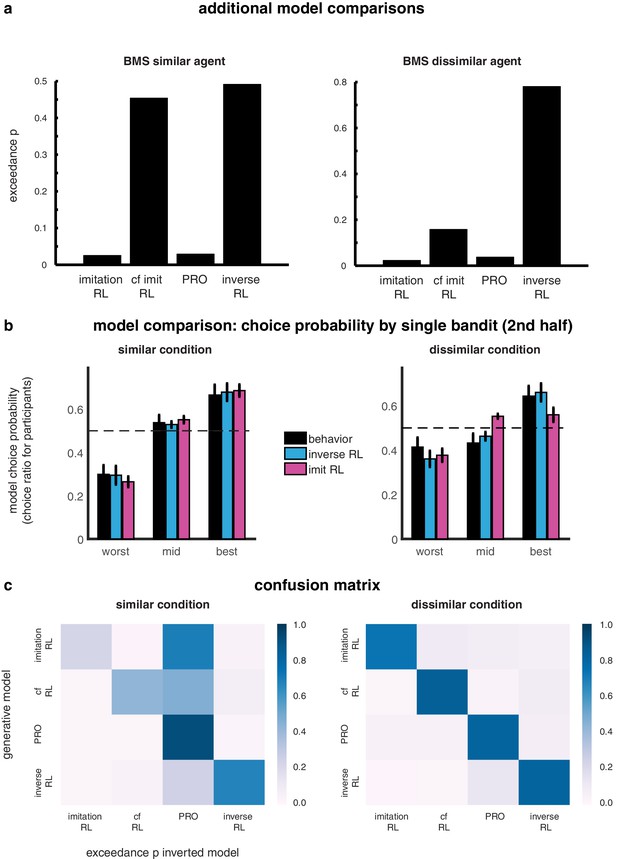
Additional model information.
(A) Bayesian Model Selection with additional models: the imitation RL with counterfactuals as described in the main text, and the PRO model (probabilistic rank order): In the PRO model, the observer assumes that the agents have distributions over the preference rankings for the different slot machines without considering the outcome distributions per se. Each rank-order has a certain likelihood of being the actual agent’s preferences over the n slot machines to be ranked. At feedback, the beliefs over the rank orders are updated in a Bayesian fashion, according to the likelihood of each rank order to be correct given the observed choice of the agent. Subsequently the participant’s choice is expressed as a soft-max function between the expected rank value of the chosen and the unchosen slot machine, with a free parameter characterizing the choice stochasticity. We find again that the inverse RL gives a better explanation of participant behavior, especially in the dissimilar condition. (B) mean choice ratio for participants (black) and choice probability for fitted models, separately for similar and dissimilar conditions (C) Confusion matrix of these four models to evaluate the performance of the BMS. Each square depicts the frequency with which each behavioral model wins based on data generated under each model and inverted by itself and all other models. The matrix illustrates that the four models are not 'confused’, especially in the dissimilar condition, hence they capture different specific strategies.
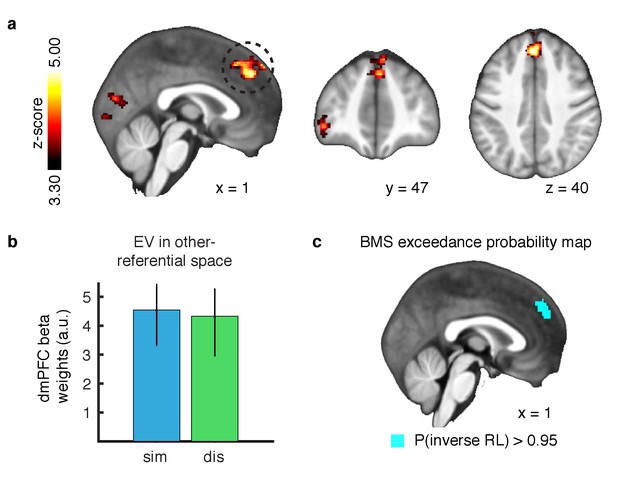
Outcome prediction signals in agent-referential preference space.
