High-throughput, single-particle tracking reveals nested membrane domains that dictate KRasG12D diffusion and trafficking
Figures
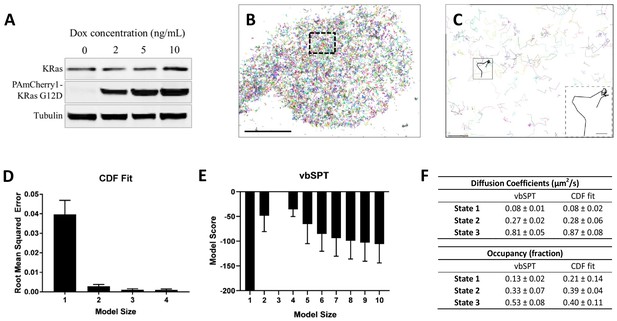
Defining the membrane diffusion model of KRasG12D using spt-PALM and vbSPT.
(A) Western blot showing the increasing expression level of PAmCherry1-KRasG12D with increasing doxycycline (Dox) concentration; (B) Example trajectory map of membrane KRasG12D acquired at 12 ms frame rate using total internal reflection fluorescence (TIRF) illumination. Each line represents an individual Ras molecule coordinate over time acquired for the duration of the movie (20 min). Only a subset of all trajectories is plotted. Scale bar: 10 μm; (C) Expanded view of the boxed region in B). Only a subset of all of the trajectories in the boxed region is shown to allow unhindered view of individual Ras trajectories. Inset shows a KRasG12D trajectory displaying multiple diffusion states. Scale bars: main figure: 1 μm; inset: 200 nm; (D) Determining the optimal model size for KRasG12D membrane diffusion using CDF fitting, with smaller root mean squared error indicating a better model (n = 8); (E) Determining the optimal model size for KRasG12D membrane diffusion using vbSPT, with smaller absolute model score (i.e., score of zero being the best global model) indicating a better model (n = 5); (F) Comparing the model parameters obtained from CDF fit and vbSPT, both using a three-state model for KRasG12D membrane diffusion. State transition probabilities were not inferred from CDF fit and therefore not included in the comparison. Error bars are 95% confidence intervals (CIs).
-
Figure 1—source data 1
Excel sheet for data used for generating panels D, E, and F.
Data for individual panels are included as separate tabs in the excel file.
- https://cdn.elifesciences.org/articles/46393/elife-46393-fig1-data1-v2.xlsx
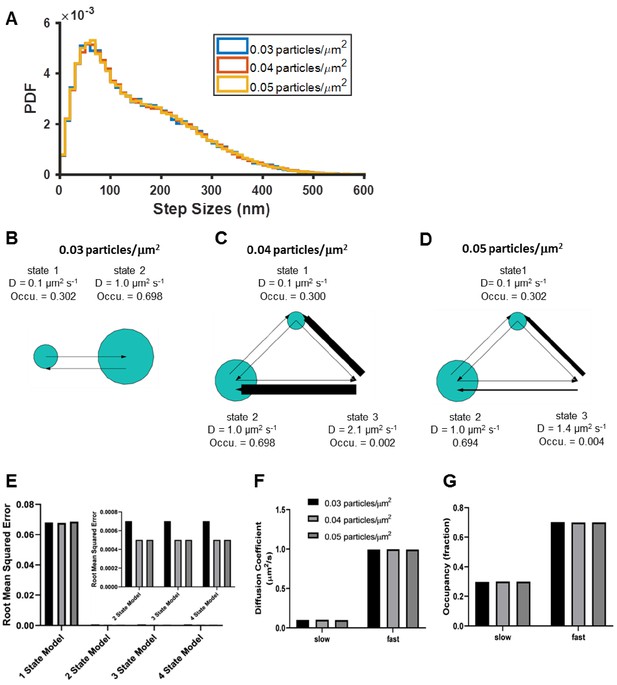
Impact of particle density on diffusion model reconstruction.
Test data were generated by simulating diffusion trajectories of two separate populations of particles with no transitions exhibiting diffusion coefficients of 0.1 and 1 µm2s−1, and occupancies of 0.3 and 0.7, respectively (see Materials and methods). About 6–10 k trajectories were synthesized (depending on the particle density) with connection distance threshold of 600 nm and analyzed using vbSPT (B–D) or CDF (E–G). (A) Histograms of step sizes at 0.03, 0.04, and 0.05 particles/µm2 per frame; B–D) show the vbSPT outputs on simulated trajectories at 0.03, 0.04, and 0. 05 particles/µm2, returning 2, 3, and three state models respectively, with the model parameters displayed next to each state; E) Goodness of CDF fitting at different model sizes, as well as the diffusion coefficients (F) and state occupancies (G) obtained from fitting to a 2-state model.
