Coverage-dependent bias creates the appearance of binary splicing in single cells
Figures
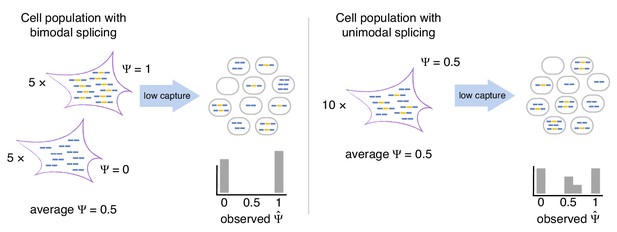
Bimodal vs unimodal models of cassette exon splicing.
In the bimodal model, some cells consistently splice in the exon, while others consistently skip it. After mRNA capture and sequencing, observations of are almost exclusively binary. In the unimodal model, individual cells express some mRNAs that splice in the cassette exon and some that skip it. Low mRNA capture dramatically reduces the number of cells in which both isoforms are observed, artificially inflating binary values.
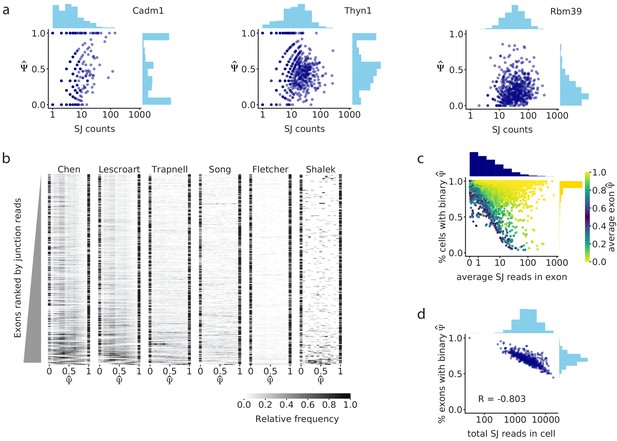
Splice junction read coverage is correlated with unimodality of splicing distributions.
(a) Comparison of splice junction read coverage and observed for three cassette exons in the Chen dataset, with low (Cadm1 exon 8; chr9: 47829377–47829409), medium (Thyn1 exon 6, chr9: 27006801–27006951), and high coverage (Rbm39 exon 3; chr2: 156178880–156178952). Each dot represents the of that exon in one cell. (b) distribution of the 300 highest coverage cassette exons with intermediate splicing (average between 0.2 and 0.8) in each of the six analyzed datasets. Each row in the heatmap shows the distribution of for one exon across all cells. (c) Relationship between the average read coverage and proportion of binary observations for each cassette exon in the Chen dataset. (d) Correlation between the total number of splice junction reads captured in each cell, and proportion of cassette exons with intermediate splicing that show binary in that cell.
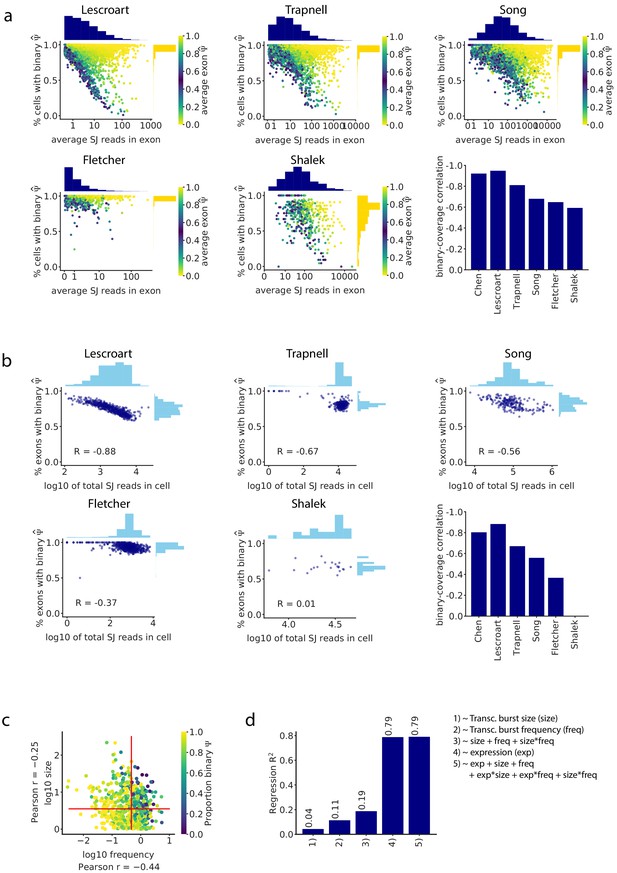
Junction read coverage determines the proportion of binary observations in all analyzed datasets.
