Combined transcriptome and proteome profiling reveals specific molecular brain signatures for sex, maturation and circalunar clock phase
Abstract
Many marine animals, ranging from corals to fishes, synchronise reproduction to lunar cycles. In the annelid Platynereis dumerilii, this timing is orchestrated by an endogenous monthly (circalunar) clock entrained by moonlight. Whereas daily (circadian) clocks cause extensive transcriptomic and proteomic changes, the quality and quantity of regulations by circalunar clocks have remained largely elusive. By establishing a combined transcriptomic and proteomic profiling approach, we provide first systematic insight into the molecular changes in Platynereis heads between circalunar phases, and across sexual differentiation and maturation. Whereas maturation elicits large transcriptomic and proteomic changes, the circalunar clock exhibits only minor transcriptomic, but strong proteomic regulation. Our study provides a versatile extraction technique and comprehensive resources. It corroborates that circadian and circalunar clock effects are likely distinct and identifies key molecular brain signatures for reproduction, sex and circalunar clock phase. Examples include prepro-whitnin/proctolin and ependymin-related proteins as circalunar clock targets.
https://doi.org/10.7554/eLife.41556.001eLife digest
Like many other sea creatures, the worm Platynereis dumerilii reproduces by dispersing eggs and sperm in the water. For these animals, timing is everything: if they fail coordinate their release, the precious reproductive cells will drift in the vastness of the ocean without ever meeting their male or female counterparts.
Internal clocks are a set of mechanisms that allow organisms to tune their internal processes to their environment. For example, the circadian clock helps many creatures to adapt to the cycle of day and night. This involves switching genes on and off according to the time of day. When a gene is activated, its information is copied into a molecule of RNA, which is then read to create proteins that will go on performing specific roles. To produce their eggs and sperm at the right time, P. dumerilii worms rely on a poorly understood internal clock which is synchronized by the moon cycle.
To investigate this ‘inner calendar’, Schenk, Bannister et al. developed a new technique that allows them to extract both RNA and proteins from the miniscule heads of the worms. The results showed that the internal clock synchronized by the lunar phases influenced the levels of many more proteins than RNA molecules. In comparison, other life events such as the worms becoming sexually mature, had a more similar impact on both protein and RNA regulation. This might suggest that the inner calendar that coordinates the worms with the moon cycle could work by changing protein, rather than RNA levels. The analysis also highlighted several molecular actors that may be essential for the worm’s inner clock to work properly. In the future, the new technique will help to dissect more finely how P. dumerilii and many other marine creatures stay synchronized with the moon, and spawn at the right time.
https://doi.org/10.7554/eLife.41556.002Introduction
Appropriate timing is essential for reproductive success, especially in organisms that reproduce via external fertilisation. In order to maximise the chances of successful mating encounters, marine animals often rely on mass spawning events that require synchronisation of behaviour and gonadal maturation, both within individuals and on a population level (Fischer, 1984; Guest, 2008; Hoeger et al., 1999; Tessmar-Raible et al., 2011).
Many species achieve this synchronisation through the interplay of multiple timing systems that operate on different time scales, ranging from months or days to hours or even minutes (reviewed in Liedvogel et al., 2011; Tessmar-Raible et al., 2011). One timing cue used for synchronised reproduction in organisms ranging from brown algae and corals to bristle worms, echinoderms and vertebrates, is provided by the changing phases of the moon (Brady et al., 2016; Coppard and Campbell, 2005; Grant et al., 2009; Kaniewska et al., 2015; Kennedy and Pearse, 1975; Oldach et al., 2017; Rahman et al., 2004; Raible et al., 2017; Saavedra and Pousão-Ferreira, 2006; Saigusa, 1988; Skov et al., 2005). Depending on the species, the predominant physical cue interpreted by organisms is either gravitation or illumination. A study in reefs at two distinct lunar time points (full moon vs. new moon) has revealed a direct impact of moonlight illumination on the transcriptome of Acropora corals, but also found that light-induced transcriptome changes are most dramatic in mature animals, when light orchestrates the actual spawning event (Kaniewska et al., 2015). While pointing at the transcriptome as a readout for organismal changes, this study therefore also illustrates that in natural samples, the molecular changes relating to illumination can be tightly interwoven with internal changes (maturation and spawning), and might in addition mask endogenous monthly oscillations as they can be produced by endogenous timing systems.
Laboratory research on animals such as the marine annelid Platynereis dumerilii or the marine midge Clunio marinus has indeed revealed evidence for such internal timers, showing that nocturnal light (in nature provided by the moon) does not only impact directly on transcription (Zantke et al., 2015), but is both necessary and sufficient to entrain endogenous monthly (circalunar) oscillators (Kaiser et al., 2016; Zantke et al., 2013), which in turn orchestrate reproduction at distinct times of the lunar month (Figure 1a).
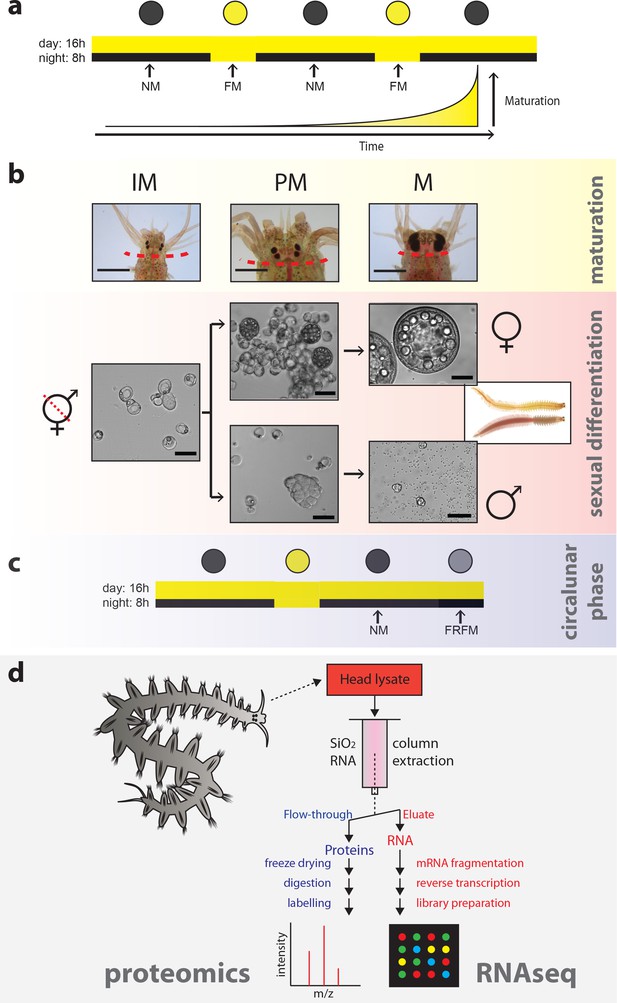
Strategy to capture the impact of maturation, sex and circalunar phase on the head transcriptome and proteome of Platynereis dumerilii.
(a) Light regime of the Platynereis culture and its correlation with maturation; daily and nocturnal light conditions are schematised. Eight consecutive nights with nocturnal light per month provide a simplified ‘full moon’ (FM) stimulus, alternating with dark nights (‘new moon’/NM) (intensity and spectra see Figure 1—figure supplement 2a–d). (b) Representative images of the different animal stages relevant for the study: IM - immature; PM - premature; M - mature. Top row: overview of heads at the respective stage; dotted line: cut site for head sampling. scale bars: 1 mm. Lower rows: representative microscopic images of coelomic cells used to diagnose each stage. Whereas immature animals display essentially only eleocytes (left), premature animals (middle) show eleocytes as well as germ cells (oocytes and spermatogonia, respectively). In mature animals (right), oocytes have reached their full size of ~180 µm in females, and male spermatogonia have differentiated into spermatozoa. Scale bars: 50 µm. (c) Scheme illustrating the two distinct circalunar phases used for sampling; NM = New Moon; FRFM = Free Running Full Moon (Zantke et al., 2014; Zantke et al., 2013). (d) Schematic flow chart of the newly developed protocol, allowing to profile the proteome and corresponding transcriptome of each sample.
-
Figure 1—source data 1
Sample IDs.
Table depicting maturation satge, sex and lunar phase of the samples included in this study.
- https://doi.org/10.7554/eLife.41556.006
-
Figure 1—source data 2
Differential expression comparisons.
Table showing the different comparions made for DET/DEP predictions.
- https://doi.org/10.7554/eLife.41556.007
In Platynereis dumerilli, sexually mature animals reproduce by engaging in stereotypical swimming behaviours classically referred to as the ‘nuptial dance’, the exact onset of which is signalled by sex pheromones and results in the coordinated release of gametes by male and female worms (Beckmann et al., 1995; Zeeck et al., 1994; Zeeck et al., 1998). Sexual dimorphisms exist in the production and response of mature worms to spawning pheromones, as well as on the level of gonadal and parapodial morphology. These differences are not obvious during the early and juvenile developmental stages, but develop within a few weeks to months prior to spawning (Figure 1a,b). The sex ratio of the worms remains constant within laboratory cultures, even under different temperature, feeding or light conditions and different population densities, consistent with genetic mechanisms underlying the sexual dimorphisms (Beckmann et al., 1995; Fischer and Dorresteijn, 2004; Schulz et al., 1989; Zeeck et al., 1994; Zeeck et al., 1998).
Studies from established molecular model systems have shown that sexual behaviour involves neurons expressing sexually-dimorphic markers, including fruitless and doublesex in Drosophila, and mab genes in Caenorhabditis (e.g. Kopp, 2012). In Platynereis, differential expression of neuropeptides in whole mature males and females suggests that sexual dimorphism at the level of hormonal regulation coincides with sexual maturation (Conzelmann et al., 2013). Given the roles of such neurohormones in other animals, it is likely that they act both locally within the brain, as well as in the periphery of the animals to orchestrate sexual dimorphism of the gonads and behaviour as the animals mature. However, no systematic information is available on sexual dimorphisms in the brain of Platynereis.
Importantly, the bristleworm brain also plays a crucial role in circadian and circalunar timing (Hauenschild, 1956a; Hauenschild, 1956b; Hauenschild, 1959; Hauenschild, 1960; Hauenschild, 1966; Hofmann, 1976; Hofmann and Schiedges, 1984; Schenk et al., 2016; Zantke et al., 2013). Its importance in reproductive timing is highlighted by the fact that tails of decapitated animals undergo sexual maturation within two weeks, but are no longer synchronised to the lunar phase (Hauenschild, 1966; Hauenschild, 1974; Schenk et al., 2016). The brain also possesses non-visual photoreceptors that likely receive light inputs for circalunar (and circadian) clock entrainment (Arendt et al., 2004; Tessmar-Raible et al., 2007; Zantke et al., 2013).
Taken together, these findings argue that the Platynereis brain integrates information on maturation, sexual differentiation, and circalunar phase in order to bring about lunar-synchronised reproduction. We therefore sought to identify the molecular signatures of these three major biological processes using an integrated molecular profiling approach, and to assess whether a circalunar component could be disentangled from the changes brought about by maturation and sexual differentiation. We carried out this analysis on two levels: First, we systematically assessed transcriptome signatures, building on our previous observation that the transcript levels of a subset of core circadian clock genes are influenced by the phase of the circalunar oscillator in Platynereis, a finding that has also been observed in corals and the reef fish Siganus guttatus (Raible et al., 2017; Altincicek and Vilcinskas, 2007 references cited therein). Second, we extended our analyses to the proteome level, reasoning that a combined quantitative transcriptomics and proteomics approach would allow us to obtain a better understanding of the major molecular changes that occur on different functional levels, and highlight molecules and pathways that are most prominent in the different processes.
This systematic approach revealed that maturation induces the largest amount of changes on both transcriptome and proteome levels, whereas the circalunar clock has a much stronger impact on the proteome than the transcriptome. Our analyses identified molecular functions, pathways and candidate genes that are diagnostic for either sex and/or maturation stage, and show that representative genes associated with these processes localise in distinct brain regions. We further identify new factors that are subject to circalunar clock-dependent changes on mRNA and/or protein level, and map their locations in the adult brain. Specifically, we demonstrate that Ependymin-related proteins (ERPs) are strongly affected on both transcript and protein levels by maturation and circalunar clock phases. We further show that the neuropeptide Whitnin/Proctolin, which recently has been correlated with the spawning event in the semilunar spawner abalone Haliotis asinina (York et al., 2012), is also regulated by the circalunar clock in Platynereis.
Taken together, our work provides a comprehensive co-evaluation of transcriptomic and proteomic regulation of stage, sex, and circalunar phase-matched tissue samples, and provides the first systematic insight into the distinct genes and molecular processes influenced by maturation, sexual differentiation and the circalunar clock in adult Platynereis dumerilii.
Results
In order to gain first systematic insight into the molecular impact of the circalunar clock in the brain, we conducted a comprehensive analysis of changes in the Platynereis head at two distinct phases of the circalunar clock: new moon (NM) and free-running full moon (FRFM). The environmental conditions are the same at both circalunar phases, FRFM describes the chronobiological conditions in which the animals would normally be presented with a nocturnal light stimulus (‘full moon’- FM), which is however not given (compare Figure 1a vs. c). Thus, their endogenous circalunar clock is under free-running conditions (i.e. FRFM, see also Zantke et al., 2013). In order to disentangle these respective circalunar molecular changes from those primarily related to maturation or sexual differentiation, we performed this analysis separately for male and female worms, across three stages of maturation (Figure 1b, Figure 1—figure supplement 1). We started our analysis with gene expression profiling by RNA sequencing (RNA-Seq), to assess effects on the transcriptome. As outlined further below, we developed an extraction method that allowed us to subsequently complement this transcriptomic analysis with a comprehensive proteomic analysis on material from the identical samples (Figure 1d, Figure 1—figure supplement 2).
A maturation-stage and sex-locked lunar head transcriptome
As the current Platynereis dumerilii genome assembly is not yet optimal for unique mapping of short reads for quantitative analyses, we generated a mapping resource based on transcript data. Whereas previous Platynereis transcriptomes were generated from whole animals during various adult life stages (Conzelmann et al., 2013), or early developmental stages (Chou et al., 2016), we reasoned that this might result in under-representation of head-specific transcripts. Moreover, when using whole animal samples, the increase in germ cells during maturation (see e.g. Schenk and Hoeger, 2010; Schenk et al., 2016 and references cited therein) could obscure low abundance expression, which might be of regulatory importance.
Therefore, we generated a head reference transcriptome assembled from RNA-Seq data combined from heads sampled under various circalunar conditions and maturation stages (see Materials and methods). The FRFM condition refers to animals kept in a standard daily 16:8 hr light dark (LD) light cycle whose circalunar clock was previously entrained by nocturnal light for at least two months, but are not exposed to nocturnal light at the expected full moon time when sampling was performed. Such ‘free-running’ conditions can thus reveal effects solely caused by the endogenous circalunar clock (Zantke et al., 2013 and Figure 1c).
Our primary assembly (Pdu_HeadRef_TS_v1) was systematically curated to (i) remove redundancies, (ii) merge scaffold information provided by published Platynereis sequences, (iii) remove sequence contaminations (e.g. prokaryotic), and (iv) flag additional sequences with high similarity to other species (see Materials and methods section), resulting in our final reference sets (Pdu_HeadRef_TS_v4 and v5, see Figure 2, Figure 2—source data 1–3 and Materials and methods section).
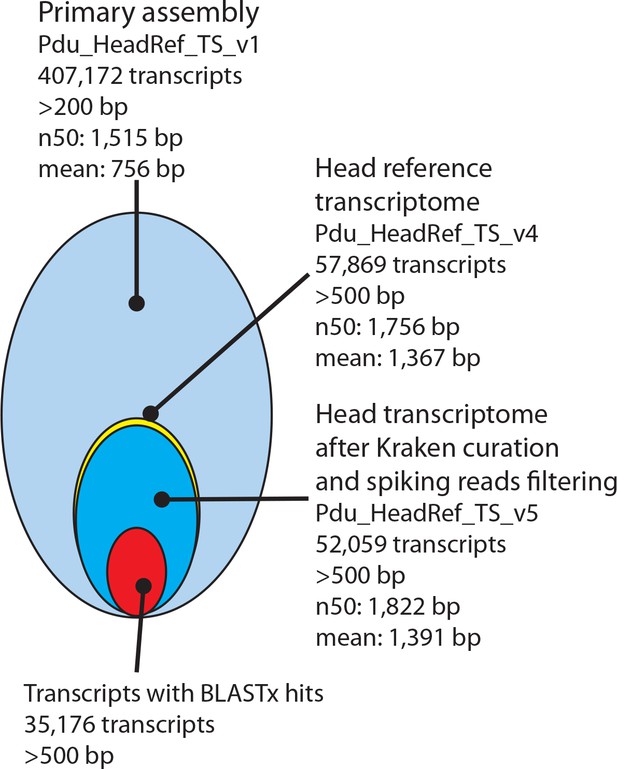
Processing and basic features of the Platynereis head transcriptome.