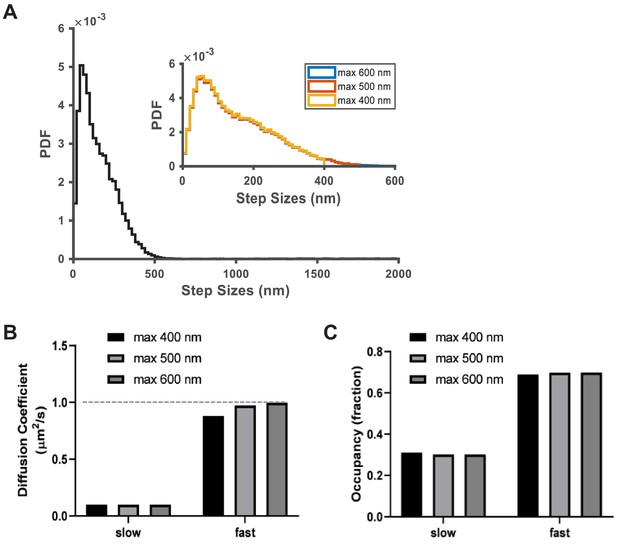
Impact of trajectory connection distance on vbSPT model output.
The connection distance threshold specifies the search radius around each particle in the current frame for its possible locations in the next frame. This is a critical parameter for linking particle coordinates into trajectories but is initially unknown. To address this challenge, we used simulated trajectories at 0.03 particles/µm2 per frame as in Figure 1—figure supplement 1, which comprises a two-state system with diffusion coefficients at 0.1 and 1 µm2/s. We first synthesized trajectories using an unrealistically large connection distance threshold of 2000 nm, and examined the step size distribution of the resulting trajectories (A, main panel), where it became clear that the vast majority of the molecules moved less than 500 nm between frames. Based on this, we chose 400 nm, 500 nm, and 600 nm as the connection distance thresholds and resynthesized the trajectories (A, inset); the histograms essentially overlap except at the large step sizes (blue: 600 nm threshold; red: 500 nm threshold; and yellow: 400 nm threshold). (B–C) Comparison of the vbSPT model outputs on trajectories synthesized using the three connection distance threshold values shows that vbSPT was able to pick the correct model size (of 2) at all three settings. However, setting the threshold value at 400 or even 500 nm caused a noticeable truncation in the step size histogram (as shown in A) and resulted in lower diffusion coefficients for the fast state while the 600 nm threshold returned the correct diffusion coefficient; the slow state was not affected. Interestingly, the threshold setting had minimal impact on the resulting outputs for state occupancies (C). These settings were used to guide the trajectory synthesis based on the experimental spt-PALM data.
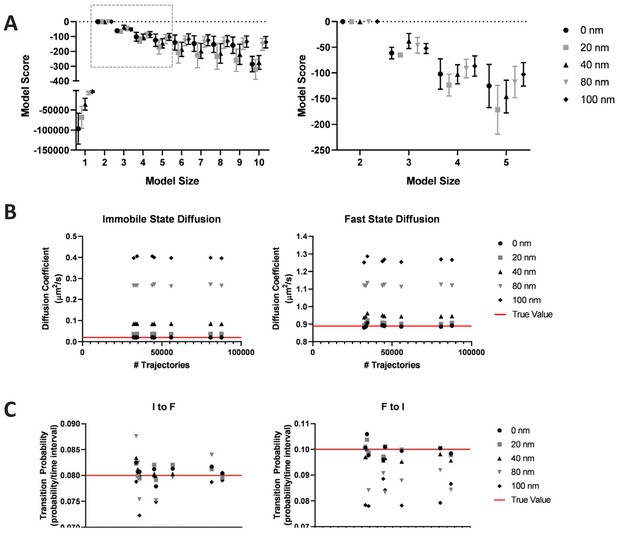
Impact of localization error on vbSPT model output.
To ensure that the three-state model was not a result of insufficient spatial precision, we have performed analysis on simulated trajectories based on a two state model comprising an immobile state (diffusion coefficient or D = 0.02 μm2/s) and a fast state (D = 0.89 μm2/s). Different levels of localization error (0 nm, 20 nm, 40 nm, 80 nm, and 100 nm) were added to the simulated trajectories and analyzed with vbSPT. The resulting model scores are shown in (A), where the right panel is the zoom-in view of the boxed area in the left panel; (B) and (C) show the impact of localization errors on the resulting diffusion coefficients and transition probability measurements, respectively.