(a) As in Figure 2c, the splice junction read coverage of an intermediate exon was anti-correlated with the proportion of cells in which it shows binary (only one isoform is observed) in all datasets. Barplot: Pearson correlation between read coverage and proportion of binary observations of each cassette exon. (b) As in Figure 2d, the total number of splice junction reads in a cell was inversely proportional to the fraction of exons that have binary values in the cell in all datasets. Barplot: Pearson correlation between the total number of captured reads in each cell and the proportion of cassette exons with binary . (c) Relationship between transcriptional burst frequency and size, and the proportion of binary observations of an exon. (d) of the linear regression prediction of the proportion of binary observations per exon based on burst frequency, size, number of reads, and a combination of these features.
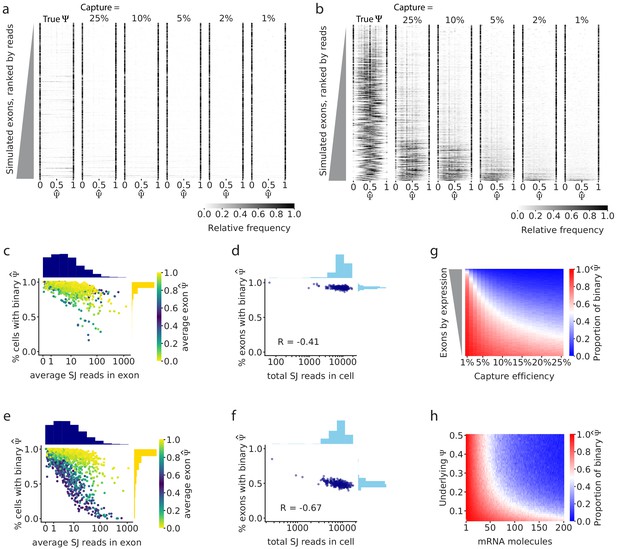
Simulations show that gene expression and capture efficiency influence the observed distribution of splicing.
(a) Simulations of alternative splicing and scRNA-seq under the binary-bimodal model, in which each cell produces one isoform or the other, but rarely both. As in Figure 2b, each row of the histogram shows for one intermediate exon across all cells. The observed distribution is similar to the true distribution, and its shape is largely unaffected by capture efficiency. (b) Simulations with the non-binary, unimodal model, in which most cells present a mixture of the two alternative isoforms. Exons with high expression have a unimodal distribution of true . Low capture efficiency results in an increase in binary observations (only one isoform observed), leading to a distortion of the observed distribution of to look bimodal. Only a handful of the highest expressed exons maintain a unimodal distribution of . Fewer exons show unimodal splicing as the capture efficiency is reduced. (c) Under the binary-bimodal model, exons with high coverage have slightly fewer binary observations, and (d) simulated cells with a high number of total splice junction reads have slightly fewer exons with binary . (e) Under the unimodal model, exons with intermediate splicing show a strong decrease in binary observations as coverage increases, as seen in real data (Figure 2c). (f) Similarly, simulated cells with high read coverage have a decrease of the proportion of binary . (g) Effect of capture efficiency on the proportion of binary observations of cassette exons with underlying = 0.5. (h) Effect of the initial number of mRNA molecules and underlying on the proportion of binary observations.

Theoretical calculations and simulations of the effect of biological and technical factors in splicing observations.
(a) Theoretical likelihood of capturing only mRNAs representing one isoform of an alternatively spliced gene in a single cell, determined by the total number of mRNAs in the cell, the of the isoforms, and a 10% capture efficiency. (b) Probability of observing a binary (only one isoform observed) given the number of mRNA molecules of that gene present in the cell, when the underlying is fixed at 0.5. (c) Effect of the number of captured mRNA molecules on the uncertainty of observations. is understood as the maximum likelihood estimate of the underlying . (d) The posterior probability of having an observed within 0.1 of the underlying . (e) Distribution of the relative error between the average observed , and the average true , in our simulations under different capture efficiency rates. The relative error is calculated as the difference between the average and the average true , scaled by the average true . (f) Distribution of the relative error between the variance in the observed , and the variance in the true , in our simulations under different capture efficiency rates.