The primary assembly consisting of 407,172 transcripts with >200 bp was successively processed by: i) collapsing multi-copy transcripts to the longest transcripts of each cluster, ii) removing transcripts shorter than 500 bp, ii) removing transcripts arising from common model organisms or potential food sources, iv) substituting less complete transcripts with previously published sequences from NCBI and the transcriptome published by Conzelmann et al. (2013), and v) adding missing sequences from NCBI. In total 35,176 Pdu_HeadRef_TS_v5 sequences have BLASTx hits against the NCBI nr- database.
-
Figure 2—source data 1
Excluded transcripts.
Table containing the IDs of the 5,727 prokaryotic contaminats present in the TS_v4 as identified by Kraken; which were excluded from TS_v5 prior to differential expression analysis.
- https://doi.org/10.7554/eLife.41556.009
-
Figure 2—source data 2
Platynereis head reference transcriptome v1 base annotation.
Annotation of TS_v1, including blastn, blastx, GO-term and THMM predictions.
- https://doi.org/10.7554/eLife.41556.010
-
Figure 2—source data 3
Platynereis head reference transcriptome v4 interpro annotation.
Interpro annotation of TS_v4, including blastx-hist, GO-terms, and KEGG-terms.
- https://doi.org/10.7554/eLife.41556.011
-
Figure 2—source data 4
Transcriptome ENA accession numbers.
Full list of European Nucleotide Archive accession numbers for all sequencing raw data and transcriptome assemblies from this study.
- https://doi.org/10.7554/eLife.41556.012
To identify gene sets that were regulated by either one of the three sampled parameters (circalunar clock phase, maturation, and sex), and assess to which extent these gene sets were distinct, we performed differential expression analyses using DESeq2 (v1.10.0, Love et al., 2014) and EdgeR (v3.12.0, Robinson et al., 2010) (Figure 3a, b, Figure 3—figure supplements 1–9, Figure 1—figure supplement 1, Figure 1—source datas 1 and 2, Figure 3—source datas 1 and 2). Transcripts passing a Benjamin-Hochberg (BH) false discovery rate (FDR) of 10% were considered as significant (FDR, Benjamini and Hochberg, 1995). To compare the results of both methods, rank sum files were generated to combine the results of both DESeq2 and EdgeR differential expression testing, to yield the final lists of differentially expressed transcripts (DETs) for each condition (Figure 3—source datas 3, 4, 5, 9, 10 and 11). Of the three major comparison groups, that is maturation, sex biased and circalunar, maturation exhibits the largest number of DETs (Altincicek and Vilcinskas, 2007); 16.52% of all transcripts, Figure 3a). Sexual differentiation was characterised by 640 DETs (1.23%), whereas 63 DETs (0.12%) appear to distinguish the two sampled phases of the circalunar clock. Of the 640 sex-biased DETs, 149 (23.28%) were also regulated by maturation and six (0.94%) by the circalunar phase (Figure 3—source data 3–5); of the 63 circalunar DETs 34 (53.97%) were also regulated by maturation. Four transcripts were regulated in all three parameters (Figure 3a).
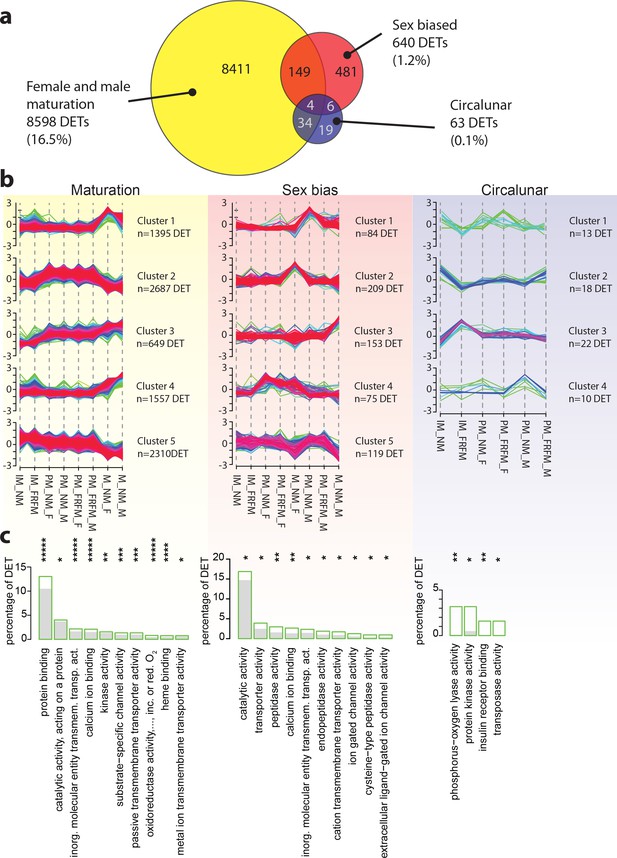
Maturation, sex and circalunar phase have distinct transcriptomic signatures in Platynereis heads.
(a) Venn diagram showing the overall numbers of genes identified as significantly regulated within and across the three different biological processes. Percent in brackets indicate the respective fraction compared to total number of analyzed IDs. (b) Soft clustering of differentially expressed genes using the Mfuzz algorithm. Maturation (yellow) and sexual differences (light red) yielded five clusters; circalunar phase-regulated (light blue) expression was best represented by four clusters. Compare to Figure 5b for similarity to regulated protein clusters. (c) Results from the GO-term enrichment analysis using the GOStats package on the differentially expressed genes in each comparison; the ten most abundant terms in the Molecular Function category are displayed (for more GO-results see Figure 3—figure supplement 1–9). Green boxes: actual percentage of differentially expressed transcripts for each term; grey bars: expected number of transcripts per category. Maturation: protein binding: GO:0005515, catalytic activity, acting on a protein: GO:0140096, inorganic molecular entity transmembrane transporter activity: GO:0015318, calcium ion binding: GO:0005509, kinase activity: GO:0016301, substrate-specific channel activity: GO:0022838, passive transmembrane transporter activity: GO:0022803, oxidoreductase activity, acting on paired donors, with incorporation or reduction of molecular oxygen: GO:0016705, heme binding: GO:0020037, metal ion transmembrane transporter activity: GO:0046873. Sex bias: catalytic activity: GO:0003824, transporter activity: GO:0005215, peptidase activity: GO:0008233, calcium ion binding: GO:0005509, inorganic molecular entity transmembrane transporter activity: GO:0015318, endopeptidase activity: GO:0004175, cation transmembrane transporter activity: GO:0008324, ion gated channel activity: GO:0022839, cysteine-type peptidase activity: GO:0008234. Circalunar phase: phosphorus-oxygen lyase activity: GO:0016849, protein kinase activity: GO:0004672, insulin receptor binding: GO:0005158, transposase activity: GO:0004803. Statistical significance was tested with a hypergeometric G-test implemented in the GOStats package. *p < 0.05, **p < 0.01, ***p < 0.001, ****p < 0.0001, *****p < 0.00001.
-
Figure 3—source data 1
Platynereis head reference transcriptome v5 read counts.
Table containing the raw read count data for TS_v5. This table is used as input for differential expression analysis.
- https://doi.org/10.7554/eLife.41556.024
-
Figure 3—source data 2
Transcriptome: DESeq normalised read counts.
Normalised read counts of TS_v5 as calculated by the DESeq2 algorithm; used to build DET expression graphs.
- https://doi.org/10.7554/eLife.41556.025
-
Figure 3—source data 3
Platynereis head reference transcriptome v5 gene universe.
Table containing the "gene universe" for the TS_v5 used as background for GO-term enrichment analysis in Figs. 3 and S3a-k.
- https://doi.org/10.7554/eLife.41556.026
-
Figure 3—source data 4
Transcriptome: DESeq output tables.
File containing DESeq predicted DETs for all 14 comparision made. Each comparison is contained within a separate tab.
- https://doi.org/10.7554/eLife.41556.027
-
Figure 3—source data 5
Transcriptome: EdgeR output tables.
File containing EdgeR predicted DETs for all 14 comparision made. Each comparison is contained within a separate tab.
- https://doi.org/10.7554/eLife.41556.028
-
Figure 3—source data 6
Transcriptome: DESeq2-EdgeR rank sum files.
This file contains DESeq2-EdgeR rank sums for predicted DETs for all 14 comparision made plus the all maturation rank sum predictions. Each comparison is contained within a separate tab. Note that while DESeq2 calculates the log2 fold change as A/B; EdgeR calculates the log2 fold change as B/A. log2FoldChange: DESeq2; log2FC: EdgeR; padj: BH-adjusted p-value DESeq2; FDR: BH-adjusted p-value EdgeR.
- https://doi.org/10.7554/eLife.41556.029
-
Figure 3—source data 7
Transcriptome: Mfuzz cluster gene IDs with cluster membership values.
This file contains the soft clustering output of RNA expression values with Mfuzz. Each comparison, maturation, sex, and lunar contains the individual transcript ID and the membership values for each cluster ranging from 0 to 1.
- https://doi.org/10.7554/eLife.41556.030
-
Figure 3—source data 8
Transcriptome: all over- and under-represented GO-Terms from all comparisions.
Table containing the results of the GO-term analysis on transcript level. Individual GO-terms are shown with their respective p-values in each of the three comparisons made (maturation, sex, and lunar), as well as their ontolgy, i.e. Molecular Function, Biological Process, or Cellular Compartment.
- https://doi.org/10.7554/eLife.41556.031
-
Figure 3—source data 9
Transcriptome: over- and under-represented GO-Terms maturation comparison.
This file contains the over- and under-representet GO-terms for the maturation comparison on transcript level. Tabs for each of the three ontologies, i.e. Molecular Function, Biological Process, and Cellular Compartment.
- https://doi.org/10.7554/eLife.41556.032
-
Figure 3—source data 10
Transcriptome: over- and under-represented GO-Terms sex bias comparison.
This file contains the over- and under-representet GO-terms for the sex comparison on transcript level. Tabs for each of the three ontologies, i.e. Molecular Function, Biological Process, and Cellular Compartment.
- https://doi.org/10.7554/eLife.41556.033
-
Figure 3—source data 11
Transcriptome: over- and under-represented GO-Terms circalunar comparison.
This file contains the over- and under-representet GO-terms for the lunar comparison on transcript level. Tabs for each of the three ontologies, i.e. Molecular Function, Biological Process, and Cellular Compartment.
- https://doi.org/10.7554/eLife.41556.034
-
Figure 3—source data 12
Transcriptome: over-represented GO-Term unique for any of the three comparisons.
This file contains those over-represented GO-terms which are unique to a given comparison on transcript level, i.e. maturation, sex, or lunar, with p-values for each of the three comparision.
- https://doi.org/10.7554/eLife.41556.035
-
Figure 3—source data 13
All transcripts significantly regulated during maturation (maturation DETs).
This file contains the IDs, mean expression values, top hit by blastX with e-value cut-off 10-4, e-value corresponding to blast hit, number of identical/ similar AA or gaps in blast alignment ("identity", "positives", "gaps") for all transcripts significantly regulated during maturation.
- https://doi.org/10.7554/eLife.41556.036
-
Figure 3—source data 14
All transcripts significantly regulated during sexual differentiation (sex DETs).
This file contains the IDs, mean expression values, top hit by blastX with e-value cut-off 10-4, e-value corresponding to blast hit, number of identical/ similar AA or gaps in blast alignment ("identity", "positives", "gaps") for all transcripts significantly regulated between males and females.
- https://doi.org/10.7554/eLife.41556.037
-
Figure 3—source data 15
All transcripts significantly regulated between circalunar phases (lunar DETs).
This file contains the IDs, mean expression values, top hit by blastX with e-value cut-off 10-4, e-value corresponding to blast hit, number of identical/ similar AA or gaps in blast alignment ("identity", "positives", "gaps") for all transcripts significantly regulated between circalunar phases.
- https://doi.org/10.7554/eLife.41556.038
To further validate our quantitative sequencing results, we selected transcripts from the top 50 ranked DETs for additional qRT-PCR analyses, preferring those that also had evidence on the protein level (see below). These experiments showed that the differences observed in our sequencing approach generally validated well when assessed by qRT-PCR on independent samples (Figure 4a–d, Figure 4—figure supplement 1). Within these experiments, the tested circalunar candidates appeared more variable (Figure 4—figure supplement 1). We noted that the majority of circalunar candidate DETs exhibited generally lower overall expression levels (Figure 4—figure supplement 1) and showed additional susceptibility to strain specific polymorphisms or phase differences in their circalunar rhythm profiles, similar to previous findings (Zantke et al., 2014).
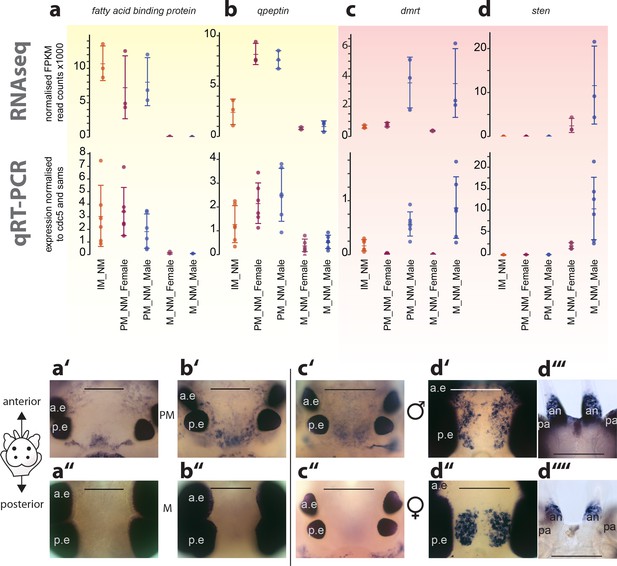
Exemplary validation of candidate genes by qRT-PCR and expression domains in the head.
(a–a’’,b–b’’) Maturation markers fatty acid binding protein and qpeptin. (c–c’’,d–d’’) Sexual differentiation markers dmrt and sten. (a–d): qRT-PCR validation of the candidates relative to the arithmetic mean of the reference genes cdc5 and sams. All graphs show the arithmetic mean with standard deviation, and the individual data points. (a’–d’’) Whole mount in situ hybridisations of the respective genes, comparing premature animals (PM, (a’,b’) with mature animals (M, a’’,b’’), and males (c’,d’,d’’’) with females (c’’,d’’, d’’’), respectively. All images except d’’’and d’’’’: dorsal views, anterior to the top, scale bar: 250 µm. d’’’ and d’’’’: ventral views, anterior top, scale bar: 200 µm. Also see Figure 4—figure supplement 1 for further validations. a.e.: anterior eye; p.e.: posterior eye, an: antenna, pa: palpa.
-
Figure 4—source data 1
Expression values relative to sams and cdc5 as obtained by qRT-PCR.
This file contains the qRT-PCR expression values of the candidate genes relative to the expression of the arithmetic mean of the reference genes sams and cdc5. Each gene is contained in a separate tab.
- https://doi.org/10.7554/eLife.41556.041
The Platynereis head transcriptome reveals clear distinctions between maturation, sexual differentiation and circalunar rhythms
After confirming the overall fidelity of the sequencing experiment, we next investigated whether the specific differentially expressed gene sets fell into different regulatory subsets. For this, we analysed the expression profiles by soft clustering analysis using the Mfuzz R-package (Futschik and Carlisle, 2005; Kumar and Futschik, 2007). Mfuzz clustering on the mean expression values (details see Materials and methods) resulted in five clusters each for maturation- and sex-related DETs, and four for the circalunar DETs (Figure 3b, gene lists Figure 3—source data 6). The maturation-related DETs showed two clusters with transcripts peaking in the premature (PM) stage, two with a peak in the mature (M) stage, and one in which the expression values declined from immature (IM) over PM to M. The two most prominent clusters, with 2,687 DETs and 2,310 DETs, respectively, were those in which expression was reduced in mature animals (clusters 3 and 5 in Figure 3b), while the single cluster showing an increase in transcript levels from IM over PM to M contained the least number of transcripts (649 DETs, cluster 3).
Clustering of the sex-related DETs set yielded four clusters with different profiles. Two of these displayed peaks of transcript levels either in mature females or mature males (clusters 2 and 3, respectively), whereas the other two clusters harboured transcripts peaking either in PM and M females, or in PM and M males (clusters 4 and 1, respectively, see Figure 3b). Cluster one was less clear, exhibiting a trough of expression in mature animals of either sex.
Due to the comparatively low number of DETs in the circalunar comparison, the patterns of the circalunar-related DET clusters were less clear than for the sex and maturation related DETs. However, transcripts belonging to clusters two and three containing 22 and 18 DET of the 63DET, respectively, clearly exhibited differences between IM_FRFM compared to IM_NM, specifically a peak in IM_FRFM (cluster three) and a trough in clusters one and two (Figure 3b).
Taken together, these results suggest that the three assayed conditions – maturation, sex, and circalunar clock phase – affected largely different sets of genes and by this delineate specific brain ‘molecular signatures’. To further analyse if the individual signatures also differed with respect to functional or molecular categories, Gene Ontology annotations were analysed using the GOStats R-package (Falcon and Gentleman, 2007).