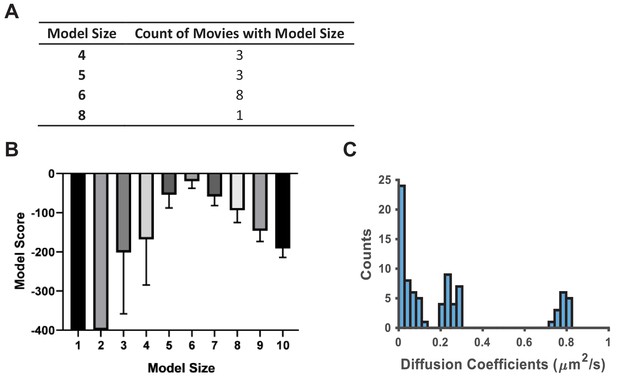
vbSPT model output on experimental spt-PALM datasets acquired at high particle densities.
When spt-PALM datasets of PAmCherry1-KRasG12D in U2OS cells were acquired at particle densities higher than 0.03 per μm2 per frame (typically around 0.05–0.1 per μm2 per frame), vbSPT outputs diffusion models with different model sizes, many reaching six or more states (A, B). However, a histogram of the diffusion coefficients of all detected states shows three clusters, indicating that a three-state model is still likely the best to recapitulate KRasG12D diffusion (C). Note that the three clusters are centered at diffusion coefficient values similar to those obtained with vbSPT or CDF analysis of spt-PALM datasets acquired at low particle densities (<0.03 particles per μm2 per frame) as in Figure 1F. All data were taken at 35 ms/frame.
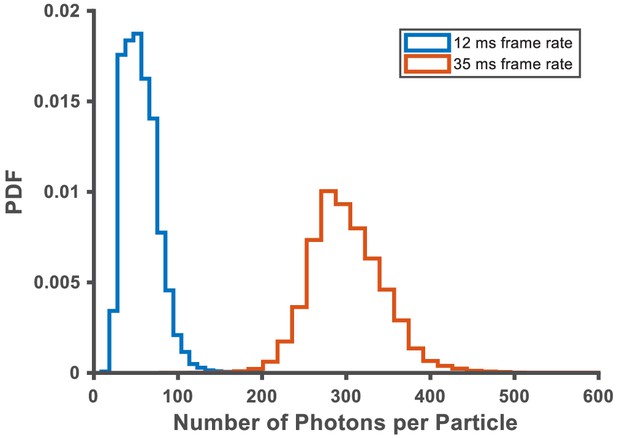
Photon yield and localization accuracy at the different frame rates used in this work.
Photon yields were calculated based on the integrated intensity above background across a 9 × 9 pixel area for each single-molecule image; the pixel intensity units were converted to the number of photons using hardware specific gain conversion factors. On average, the photon yield for single PAmCherry1 molecules at 12 ms and 35 ms frame acquisition time was ~56 photons and ~301 photons, corresponding to ~26 nm and ~10 nm localization precisions, respectively.
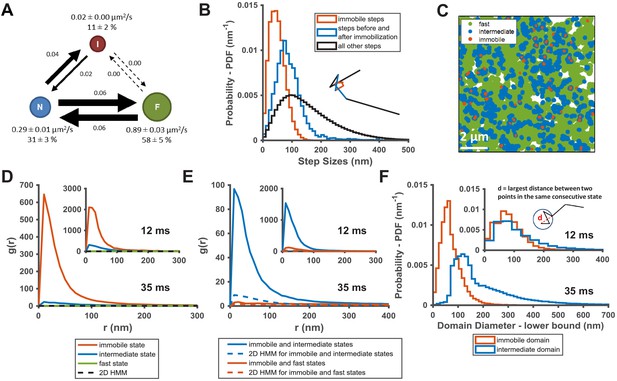
KRasG12D diffusion states are associated with distinct membrane domains.
(A) The three-state model for KRasG12D diffusion with F, N, and I, representing the fast, the intermediate, and the immobile states, respectively. Model parameters were inferred using vbSPT on spt-PALM datasets with at least 30,000 trajectories obtained on cells induced with 2 ng/mL Dox. The arrows indicate state transitions (i.e. the probability of switching to a different state in the next frame) and the area of the circle and the thickness of the arrows are both roughly scaled to reflect their relative values. All parameters were derived from data acquired at 12 ms frame interval except for the diffusion coefficient of the immobile state, which was inferred from data taken at 35 ms frame interval. Error bars are 95% CIs (n = 8); (B) Step size histograms for immobilization events (red), one step before or after the immobilization event (blue), and all other steps (black). A diffusion step was part of an immobilization event if immobile state was assigned to that trajectory segment by vbSPT (n = 14, see Materials and methods); (C) Map of the membrane locations where KRasG12D molecules exhibit specific diffusion states (referred to as state coordinates) within a one-minute duration (taken from a spt-PALM dataset of ~20 min total duration). Red, blue, and green dots represent locations of the immobile, the intermediate, and the fast states, respectively, with each rendered circles scaled proportionally to the mean diffusion coefficient for the state; (D) Pair correlation analysis on the averaged state coordinates across multiple, one-minute segments of longer spt-PALM datasets. The same color coding as in B) was used to distinguish the three states. For this analysis, molecules in the same diffusion state in successive frames only contributed a single, averaged state coordinate. The average state coordinates of all molecules captured within a one-minute segment were used for correlation analysis, and the results from multiple one-minute segments were averaged to yield the plot. The negative control was generated through a 2D Markovian simulation, and the resulting trajectories were analyzed the same as the experiment (see Materials and methods); (E) Cross correlation analysis between pairs of diffusion states. The state coordinates were processed the same way as in D) prior to the correlation analysis, except that the correlation was performed between two different diffusion states. The negative control was generated through a 2D Markovian simulation, and the resulting trajectories were analyzed the same as the experiment (see Materials and methods); (F) Estimating the lower bound size for the immobile and the intermediate domains. The estimation was based on the maximum distance traveled by the molecule while in the same diffusion state. *D-F) The main panel shows results inferred from data taken at 35 ms frame intervals for improved localization precision. The inset shows the data taken at 12 ms/frame (n = 14 for 12 ms and n = 7 for 35 ms datasets).