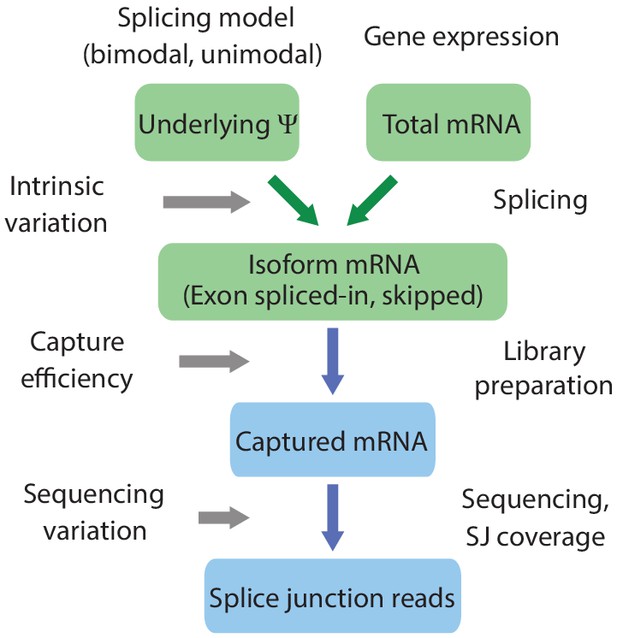
Schematic of scRNA-seq splicing simulator.
Simulator of scRNA-seq splicing data. Green elements represent biological variables; blue elements represent technical processes. The underlying is drawn from a Beta distribution with either a bimodal or unimodal shape. Individual gene expression determines the total number of mRNAs, and these mRNAs are spliced stochastically according to , producing isoforms that splice in or skip the exon. mRNAs are captured with a probability drawn from a normal distribution. Sequencing produces splice junction reads, which determine the observed .
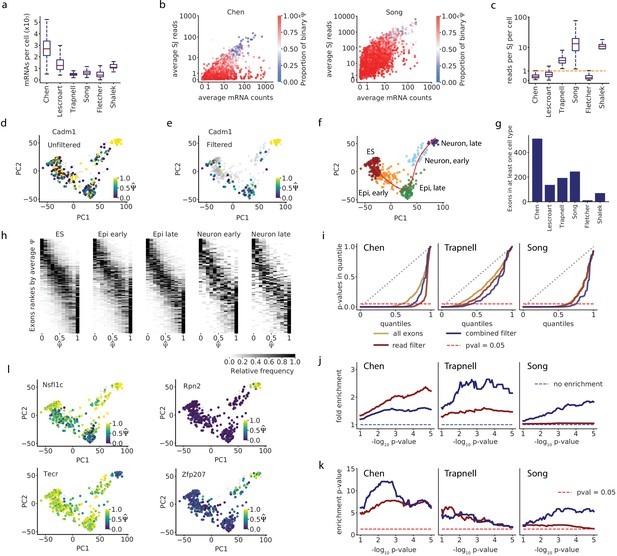
Accounting for coverage biases reveals unimodal splicing distributions and differential splicing.
(a) Estimated total mRNA molecules captured per cell. (b) Estimated number of recovered mRNAs vs splice junction reads for cassette exons, averaged across cells. Each dot corresponds to an exon, and its color indicates the proportion of cells in which it has a binary observation (only one isoform observed). We analyzed exons with average between 0.05 and 0.95. (c) Per-cell splice junction coverage rate in each dataset. (d) Cadm1 exon 8 alternative splicing appears binary in many cells in the Chen dataset. Correlation with lineage pseudotime: Spearman’s = 0.1. (e) Cadm1 exon after removing cells with fewer than 10 recovered Cadm1 mRNA molecules and fewer splice junction reads than expected from 10 mRNAs (grey). Spearman’s = 0.52. (f) PCA projection and clustering of single cells in the Chen dataset, showing differentiation of mouse ES cells into neurons. Red line, lineage inferred with Slingshot. (g) Number of cassette exons with observations from at least 10 mRNA reads in at least 50% of cells in any cluster. (h) Stacked histograms showing the distribution of observed of exons as in (g), in each cell cluster of the Chen dataset. Observations with fewer than 10 mRNA molecules were removed. We show exons with average ranging from 0.1 to 0.9 per cluster. (i) QQ-plot comparing the quantiles of a uniform distribution (x-axis) with the quantiles of the distributions of p-values from the Kruskal-Wallis test (y-axis). A diagonal line (gray dotted line) would mean the p-values are uniformly distributed. A lower area under the curve indicates enrichment for low p-values. The point on the x-axis at which each line crosses the dotted red line indicates the proportion of p-values that are below 0.05 in the distribution. (j) Fold enrichment of exons with a Kruskal-Wallis p < in the set of exons selected with the mRNA-based filter (blue), and exons selected with a flat read minimum filter (red). (k) Significance p-value of the enrichment, estimated with the hypergeometric test and adjusted for FDR. (l) Example exons that pass the overall filter criteria in the Chen dataset and have p<0.05 in the Kruskal-Wallis test.