These analyses revealed that overall 239 out of a total 4,775 GO-terms were significantly enriched in our comparisons (Figure 3c; Figure 3—figure supplements 1–9, Figure 3—source data 7–11). When analysing the ten most abundant GO-terms, these confirmed the above notion of distinct signature processes for maturation, sexual differences and circalunar phase. Specifically, in the Molecular Function category, ‘Protein binding’ is the most abundant, significantly-enriched single term for maturation, whereas ‘catalytic activity’ and ‘phosphorous-oxygen lyase activity’ are most enriched for the sex-specific and circalunar expression comparisons, respectively (Figure 3c). The Biological Processes and Cellular Compartment ontologies also showed pronounced differences in significantly over- and under-represented GO-terms (Figure 3—figure supplements 1–9, Figure 3—source datas 6 and 7). We found little overlap between all the 239 significantly enriched GO-terms when sorted by our three analysis categories: 131 out of 142 were uniquely present in maturation DETs, 83 of 97 were unique to sex differences and ten of fifteen were specific for the circalunar phase DETs (see Figure 3—figure supplements 2–9 and Figure 3—source data 11). No GO-terms were shared between maturation and circalunar DETs. Taken together, these results suggest that maturation, sexual differentiation and circalunar timing – while being tightly coordinated processes - can be clearly distinguished on a molecular level and are likely driven by different biological mechanisms.
The view that these processes can be well separated in Platynereis is further supported by the exemplary whole mount in situ hybridisations (ISH): Maturation candidates tested by in situ hybridisation generally showed broadly-distributed expression over the whole brain. One prominent region was found around the posterior eye pair, where two kidney shaped regions of expression were visible, and another in the medial brain between the eyes (Figure 4a’,b’,a’’,b’’). Sex biased transcripts exhibited similarly broad expression domains, with noted dimorphism of expression domains, and/or staining intensity reflective of expression level differences between males and females (Figure 4c’,d’,c’’,d’’, d’’’, d’’’’).
Establishment of a combined transcriptomic and proteomic profiling procedure
While transcriptomic analyses provide broad-scale insights into molecular dynamics that occur on the level of mRNA regulation (transcription itself and transcript stability regulation), most physiological processes are driven by protein function, but as has been pointed out, the knowledge about the proteome changes in lunar spawning cycles is missing (Zoccola and Tambutté, 2015) We therefore complemented our transcriptomic study with a systematic proteomic analysis. This objective posed two challenges: (i) whereas general transcript analyses have been previously performed for Platynereis (Chou et al., 2016; Conzelmann et al., 2013), no comparable reference set exists yet at proteome level; (ii) we anticipated that maximal comparability between transcriptomic and proteomic data would require a method that was able to isolate both RNA and protein from the same specimen.
To minimise sampling bias and to facilitate comparisons between the transcriptome and proteome data, we took advantage of the different chemical properties of ribonucleic acid and proteins, and the fact that most of the protein is expected to elute as the flow-through from RNA extractions that use SiO2-based columns under denaturing conditions.
However, in these flow-through fractions, proteins are highly diluted and we found that the high concentrations of guanidin thiocyanate (GuSCN) impair downstream analyses (data not shown). Thus, we first established an appropriate method for concentrating the protein fraction. We freeze-dried and then reconstituted the sample in the original volume, followed by protein precipitation. Due to the presence of thiocyanate in the samples acid based precipitations were not feasible. We tested different alcohol-based precipitation protocols (details see Materials and methods), and found that a 5:1 dilution with acetone yielded the best recovery. The resulting pellets were washed thoroughly in ice-cold EtOH to remove any traces of GuSCN which may damage the filters (FASP protocol, Wiśniewski et al., 2009). We found no evidence for non-precipitated proteins in the acetone precipitation supernatant (for further details see Materials and methods) encouraging us to continue with quantitative proteomic analyses.
To enable quantitative proteomic analysis, open reading frames (ORFs) > 100 amino acids were predicted for all transcripts in the HeadRef_Tsv4 transcriptome for all six possible reading frames, resulting in 92,645 predicted protein sequences (45,065 transcripts; Pdu_HeadRef_prot_v4). In total we identified 2,290 proteins with at least two unique peptides identified per protein from our experimental samples. The number of identified proteins with these stringent criteria matches well with analyses from other invertebrate head/brain proteomes; 2,987 proteins were identified in Apis mellifera (Hernández et al., 2012) and 3,004 proteins in the larval brain of Bombyx mori (Li et al., 2016), despite the fact that both of these studies employed an unlabelled shotgun proteomics approach and required only one unique peptide match per protein.
To further compare to existing datasets, we correlated the normalised log10-transformed transcriptome and proteome expression values to each other. This showed a significant, but relatively low correlation (cor.test()) Pearson-correlation coefficient of 0.482 (Figure 5—figure supplement 1). This relatively low correlation between transcript and protein levels is highly consistent with previous transcriptome-proteome comparisons studies. Correlations with very similar R-values were found for the developmental transcriptome/proteome in Drosophila melanogaster (Casas-Vila et al., 2017) and sexual difference transcriptome/proteome comparisons in C.elegans (Tops et al., 2010). A comparison of protein and mRNA expression levels for multiple stages of C.elegans development resulted in even much lower R values (Grün et al., 2014). These (and further) studies imply that only limited conclusions about the level of protein can be drawn based on its transcript level in an organism, further emphasising the importance of proteomic comparisons. The consistency with other studies provides further evidence that our methodological approach did not negatively impact on the proteome data quality.
As final quality estimation, we took advantage of another Platynereis head proteome that was independently generated using a conventional sample processing method, a label-free approach (see Materials and methods) and did not include large-scale comparisons between different maturation- or sex-specific stages. In total, 3,847 proteins were detectable in this conventionally-processed (see Supplementary file 1), label-free proteome set, out of which 2,105 proteins overlapped with the transcriptome/proteome co-processed proteome. To test for possible large-scale systematic compositional biases caused by the different sample processing, we performed a GO-term analysis with both sets comparing to all possible annotated GO-terms and relative frequencies present in the transcriptome dataset (taking all three categories - molecular process, cellular compartment and function - into account). In the 2,290 proteins detected in the transcriptome/proteome co-processed set, 372 significantly over- and 244 under-represented GO-terms were present; whereas in the conventionally-processed set (3,847 proteins) 444 G0-terms were over- and 248 under-represented (see Figure 5—source datas 3 and 4). Overall, the types of over- and under-represented GO-terms were similar and, in the majority, overlapped (Figure 5—source data 3–5), suggesting that the transcriptome/proteome co-processing of our samples did not induce any significant bias in terms of functional complexity.
A maturation-stage and sex locked circalunar head proteome
We next analysed the proteome with respect to the different sampling conditions. To enable robust comparison between all conditions, we defined a subset of ‘quantifiable protein’ comprising only those detected uniquely in at least 2 BR and in 3 out of the 5 TR per BR for all samples, yielding a total of 1,064 proteins; a number comparable to studies of the mosquito Aedes aegypti head proteome, applying similar stringency filters (1,139 proteins, Nunes et al., 2016). We tested for significantly different protein abundances using ROTS and LIMMA (Elo et al., 2008; Elo et al., 2009; Ritchie et al., 2015) statistics and combined the results from both tests using the same rank sum approach used previously for the transcriptome DET analyses (Figure 5—source datas 6–8 and 19–21). As for the transcriptome data, the largest proportion of proteins, that is 693 proteins were differentially expressed over the process of maturation, corresponding to 65.13% of quantifiable proteins (Figure 5a). Contrary to the transcriptome profiling results, however, sexual differentiation accounted for only 17 differentially expressed proteins (DEP, 1.60%). Conversely, circalunar phase affected 261 DEP (24.53%), significantly exceeding the amount observed in the transcriptomic analysis. Of the 17 sex biased proteins, 12 were regulated during maturation, as were 177 of the 261 (67.82%) circalunar proteins. Six proteins were affected by all three processes (Figure 5a).
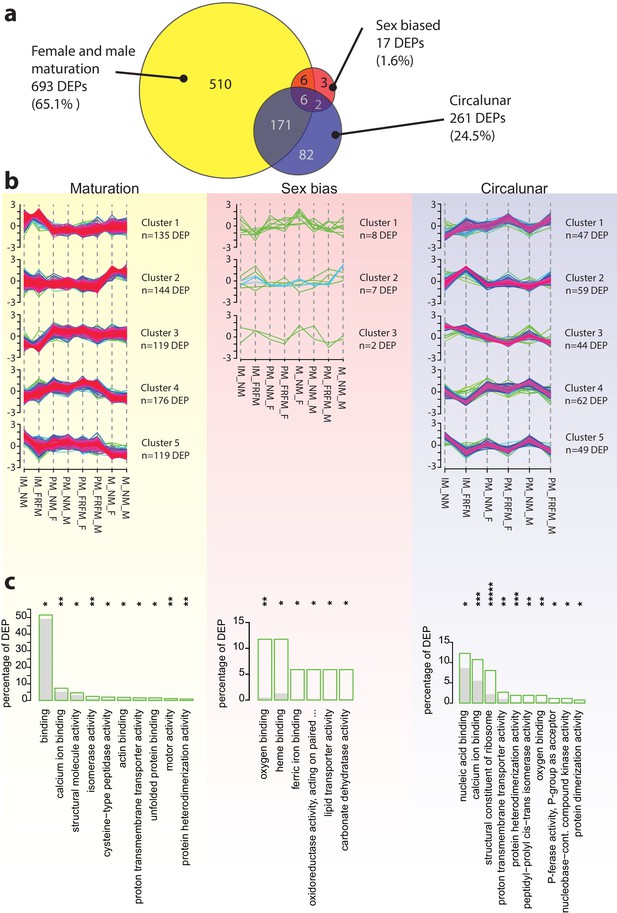
The Platynereis head proteome is differentially affected by maturation, sex and circalunar phase.
(a) Venn diagram showing the proteins significantly regulated within and across the three different biological processes in the three major comparisons made (brackets indicate respective fraction of total comparable proteins) (b) Soft clustering of differentially expressed proteins using the Mfuzz algorithm. The expression data for maturation and circalunar phase are best represented by five and for sexually dimorphic protein expression by three clusters. Compare to Figure 3b for similar dynamics in the regulated transcript clusters. (c) GO-term enrichment analysis using the GOStats package on the differentially expressed proteins in each comparison showing the ten most abundant terms in the Molecular Function category (further GO-term analyses: see Figure 5—figure supplement 2–6). Green boxes show the percentage of differentially expressed proteins for each term; the grey bars depict the expected amount of proteins per category. Maturation: binding: GO:0005488, calcium ion binding: GO:0005509, structural molecule activity: GO:0005198, isomerase activity: GO:0016853, cysteine-type peptidase activity: GO:0008234, actin binding: GO:0003779, proton transmembrane transporter activity: GO:0015078, unfolded protein binding: GO:0051082, motor activity, GO:0003774, protein heterodimerization activity, GO:0046982. Sex bias: oxygen binding: GO:0019825, heme binding: GO:0020037, ferric iron binding: GO:0008199, oxidoreductase activity, acting on paired donors, with incorporation or reduction of molecular oxygen, reduced pteridine as one donor, and incorporation of one atom of oxygen (oxidoreductase activity, acting on paired...): GO:0016714, lipid transporter activity: GO:0005319, carbonate dehydratase activity: GO:0004089. Circalunar phase: nucleic acid binding: GO:0003676, calcium ion binding: GO:0005509, structural constituent of ribosome: GO:0003735, proton transmembrane transporter activity: GO:0015078, protein heterodimerization activity: GO:0046982, peptidyl-prolyl cis-trans isomerase activity: GO:0003755, oxygen binding: GO:0019825, phosphotransferase activity, phosphate group as acceptor: GO:0016776, nucleobase-containing compound kinase activity: GO:0019205, protein dimerization activity: GO:0046983. Statistical significance was tested with a hypergeometric test implemented in the GOStats package. *p < 0.05, **p < 0.01, ***p < 0.001, *****p < 0.00001.
-
Figure 5—source data 1
Labelled proteomics: detected proteins and intensities, filtered and unfiltered.
Table contataining protein intensities and calculation of the normalised intensities for both, all 2,290 detected proteins and the BR and TR filtered final list containing 1,064 proteins used for DEP prediction. Used for DEP graphs.
- https://doi.org/10.7554/eLife.41556.050
-
Figure 5—source data 2
Platynereis head reference proteome v4 gene universe.
Table containing the "gene universe" for the Prot_v4 used as background for GO-term enrichment analysis in Figs. 5 and S6a-g.
- https://doi.org/10.7554/eLife.41556.051
-
Figure 5—source data 3
Proteome comparison: all over- and under-represented GO-Terms labelled proteomics.
Table containing the over-and under-represented GO-terms using all 2,290 proteins deteced in the labelled proteome dataset. GO-terms are shown with their respective p-values, ontology (Molecular Function, Biological Process, Cellular Compartment), and test direction, i.e. over- or under-represented.
- https://doi.org/10.7554/eLife.41556.052
-
Figure 5—source data 4
Proteome comparison: all over- and under-represented GO-Terms unlabelled proteomics.
Table containing the over-and under-represented GO-terms using all 3,847 proteins deteced in the unlabelled proteome dataset. GO-terms are shown with their respective p-values, ontology (Molecular Function, Biological Process, Cellular Compartment), and test direction, i.e. over- or under-represented.
- https://doi.org/10.7554/eLife.41556.053
-
Figure 5—source data 5
Proteome comparision: over- and under-represented GO-Terms shared between labelled and unlabelled proteomics.
Table containing the over-and under-represented GO-terms shared between the labelled 2,290 proteins and the unlabelled 3,847 proteins sets. GO-terms are shown with p-values, actual counts, expected counts, number of IDs per category for both set, respectively; as well as ontology (Molecular Function, Biological Process, Cellular Compartment).
- https://doi.org/10.7554/eLife.41556.054
-
Figure 5—source data 6
Proteome: ROTS results.
This file contains the ROTS predicted DEPs for all 14 comparision made. Each comparison is contained within a separate tab.
- https://doi.org/10.7554/eLife.41556.055
-
Figure 5—source data 7
Proteome: LIMMA results.
This file contains the LIMMA predicted DEPs for all 14 comparision made. Each comparison is contained within a separate tab.
- https://doi.org/10.7554/eLife.41556.056
-
Figure 5—source data 8
Proteome: ROTS-LIMMA rank sum files.
This file contains the ROTS-LIMMA rank sums for predicted DEPs for all 14 comparision made plus the all maturation rank sum predictions. Each comparison is contained within a separate tab.
- https://doi.org/10.7554/eLife.41556.057
-
Figure 5—source data 9
Proteome: Mfuzz cluster protein IDs with cluster membership values.
This file contains the soft clustering output of protein expression values with Mfuzz. Each comparison, maturation, sex, and lunar contains the individual transcript ID and the membership values for each cluster ranging from 0 to 1.
- https://doi.org/10.7554/eLife.41556.058
-
Figure 5—source data 10
Proteome: all over- and under-represented GO-Terms from all comparisions.
Table containing the results of the GO-term analysis on transcript level. Individual GO-terms are shown with their respective p-values in each of the three comparisons (maturation, sex, and lunar), as well as their ontolgy, i.e. Molecular Function, Biological Process, or Cellular Compartment.
- https://doi.org/10.7554/eLife.41556.059
-
Figure 5—source data 11
Proteome: over- and under-represented GO-Terms maturation comparison.
This file contains the over- and under-representet GO-terms for the maturation comparison on protein level. Tabs for each of the three ontologies, i.e. Molecular Function, Biological Process, and Cellular Compartment.
- https://doi.org/10.7554/eLife.41556.060
-
Figure 5—source data 12
Proteome: over- and under-represented GO-Terms sex bias comparison.
This file contains the over- and under-representet GO-terms for the sex comparison on protein level. Tabs for each of the three ontologies, i.e. Molecular Function, Biological Process, and Cellular Compartment.
- https://doi.org/10.7554/eLife.41556.061
-
Figure 5—source data 13
Proteome: over- and under-represented GO-Terms circalunar comparison.
This file contains the over- and under-representet GO-terms for the lunar comparison on protein level. Tabs for each of the three ontologies, i.e. Molecular Function, Biological Process, and Cellular Compartment.
- https://doi.org/10.7554/eLife.41556.062
-
Figure 5—source data 14
Lunar proteome repetion: detected proteins and intensities, filtered and unfiltered.
Table contataining protein intensities and calculation of the normalised intensities for both, all 2,092 detected proteins and the BR and TR filtered final list containing 1,671 proteins used for DEP prediction from the lunar proteome repetion experiment.
- https://doi.org/10.7554/eLife.41556.063
-
Figure 5—source data 15
Lunar proteome repetion: ROTS-LIMMA significant proteins.
This file contains the ROTS-LIMMA rank sums for predicted DEPs for the repeat lunar proteome experiment using only IM sample, containg the 1,671 filtered and analysed proteins.