-
Figure 2—source data 1
Excel sheet for data used for generating panels B, D, E, and F.
Data for individual panels are included as separate tabs in the excel file.
- https://cdn.elifesciences.org/articles/46393/elife-46393-fig2-data1-v2.xlsx
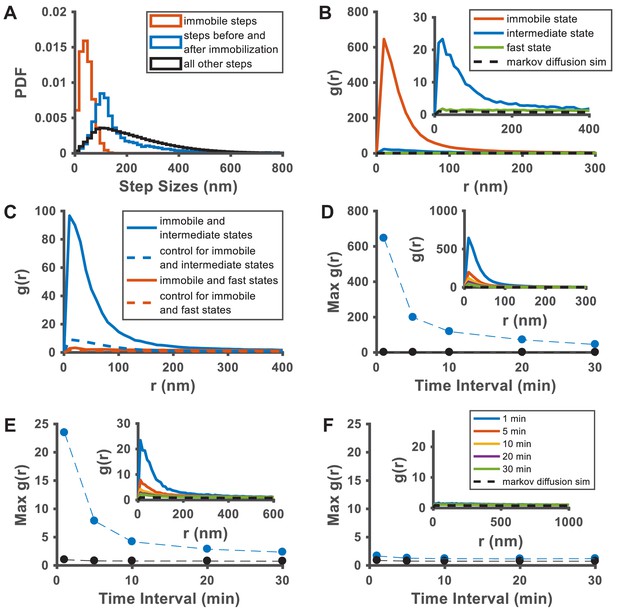
Spatial analysis of KRasG12D membrane domain properties using data acquired at 35 ms per frame.
As spt-PALM data acquired at 35 ms/frame showed better single-molecule localization accuracy than those at 12 ms/frame, we aimed to perform similar analysis of the domain properties to that shown in Figure 2 and 3 using data taken at 35 ms/frame (n = 7). (A) Step size histograms for the immobilization events (red), the steps directly before and after the immobilization events (blue), and all other steps (black); B) Pair correlation analysis on the averaged positions of the three states for one-minute temporal slices of the raw spt-PALM image stack (see Materials and methods), shows the same trend as observed with data taken at 12 ms/frame acquisition rate. Note the somewhat reduced spatial correlation for the intermediate domain (state) compared with that obtained with data taken at 12 ms/frame (Figure 2D); C) Cross-correlation analysis between the three membrane domains, performed on the same one-minute slices of the raw spt-PALM image stack; D–F) Peak amplitudes of autocorrelation g(r) at different time intervals. The steadily decreasing g(r) with increasing time intervals indicates the lifetimes of the immobile and the intermediate domains to be on the order of minutes.
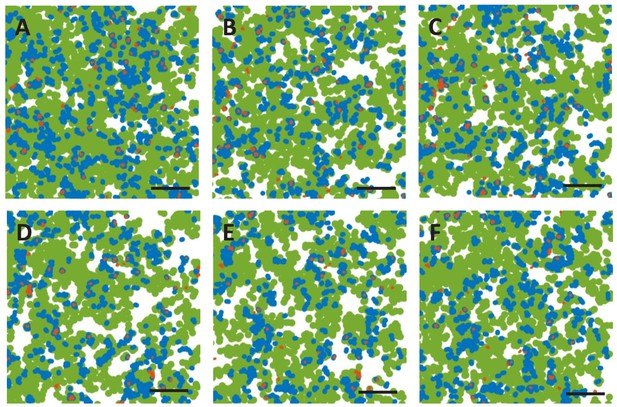
Temporal evolution of the membrane domains associated with each KRasG12D diffusive state.