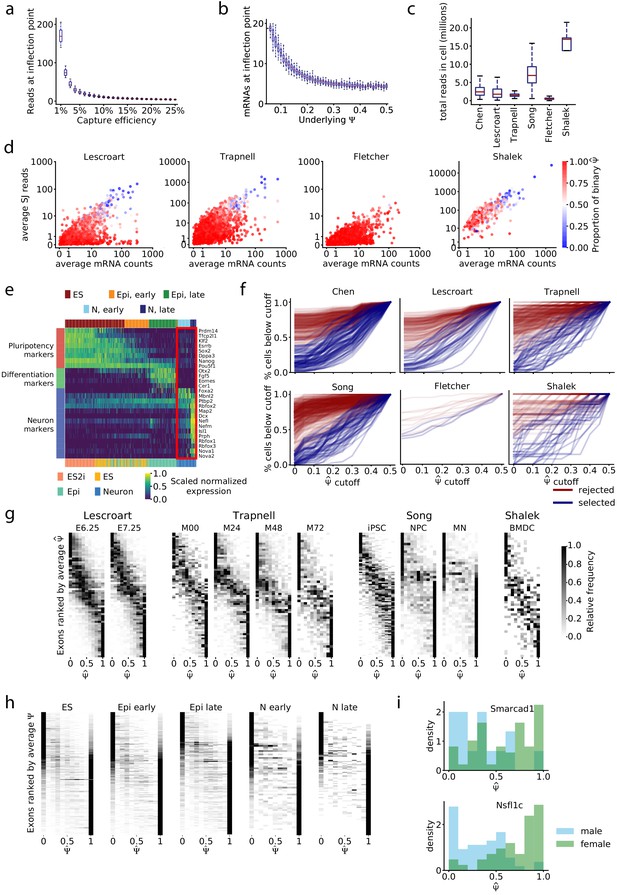
Relationship between read coverage, captured mRNAs, and binarity in single cell datasets.
(a) Average number of splice junction reads required to have a 50% likelihood of observing both isoforms, from simulations in Figure 3j. (b) Total mRNAs required to have a 50% likelihood of observing both isoforms, from simulations in Figure 3k. (c) Total mapped reads per cell for each dataset. (d) Estimated average number of recovered mRNAs vs. average number of splice junction reads for each exon. (e) Expression of pluripotency and neuron differentiation marker genes in the Chen dataset; expression is scaled by each row’s maximum and minimum. Cells are ordered by inferred pseudotime. Top color key, clusters from agglomerative clustering; bottom color key, labels from Chen et al. The red box highlights two sub-groups of neurons identified by clustering. (f) Visualization of the distribution of observations toward extreme values in the first cluster of cells of each dataset. For each cutoff in the x-axis, the y-axis corresponds to the proportion of cells that have or . Blue lines, exons passing the mRNA-based filter; red lines, exons removed by filter. (g) Stacked histograms, as in Figure 4h, for other datasets. The Fletcher dataset is excluded because only five exons pass the mRNA filter. (h) Stacked histograms of exons that do not pass the filter in the Chen dataset; exons were subsampled to match the number that do pass the filter in each cell type cluster. (i) Sex-dependent distribution of of the two bimodal exons of the Chen dataset after cell-type stratification: Smarcad1 exon 3 (chr6: 65043836–65044108) in early Epi cells, Nsfl1c exon 4 (chr2: 151502455–151502460) in late Epi cells.