- https://doi.org/10.7554/eLife.41556.064
-
Figure 5—source data 16
Lunar proteome set1-set2 ovelapping IDs ROTS-LIMMA significant proteins in set1.
This file contains the ROTS-LIMMA rank sums of DEPs predicted as significant in the first proteomics experiment (set1); using only the 1,017 proteins detected in both, the first (set1) and the second experiment (set2) as input data.
- https://doi.org/10.7554/eLife.41556.065
-
Figure 5—source data 17
Lunar proteome set1-set2 ovelapping IDs ROTS-LIMMA significant proteins in set2.
This file contains the ROTS-LIMMA rank sums of DEPs predicted as significant in the second proteomics experiment (set2); using only the 1,017 proteins detected in both, the first (set1) and the second experiment (set2) as input data.
- https://doi.org/10.7554/eLife.41556.066
-
Figure 5—source data 18
Lunar proteome: all ROTS-LIMMA significant proteins with BLASTx annotation.
This file contains all DEPs predicted as significantly circalunar different by either the first, the second, or both proteome experiments. All proteins have also a BLASTx annotions where possible. Positive identification is marked by '+' in the respective column.
- https://doi.org/10.7554/eLife.41556.067
-
Figure 5—source data 19
All proteins significantly regulated during maturation (maturation DEPs).
This file contains the IDs, mean expression values, top hit by blastX with e-value cut-off 10-4, e-value corresponding to blast hit, number of identical/ similar AA or gaps in blast alignment ("identity", "positives", "gaps") for all proteins significantly regulated during maturation.
- https://doi.org/10.7554/eLife.41556.068
-
Figure 5—source data 20
All proteins significantly regulated during sexual differentiation (sex DEPs).
This file contains the IDs, mean expression values, top hit by blastX with e-value cut-off 10-4, e-value corresponding to blast hit, number of identical/ similar AA or gaps in blast alignment ("identity", "positives", "gaps") for all proteins significantly regulated between males and females.
- https://doi.org/10.7554/eLife.41556.069
-
Figure 5—source data 21
All proteins significantly regulated between circalunar phases (lunar DEPs).
This file contains the IDs, mean expression values, top hit by blastX with e-value cut-off 10-4, e-value corresponding to blast hit, number of identical/ similar AA or gaps in blast alignment ("identity", "positives", "gaps") for all proteins significantly regulated between circalunar phases.
- https://doi.org/10.7554/eLife.41556.070
We are aware that transcriptomic versus proteomic comparisons are of course different in that RNA sequencing will allow to quantify most transcript types present in a given sample, whereas quantitative proteomics can at present only measure the most abundant proteins present in this sample. This likely explains the difference in the number of sex-specific DETs vs. DEPs, but not the difference observed in the numbers of circalunar DETs vs. DEPs.
To further assimilate the proteomic data with the transcriptomic profiles, we performed the same type of soft cluster analysis of the significant DEPs using the Mfuzz algorithm (Futschik and Carlisle, 2005; Kumar and Futschik, 2007) to assess if co-regulated clusters existed for the respective processes. Maturation as well as circalunar DEPs each clustered robustly into five protein expression clusters, whereas the sex-specific DEPs showed only weak associations to three clusters (Figure 5b, gene lists Figure 5—source data 9). A comparison between protein and RNA expression clusters for the maturation process revealed similar dynamic groups (Figure 3b vs. 5b), however, these were not necessarily populated by corresponding transcripts and proteins, as only 40.12% (278 out of 693) of DETs showed a similar profile of regulation on the protein level. This finding suggests that transcript to protein changes are ‘offset’ in time for the majority of the transcripts. Comparisons between DET and DEP clusters were less robust for the sex-specific and circalunar categories, given that only few DEPs or DETs were identified, respectively.
GO enrichment analysis of the DEPs showed that out of a total of 2,448 GO terms present in all 2,290 detectable proteins, 121 GO terms were enriched. Of these, 75 (62.5%) GO terms were found to be enriched in maturation DEPs, 17 in sex biased DEPs, and 46 in circalunar DEPs. Like for the transcriptome, there was little overlap between the GO term enrichments across the three different analysed biological processes (Figure 5c, Figure 5—figure supplements 2–6, Figure 5—source data 10–13).
Additional proteomic analyses confirm the large change in protein abundance between different circalunar phases
In our initial proteomic analysis for differences between NM and FRFM stages we discovered a much higher amount of differentially expressed proteins than we would have expected based on the transcriptome data (0.12% DET vs. 24.53% DEP, Figures 3a and 5a). This difference is specific for the circalunar comparison and not present or opposite for the maturation and sex-biased comparisons, respectively (for further interpretation and discussion- see discussion section below). In order to verify that this result was not caused by sampling, extraction or measurement artefacts we repeated the proteomics analysis using independent samples. We limited this analysis to IM stages, which showed a large proportion of differences between circalunar phases and are less affected by sexual differentiation and maturation.
This second round (filtered as above) led to a set of 1,671 quantifiable proteins (Figure 5—source data 14), out of which 1,017 were also present in the initial (1,064 quantifiable proteins) dataset, thus 95.58% of the proteins initially passing our stringent quality filter criteria again passed these criteria, indicating a high reliability and reproducibility of protein detection for this sample type. Of the 1,671 proteins of the new dataset, 173 (10.35 %, Figure 5—source data 15) were significantly differentially expressed. Re-analysing the dataset by using only the 1,017 quantifiable proteins shared between the first and second dataset resulted in 64 DEPs (testing was limited to IM animals, see above) comparable to the 70 DEPs in IM animals in the first analysis (see Figure 5—source datas 8, 16 and 17).
To identify commonly regulated proteins in all tested proteome datasets, irrespective of frequently occurring sequence differences (e.g. due to isoforms or alleles), which could obscure identical ID-calling, we ran BLASTx against the Uniprot DB for all 365 circalunar DEPs identified from both proteomic analyses (i.e. the initial circalunar IM, PM_Female and PM_Male sets and the second IM set) and grouped these according to their BLASTx annotations. We then manually inspected the DEPs for similar identities. Protein groups with similar identities or individual proteins that were either regulated in at least one dataset of the first proteomic analysis and the second proteomic analysis or in all three conditions (IM, PM male, PM female) of the first proteomic analysis (only one –IM - condition was tested in the second proteomic analysis) were marked as candidates.
This resulted in 27 protein groups (i.e. identified proteins with related, but not identical ID) and 29 individual protein IDs, which were repeatedly detected in the different quantitative proteomic comparisons (Figure 5—source data 18).
Finally, we tested if the high numbers of circalunar regulated proteins could be explained by the lower number of proteins compared to the high transcriptome number, which reduces the stringency of the Benjamini Hochberg multiple testing algorithm (Benjamini and Hochberg, 1995) used to calculate the FDR. We tested how many transcripts are significantly regulated, if we focus the analyses on the top 5,000 or 1,000 expressed transcripts. For the 5,000 most highly expressed transcripts we found 20 circalunar DET (0.40% compared to 0.12% in the complete transcriptome set), a 3.3-fold enrichment; 262 sex different DET (5.24% compared to 1.22% in the complete transcriptome set), a 4.3-fold enrichment and 2,435 maturation regulated DET (48.70% compared to 16.52% in the complete transcriptome set), a 3.3-fold enrichment. For the ,1000 most highly expressed transcripts we found four circalunar DET (0.40% compared to 0.12% in the complete transcriptome set), a 3.3-fold enrichment; 52 sex different DET (5.20% compared to 1.22% in the complete transcriptome set), a 4.3-fold enrichment and 546 maturation regulated DET (54.60% compared to 16.52% in the complete transcriptome set), a 3.3-fold enrichment. This means that statistical differences (due to overall lower numbers tested) can explain the overall increase in the maturation regulated proteins, but cannot explain the about 245-fold increase we observe between circalunar transcriptome and proteome.
Characterisation of circalunar proteome regulated candidates reveals ependymin-related genes and prepro-whitnin as putative circalunar clock phase signalling molecules
We next started to characterise several circalunar DEP candidates and provide a showcase of five of them. To gain a better understanding as to where these proteins are synthesised and possibly active, we analysed their expression in adult heads. These expression analyses are based on whole mount in situ hybridisations, which we think is justified to do as we were interested in their place of origin.
A larger group that caught our attention consisted of iron binding proteins. We selected two of these for validation: Myohaemerythrin (myoHr), and Somaferritin. MyoHr is a representative of the Haemerythrins, small oxygen-binding non-haem iron proteins present in all phyla except for in Deuterostomes; myoHr in particular appears to be restricted to marine invertebrates (Coates and Decker, 2017; Costa-Paiva et al., 2017). Ferritins are intracellular iron storage proteins, which additional to their role iron metabolism have been implicated in oxidative stress resistance and immunology (Altincicek and Vilcinskas, 2007; Andrews et al., 1992; Pham and Winzerling, 2010).
Another circalunar DEP that caught our attention was annotated as Whitnin precursor. Whitnin is the mollusc orthologue of the insect neuropeptide Proctolin (Veenstra, 2010, Figure 7—figure supplement 1, Figure 7—source datas 1 and 2). Of note, prepro-whitnin was shown to be differentially regulated on transcript level in the nervous system of abalone Haliotis asinina over the course of two weeks. Maximal expression occurred just prior to the semi-circalunar spawning events of this organism (York et al., 2012). Whitnin is thus a potential candidate for relaying the circalunar signal to other cells. The last two DEPs we focused on both belong to the large family of Ependymin-related proteins. These are highly sequence-related and could either represent different alleles of the same gene or are the result of a recent gene duplication event (Figure 6a, Figure 6—figure supplement 1, Figure 6—source datas 1 and 2).
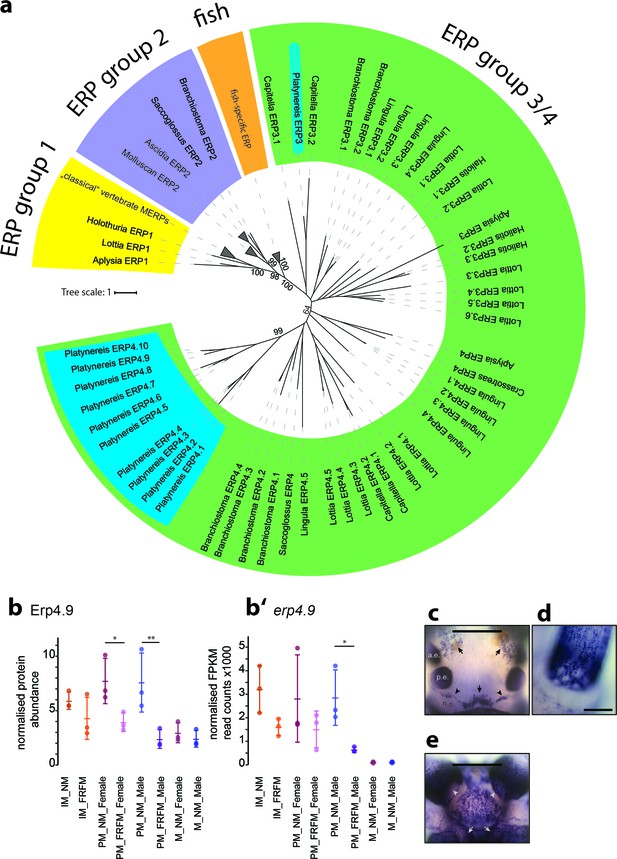
Members of a lineage-specific expansion of Ependymin-related proteins are subject to circalunar regulation on RNA and protein level.
(a) Unrooted maximum likelihood phyolgenetic tree of Ependymin-related proteins based on the analysis published by Suárez-Castillo and García-Arrarás, 2007 including eleven of 15 Platynereis Ependymin-related proteins (ERP4.1–4.10; ERP3; blue boxes) and various additional invertebrate Ependymin sequences. The phylogeny reveals four distinct groups of Ependymin-related proteins (ERP) that are termed ERP1, ERP2, ERP3/4 and fish-specific ERPs; group-specific expansions of ERPs is observed at several places in the phylogeny. All identified Platynereis Ependymins (blue) fall into the ERP3/4 clade. A full tree with un-collapsed nodes, the alignment, NCBI accession numbers and the Platynereis sequence identifiers are provided in Figure 6—figure supplement 1 and Figure 6—source datas 1 and 2. (b,b’) Protein and RNA-Seq expression profiles validate Platynereis ERP4.9 as a new target of circalunar phase on RNA and protein level. (b) Normalised protein expression profile; (b’) DESeq2 normalised RNA expression profile. The graphs show the arithmetic mean with standard deviation, together with individual data points. (c–e) RNA expression pattern of erp4.9 in immature adult Platynereis heads. a.e.: anterior eye; p.e.: posterior eye; n.o.: nuchal organ. (c) Dorsal view, anterior to the top. Characteristic expression is observed around the dorsal blood vessel (central arrow), anterior of the nuchal organ (arrowheads) and next to the anterior eyes (arrows). Scale bar: 250 µm. (d) Dorsal view of the base of a prostomial cirrus, scale bar: 50 µm. (e) Ventral view, anterior to the top. Expression is observed in a plate-like structure at the bottom of the head, as well as around the mouth opening (arrows) and the palps (arrow heads). Scale bar: 250 µm. For additional circalunar-regulated ERPs see Figure 6—figure supplement 2 and Figure 5—source datas 16 and 17.
-
Figure 6—source data 1
Ependymins alignment.
Fasta-file containing the ependymin protein alignement; used for the ERP trees in Figs. 6 and Figure 6-figure supplement 1.
- https://doi.org/10.7554/eLife.41556.074
-
Figure 6—source data 2
Ependymins alignment sequence IDs/accession numbers.
Table containing the TS_v5 IDs for the Platynereis Ependymins as well as the NCBI IDs for the other ERPs.
- https://doi.org/10.7554/eLife.41556.075
Ependymin-related proteins (ERPs) are a group of small extracellular glycoproteins originally identified as one of the most abundant proteins in teleost-fish cerebrospinal fluid (Shashoua, 1991; Suárez-Castillo and García-Arrarás, 2007). Later, ERPs were discovered in other vertebrates and due to their complete absence from all ecdysozoan genomes and transcriptomes analysed so far, were considered to be vertebrate specific (Suárez-Castillo and García-Arrarás, 2007; Andrews et al., 1992) references cited therein). However, recently ERPs have been identified in an increasing number of non-ecdysozoan invertebrates, including echinoderms, hemichordates, molluscs, annelids and even cnidarians (Hall et al., 2017; Suárez-Castillo and García-Arrarás, 2007). A re-occurring feature of ERPs are their multiple group-specific gene duplications (Hall et al., 2017; Suárez-Castillo and García-Arrarás, 2007). This can be on a higher level - such as the teleost-specific or echinoderm-specific multiplications, but also on the level of species (groups), as pointed out for the Acanthaster planci species group (Hall et al., 2017), or as we also observed in Platynereis dumerilii. We identified 15 ependymin-like sequences in the transcriptome, most of which cluster together in one specific subgroup (Figure 6a, Figure 6—figure supplement 1, Figure 6—source datas 1 and 2). Seven Pdu-ERPs were detected in the proteome, all of which were significantly differentially abundant between the two circalunar phases (Figure 5—source data 18). Of those, two showed correlated changes between transcript and proteome level (Figure 6b,b’ and Figure 6—figure supplement 2) and were subsequently investigated for their expression patterns.
Both Pdu-erps exhibited identical patterns, covering sensory appendages of the head; palpae and peristomial cirri, a large expression domain on the ventral side of the head, as well as an anterior and posterior domain in the dorsal brain (Figure 6c–e, Figure 6—figure supplement 2). The other investigated circalunar candidates exhibited overall different expression domains (Figures 6c–e and 7): Pre-pro-whitnin is expressed in four main regions. Besides expression in single cells of the peristomial cirri, it is expressed in two areas located in the medial forebrain (Figure 7a’,a’’), and one small paired cluster slightly anterior to and below the anterior pair of adult eyes (Figure 7a’’’).
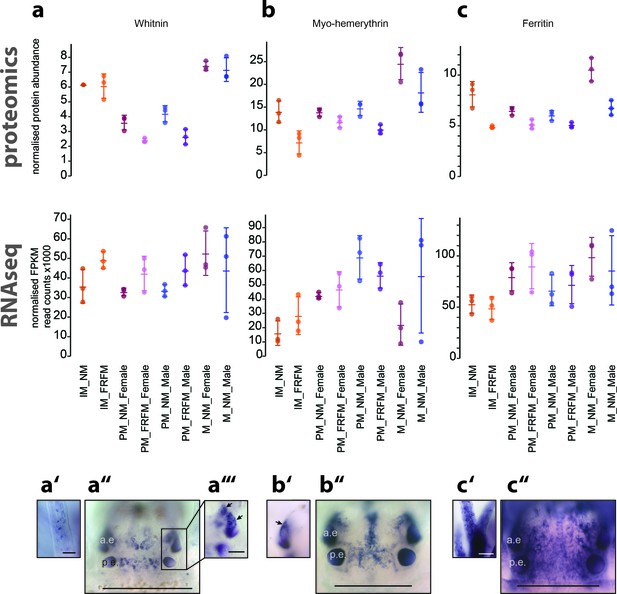
Proteome analyses reveal additional molecular markers for circalunar phase with distinct expression profiles and proposed functions.