The three membrane domains associated with the immobile, intermediate, and fast states of KRasG12D are labeled with red, blue, and green, respectively. The domain maps were generated using the same approach as described for Figure 2C (12 ms frame interval), with each panel representing the domain map within a 1 min duration with 0.5 min overlap. Thus, A-C represent total of 3.5 min time period. Of note, the maps were generated without position averaging, and therefore each trajectory contributes two or more points (including the beginning and the end) in the corresponding plot. Scale bars, 2 µm. See also Video 2.
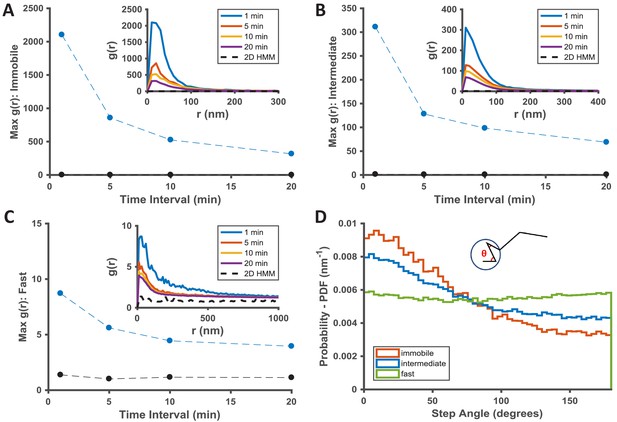
Temporal properties of the KRasG12D-associated immobile and intermediate domains.
(A–C) Pair correlation analysis of the state coordinates at different time intervals (1, 5, 10, and 20 min). The amplitude (maximal g(r) value) of the pair correlation function at each time interval was plotted in the main panel with the raw pair correlation plots shown in the inset. A-C show pair correlation functions of averaged coordinates for the immobile, the intermediate, and the fast states, respectively (see Materials and methods). The negative control in each case was generated through a 2D Markovian simulation, and the resulting trajectories were analyzed the same as the experiment (see Materials and methods); D) Deflection angle analysis on KRasG12D diffusion trajectories separated by diffusion states (red: immobile; blue: intermediate; green: fast). The deflection angle was calculated as the angle between two successive segments of the trajectory while the molecule was in the same diffusion state. *Results shown for data acquired at 12 ms/frame (n = 14).
-
Figure 3—source data 1
Excel sheet for data used for generating all the panels.
Data for individual panels are included as separate tabs in the excel file.
- https://cdn.elifesciences.org/articles/46393/elife-46393-fig3-data1-v2.xlsx
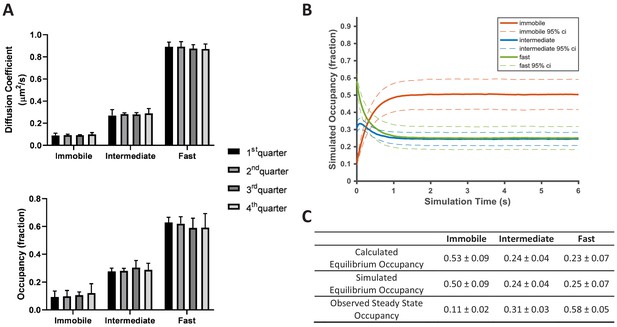
KRasG12D diffusion on the cell membrane is in a non-equilibrium steady state.
(A) Time invariance of the KRasG12D diffusion model. A single ~20 min spt-PALM dataset was segmented into four quarters with each quarter containing ~10,000 trajectories (in ~5 mins), each analyzed separately using vbSPT to obtain the model parameters such as the diffusion coefficients (upper panel) and the state occupancies (lower panel). Results from multiple spt-PALM datasets were grouped and plotted (n = 4); B) Temporal evolution of the KRasG12D diffusion model in simulated runs. The system was setup according to the experimental model parameters (number of states, state occupancies, diffusion coefficients, and state transition rates) as shown in Figure 2A. The system was then allowed to evolve based on the input, with the new state occupancies recorded every time step (12 ms) and plotted (see Materials and methods). Similar to Figure 2A, only movies with minimum of 30,000 trajectories were simulated (n = 8); C) Table summarizing the calculated, simulated, and experimentally observed occupancies for each of the states. *All error represents 95% CIs.
-
Figure 4—source data 1
Excel sheet for data used for generating all the panels.
Data for individual panels are included as separate tabs in the excel file.