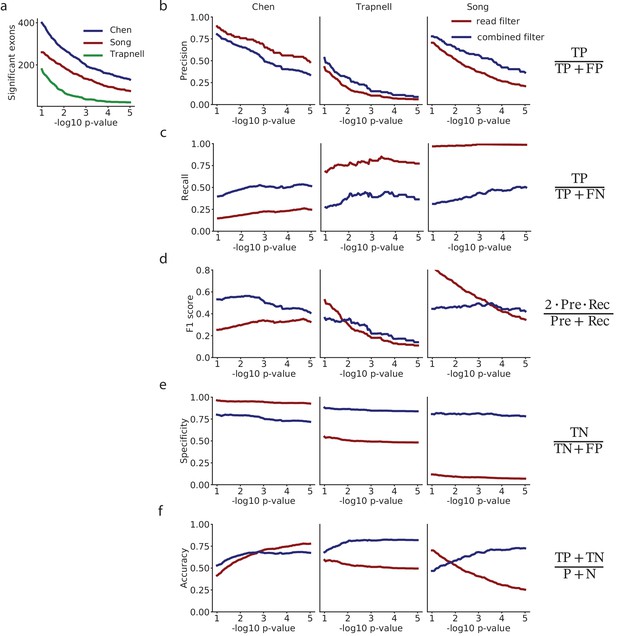
Analysis of differential splicing among selected exons with the Kruskal-Wallis analysis of variance.
(a) Comparison of the precision of selecting exons with low p-values, for the mRNA-based filter versus selecting exons on a flat minimum of 10 reads. (b) Recall. (c) F1-score. (d) Specificity. (e) Accuracy. (f) Number of exons with in each dataset.
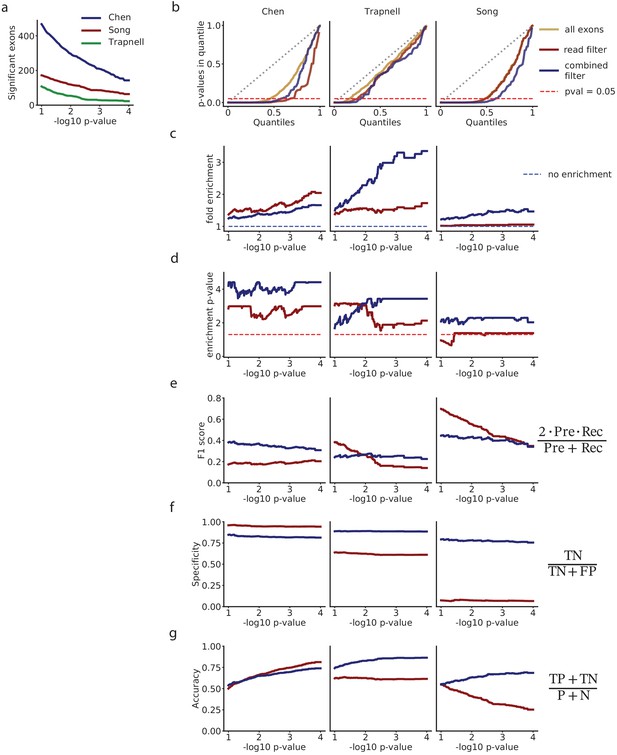
Analysis of differential splicing among selected exons with the autocorrelation test.
(a) Number of exons with p in each dataset. (b) QQ-plot comparing the quantiles of a uniform distribution, with the quantiles of the distributions of p-values from the autocorrelation test. (c) Fold enrichment of exons with an autocorrelation p < in the set of exons selected with the mRNA-based filter (blue), and exons selected with a flat read minimum filter (red). (d) Significance p-value of the enrichment shown in (c). For each p-value limit , we estimate the significance with the hypergeometric test, and correct for multiple testing using the Benjamini-Hochberg FDR adjustment. (e) F1-score. (f) Specificity. (g) Accuracy.
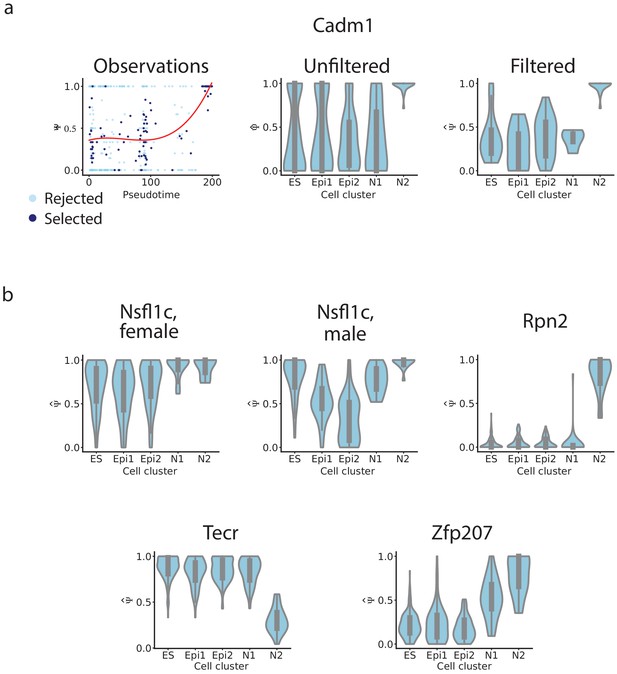
Distribution of of example exons.