(a–a’’’) The neuropeptide Whitnin, a’- peristomeal cirrus, a’’- head, a’’’- indicted head expression in a’’ with different focus. (b–b’’) The oxygen storage protein Haemerythrin, b’’- head, b’- lateral head aspects below the focal plane of b’’. (c–c’’) The iron storage protein Ferritin. c’- peristomeal cirrus (a–c) compares the normalised protein expression profiles (top, ‘proteomics’) with the corresponding DESeq2 normalised mRNA expression profile (bottom, ‘RNA-Seq’). All graphs show the arithmetic mean with standard deviation, and the individual data points. (a’–c’’) Expression domains as characterised by whole-mount in situ hybridisation; (a’’,b’’,c’’) dorsal views of immature Platynereis heads stained with riboprobes of the corresponding transcript; All images are oriented with the anterior side to the top; scale bar: 250 µm. a.e.: anterior eye, p.e.: posterior eye.
-
Figure 7—source data 1
Whinin-Proctolin alignment.
Fasta-file containing the Whitnin-Proctolin protein alignement; used for the tree in Figure 7-figure supplement 1.
- https://doi.org/10.7554/eLife.41556.078
-
Figure 7—source data 2
Whitnin-Proctolin alignment sequence IDs/accession numbers.
Table containing the TS_v5 IDs for the Platynereis Whitnin as well as the NCBI IDs for the Whitnin/Protolins used in file Figure 7-figure supplement 1.
- https://doi.org/10.7554/eLife.41556.079
Similarly, myohr was present in the medial brain and to a lesser part around the posterior eyes (Figure 7b’b’’). Different to whitnin and myohr, ferritin showed a broad expression in the head (Figure 7c’,c’’). The fact that the expression domains are already largely divergent among these few investigated circalunar candidates, suggests that the effect of the circalunar clock is not confined to specific regions of the head or brain.
Discussion
In this study, we pioneered a combined proteomic and transcriptomic profiling approach to identify coordinated-yet specific changes associated with maturation, sex and circalunar phase in the marine annelid Platynereis dumerilii. The analyses profited from our newly established and benchmarked protocol for the isolation and subsequent quantitative analysis of RNA and protein from the same tissue sample, which does not compromise the complexity of the detected proteins. We expect this method to be widely applicable and be particularly useful for studies that are restricted by the amount of samples that can be obtained and analysed.
Using this protocol for Platynereis dumerilii, we find that ~ 17 percent of all analysed transcripts and ~66% of the analysed proteins undergo regulation during maturation. This indicates that the maturation process is the biggest driving force for changes on both transcriptome and proteome levels as worms approach the single circalunar-synchronised spawning event of their lifecycle. This finding is consistent with the fact that physiology, metabolism, body morphology and behaviour change dramatically during the maturation phase: Animals change from a benthic, solitary, and feeding life style to a sexually mature, anorexic state that prepares them for their ‘nuptial dance’, during which germ cells are released. While this process is accompanied by major morphological changes in the trunk (in particular, the generation of germ cells that amass to up to 40% of the body mass, accompanied by a deprivation of up to 60% of longitudinal muscle fibres), the head is known to be a major source of factors that orchestrate this transition (Hauenschild, 1956a; Hauenschild, 1956b; Hauenschild, 1966; Hofmann, 1976; Schenk et al., 2016). The observed changes in the head transcriptome and proteome strongly support this notion, and the recent identification of a major brain hormone component that regulates maturation onset and progression (Schenk et al., 2016) provides an interesting entry point to understand how these significant changes are hormonally regulated and conveyed to the entire body.
Maturation of Platynereis is also accompanied by a significant increase of overall eye volume (Fischer and Brökelmann, 1966). In line with the described expansion of the outer segments of the eye photoreceptors – that typically harbour the photopigments – we observed a significant increase in transcript abundance of the Platynereis r-opsin1 gene, a major opsin gene expressed in the adult eye photoreceptors (Arendt et al., 2002).
Comparing the observed transcriptome changes to the changes found in other species shows variable similarities, depending on brain regions and species. A similar study of the honeybee brain showed that ~40% of the assessed transcripts exhibited changes associated with maturation, the majority of which (~61%) were associated with caste behaviour (Whitfield et al., 2003). Other species show less pronounced changes, such as in the human neocortex, in which about 9% of the transcripts were reported to change during postnatal development (Kang et al., 2011); in zebrafish ~20% of the pituitary transcripts were differentially expressed during sexual maturation (He et al., 2014), while ~11% of the murine hippocampus transcriptome were differentially expressed during maturation (Bundy et al., 2017) and the Mediterranean fruit fly Ceratitis capitata exhibited up to 7% of head transcriptome changes during maturation (Gomulski et al., 2012).
Overall proteome changes in the brain during development/maturation show a distribution range in vertebrates from 2–8% (Ori et al., 2015; Walther and Mann, 2011), while 15–17% changed in the larval brain development of Bombyx mori and Apis mellifera, respectively (Hernández et al., 2012; Li et al., 2016).
Sexual differences in the brain transcriptome are highly dependent on the species studied. In guppies ~ 13% and in the beetle Callosobruchus maculatus ~17% of the brain`s transcripts have been described to be sex biased (Sharma et al., 2014; Immonen et al., 2017); while in birds around ~2% of the brain transcripts (Naurin et al., 2011) and human brain ~1.1% of the transcripts show a sex bias (Kang et al., 2011).
While the observed transcriptome and proteome changes in Platynereis dumerilii heads during development, growth and maturation and brain transcriptome changes between different sexes are thus within the range of expectations, we somewhat unexpectedly found that the circalunar phase has little impact on transcript expression changes, but more prominently affects protein level changes. Specifically, we find that only 0.12% of the transcripts we analysed are regulated. This is more than 20-fold less than what has been shown as an impact of the circadian clock on the regulation for brain/head transcriptome in several animals, for example Aedes aegypti (6.7%, Leming et al., 2014), Drosophila melanogaster (2.8%, Hughes et al., 2012) and Mus musculus (3–4%, Zhang et al., 2014). Directly equivalent studies on head/brain proteomes have not been performed, instead specific central nervous system parts of mice and rats have been investigated. For the murine suprachiasmatic nucleus, 13–20% of the identified and quantifiable proteins (871 and 2,112, respectively) exhibited circadian abundance changes (Chiang et al., 2014; Deery et al., 2009), compared to about 5% of transcriptome changes in the same tissue (Ueda et al., 2002). Thus, the proteomic changes we observe between two different circalunar time points (with a similar number of quantifiable proteins) are comparable to the circadian changes observed in the nervous system of other animals.
While it is possible that we underestimate the amount of circalunar transcriptomic changes due to limited sampling resolution (only sampling two circalunar time points and one circadian time point), as well as stringent multiple comparisons statistics (see tests in result section), it is not obvious why such an underestimation effect should be different for the proteome under the same conditions. Alternatively, it is also possible that the level of variability is higher in the transcriptome than in the proteome, which – due to the higher experimental noise - would lead to a lower number of detected transcripts compared to the proteome. However, we do not see evidence for such a scenario in our data.
We therefore suggest as the most parsimonious explanation that we underestimate the occurring circalunar changes in both transcriptome and proteome, but that the observed relative difference is representative. This implies that, differing from the known circadian clock studies, the circalunar oscillator has a significantly stronger impact on the proteome than on the transcriptome. Thus, the mechanisms responsible for circalunar reproductive timing possibly operate and might be coordinated more strongly at post transcriptional, translational/post-translational level(s), than on transcript level(s).
Materials and methods
Reagent type (species) or resource | Designation | Source or reference | Identifiers | Additional information |
---|---|---|---|---|
Strain, strain background (Platynereis dumerilii, immature) | IM_NM; IM_FRFM | MFPL Fish and Marine Facility | NA | NA |
Strain, strain background (Platynereis dumerilii, Female) | PM_NM_F; PM_FRFM_F; M_NM_F | MFPL Fish and Marine Facility | NA | NA |
Strain, strain background (Platynereis dumerilii, Male) | PM_NM_M; PM_FRFM_M; M_NM_M | MFPL Fish and Marine Facility | NA | NA |
Recombinant DNA reagent | pJet1.2 (plasmid) | Thermo Scientific | Thermo Scientific: K1232 | |
Sequence-based reagent | primers see Supplementary file 2 | this study | qPCR primers were designed exon-intron spanning using the Roche Universal probe library homepage (see Materials and methods section for details). Primers for cloning were designed based on sequences derived from the transcriptome assembly, the amplicon was aimed to be in the coding region of the transcript where possible, amplicons generally had a length between 500 bp and 2,000 bp. | |
Commercial assay or kit | RNeasy kit | Qiagen | Qiagen: 74106 | |
Commercial assay or kit | RNase-free DNase | Qiagen | Qiagen: 79254 | |
Commercial assay or kit | Dynabeads mRNA-purification kit | Invitrogen | Invitrogen: 61006 | |
Commercial assay or kit | RNA fragmentation kit | Ambion | Ambion: AM8740 | |
Commercial assay or kit | SuperScript VILO Kit | Invitrogen | Invitrogen: 11754050 | |
Commercial assay or kit | Mini Quick Spin DNA Columns | Roche | Roche: 11814419001 | |
Commercial assay or kit | E. coli DNA Polymerase I | Invitrogen | Invitrogen: 18010–025 | |
Commercial assay or kit | E. coli DNA ligase | Invitrogen | Invitrogen: 18052–019 | |
Commercial assay or kit | RNase H | Promega | Promega: M4281 | |
Commercial assay or kit | 5X second strand buffer | Invitrogen | Invitrogen: 10812–014 | |
Commercial assay or kit | MiniElute Reaction clean-up kit | Qiagen | Qiagen: 28004 | |
Software, algorithm | Fastqc | https://www.bioinformatics.babraham.ac.uk/projects/fastqc/ | NA | NA |
Software, algorithm | Cutadapt | https://doi.org/10.14806/ej.17.1.200 | NA | NA |
Software, algorithm | NextGenMap | http://cibiv.github.io/NextGenMap/ | NA | NA |
Software, algorithm | Trinity | https://github.com/trinityrnaseq/trinityrnaseq/wiki | NA | NA |
Software, algorithm | Cegma | https://doi.org/10.1093/bioinformatics/btm071 | NA | NA |
Software, algorithm | MUMmer | https://doi.org/10.1002/0471250953.bi1003s00 | NA | NA |
Software, algorithm | Kraken | https://doi.org/10.1186/gb-2014-15-3-r46 | NA | NA |
Software, algorithm | bedtools | https://bedtools.readthedocs.io/en/latest/ | NA | NA |
Software, algorithm | HTSeq | https://doi.org/10.1093/bioinformatics/btu638 | NA | NA |
Software, algorithm | R | https://www.r-project.org/ | NA | NA |
Software, algorithm | DESeq2 | Bioconductor | NA | NA |
Software, algorithm | EdgeR | Bioconductor | NA | NA |
Software, algorithm | LIMMA | Bioconductor | NA | NA |
Software, algorithm | ROTS | Bioconductor | NA | NA |
Software, algorithm | Mfuzz | Bioconductor | NA | NA |
Software, algorithm | GOstats | Bioconductor | NA | NA |
Software, algorithm | IQ-TREE | http://www.iqtree.org | NA | NA |
Animals
Platynereis dumerilii were housed in plastic boxes and kept in a 1:1 mixture of Natural Sea water (NSW) and Artificial Sea water (ASW, 30 ‰ Tropic Marine).
Worms were either grown in open culture rooms, or in isolated shelf systems and exposed to a circadian light regime of 16 hr:8 hr light:dark regime (Figure 1a), following classical culture conditions (Hauenschild and Fischer, 1969), with a circalunar light regime consisting of nocturnal illumination constituting full moon (FM) provided for eight nights, every 21 days (see below for detailed light conditions). To allow for constant availability of mature worms and animals are housed in culture rooms with different circalunar entrainment regimes. Animals from the in-phase (IP) rooms receive the artificial nocturnal light stimulus (‘moon’) coinciding with the time of full moon in nature; while animals from the out-phase (OP) room receive the moon stimulus when IP room animals are under new moon conditions.
For FRFM sampling, worms were transferred to culture rooms without nocturnal light (i.e. from IP rooms to OP rooms or vice versa) prior to the first night of the FM stimulus. Specifics are outlined below and summarised for all samples analysed in Figure 1—source data 1.
Animal staging and head sampling
Request a detailed protocolAnimals were sourced from multiple boxes of VIO1 mix and VIO11 mix substrains, originating from the B32134 strain (Zantke et al., 2014). Animals were staged based on the visibility of germ cells, and grouped into immature (IM, no germ cells visible), premature (PM), and epitokous non-swarming mature (M) males and females (see Figure 1b). Mature animals were defined as having undergone metamorphosis, exhibiting typical yellow (females) or red/white (male) colouration, but were not yet actively swimming (i.e. prior to spawning, Figure 1b). Large PM animals were selected for staging 3–5 days prior to sampling.
Animals were first anesthetised for 10 min in a 1:1 mixture of 7.5% (w/v) MgCl2 and ASW. Sex and stage were checked by making a small incision in the trunk wall between two parapodia and gently squeezing out a sample of the coelomic contents. Coelomic cells (eleocytes and oocytes/spermatogonia) were collected in Nereis balanced salt solution (Heacox et al., 1983) in a 96-well plate, kept on ice, and imaged using an Axiovert 200M Microscope (Carl Zeiss, Germany), with a Coolsnap HQ monochrome camera (Photometrics, Vision Systems GmbH, Austria). Staging criteria were devised to obtain male and female animals in equivalent early stages of germ cell maturation. PM males were defined as those with germ cells at late spermatogonia (Spg) I stage as defined by Meisel, 1990: characterised by the presence of many large clusters with opaque, smooth, cloud-like appearance, that may also be beginning to dissociate into smaller kidney bean-shaped clusters. PM females were defined as those with a maximum oocyte diameter of 52–75 µm (Figure 1b). Oocyte sizes were measured from images taken of oocytes extracted from individual females, using the straight line/measure tool in ImageJ (v1.5g, http://imagej.nih.gov/ij/). Measurements in pixels or inches were converted to µm according to the appropriate scale conversion for the obtained images.
After staging, animals were allowed to recover in NSW/ASW for 24–48 hr. Two days prior to head sampling worms were transferred into sterile filtered NSW containing 0.125 mg/mL ampicilin and 0.500 mg/mL streptomycin-sulphate; after 24 hr, the antibiotics concentration was reduced to 50% for approximately 16 hr, until animals were sampled the following day. For all analyses, pools of 6 heads per biological replicate were sampled at Zeitgeber time 4.
For sampling, animals were anesthetised with MgCl2 as before and the head was cut directly behind the posterior eyes, and in front of the first parapodial segment (red dotted line in Figure 1b). Cut heads were quickly rinsed in NBSS and snap frozen in liquid N2 in pools of six in 2 mL tubes containing metal beads. Heads of mature animals were frozen in pools of 2 or three to ensure more efficient RNA extraction.
Samples for initial transcriptome assembly sequencing analysis were obtained from multiple samplings performed in January - March 2014. Biological replicate samples for quantitative transcriptomics were obtained from multiple samplings between November 2014 and February 2015. Samples with best RNA concentrations and quality were selected for sample preparation for RNA-Seq and matched protein fractions were analysed for quantitative proteomics.
Spectral properties of circadian and circalunar illumination regimes
Request a detailed protocolLight spectra and intensities in the culture room were measured with an ILT950 spectrometer (International Light Technologies Inc Peabody, USA). Data were acquired over 300 ms, and 30 spectra averaged to yield one intensity spectrum, in total five measurement per condition were carried out, and the arithmetic mean of the five spectra was used. Animals grown in an ‘in-phase’ culture room were exposed to daylight stimulus of 0.0128 µWm−2 (3.4566 × 1010 photons s−2m−2) and 0.0001 µWm−2 (4.1480 × 108 photons s−2m−2) full-moon moonlight stimulus; animals grown in an ‘out-phase’ culture room were exposed to daylight stimulus of 0.0085 µWm−2 (2.3090 × 1010 photons s−2m−2) and 0.0002 µWm−2 (6.5192 × 108 photons s−2m−2). Full-moon moonlight stimulus (Figure 1—figure supplement 2a–d).
RNA and protein extraction
Request a detailed protocolFrozen heads were re-suspended in 350 µL RLT-buffer (RNeasy-Kit, Qiagen) and the head capsule was broken for 2 Min at 30 Hz in a Tissue Lyser II (Retsch GmbH). RNA was then extracted following the manufacturer`s protocol with additional on-column DNaseI digest. Finally, the RNA was eluted in 30 µL nuclease-free H2O. RNA concentrations and integrity were assessed using the Agilent 2100 Bioanalyzer (Agilent, Nanochip, Total RNA protocol).