- https://cdn.elifesciences.org/articles/46393/elife-46393-fig4-data1-v2.xlsx
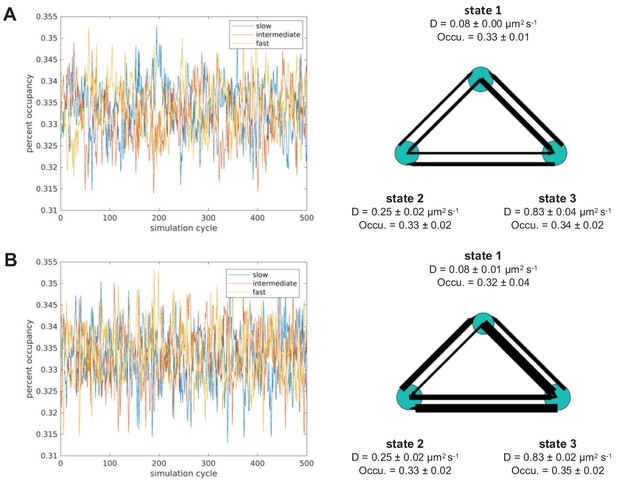
Validating vbSPT output accuracy on simulated trajectories using different model parameter inputs.
We simulated steady state systems using three states, with diffusion coefficients of 0.08, 0.26, 0.84 µm2/s and the same occupancy for each state (0.33). The state transition probabilities used for (A) were pii = 0.8 and pij = 0.1, which give rise to equal mass flow between each pairs of states; those used for (B) were p12 = p23 = p31 = 0.1 (counter-clockwise) and p13 = p32 = p21 = 0.8 (clockwise). Each simulation generated 5000 trajectories, which were then analyzed using vbSPT; each model was simulated five times, and the exemplary models with averaged model parameters are shown on the right. The resulting diffusion parameter outputs confirm that vbSPT was able to accurately determine parameters for both balanced (A, right) and non-balanced (B, right) state transitions. Error bars show 95% confidence interval.
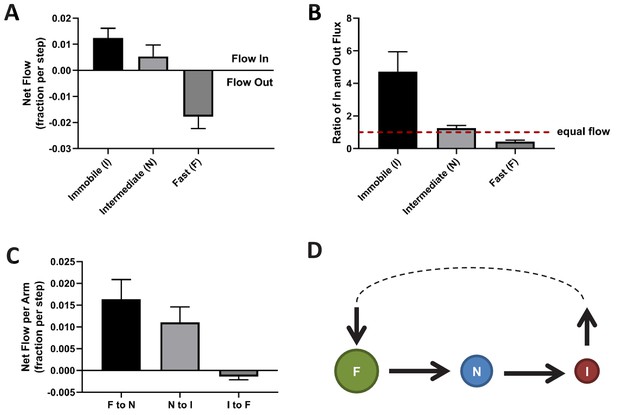
Directional mass flow between KRasG12D diffusion states.
(A) Net mass flow per state, defined as the difference between the influx (positive) and the outflux (negative) for each state and expressed as the fraction (of total KRasG12D population) entering (positive, flow in) or leaving (flow out, negative) the state per time interval; (B) Ratio of in- and outflux for each state. A ratio of one (dashed line) represents equal in- and outflux for the state, greater than one represents more influx than outflux, and less than one represents net outflux of mass from the state; (C) Net mass flow per arm (pair of states) in the KRasG12D diffusion model (Figure 2A). F to N and N to I are not significantly different. The states were ordered in a clock-wise direction, and the net mass flow in the direction was calculated as the difference between forward and backward mass flows, with a positive value indicating net flow in the indicated direction and a negative value the opposite direction; (D) Model for KRasG12D trafficking between the diffusion states and between the membrane system and the environment (cytosol). Arrows indicate the directional mass flow, and the dashed line represents unknown mechanisms connecting the fast and the immobile states. *All error bars are 95% CIs (n = 22).
-
Figure 5—source data 1
Excel sheet for data used for generating panels A, B, and C.
Data for individual panels are included as separate tabs in the excel file.
- https://cdn.elifesciences.org/articles/46393/elife-46393-fig5-data1-v2.xlsx
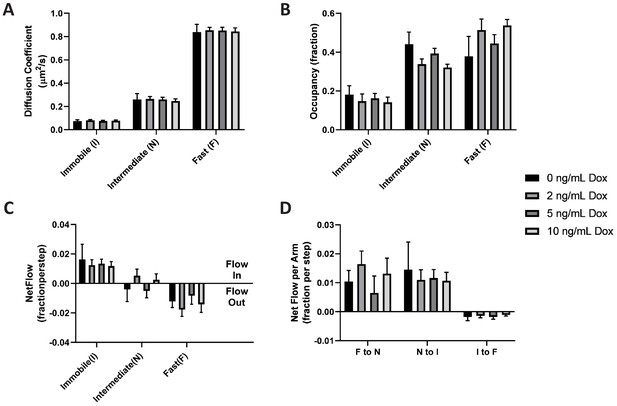
KRasG12D diffusion properties remain constant over a broad range of expression levels.
