Protein Evolution: Building bigger beta-barrels
You would have a difficult time today if you were looking for a cooper to make you a bespoke wooden barrel. But if you were lucky enough to find one, you would likely tell them the desired height, girth and shape of your new barrel. They would then disappear into a workshop and painstakingly create a series of long wooden boards to serve as the staves – each with precise dimensions, curvature and beveling of edges to meet your specifications – and a few days later you would be the proud owner of a new, one-of-a-kind wooden barrel.
While coopering is an ancient trade with a thousand years of history, nature has been building barrel-shaped proteins for even longer. Today, as protein engineering advances to the point where we hope to build synthetic, bespoke molecular barrels for a range of applications, it will be essential to understand how nature evolved barrels and learn the tricks of the trade.
Building barrels from wood or from amino acids presents different challenges. Let us say that you find your barrel is too small and you would like to make the opening at the top wider. You would be dismayed to learn that because the dimensions of the wooden staves are unique to that design, your cooper cannot simply add more staves to make the opening wider – a new barrel would need to be built. In contrast, biology creates new protein forms by tweaking existing ones using the tools of genetic variation: mutation, duplication and recombination.
Barrel-shaped proteins called OMBBs (which is short for outer-membrane beta-barrels) are found in the outer membranes of gram-negative bacteria, with beta strands playing the role of staves. These OMBBs always contain an even number of strands, with each pair adding a fraction of a nanometer to the diameter of the barrel opening: the smallest OMBBs have just eight beta strands and the largest we know of contain 26 (Franklin et al., 2018b). By varying the number of beta strands they have, natural OMBBs can dictate the transit of proteins and molecules through them based on size. The ability to similarly adjust the dimensions of synthetic barrels by adding or removing strands would enable researchers to design and build structures called nanopores that could, for example, be used to sequence DNA, or to sense chemicals in the environment with high selectivity and sensitivity (Trick et al., 2014).
OMBBs are repeat proteins – a diverse class of proteins that consist of two or more copies of a simpler structural unit, which is thought to be a 'beta hairpin' (that is, a structure in which two beta strands are joined together at one end to create a structure shaped like a hairpin; Remmert et al., 2010). The repetitive nature of these structures, combined with the exclusion of water in the membrane in which they are embedded, reduces the complexity of their amino acid sequences. While less complexity may sound like a good thing, it makes it more difficult to use phylogenetic methods to unravel the evolutionary histories and relationships of the beta-barrels found in nature today. Now, in eLife, Joanna Slusky of the University of Kansas and colleagues – including Meghan Franklin as first author – report how they have combined sequence and structural information on over 50,000 homologs of OMBBs to explore the evolutionary origins of these proteins (Franklin et al., 2018a).
The results are surprising. Rather than growing linearly from eight strands to 12 or more, the evolutionary road appears to have been replete with forks and alternate paths. Instead, transitions occur through unexpected mechanisms such as loop-to-strand conversions or the duplication of certain protein domains. In related work, the same team recently reported that certain classes of OMBBs, specifically lysins and efflux pumps, evolved independently of the remainder of OMBBs (Franklin et al., 2018b). Taken together, these results suggest that the emergence of OMBBs with large barrels happened through multiple pathways (Figure 1).
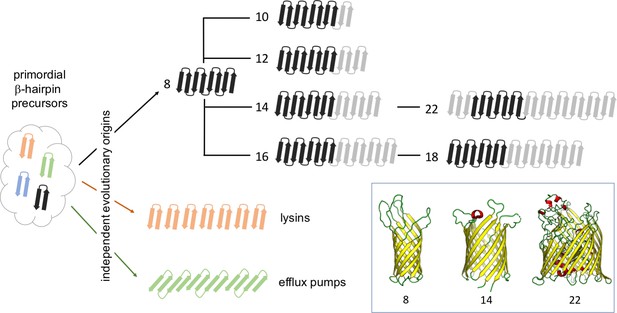
The evolutionary history of beta-barrels.
Starting from ancestral pools of β-hairpins, each made of two beta strands (left), the evolution of outer-membrane beta-barrels (OMBBs) containing between eight and 26 strands is thought to have occurred independently multiple times. Beta-barrels containing 10 or more strands evolved as a result of a hairpin duplication at the N-terminus of eight-strand barrels, and it is thought that the folding of these proteins occurs at the C-terminus. The strands in OMBBs form a single continuous chain, and the dark black arrows show strands that span the membrane in which the beta-barrel is embedded; OMBBs with 24 and 26 strands are not shown. Lysins and efflux pumps are examples of multi-chain beta-barrels that evolved independently of single-chain OMBBs and of each other. The inset shows the three-dimensional structures of OMBBs with eight, 14 and 22 beta strands.