To recover proteins from the RNAeasy column flow-through we initially collected all flow-throughs, that is RLT + RW1+RPE. As this combination has a final EtOH content of 45% it readily precipitated proteins when incubated over night at −20°C. To optimise protein recovery we then collected different combinations off column flow-throughs, namely RLT, RLT + RW1, and RLT + RW1+RPE serving as control. RLT and RLT-RW1 were then precipitated by making them either 50% in acetone, 80% in acetone, or 45% in EtOH to test for the effect of RPE on the precipitation. Analysis of the precipitates by Tricine-SDS-PAGE (Schägger and von Jagow, 1987) showed that a four-fold excess of acetone (80% final concentration) added to RLT + RW1 yielded the most quantitative result. Thus, finally the flow-throughs RLT and RW1 were collected and snap frozen in liquid N2 for protein extraction and subsequent LC-MS/MS analysis.
After protein extraction via acetone precipitation (see description in results), we controlled for the presence of non-precipitated proteins in the acetone precipitation supernatant on a monolithic C18 column and measuring the eluate at 214 nm. For this, the supernatant was dried down in vacuo, reconstituted in 0.1% trifluoro-acetic acid and concentrated on a C18 SPE column from which it was eluted with 90% acetonitrile, 0.1% trifluoro-acetic acid. The UV absorbing compounds eluted in a region typical for peptides and not for proteins.
For the multiplexing strategy of RNA-Seq and proteomic samples see Figure 1—figure supplement 2e,f.
cDNA library preparation for RNA-Seq
Request a detailed protocolThe total RNA input used for sample preparation was 700 ng-1,500ng total RNA per sample. PolyA-enriched mRNA was purified from total RNA input using the Dynabeads mRNA-purification kit (Invitrogen, #61006) according to the manufacturer`s protocol, and eluted in a final volume of 50 µL. Ribosomal RNA (rRNA) contamination was assessed by Agilent 2100 Bioanalyzer (Total RNA protocol) according to manufacturer`s instructions. Samples with rRNA content >25% were subjected to a second round of mRNA purification and re-evaluated by Bioanalyzer as previously. The total volume of purified mRNA was adjusted to 81 µL with nuclease-free water, 9 µL of fragmentation solution (RNA fragmentation kit; Ambion, #AM8740) was added and samples were kept on ice. Fragmentation was carried out at 75°C for 3 min in a thermoblock, at 3 min samples were immediately cooled on ice and 11 µL of stop solution (RNA fragmentation kit, Ambion) was added. Cleanup was performed using the RNeasy Kit and RNA was eluted in 30 µL. Fragment size distribution and concentration was analysed by Bioanalyzer (mRNA protocol).
First strand cDNA synthesis was carried out with the SuperScript VILO Kit (Invitrogen, #11754050) in a 50 µL reaction with an additional 7.5 µg random hexamers (Invitrogen, #48190011) added to the reaction to increase cDNA yield. Reactions were incubated for 10 Min at 25°C (priming), 60 Min at 42°C (reverse transcription), and 5 Min at 85°C (termination). Reaction clean-up was carried out by gel filtration using Sephadex G50-columns (Mini Quick Spin DNA Columns; Roche #11814419001) according to the manufacturer’s protocol. Second-strand cDNA was synthesised in a final reaction containing the first strand cDNA reaction and final concentrations of: 550 µM of each dATP, cGTP, dCTP (Thermo Scientific, #R0181), and dUTP (deoxy-UTP sodium salt; Roche, #11934554001); 0.300 U DNA Polymerase I (E.coli; Invitrogen, #18010–025), 0.073 U E. coli DNA ligase (Invitrogen, #18052–019), and 0.015 U RNase H (Promega, #M4281); 20 µL 5X second strand buffer (Invitrogen, #10812–014), in a final volume of 100 µL. Reactions were incubated for 2 hr at 16°C then purified with by the MiniElute Reaction clean-up kit (Qiagen, #28004), eluted in 2 × 10 µL volumes in a single reaction tube. Samples were stored at −20°C prior to final library preparation for sequencing.
RNA-Seq library preparation and sequencing
Request a detailed protocolQuality control for concentration, size selection, adapter ligation, pre-amplification PCR and dilution for sequencing was performed according to standard procedures and carried out by the VBCF Next-Generation Sequencing facility services (VBCF-NGS, Vienna Biocenter Campus, https://www.vbcf.ac.at/facilities/next-generation-sequencing/). Samples for initial transcriptome assembly were sequenced on the HiSeq2000 (Illumina) in single lane format (one sample per lane for each of: IM-NM +FM combined, IM-FRFM, PM_NM_male, PM_NM_female, PM_FRFM_male, PM_FRFM_female, M_NM_male, M_NM_female). The IM_NM + FM sample was run in paired-end 100 bp format (PE100), all other samples were analysed as single-end 100 bp (SE100). Biological replicate samples for differential expression analysis were run using SE100 sequencing over seven lanes, in a 6 × 5 plex (Figure 1—figure supplement 2e) and 1 × 6 plex format on the HiSeq2500 instrument (Illumina). The 6-plex lane was a technical replicate lane and contained a single technical replicate from IM samples of each of the other six 5-plex lanes. Read data was quality checked, de-multiplexed and converted to. bam format by NGS facility bioinformatics staff (VBCF-NGS). Reads for individual sequencing libraries are deposited in the European Nucleotide Archive (ENA) under accession number PRJEB27496 (Figure 2—source data 4).
Transcriptome assembly, curation, annotation and read mapping
Request a detailed protocolSequence reads from the eight libraries sequenced on single lanes for deep coverage were used for transcriptome assembly. Paired-end reads from the IM_NM + FM sample were used as a scaffold for the base and combined with the SE100 reads from the other seven libraries for the final assembly. The raw Illumina reads (totalling 1,667,584,873 reads) were quality checked using Fastqc. Cutadapt (v1.9.1, Martin, 2011) to remove the adapter sequences (-b option) of the paired end reads and to quality trim the reads (-q 20) requiring that the remaining sequence was at least 13 bp long (-m 13). We used ngm-utils interleave (v0.4.5) to reorder the fastq files and filter out reads not having a paired read anymore. The so processed reads were merged and used as input for the RNA-Seq assembly with Trinity (v2.0.2, Grabherr et al., 2011). Paired end as well as singleton reads were provided as input to Trinity which was run without taking the strand into account. This initial Pdu_HeadRef_TS_v1 assembly comprised a total of 407,172. Of these multiple copies, only the longest sequence per cluster was selected and this Pdu_HeadRef_TS_v2 assembly was filtered by size to retain transcripts greater than 500 bp in size leading to Pdu_HeadRef_TS_v3 with 64,335 sequences. Finally, the completeness of our RNA-Seq assembly was tested using Cegma (v2.5, Parra et al., 2007), revealing that 85.1% of the genes predicted to be present were present full length, and 93.6% were present either full length or partially.
The Pdu_HeadRef_TS_v3 assembly was then analysed for eukaryotic contaminations using MUMmer (v3.23, Delcher et al., 2003) with a customised script (https://github.com/fritzsedlazeck/sge_mummer) to check for exact hits to common model organism genomes [human (GRCh38), mouse (GRCm38), zebrafish (Zv9), medaka (MEDAKA1), Arabidopsis (TAIR10), Drosophila (BDGP6), C. elegans (WBcel235)], as well as dinoflagellates (Oxyrrhis) and algae (Tetraselmis) used as Platynereis food. Platynereis sequences which had 90% or higher identity and at least 90% of the RNA-Seq scaffold aligned with sequences from the above mentioned organisms were considered putative contaminations. In order to prevent the exclusion of highly conserved Platynereis orthologues, these identified putative eukaryotic contaminations were not excluded from the list, but marked with a tentative suffix (‘_contamination’) to enable them to be distinguished, investigated and post-filtered from downstream analyses, if necessary.
Further curation was then applied to remove redundancy; and these sequences were assembled together with a previously published Platynereis dumerilii transcriptome (herein refered to as Conz_TS: Conzelmann et al., 2013) containing ~350,000 individual contigs. In addition we checked for the presence of all Platynereis transcripts present in GenBank and added/replaced shorter assembled contigs from our assembly with the NCBI sequences where necessary. These sequences were aligned with MUMmer and replaced the scaffolds in our transcriptome if the alignment showed at least 90% identity, at least 200 bp and more than 70% of the scaffold were aligned to the previously published scaffold and the published sequences was longer then our scaffold (if the transcript was absent the sequence from NCBI was added; if the contig from our assembly was shorter than the ncbi sequence then the contig was replaced by the ncbi sequence). We then self-aligned the so obtained scaffolds using MUMmer and kept only one copy of the sequence if the sequences had over 98% identity and one of the copies is covered at least 99% of the length of the other and was longer, otherwise both sequences were kept. This resulted in Pdu_HeadRef_TS_v4 containing 57,869 total sequences. For this assembly we obtained the longest open reading frame (ORF) for all six frames (Pdu_HeadRef_prot_v4) by using Transdecoder (v2.0.1, http://transdecoder.sourceforge.net) and keeping the longest ORF per frame.
To identify prokaryotic contaminations we used Kraken (v0.10.6, Wood and Salzberg, 2014), which uses k-mer matching to identify hits between the query sequences (Pdu_HeadRef_TS_v4) with the default database. To avoid falsely marking orthologues genes we only marked scaffolds that aligned to two different genomes. This approach identified 6,227 putative contigs of prokaryotic origin. We identified 500 of these to be verified Platynereis genes from GenBank, and thus constituting false positives, leaving a total of 5,727 contaminating sequences (9.9% of the total TS_v4 transcriptome, Figure 2—source data 1). These, together with 83 transcripts spiking in one sample (IM_NM) discovered during data processing for differential expression analysis (see below) were then removed to generate the final Pdu_HeadRef_TS_v5 assembly containing 52,059 transcripts.
Base annotation of the TS_v1 assembly was performed using Trinnotate (v2.02) to identify blastx, blastp, HMMER, GO terms etc. for each contig (Figure 2—source data 2). The Pdu_HeadRef_TS_v5 assembly was aligned using blastx against the NCBI_nr_protein database (v_April_2016) and NCBI_ensembl_mouse protein database (v_April_2016) to identify putative protein orthologues with e-value cut-offs at 10−4.
Protein domains, GO term annotations, PANTHER IDs, and pathway analysis etc was additionally analysed and reported for all 57,869 transcripts using InterProScan (v5, using standard parameters). Nucleotide sequences were used as input, split into 18 subsets of ≤3,000 transcripts and first translated using EMBOSS getorf (http://emboss.sourceforge.net/apps/cvs/emboss/apps/getorf.html) and translated sequences were then subject to InterProScan analysis. The results were compiled into a single file merged in addition with the protein_nr and mouse_nr blastx results (Figure 2—source data 3). Further individual reciprocal blastx searches against ncbi_nr protein database were performed in addition to phylogenetic analyses to confirm the orthology of specific candidate genes of interest.
Read mapping and differential expression analysis of RNA-Seq data and selection of top regulated candidates for validation
Request a detailed protocolThe curated Pdu_HeadRef_TS_v4 assembly was used to map all sequencing reads (adapter filtered and quality trimmed) from the replicate head samples for differential expression analysis using NextGenMap (v0.4.13, Sedlazeck et al., 2013) with default parameters. Subsequently, reads were quality filtered (-q20) and converted to a bam file using samtools. The coverage per scaffold was assessed using bedtools (v2.17.0) multicov. The obtained Pdu_HeadRef_TS_v4 count data (generated by HTseq v0.6.1) were processed by first, collapsing the technical replicates (see above and Figure 1—figure supplement 1e) by sum, then by filtering out 83 transcripts with extremely uneven counts in IM_NM sample [counts IM_NM.2>=10 x counts(IM_NM.1+IM_NM.3)] and finally by removing the 5,727 contaminations identified by KRAKEN leading to a 52,059 transcripts (equivalent to Pdu_HeadRef_TS_v5) containing input table (S5_Pdu_HeadRef_TS_v5_ReadCounts). Then differential expression analysis was performed for 14 pairwise comparisons (Figure 1—figure supplement 1, Figure 1—source data 2) and the DESeq derived normalised read counts were used to plot gene expression profiles (Figure 3—source data 2) using the R packages DESeq2 (v1.10.0, Love et al., 2014) and EdgeR (v3.12.0, Robinson et al., 2010). DESeq2 was run with standard parameters and EdgeR was run applying the generalised linear model (Hughes et al., 2012) algorithm. Output lists were cut off using a Benjamini-Hochberg FDR (Benjamini and Hochberg, 1995) of 0.1 (10%).
To filter candidate lists for validation, separate lists of differentially expressed transcripts (DETs) from DESeq2 and EdgeR were merged by transcript ID and rankings were applied separately to the DESeq2 and EdgeRGLM-derived lists, where transcripts were assigned a rank from one to n (where n is the number of transcripts), based on ranking by lowest to highest adjusted p-value (padj/FDR). The DESeq2 and EdgeRGLM rank values for each transcript were then added to give a rank sum value, and the table was re-sorted by this rank sum value from lowest to highest. For maturation comparisons, ‘unisex’ candidate lists were further defined as subsets of transcripts predicted as differentially-expressed between stages, for both male and female comparisons (e.g. if transcript A is up-regulated in IM_NM vs PM_NM_male and up-regulated IM_NM vs PM_NM_female, it is classed as ‘unisex’ up-regulated for IM_NM vs PM_NM). A schematic overview of the comparison procedure can be found in Figure 1—figure supplement 1, and see also Figure 1—source data 2.
Mass spectrometry
Request a detailed protocolProteins for MS analysis were extracted from the same samples used for RNA sequencing, by collecting the first two flow-through fractions from the SiO2-based RNA extraction columns. The pooled flow-through samples were snap frozen in liquid nitrogen and stored at −80°C until further use.
To recover proteins, the flow-through fractions were first lyophilised then re-suspended in H2O to a final volume of 1.0 mL and 4.0 mL of cold acetone was added and proteins were precipitated over-night at −20°C. The precipitate was then collected by centrifugation for 30 min by centrifugation at 3,500xg at 4°C. The resulting pellet and a quarter of the supernatant were transferred in to a fresh tube and the remaining supernatant split into three equal parts which were then centrifuged for 30 Min at 14,000xg and 4°C to collect the remaining proteins. Pellets were then washed with 100 µL −20°C-cold EtOH to remove the co-precipitated GuSCN from the RNA-extraction buffer and allowed to dry over-night, dissolved in 100 µL UA-buffer [8M urea, 50 mM triethylammonium bicarbonate (TEAB), pH 8.5] by sonification, and finally re-pooled. Proteins were digested for MS/MS-analysis using the Filter Aided Sample Preparation method (Wiśniewski et al., 2009). Briefly, the samples were reduced by 10 mM DTT for 30 Min at RT in UA, applied to an YM-30 filter membrane, centrifuged and washed. Thiols were alkylated for 30 Min with 50 mM iodoacetamide at RT in UA centrifuged and washed with 50 mM TEAB. Proteins were then digested with trypsin (1:50 trypsin:protein ratio) overnight at 37°C in 50 mM TEAB. Digested peptides were eluted from filters by centrifugation and acidified by the addition of trifluoroacetic acid, and desalted by solid phase extraction (SPE) with Sep-Pak C18 columns (Waters). Eluates were dried in a speed-vac and the resulting pellets were dissolved in 100 µM TEAB and labelled with TMT isobaric tags (TMTsixplex Isobaric Label Reagent, Thermo Fisher Scientific) according to manufacturer's protocol. Labelling efficiency was assessed by a LC-MS/MS run on Q Exactive plus Orbitrap mass spectrometer (Thermo Fisher Scientific) for each label separately and found to be complete when semi- or unlabelled peptides were less than 1% of total PSMs. Once maximal labelling efficiency was confirmed the reaction was quenched with 8 µL 5% hydroxylamine per reaction vial (15 min incubation time at room temperature). FASP-digested and labelled samples were then combined in a 5-plex multiplex format for Set1 and a 6-plex format for Set2. Peptides were separated on an Ultimate 3000 RSLC nano-flow chromatography system using a pre-column for sample loading (PepMapAcclaim C18, 2 cm ×0.1 mm, 5 μm,) and a C18 analytical column (PepMapAcclaim C18, 50 cm ×0.75 mm, 2 μm, all Dionex-Thermo Fisher Scientific), applying a linear gradient over for 4 hr from 2% to 35% solvent B (80% acetonitrile, 0.1% formic acid; solvent A 0.1% formic acid) at a flow rate of 230 nL/min. Eluting peptides were analysed on a Q Exactive Plus Orbitrap mass spectrometer equipped with a Proxeon nanospray source (all Thermo Fisher Scientific), operated in a data-dependent mode. Survey scans were obtained in a mass range of 380–1,650 m/z with lock mass off, at a resolution of 70,000 at 200 m/z and an AGC target value of 3 × 106. The 12 most intense ions were selected with an isolation window width of 1.2 Da, fragmented in the HCD cell at 35% collision energy and the spectra recorded at a target value of 1 × 105 and a resolution of 17,500. The peptide match and exclude isotope features were enabled and selected precursors were dynamically excluded from repeated sampling for 40 s. Five technical replicate runs were analysed for each set of multiplexed samples.