Spt-PALM trajectories of KRasG12D were acquired at 12 ms/frame after inducing the cells at 0, 2, 5, and 10 ng/mL Dox for 36–48 hr, and the diffusion models were inferred as described previously using vbSPT. All aspects of the diffusion model discussed earlier, including diffusion coefficients (A), state occupancies (B), net mass flow per state (C), and net mass flow per arm (pair of states, (D) at the different Dox concentrations were analyzed and compared. Error bars represent 95% CIs (n = 12 for 0 ng/mL Dox, n = 22 for 2 ng/mL Dox, n = 30 for 5 ng/mL Dox, and n = 18 for 10 ng/mL Dox).
-
Figure 6—source data 1
Excel sheet for data used for generating all the panels.
Data for individual panels are included as separate tabs in the excel file.
- https://cdn.elifesciences.org/articles/46393/elife-46393-fig6-data1-v2.xlsx
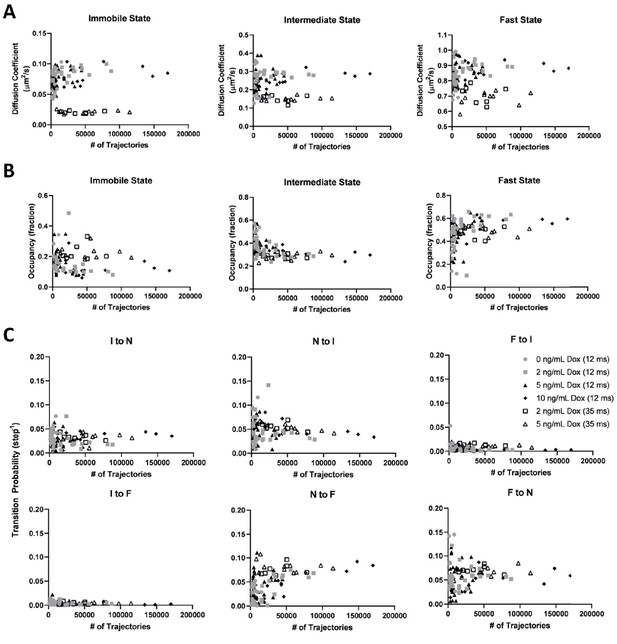
vbSPT model outputs from KRasG12D diffusion trajectories acquired at different conditions (frame rate, total number of trajectories, and Dox concentration).
All experimental spt-PALM data on PAmCherry1-KRasG12D (in U2OS cells) acquired with 12 or 35 ms frame acquisition times and under 0, 2, 5, or 10 ng/mL Dox concentrations were pooled (symbols as indicated), and vbSPT outputs of the diffusion coefficients (A), state occupancies (B), and state transition probabilities (C) were plotted against the total number of trajectories. As shown in (A) and (B), the diffusion coefficients and the occupancies typically converge relatively quickly at a few thousand trajectories. Additionally, the diffusion coefficients derived from datasets obtained at 35 ms/frame are consistently lower than those obtained with 12 ms/frame datasets, a result of both localization precision (particularly for the immobile state) and trajectory smearing (predominantly for the faster diffusive states). Transition probabilities (C) required more trajectories to converge. However, all model parameters converged at similar values regardless of Dox concentration (i.e., KRasG12D expression level). There are a total of 82 data points for the 12 ms dataset (n = 12 for 0 ng/mL Dox, n = 22 for 2 ng/mL Dox, n = 30 for 5 ng/mL Dox, and n = 18 for 10 ng/mL Dox), and 18 data points for the 35 ms frame rate (n = 7 for 2 ng/mL Dox and n = 11 for 5 ng/mL Dox).
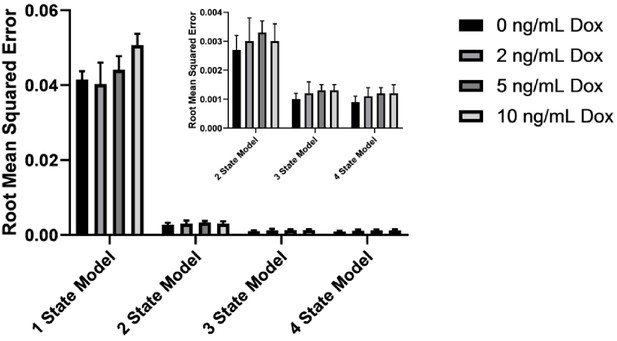
A three-state model remains optimal for describing KRasG12D diffusion over a broad range of expression levels.
The root mean squared error shown here is for CDF fitting of spt-PALM trajectories obtained at 0–10 ng/mL Dox (n = 12 for 0 ng/mL Dox, n = 22 for 2 ng/mL Dox, n = 30 for 5 ng/mL Dox, and n = 18 for 10 ng/mL Dox), with all trajectories acquired at optimal conditions (<0.03 particles/µm2 per frame and frame acquisition time 12 ms/frame). CDF fitting was used to fit data to one, two, three, and four state models, and the residual errors were calculated (as in Figure 1D).