Another long-standing puzzle is how OMBBs fold into their final three-dimensional structure. OMBBs face challenges on two fronts: the constraints that apply to the folding of all repeat proteins (Björklund et al., 2006; Wright et al., 2005); and the need to coordinate folding with the insertion of the protein into a membrane (Fleming, 2014). Franklin et al. noted that elements of the original eight-strand sequence have persisted at the C-terminus of larger barrels, suggesting that this region might be responsible for the initial stages of protein folding. Moreover, previous efforts to design even modest variants of an eight-strand barrel were largely unsuccessful (Stapleton et al., 2015): this is consistent with the eight-strand barrels that are observed in nature evolving to have a central role in the folding of OMBBs. This suggests that the best way to engineer larger barrels is to focus on adding new strands to the N-terminus of an eight-strand OMBB.
In exposing the complex evolutionary history of OMBBs, Franklin et al. have given us insights into how nature builds complex proteins from simpler parts. Hopefully, coopers working at the molecular scale can learn from natural evolution and identify new rules for successfully engineering synthetic barrels.
References
-
Expansion of protein domain repeatsPLOS Computational Biology 2:e114.https://doi.org/10.1371/journal.pcbi.0020114
-
Energetics of membrane protein foldingAnnual Review of Biophysics 43:233–255.https://doi.org/10.1146/annurev-biophys-051013-022926
-
Evolution of outer membrane beta-barrels from an ancestral beta beta hairpinMolecular Biology and Evolution 27:1348–1358.https://doi.org/10.1093/molbev/msq017
Article and author information
Author details
Publication history
- Version of Record published: January 21, 2019 (version 1)
Copyright
© 2019, Nanda
This article is distributed under the terms of the Creative Commons Attribution License, which permits unrestricted use and redistribution provided that the original author and source are credited.
Metrics
-
- 1,989
- views
-
- 187
- downloads
-
- 5
- citations
Views, downloads and citations are aggregated across all versions of this paper published by eLife.
Download links
Downloads (link to download the article as PDF)
Open citations (links to open the citations from this article in various online reference manager services)
Cite this article (links to download the citations from this article in formats compatible with various reference manager tools)
Further reading
-
- Evolutionary Biology
The RNA world hypothesis proposes that during the early evolution of life, primordial genomes of the first self-propagating evolutionary units existed in the form of RNA-like polymers. Autonomous, non-enzymatic, and sustained replication of such information carriers presents a problem, because product formation and hybridization between template and copy strands reduces replication speed. Kinetics of growth is then parabolic with the benefit of entailing competitive coexistence, thereby maintaining diversity. Here, we test the information-maintaining ability of parabolic growth in stochastic multispecies population models under the constraints of constant total population size and chemostat conditions. We find that large population sizes and small differences in the replication rates favor the stable coexistence of the vast majority of replicator species (‘genes’), while the error threshold problem is alleviated relative to exponential amplification. In addition, sequence properties (GC content) and the strength of resource competition mediated by the rate of resource inflow determine the number of coexisting variants, suggesting that fluctuations in building block availability favored repeated cycles of exploration and exploitation. Stochastic parabolic growth could thus have played a pivotal role in preserving viable sequences generated by random abiotic synthesis and providing diverse genetic raw material to the early evolution of functional ribozymes.
-
- Ecology
- Evolutionary Biology
Seasonal animal dormancy is widely interpreted as a physiological response for surviving energetic challenges during the harshest times of the year (the physiological constraint hypothesis). However, there are other mutually non-exclusive hypotheses to explain the timing of animal dormancy, that is, entry into and emergence from hibernation (i.e. dormancy phenology). Survival advantages of dormancy that have been proposed are reduced risks of predation and competition (the ‘life-history’ hypothesis), but comparative tests across animal species are few. Using the phylogenetic comparative method applied to more than 20 hibernating mammalian species, we found support for both hypotheses as explanations for the phenology of dormancy. In accordance with the life-history hypotheses, sex differences in hibernation emergence and immergence were favored by the sex difference in reproductive effort. In addition, physiological constraint may influence the trade-off between survival and reproduction such that low temperatures and precipitation, as well as smaller body mass, influence sex differences in phenology. We also compiled initial evidence that ectotherm dormancy may be (1) less temperature dependent than previously thought and (2) associated with trade-offs consistent with the life-history hypothesis. Thus, dormancy during non-life-threatening periods that are unfavorable for reproduction may be more widespread than previously thought.