Data analysis was performed with Proteome Discoverer 2.1 using Sequest to search against the Platynereis proteome database (Pdu_HeadRef_prot_v4, ProteomeXchange: identifier PXD010532). Carbamidomethylation of cysteines, TMT-labelling on peptide N-termini and lysines were selected as fixed modifications, oxidation of methionine as variable modification. Precursor tolerance was set to 10ppm, fragment tolerance to 0.02 Da. Data were filtered for an FDR of 1% at PSM and protein level. Quantification was performed via the reporter ions quantifier mode in PD 2.1 based on the signal/noise values (herein referred to as intensity). Spectra with isolation interference greater 30% were not used for quantification. Protein intensity values were calculated for each biological replicate as the average intensity of all peptides per protein across all five technical replicates (herein referred to as Mean Intensities per protein). Mean intensities per protein were then normalised between isobaric ion channels to obtain a 1:1:1:1:1 ratio of reporter ion intensities based on the summed reporter intensities across all proteins per channel, within each set of 5 multiplexed samples (herein these expression values are referred to as Norm. Mean intensities per protein). Quantifiable proteins were further defined as those with at least two uniquely mapping peptides and detected in at least 2/3 biological replicates per sample group.
To normalise for variation in protein intensity distributions between individual samples, an additional channel median normalisation was applied, whereby the median protein intensity value was calculated for each sample (‘channel median value’) and all individual protein values per sample were then divided by this channel median value. We performed an additional inter-replicate median normalisation to account for inter-run variation between biological replicate sets within each of the NM and FRFM experiments, by: i) calculating for each biological replicate set; the median intensity across all five samples per protein, ii) calculating conversion factors to equalize the median intensity per protein between biological replicate sets. Conversion factors (CF) were calculated for the NM set relative to BR1, for example: CF.BR2 = Median_NM_BR1/Median_NM_BR2; CF.BR3 = Median_NM_BR1/Median_NM_BR3, and relative to BR3 for the FRFM set, for example: CF.BR1 = Median_FRFM_BR3/Median_FRFM_BR1; CF.BR2 = Median_FRFM_BR3/Median_FRFM_BR3. All intensity values per protein per biological replicate were then multiplied by the respective conversion factor. To enable normalisation and comparison of the data from the NM and FRFM experiments we included a spiked-control sample from the NM_BR1 set (TMT-128_PM_NM_female.1) in each of the FRFM biological replicate sets. Therefore, as a final step the FRFM intensity values were normalised relative to those of the NM_BR1 set by multiplying the values for each protein in each BR set, by corresponding conversion factors (CF) calculated as the ratio of the NM_BR1 TMT-128_PM_NM_female1 intensity/intensity value recorded for this sample in FRFM BR sets 1, 2 and 3 (e.g. CF.FRFM1 = TMT-128_PM_NM_female1:NM/TMT-128_PM_NM_female1:FRFM_BR1; CF.FRFM2 = TMT-128_PM_NM_female1:NM/TMT-128_PM_NM_female1:FRFM_BR2). The effect of normalisation resulted in median-centred data and decreased variability in the distribution of protein intensity values between biological replicates (Figure 5—figure supplement 7 and Figure 5—source data 1). TMT-based quantitative proteomics data were acquired using the VBCF instrument pool (www.vbcf.ac.at).
For benchmarking the protein set obtained with the described method, results were also compared with a set of proteins identified by a more conventional unlabelled proteomic analysis on separate head samples (Bileck, Gerner et al., unpublished). Briefly, for this analysis, tissue samples were incubated in 100 µl sample buffer (7.5M urea, 1.5M thiourea, 4% CHAPS, 0.05% SDS, 100 mM DTT) for 4 hr and lysed using an ultrasonic probe. An adapted filter aided sample preparation protocol was used for protein digestion as described (Slany et al., 2016). Cleaned peptide samples were reconstituted in 5 µl 30% formic acid containing 10 fmol each of 4 synthetic standard peptides and diluted with 40 µl mobile phase A (98% H2O, 2% acteonitrile, and 0.1% formic acid) and analysed as described previously (Slany et al., 2016). Identification of proteins was performed using the MaxQuant software (v1.5.2.8) including the Andromeda search (Cox and Mann, 2008) to map the identified peptides against the Pdu_HeadRef_prot_v4 database. A minimum of two peptide identifications, at least one of them unique, was required for positive protein identification. For peptides and proteins, a FDR of less than 0.01 was applied.
Differential abundance analysis of proteomics data
View detailed protocolDifferential abundance analysis for the normalised proteomic data for the subset of 1064 quantifiable proteins was performed using both Linear Models for Microarray and RNA-Seq Data (Li et al., 2016, http://bioinf.wehi.edu.au/limma) and the Reproducibility-Optimised Test Statistic (ROTS) package for R (Elo et al., 2008; Elo et al., 2009, http://www.btk.fi/research/research-groups/elo/software/rots/). Both ROTS and LIMMA are able to handle missing values, which is a common feature of proteomic datasets. ROTS and LIMMA were used to analyse differential expression using a BH FDR of 0.1 (10%), for the 14 pairwise comparisons (Figure 1—figure supplement 1, Figure 1—source data 2) defined previously for RNA-Seq differential expression analysis using DESeq2 and EdgeR, details with regard to the statistical testing are given below in the section Statistics. The same rank sum approach was taken to merge the results of LIMMA and ROTS predictions and rank candidates according to adjusted pvalues. Candidates were further classified into unisex groupings for maturation stage comparisons.
Testing for statistical effects in the number of analysed transcripts
Request a detailed protocolTo test whether the different input list sizes of transcripts and proteins, 52,059 and 1,064 respectively, might affect the relative amount of DE predictions, we also performed DESeq2 and EdgeR analysis on the 1000 and 5,000 most abundant transcripts. For this, we calculated the mean read counts over all comparison per transcript, sorted the transcript list and chose the 1,000 or 5,000 transcripts with the highest average number of read counts. These reduced lists were then subjected to DE analysis by DESeq2 and EdgeR as before, and rank sum lists of these analyses were then generated as outlined above.
Quantitative PCR
Request a detailed protocolqRT-PCR, including primer design, primer testing for efficiency, cDNA synthesis protocol, reaction chemistry, cycle conditions and instrumentation, was performed as described previously (Schenk et al., 2016), with the following modifications: Total RNA (200 ng per sample) was reverse transcribed to cDNA using the Quantitect reverse transcription kit (Qiagen, Cat# 205310), with incubation at 42°C for 20 min for reverse transcription. Final cDNA reactions were diluted to a final volume of either 95 µL for low-expression level and lunar candidate qRT-PCR assays, or 350 µL for sex-biased and maturation candidate qPCR assays and all qRT-PCR reactions were performed in 15 µl final volume, using 3 µL of cDNA template. Primers for qPCR assays (intron-spanning where possible) were designed using the Universal Probe Library assay design tool (Roche, Supplementary file 2 ).
Target genes and reference controls were analysed in duplicate reactions for all samples. Plate control cDNA and –RT controls for the target gene(s) were included on each plate as quality controls for inter-plate consistency. Cell cycle serine/threonine kinase (cdc5, Dray et al., 2010; Zantke et al., 2013) and S-adenosyl methionine synthetase (sams, Schenk et al., 2016), were measured as reference genes, based on previous evaluation as stable qPCR reference controls (Schenk et al., 2016). Expression levels for target genes were calculated using the Δ-Ct method, using the mean of cdc5 and sams Ct values for relative normalisation (Figure 4—source data 1). Calculated Δ-Ct values were exponentially transformed (2-(Δ- Ct)) to give the final normalised relative expression values. Statistical analysis was performed as outlined below.
Sequence analysis tools
Request a detailed protocolCLC Main workbench (version seven and higher) were used for routine sequence analysis, small-scale BLASTx and BLASTp searches against NCBI, assemblies, basic multiple sequence alignments, and sequence annotations. Phylogenetic trees for ependymin-related proteins (ERPs) and Whitnin/Proctolin were generated from multiple sequence alignments (MUSCLE: http://www.drive5.com/muscle, Edgar, 2004), see Figure 6—source datas 1 and 2; Figure 7—source datas 1 and 2) and IQ-TREE (v1.6.3, Minh et al., 2013; Nguyen et al., 2015), ultra fast bootstrapping (Hoang et al., 2018), and the integrated ModelFinder (Kalyaanamoorthy et al., 2017).
Simple Modular Architecture Research Tool (SMART, http://smart.embl-heidelberg.de) was used for analysis of protein domains in individual sequences. Functional descriptions of individual orthologue proteins were also sourced from the Uniprot database (http://www.uniprot.org).
Cloning and plasmids used in this study
Request a detailed protocolCandidate genes of interest were cloned from cDNA reverse transcribed from total RNA of heads from appropriate sex, stage and circalunar condition, using the Transcriptor high fidelity cDNA synthesis kit (Roche, #05081955001). Final cDNA reactions were diluted to a final volume of 50 µL with nuclease free water. PCR: 20 µl final reaction volume, 1–5 µl of cDNA template, Phusion polymerase (Thermo Scientific). PCR programs: 98°C denaturing cycles of 30 s, annealing temperatures ranging from 58–62°C with 20 s annealing times and extension at 72°C for 35–40 cycles (primer details- Supplementary file 2). PCR products were analysed by agarose gel electrophoresis (GelGreen, Biotium), bands of expected size were isolated using the Qiagen gel extraction kit according to the manufacturer’s instructions. Extracted DNA was ligated into the pJET1.2 vector DNA (CloneJET PCR cloning kit, Thermo Scientific). Plasmid DNA was purified after colony PCR using a standard alkaline lysis protocol with sequential precipitation using isopropanol and 70% ethanol (Birnboim and Doly, 1979). DNA pellets were resuspended in 30–50 µL of nuclease free water and verified by Sanger sequencing (Microsynth, Austria). Plasmid cloning info- Supplementary file 2.
Whole mount in situ hybridisation
Request a detailed protocolAnimals for in situ hybridisation (ISH) were sourced from culture boxes, sexed and staged according to the aforementioned criteria and maintained in separate boxes in antibiotic-free ASW under respective circalunar conditions for at least 48 hr prior to sampling. ISH for head tissues was carried out as previously described (Tessmar-Raible et al., 2005; Zantke et al., 2013). DNA templates for in vitro transcription were generated by amplifying sense and antisense sequences from vector DNA templates using Sp6-conjugated pJET1.2 forward and reverse primer combinations (see Supplementary file 2). Probes were purified using the RNeasy kit (Qiagen) according to manufacturer’s instructions, eluted in 30 µL of RNase-free water, probe quality analysed by agarose gel electrophoresis and diluted to a concentration of 100 ng/µL or 50 ng/µL with hybridisation solution and stored at −20°C. For ISH 500 or 1000 ng of probe were used depending on the expression of the gene as judged from RNA-Seq. Stained tissues were stored in 80–100% glycerol/1X PTW at 4°C in 2 mL tubes.
For all genes assessed sense controls were run in parallel in pools of 3–5.
In situs were imaged using an Axioplan Z2 Microscope (Carl Zeiss, Germany), with AxioCam MRc5 colour CCD camera (Carl Zeiss, Germany) and captured using ZenPro Software (v2.0, Carl Zeiss, Germany). Images were saved in TIFF format.
Image analysis and processing
Request a detailed protocolWhere necessary brightness and contrast adjustments and cropping of images in TIFF format was carried out using ImageJ (v1.50i) or Adobe Photoshop CC (v2015). Figures were constructed using Adobe Illustrator CC (v2015).
Data analysis methods RNA-Seq and proteomics functional analyses
Soft clustering for identification of similarly regulated genes
Request a detailed protocolExpression profile clustering was performed using the Mfuzz package (v2.32.0, Futschik and Carlisle, 2005; Kumar and Futschik, 2007, http://mfuzz.sysbiolab.eu) for R. Briefly, input tables of mean read counts, or mean normalised protein intensity values were organised in columns with separate rows for each protein or transcript. Input data was standardised using the standardise() function of the Mfuzz package and the estimate of fuzziness (m1) was calculated using mestimate(). The number of clusters was determined by analysing cluster dendograms generated from a distance matrix using hclust() and cutree() to visualise different cluster numbers relative to the dendogram branching. Cluster information was used to group candidate transcript and protein expression profiles by likeness and membership were used as a measure of fit for individual profiles to the cluster mean profile.
GOstats analysis for predictive functional annotation
Request a detailed protocolGene ontology (GO) analysis was performed using the GOstats package (v2.46.9, Falcon and Gentleman, 2007), adapting the procedure described by Carlson for uncommon model organisms (https://www.bioconductor.org/packages/release/bioc/vignettes/GOstats/inst/doc/GOstatsForUnsupportedOrganisms.pdf). Briefly, the background gene set (gene universe) files (Figure 3—source data 3) were generated using GO terms retrieved from the InterProScan results containing evidence code ‘IAE’. For GO enrichment testing of differentially expressed genes (DEGs) from RNA-Seq experiments, and for testing over and under-represented GO ids in the detected set of proteins against the transcriptome, the gene universe was derived from the full set of GO terms for the whole Pdu_HeadRef_Ts_v5 transcriptome, and comprised 2,472 unique GO terms corresponding to 16,921 transcripts (Figure 3—source data 3). For differential expression of proteins the gene universe size was derived from the set of 2,290 quantifiable proteins, and comprised 1,035 GO terms corresponding to 1,751 proteins (Figure 5—source data 2). To perform hypergeometric testing using the selected gene universe, GO2All mapping was first performed using the Human AnnotationDbi package and the resulting GOALLFrame object was used to generate a customised GeneSetCollection for the hypergeometric testing, leading to 4,775 unique GO-terms in the transcriptome universe; and 2,448 unique GO-terms in the protein background. Tests for over and under-representation of GO terms were run for all sets of candidates, for all ontologies (Biological Process: BP, Molecular Function: MF, Cellular Compartment: CC) using conditional parameters. Ranking of GO enrichment was performed using the p-value, which indicates the relative significance of enrichment of genes in the tested gene list compared to the specified gene universe.
Individual ontologies (BP, MF, CC) were analysed for over and/or under-representation for each subset of candidate transcripts/proteins and enriched GO terms were linked to corresponding transcript ids using the original gene universe file, and by mapping to parent terms using acyclic graphs from the GO.db package.
Statistics
All statistical analyses were performed with R (R Core Development Team, 2015), and various statistical packages available for R.
Analysis of differentially expressed genes was performed with EdgeR (McCarthy et al., 2012; Robinson et al., 2010) and DESeq2 (Love et al., 2014) using custom made scripts, considering a BH FDR of 0.1 as statistically significant.
Proteomic data were analysed using either LIMMA (Ritchie et al., 2015) and ROTS (Elo et al., 2008; Elo et al., 2009). LIMMA uses linear modelling to test the fit of obtained expression data to the null hypothesis based on the defined experimental design. The script used was modified based on a previously available application for labelled-proteomics data (Kammers et al., 2015, http://www.biostat.jhsph.edu/~kkammers/software/eupa/R_guide.html), and used the more conservative BH FDR rather than q-values (Storey and Tibshirani, 2003) originally in the script. ROTS is a modified form of the t-test, which uses bootstrapping to analyse differential expression between sample groups using random-subsets of samples. From the collective results a modified test statistic is calculated based on the reproducibility of the list of differentially expressed predictions (Elo et al., 2008).
GO-Term analysis was performed using the GOstats package (v2.46.0), which applies a hypergeometric test to test for significance. We used the conditional algorithm of hypergeometric test which takes into account the hierarchical structure of the GO-terms by testing the leaves (GO-Terms with no child-terms) first. The conditioning then leads to the elimination of those genes associated with a GO-Term which have been tested as significant in the GO-term`s children (Falcon and Gentleman, 2007).
qRT-PCR data were first checked for normality by the Shapiro-Wilk test (Shapiro and Wilk, 1965) using the generic shapiro.test() function. Statistical significance was then assessed by an unpaired t-test with Welch`s correction for heteroskedasticity (Welch, 1947) with at least five biological replicates per group. Corrections for multiple testing were done applying the BH algorithm (Benjamini and Hochberg, 1995).
Data availability
The data generated and analysed during this study are included in the published article, its supplementary information files, and the following repositories: The mass spectrometry proteomics raw data and the translated protein database FASTA file have been deposited in the PRIDE database repository (Vizcaíno et al., 2016) with the dataset identifier PXD010532. Transcriptome data (RNA-Seq read libraries) have been submitted to the sequence read archive (SRA) through ENA; accession numbers: see Figure 2—source data 4. Transcriptome data (assembled sequences): ENA repository; accession number: PRJEB27496. Sequences of cloned coding sequences validated in this study: GenBank repository; accession numbers: MH587646-MH587650, MH645920-MH645924, MH678618 and MH678619.
-
NCBI GenBankID MH645920. Combined transcriptome and proteome profiling reveals specific molecular brain signatures for sex, maturation and circalunar clock phase.
