Method for identification of condition-associated public antigen receptor sequences
Figures
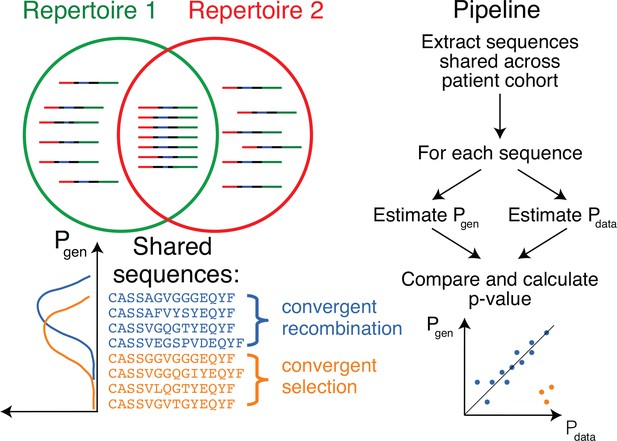
Method principle and pipeline.
(Top left) Sequence overlap between two TCR or BCR repertoires. (Bottom left) There are two major mechanisms for sequence sharing between two repertoires: convergent recombination and convergent selection. Because convergent recombination favors sequences with high generation probabilities, these two classes of sequences have different distributions of the generative probability, . (Right) We estimate the theoretical for each sequence and compare it to , which is empirically derived from the sharing pattern of that sequence in the cohort. Comparison of these two values allows us to calculate the analog of a p-value, namely the posterior probability that the sharing pattern is explained by the convergent recombination alone, with no selection for a common antigen.
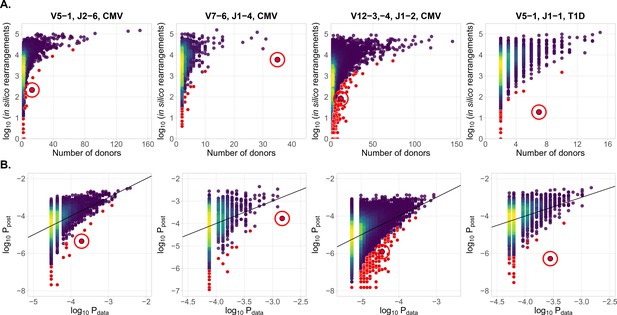
Identification of condition-associated clonotypes using generative probability
(A) CDR3aa of antigen specific clonotypes (red circles) have less generative probability than other clonotypes shared among the same number of donors. The number of in silico rearrangements obtained for each TCR sequence in our simulation (which is proportional to generation probability for each clonotype in a given VJ combination ), plotted against the number of patients with that TCR clonotype. (B) Model prediction of generative probabilities agrees well with data. To directly compare to data, we estimate the empirical probability of occurrence of sequences, , from its sharing pattern across donors (see Materials and methods). In A. and B. red dots indicate significant results (adjusted , Holm’s multiple testing correction), while red circles point to the responsive clonotypes identified in the source studies.
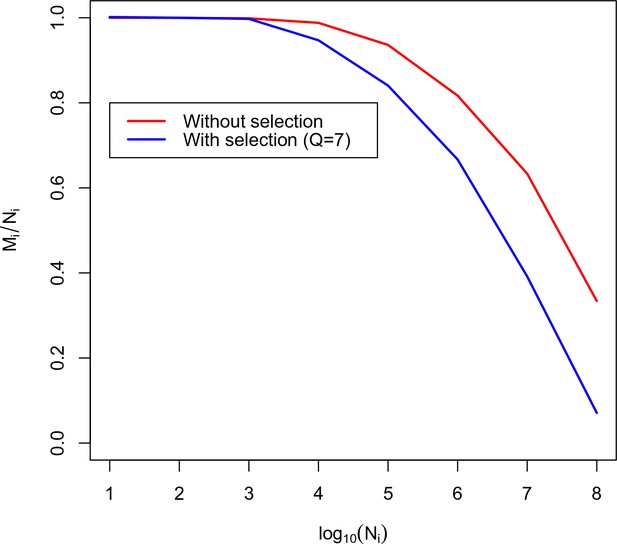
Calibration curve for TRBV5-1 TRBJ2-6 combination.
Here we plot the fraction of unique amino acid sequences to recombination events against the logarithm of the number of recombination events. The blue line corresponds to the theoretical solution with selection, the red line corresponds to the theoretical solution without selection.
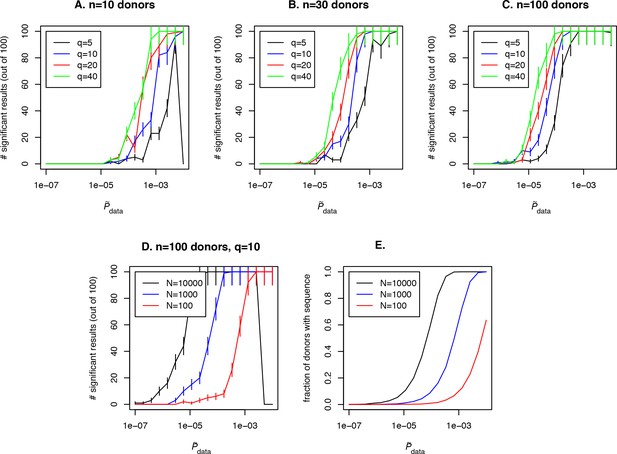
Simulation of the method performance with different cohort sizes, sequencing depths, effect sizes and target clone abundances in population.
In panels (A. B. C) we plot the number of simulations (out of 100) where a clone with a given effect size (line color, see legend) and (x-axis) is found to be significant using our approach, for cohort sizes of 10, 30 and 100 donors respectively. Larger cohort sizes and effect sizes make it possible to resolve clonotypes with lower abundance in the population. In panel (D) we show the effect of sequencing depth for fixed : larger numbers of clonotypes sequenced per donor allow us to resolve less frequent clones, since a clone of a given is detected in a larger fraction of donors (panel E).
Tables
Published antigen-specific clonotypes used to test the algorithm.
https://doi.org/10.7554/eLife.33050.005CDR3aa | V-segment | J-segment | Antigen source | Ref. |
---|---|---|---|---|
CASSLAPGATNEKLFF | TRBV07-06 | TRBJ1-4 | CMV | (Emerson et al., 2017) |
CASSPGQEAGANVLTF | TRBV05-01 | TRBJ2-6 | CMV | (Emerson et al., 2017) |
CASASANYGYTF | TRBV12-3,−4 | TRBJ1-2 | CMV | (Emerson et al., 2017) |
CASSLVGGPSSEAFF | TRBV05-01 | TRBJ1-1 | self | (Seay et al., 2016; Gebe et al., 2009) |
Output of the algorithm for sequences from Table 1.
https://doi.org/10.7554/eLife.33050.006CDR3aa | V | J | Ag.source . | p-value rank | p-value | Effect size |
---|---|---|---|---|---|---|
CASSLAPGATNEKLFF | 07–06 | 1–4 | CMV | 1/1637 | 8.8 | |
CASSPGQEAGANVLTF | 5–01 | 2–6 | CMV | 1/5549 | 42.3 | |
CASASANYGYTF | 12–3,−4 | 1–2 | CMV | 40/27669 | 28.8 | |
CASSLVGGPSSEAFF | 5–01 | 1–1 | self | 1/2646 | 524 |
Additional files
-
Transparent reporting form
- https://doi.org/10.7554/eLife.33050.008