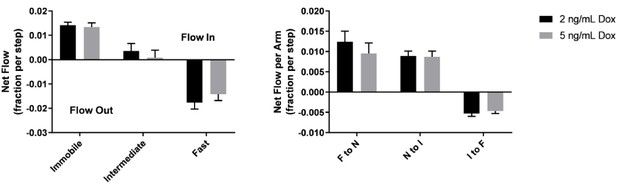
Net mass flow between KRasG12D diffusion states is independent of expression level.
Net flow analysis on datasets acquired at 35 ms/frame from cells induced to express PAmCherry1-KRasG12D at 2 or 5 ng/mL Dox.
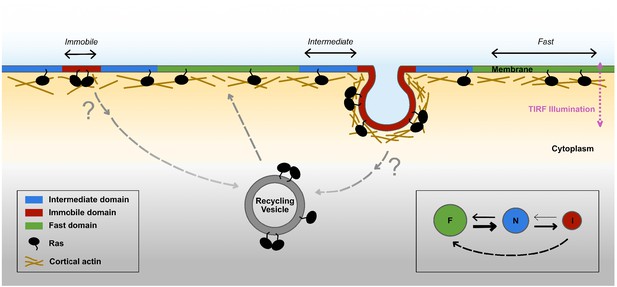
Proposed model for membrane nanodomains regulating KRasG12D mobility and trafficking.
For KRasG12D, the cell membrane comprises of at least three different compartments conferring each of the three diffusion states , namely the fast (and free), the intermediate, and the immobile diffusion states, depicted as green, blue, and red regions, respectively. The membrane compartments associated with the immobile and the intermediate states of KRasG12D are nanoscopic membrane structures. KRasG12D is continuously removed from the immobile state, some of which may be mediated via endocytosis. Internalized KRasG12D molecules are eventually transported back to the membrane as fast diffusing species through incompletely understood recycling processes. KRasG12D immobilization domains could locally enrich KRasG12D molecules to facilitate KRasG12D multimer formation and potentially signaling. The arrows in the inset (bottom right) indicate mass flows between each pair of states.
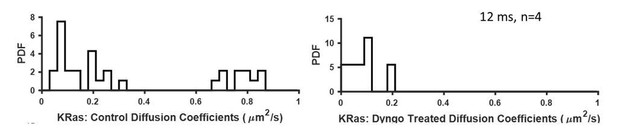
Effect of dynamin inhibition (with Dyngo 4a) on KRasG12D diffusion.
Shown in the plots were diffusion constants for all detected states by vbSPT. Untreated cells (left) were used as control (similar to that shown in Figure 1—figure supplement 5C). Right, U2OS-PAmCherry1-KRasG12D cells were treated with Dyngo 4a (50 µM) for 30 min prior to spt-PALM and imaged for another 30 min before switching to a new sample.
Videos
Clip of a raw spt-PALM video showing PAmCherry1-KRasG12D diffusion on U2OS cell membrane.
Data acquired at 35 ms/frame. Cells were induced with 5 ng/mL doxycycline before imaging.
Time-lapse (1 min/frame) video of the domain map calculated from individual trajectories within each substack.
Red: immobilization domains (sites); blue: intermediate domains; green: fast (free) domains. The image area is around 10 × 10 μm2.
Tables
Reagent type (species) or resource | Designation | Source or reference | Identifiers | Additional information |
---|---|---|---|---|
Cell line (Homo-sapiens) | U2OS | ATCC | RRID: CVCL_0042 ATCC Cat#: HTB_96 | Parent cell line for generating U2OS-tetR |
Cell line (Homo-sapiens) | U2OS-tetR | This paper. | RRID: CVCL_XZ88 | Single U2OS clone stably expressing the tet repressor (tetR). |
Cell line (Homo-sapiens) | U2OS-tetR PA-mCherry1-KRas G12D | This paper. | RRID: CVCL_XZ89 | Single U2OS cell clone stably expressing PAmCherry1-KRasG12Dunder Doxycycline regulation |
Antibody | anti-RAS (mouse monoclonal) | Abcam | RRID: AB_941040 Abcam Cat#: ab55391 | Mouse monoclonal antibody |
Antibody | anti-beta-Tubulin (mouse monoclonal) | Thermo Fisher | RRID: AB_86547 ThermoFisher Cat#: 32–2600 | Mouse monoclonal antibody |
Software, algorithm | vbSPT | Perssonet al. | RRID: SCR_017554 | Algorithm for extracting diffusion parameters from SPT data |
Software, algorithm | μManager | Invitrogen | RRID: SCR_000415 | Micro-manager open source microscopy platform |