-
NCBI GenBankID MH678618. Combined transcriptome and proteome profiling reveals specific molecular brain signatures for sex, maturation and circalunar clock phase.
-
European Nucleotide ArchiveID PRJEB27496. Combined transcriptome and proteome profiling reveals specific molecular brain signatures for sex, maturation and circalunar clock phase.
-
EBI PRIDEID PXD010532. Combined transcriptome and proteome profiling reveals specific molecular brain signatures for sex, maturation and circalunar clock phase.
-
NCBI GenBankID MH587646. Combined transcriptome and proteome profiling reveals specific molecular brain signatures for sex, maturation and circalunar clock phase.
-
NCBI GenBankID MH587647. Combined transcriptome and proteome profiling reveals specific molecular brain signatures for sex, maturation and circalunar clock phase.
-
NCBI GenBankID MH587648. Combined transcriptome and proteome profiling reveals specific molecular brain signatures for sex, maturation and circalunar clock phase.
-
NCBI GenBankID MH587649. Combined transcriptome and proteome profiling reveals specific molecular brain signatures for sex, maturation and circalunar clock phase.
-
NCBI GenBankID MH587650. Combined transcriptome and proteome profiling reveals specific molecular brain signatures for sex, maturation and circalunar clock phase.
-
NCBI GenBankID MH645921. Combined transcriptome and proteome profiling reveals specific molecular brain signatures for sex, maturation and circalunar clock phase.
-
NCBI GenBankID MH645922. Combined transcriptome and proteome profiling reveals specific molecular brain signatures for sex, maturation and circalunar clock phase.
-
NCBI GenBankID MH645923. Combined transcriptome and proteome profiling reveals specific molecular brain signatures for sex, maturation and circalunar clock phase.
-
NCBI GenBankID MH645924. Combined transcriptome and proteome profiling reveals specific molecular brain signatures for sex, maturation and circalunar clock phase.
-
NCBI GenBankID MH678619. Combined transcriptome and proteome profiling reveals specific molecular brain signatures for sex, maturation and circalunar clock phase.
References
-
Structure, function, and evolution of ferritinsJournal of Inorganic Biochemistry 47:161–174.https://doi.org/10.1016/0162-0134(92)84062-R
-
Development of pigment-cup eyes in the polychaete Platynereis dumerilii and evolutionary conservation of larval eyes in BilateriaDevelopment 129:1143–1154.
-
Effects of the volatile fraction of crude oil on spawning behaviour of nereids (Annelida, Polychaeta)Marine Environmental Research 40:267–276.https://doi.org/10.1016/0141-1136(94)00145-F
-
Controlling the false discovery rate: a practical and powerful approach to multiple testingJournal of the Royal Statistical Society: Series B 57:289–300.https://doi.org/10.1111/j.2517-6161.1995.tb02031.x
-
A rapid alkaline extraction procedure for screening recombinant plasmid DNANucleic Acids Research 7:1512–1523.
-
The developmental proteome of Drosophila melanogasterGenome Research 27:1273–1285.https://doi.org/10.1101/gr.213694.116
-
Immunological properties of oxygen-transport proteins: hemoglobin, hemocyanin and hemerythrinCellular and Molecular Life Sciences 74:293–317.https://doi.org/10.1007/s00018-016-2326-7
-
Discovery and evolution of novel hemerythrin genes in annelid wormsBMC Evolutionary Biology 17:e85.https://doi.org/10.1186/s12862-017-0933-z
-
Using MUMmer to identify similar regions in large sequence setsCurrent Protocols in Bioinformatics 00:10.3.1–10.310.https://doi.org/10.1002/0471250953.bi1003s00
-
MUSCLE: multiple sequence alignment with high accuracy and high throughputNucleic Acids Research 32:1792–1797.https://doi.org/10.1093/nar/gkh340
-
Reproducibility-optimized test statistic for ranking genes in microarray studiesIEEE/ACM Transactions on Computational Biology and Bioinformatics 5:423–431.https://doi.org/10.1109/tcbb.2007.1078
-
Das Auge von Platynereis dumerilii (Polychaeta). Sein Feinbau im ontogenetischen und adaptiven WandelZeitschrift für Zellforschung und Mikroskopische Anatomie 71:217–244.
-
BookControl of oocyte differentiation in nereids (Annelida, Polychaeta) - facts and ideasIn: Fischer A, Pfannenstiel HD, editors. Fortschritte Der Zoologie, 29. Stuttgart: Gustav Fischer Verlag. pp. 227–245.
-
Noise-robust soft clustering of gene expression time-course dataJournal of Bioinformatics and Computational Biology 03:965–988.https://doi.org/10.1142/S0219720005001375
-
Full-length transcriptome assembly from RNA-Seq data without a reference genomeNature Biotechnology 29:644–652.https://doi.org/10.1038/nbt.1883
-
The lunar cycle: a cue for amphibian reproductive phenology?Animal Behaviour 78:349–357.https://doi.org/10.1016/j.anbehav.2009.05.007
-
Hormonale Hemmung der Geschlechtsreife und Metamorphose bei dem Polychaeten Platynereis dumeriliiZeitschrift für Naturforschung B 11:125–132.https://doi.org/10.1515/znb-1956-0302
-
Notizen: Weitere Versuche zur Frage des Juvenilhormons bei PlatynereisZeitschrift für Naturforschung B 11:610–612.https://doi.org/10.1515/znb-1956-9-1026
-
Abhängigkeit der Regenerationsleistung von der inneren Sekretion im Prostomium bei Platynereis dumeriliiZeitschrift für Naturforschung B 15:52–55.https://doi.org/10.1515/znb-1960-0111
-
Der hormonale Einfluss des Gehirns auf die sexuelle Entwicklung bei dem polychaeten Platynereis dumeriliiGeneral and Comparative Endocrinology 6:26–73.https://doi.org/10.1016/0016-6480(66)90108-0
-
Großes zoologisches PraktikumPlatynereis dumerilii, Großes zoologisches Praktikum, Gustav Fischer Verlag Stuttgart.
-
Normalisierung der geschlechtlichen Entwicklung kopfloser Fragmente junger ♀♀ von Platynereis dumerilii (Polychaeta) durch Behandlung mit konservierten Prostomien juveniler IndividuenHelgoländer Wissenschaftliche Meeresuntersuchungen 26:63–81.https://doi.org/10.1007/BF01613305
-
Zebrafish pituitary gene expression before and after sexual maturationJournal of Endocrinology 221:429–440.https://doi.org/10.1530/JOE-13-0488
-
Worker honeybee brain proteomeJournal of Proteome Research 11:1485–1493.https://doi.org/10.1021/pr2007818
-
UFBoot2: improving the ultrafast bootstrap approximationMolecular Biology and Evolution 35:518–522.https://doi.org/10.1093/molbev/msx281
-
Regeneration and endocrinology in the polychaete Platynereis dumeriliiWilhelm Roux's Archives of Developmental Biology 180:47–71.https://doi.org/10.1007/BF00848884
-
BookBrain hormone levels and feed-back regulation during gametogenesis, metamorphosis and regeneration in Platynereis dumerilii - An experimental approachIn: Fischer A, Pfannenstiel H. D, editors. Fortschritte Der Zoologie, 29. Stuttgart New York: Gustav Fischer Verlag. pp. 73–79.
-
Deep sequencing the circadian and diurnal transcriptome of Drosophila brainGenome Research 22:1266–1281.https://doi.org/10.1101/gr.128876.111
-
Mating changes sexually dimorphic gene expression in the seed beetle Callosobruchus maculatusGenome Biology and Evolution 9:677–699.https://doi.org/10.1093/gbe/evx029
-
Detecting significant changes in protein abundanceEuPA Open Proteomics 7:11–19.https://doi.org/10.1016/j.euprot.2015.02.002
-
Lunar synchronization of the monthly reproductive rhythm in the sea urchin centrostephanus coronatus VerrillJournal of Experimental Marine Biology and Ecology 17:323–331.https://doi.org/10.1016/0022-0981(75)90007-6
-
Dmrt genes in the development and evolution of sexual dimorphismTrends in Genetics 28:175–184.https://doi.org/10.1016/j.tig.2012.02.002
-
The genetics of migration on the moveTrends in Ecology & Evolution 26:561–569.https://doi.org/10.1016/j.tree.2011.07.009
-
Differential expression analysis of multifactor RNA-Seq experiments with respect to biological variationNucleic Acids Research 40:4288–4297.https://doi.org/10.1093/nar/gks042
-
ThesisZur Hormonabhängigkeit der Spermatogenese bei Platynereis dumerilii: Licht- und elektronenmikroskopische Befunde sowie experimentelle Untersuchungen in vivo und in vitro. PhD-ThesisRuhr-Universität, Bochum.
-
Ultrafast approximation for phylogenetic bootstrapMolecular Biology and Evolution 30:1188–1195.https://doi.org/10.1093/molbev/mst024
-
IQ-TREE: a fast and effective stochastic algorithm for estimating maximum-likelihood phylogeniesMolecular Biology and Evolution 32:268–274.https://doi.org/10.1093/molbev/msu300
-
Transcriptome dynamics over a lunar month in a broadcast spawning acroporid coralMolecular Ecology 26:2514–2526.https://doi.org/10.1111/mec.14043
-
Insect ferritins: typical or atypical?Biochimica Et Biophysica Acta (BBA) - General Subjects 1800:824–833.https://doi.org/10.1016/j.bbagen.2010.03.004
-
SoftwareR: A language and environment for statistical computingR Foundation for Statistical Computing, Vienna, Austria.
-
Lunar cycle in the reproductive activity in the forktail rabbitfishFish Physiology and Biochemistry 28:443–444.https://doi.org/10.1023/B:FISH.0000030623.49948.3c
-
An overview of monthly rhythms and clocksFrontiers in Neurology 8:eCollection 2017.https://doi.org/10.3389/fneur.2017.00189
-
A preliminary study on the effect of lunar cycles on the spawning behaviour of the gilt-head sea bream, Sparus aurataJournal of the Marine Biological Association of the UK 86:899–901.https://doi.org/10.1017/S0025315406013841
-
Entrainment of tidal and semilunar rhythms by artificial moonlight cyclesThe Biological Bulletin 174:126–138.https://doi.org/10.2307/1541779
-
Lipid accumulation and metabolism in polychaete spermatogenesis: role of the large discoidal lipoproteinMolecular Reproduction and Development 77:710–719.https://doi.org/10.1002/mrd.21208
-
The atokous-epitokous border is determined before the onset of heteronereid development in Platynereis dumerilii (Annelida, Polychaeta)Roux's Archives of Developmental Biology 198:29–33.https://doi.org/10.1007/BF00376367
-
Ependymin, a brain extracellular glycoprotein, and CNS plasticityAnnals of the New York Academy of Sciences 627:94–114.https://doi.org/10.1111/j.1749-6632.1991.tb25916.x
-
Contribution of human fibroblasts and endothelial cells to the hallmarks of inflammation as determined by proteome profilingMolecular & Cellular Proteomics 15:1982–1997.https://doi.org/10.1074/mcp.M116.058099
-
Molecular evolution of the ependymin protein family: a necessary updateBMC Evolutionary Biology 7:e23.https://doi.org/10.1186/1471-2148-7-23
-
Neurohormones and neuropeptides encoded by the genome of Lottia gigantea, with reference to other mollusks and insectsGeneral and Comparative Endocrinology 167:86–103.https://doi.org/10.1016/j.ygcen.2010.02.010
-
2016 update of the PRIDE database and its related toolsNucleic Acids Research 44:D447–D456.https://doi.org/10.1093/nar/gkv1145
-
Accurate quantification of more than 4000 mouse tissue proteins reveals minimal proteome changes during agingMolecular & Cellular Proteomics 10:M110.004523.https://doi.org/10.1074/mcp.M110.004523
-
Universal sample preparation method for proteome analysisNature Methods 6:359–362.https://doi.org/10.1038/nmeth.1322
-
Annual, Lunar and Tidal Clocks: Patterns and Mechanisms of Nature’sEnigmatic RhythmsKeeping clockscoordinated: Crosstalk between light, circadian and circalunar clocks, Annual, Lunar and Tidal Clocks: Patterns and Mechanisms of Nature’sEnigmatic Rhythms, Japan, Springer.
-
Uric acid: the sperm-release pheromone of the marine polychaete Platynereis dumeriliiJournal of Chemical Ecology 24:13–22.https://doi.org/10.1023/A:1022328610423
Article and author information
Author details
Funding
European Research Council ((FP7/2007-2013)/ERC Grant Agreement 260304)
- Florian Raible
European Research Council ((FP7/2007-2013)/ERC Grant Agreement 337011)
- Kristin Tessmar-Raible
University of Vienna (Research Platform Rhythms of Life)
- Arndt von Haeseler
- Christopher Gerner
- Florian Raible
- Kristin Tessmar-Raible
Austrian Science Fund (#AY0041321)
- Kristin Tessmar-Raible
Austrian Science Fund (#P28970)
- Kristin Tessmar-Raible
Austrian Science Fund (#I2972)
- Florian Raible
Austrian Science Fund (#M1478)
- Sven Schenk
Vienna International PostDoctoral Program for Molecular Life Sciences (#GM100402)
- Stephanie C Bannister
European Molecular Biology Organization (YIP #2893)
- Kristin Tessmar-Raible
The funders had no role in study design, data collection and interpretation, or the decision to submit the work for publication.
Acknowledgements
We thank the members of the Tessmar-Raible and Raible groups for discussions, Andreij Belokurov and Margaryta Borysova for excellent worm care at the MFPL Fish and Marine facility.
Version history
- Received: October 7, 2018
- Accepted: January 15, 2019
- Version of Record published: February 15, 2019 (version 1)
- Version of Record updated: February 18, 2019 (version 2)
Copyright
© 2019, Schenk et al.
This article is distributed under the terms of the Creative Commons Attribution License, which permits unrestricted use and redistribution provided that the original author and source are credited.
Metrics
-
- 8,277
- views
-
- 625
- downloads
-
- 43
- citations
Views, downloads and citations are aggregated across all versions of this paper published by eLife.
Download links
Downloads (link to download the article as PDF)
Open citations (links to open the citations from this article in various online reference manager services)
Cite this article (links to download the citations from this article in formats compatible with various reference manager tools)
Further reading
-
- Ecology
Diaphorina citri serves as the primary vector for ‘Candidatus Liberibacter asiaticus (CLas),’ the bacterium associated with the severe Asian form of huanglongbing. CLas-positive D. citri are more fecund than their CLas-negative counterparts and require extra energy expenditure. Therefore, understanding the molecular mechanisms linking metabolism and reproduction is of particular importance. In this study, we found adipokinetic hormone (DcAKH) and its receptor (DcAKHR) were essential for increasing lipid metabolism and fecundity in response to CLas infection in D. citri. Knockdown of DcAKH and DcAKHR not only resulted in the accumulation of triacylglycerol and a decline of glycogen, but also significantly decreased fecundity and CLas titer in ovaries. Combined in vivo and in vitro experiments showed that miR-34 suppresses DcAKHR expression by binding to its 3’ untranslated region, whilst overexpression of miR-34 resulted in a decline of DcAKHR expression and CLas titer in ovaries and caused defects that mimicked DcAKHR knockdown phenotypes. Additionally, knockdown of DcAKH and DcAKHR significantly reduced juvenile hormone (JH) titer and JH signaling pathway genes in fat bodies and ovaries, including the JH receptor, methoprene-tolerant (DcMet), and the transcription factor, Krüppel homolog 1 (DcKr-h1), that acts downstream of it, as well as the egg development related genes vitellogenin 1-like (DcVg-1-like), vitellogenin A1-like (DcVg-A1-like) and the vitellogenin receptor (DcVgR). As a result, CLas hijacks AKH/AKHR-miR-34-JH signaling to improve D. citri lipid metabolism and fecundity, while simultaneously increasing the replication of CLas, suggesting a mutualistic interaction between CLas and D. citri ovaries.
-
- Ecology
Spatial and temporal associations between sympatric species underpin biotic interactions, structure ecological assemblages, and sustain ecosystem functioning and stability. However, the resilience of interspecific spatiotemporal associations to human activity remains poorly understood, particularly in mountain forests where anthropogenic impacts are often pervasive. Here, we applied context-dependent Joint Species Distribution Models to a systematic camera-trap survey dataset from a global biodiversity hotspot in eastern Himalayas to understand how prominent human activities in mountain forests influence species associations within terrestrial mammal communities. We obtained 10,388 independent detections of 17 focal species (12 carnivores and five ungulates) from 322 stations over 43,163 camera days of effort. We identified a higher incidence of positive associations in habitats with higher levels of human modification (87%) and human presence (83%) compared to those located in habitats with lower human modification (64%) and human presence (65%) levels. We also detected a significant reduction of pairwise encounter time at increasing levels of human disturbance, corresponding to more frequent encounters between pairs of species. Our findings indicate that human activities can push mammals together into more frequent encounters and associations, which likely influences the coexistence and persistence of wildlife, with potential far-ranging ecological consequences.