(a) Skipped Cadm1 exon 8 (chr9: 47829377–47829409). We show the relationship between pseudotime and observed ; as well as violinplots of the distribution of in each cluster before filtering, and after filtering. (b) Violinplots of the distribution of in each cluster after filtering for the exons in Figure 4k: Nsfl1c exon 4 (chr2: 151502455–151502460), Rpn2 exon 16 (chr2: 157323223–157323270), Tecr exon 4 (chr8: 83573411–83573455), Zfp207 exon 8 (chr11: 80393084–80393176).
Tables
Prevalence of bimodal splicing distributions before and after filtering.
Dataset | Cell type cluster | Cells | Exons | Bimodal | % bimodal | Selected | Selected bimodal | % bimodal selected | p-val (adj) |
---|---|---|---|---|---|---|---|---|---|
Chen | ES | 217 | 446 | 118 | 26% | 94 | 0 | 0% | 3.3e-14 |
Chen | Epi, early | 98 | 402 | 107 | 27% | 98 | 1 | 1% | 9.3e-14 |
Chen | Epi, late | 104 | 516 | 136 | 26% | 76 | 1 | 1% | 1.1e-09 |
Chen | Neuron, early | 47 | 364 | 117 | 32% | 43 | 0 | 0% | 3.6e-08 |
Chen | Neuron, late | 22 | 517 | 146 | 28% | 61 | 0 | 0% | 1.1e-09 |
Lescroart | Heart E6.75 | 172 | 286 | 77 | 27% | 33 | 0 | 0% | 2.0e-05 |
Lescroart | Heart E7.25 | 341 | 291 | 78 | 27% | 36 | 0 | 0% | 8.0e-06 |
Trapnell | Myoblast 00 hr | 35 | 400 | 142 | 36% | 41 | 0 | 0% | 1.2e-08 |
Trapnell | Myoblast 24 hr | 89 | 251 | 97 | 39% | 27 | 0 | 0% | 1.2e-06 |
Trapnell | Myoblast 48 hr | 72 | 242 | 97 | 40% | 32 | 1 | 3% | 9.1e-07 |
Trapnell | Myoblast 72 hr | 35 | 252 | 101 | 40% | 24 | 1 | 4% | 5.1e-05 |
Song | iPSC | 62 | 616 | 269 | 44% | 55 | 0 | 0% | 3.3e-14 |
Song | NPC | 73 | 212 | 92 | 43% | 28 | 1 | 4% | 1.2e-06 |
Song | MN | 67 | 168 | 82 | 49% | 19 | 4 | 21% | 9.4e-03 |
Shalek | BMDC | 13 | 149 | 51 | 34% | 27 | 1 | 4% | 6.6e-05 |
Single-cell RNA-seq datasets.
Dataset | Organism | Biological process | Cells | No. ASE | Mean reads/event | Protocol | Accession | Reference |
---|---|---|---|---|---|---|---|---|
Chen | mouse | mES neuron differentiation | 488 | 3276 | 3.1 | Smart-seq2 | GSE74155 | Chen et al., 2016 |
Lescroart | mouse | cardiomyogenesis | 598 | 3007 | 3.2 | Smart-seq2 | GSE100471 | Lescroart et al., 2018 |
Trapnell | human | skeletal myogenesis | 314 | 4457 | 14.4 | Smart-seq | GSE52529 | Trapnell et al., 2014 |
Song | human | iPS motor neuron differentiation | 206 | 5355 | 88.8 | Smart-seq | GSE85908 | Song et al., 2017 |
Fletcher | mouse | olfactory neurogenesis | 849 | 684 | 2.7 | Smart-seq | GSE95601 | Fletcher et al., 2017 |
Shalek | mouse | bone-marrow-derived dendritic cells | 18 | 380 | 213.4 | Smart-seq | GSE41265 | Shalek et al., 2013 |