(A) Neural response to parametric changes in inverse RL outcome prediction in agent-referential space. Activity in dmPFC at the time of presumptive agent decision significantly correlated with outcome prediction inferred from the inverse RL model, independent of condition. All depicted clusters survive whole brain cluster correction FWE at p<0.05, with a height threshold of p<0.001. Z-score map threshold as indicated, for illustrative purposes. (B) Effect sizes of the outcome prediction correlation in dmPFC cluster separately for each condition (similar = blue, dissimilar = green, parameter estimates are extracted with a leave-one-out procedure, mean ±SEM across participants, p<0.05 for both conditions). (C) Group-level exceedance probability map of the Bayesian Model Selection, comparing predictions from the imitation RL against inverse RL voxelwise in an anatomically defined dmPFC region. The depicted map was thresholded to show voxels where the exceedance probability for the inverse RL model is greater than p=0.95, revealing that the anterior part of dmPFC is much more likely to encode inverse RL computations.
-
Figure 3—source data 1
areas exhibiting significant changes in BOLD associated with predicted outcome in similar and dissimilar.
OFC: orbitofrontal cortex, dmPFC: dorsomedial prefrontal cortex. x y z in MNI coordinates.
- https://doi.org/10.7554/eLife.29718.007
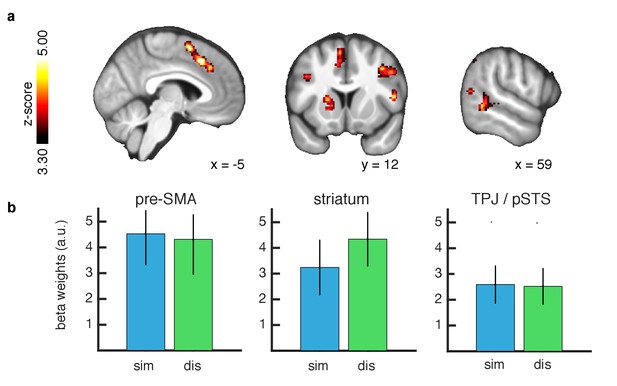
Learning signals during action feedback.
(A) Z-statistic map of the inverse RL entropy signals during agent choice revelation is presented, relating to the update of the food distributions within the chosen slot machine. From left to right slices depict pre-SMA, striatum and TPJ/pSTS. All depicted clusters survive whole brain cluster correction FWE at p<0.05, with a height threshold of p<0.001. Maps are thresholded at Z-statistic values as indicated, for display purposes. (B) Effect sizes of the entropy correlations for all clusters, separately for each condition (similar = blue, dissimilar = green, L1O procedure, mean ±SEM across participants, all p < 0.05 for both conditions).
-
Figure 4—source data 1
areas exhibiting significant changes in BOLD associated with entropy signals.
Pre-SMA: pre-supplementary motor area. TPJ: temporo-parietal junction. dlPFC: dorsolateral prefrontal cortex. x y z in MNI coordinates.
- https://doi.org/10.7554/eLife.29718.009
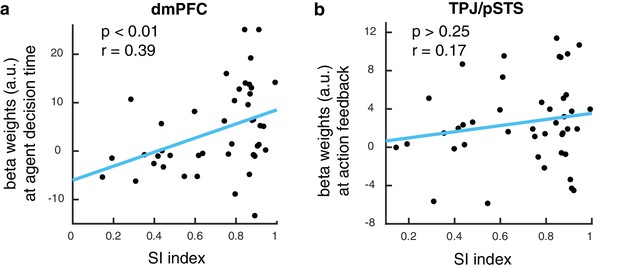
dmPFC signal predicts performance in slot machine game.
(A) Scatter plot showing beta estimates of outcome prediction signals in the dmPFC ROI across participants, plotted against the social information integration index (SI index), which characterizes each participant’s performance in the slot machine game. The higher the score, the better the participants are at inferring the best option for themselves from observing the other. (B) Scatter plot of entropy update signals in the TPJ/pSTS ROI across participants plotted against the social information integration index.
Additional files
-
Transparent reporting form
- https://doi.org/10.7554/eLife.29718.011